Automate legal research, eDiscovery, and precedent analysis - Let our AI Legal Assistant handle the complexity. (Get started for free)
Unveiling the Anatomy of Scams Key Identifiers in AI-Assisted Contract Reviews
Unveiling the Anatomy of Scams Key Identifiers in AI-Assisted Contract Reviews - AI-Generated Voice Cloning Techniques in Contract Fraud
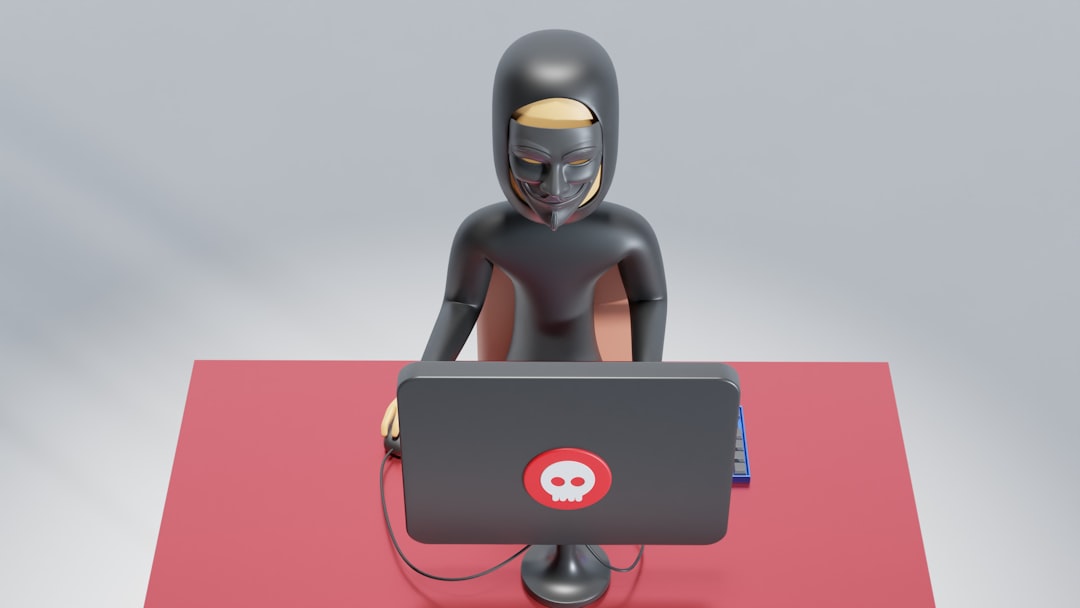
The ability of artificial intelligence to replicate human voices with remarkable accuracy has introduced a new dimension to contract fraud. Scammers are exploiting voice cloning technology to impersonate individuals involved in contractual agreements, manipulating them into making decisions or divulging sensitive information. This tactic has prompted organizations like the Federal Trade Commission to address the issue head-on. Initiatives such as the Voice Cloning Challenge emphasize the need for finding ways to combat this threat. The ease with which AI can clone voices – sometimes with only a few seconds of audio – makes these scams increasingly difficult to detect. As voice cloning technology continues to advance, the authenticity of spoken interactions becomes more challenging to verify. This presents a significant threat, necessitating greater public awareness and proactive measures to protect individuals and organizations from this evolving type of fraud. The continuous development of more realistic-sounding AI voices further complicates the problem. It underscores the urgency for organizations, consumers, and regulatory bodies to stay informed and equipped to combat the evolving landscape of AI-driven contract fraud.
The increasing sophistication of AI-driven voice cloning presents a significant threat to contract integrity. These techniques leverage deep learning models to meticulously capture and recreate a person's voice, producing incredibly realistic synthetic audio. Worryingly, only a brief audio snippet, potentially as short as a few seconds, can be sufficient for algorithms to generate a convincing voice clone.
These clones can now reproduce not just the basic voice characteristics but also intricate details like individual speaking patterns, hesitations, and emotional nuances, making it challenging for the human ear to detect any artificiality. This makes it particularly difficult to rely solely on audio evidence in legal contexts involving contracts. Furthermore, the ability to generate entire conversations, mimicking typical dialogue and speech habits of a specific person, amplifies the risk. Scammers can craft deceptive conversations during contract negotiations or reviews to mislead or defraud others.
The combination of voice cloning with other AI-driven tools, like text generation, further complicates the problem. It enables scammers to design scripts that seem natural and tailored to the victim, increasing the likelihood of success in social engineering scams. This evolution of voice cloning raises serious ethical concerns, as malicious actors can leverage this technology for identity theft, falsely creating an aura of authority in contractual agreements.
Our existing legal systems are struggling to effectively address this rapidly advancing threat. Traditional fraud and identity theft laws aren't equipped to deal with the complex nuances of synthetic voice misuse in contractual disputes. Unfortunately, current forensic techniques have shown a low success rate in detecting AI-generated voices, underscoring the urgent need for improved methods of authentication and detection within the legal arena. This evolving landscape necessitates the development of more robust tools and approaches to ensure the authenticity and reliability of audio evidence, especially in the crucial context of contract reviews and enforcement.
Unveiling the Anatomy of Scams Key Identifiers in AI-Assisted Contract Reviews - Image Manipulation and Deepfakes in Rental Agreement Scams
The use of manipulated images and deepfakes in rental agreement scams is a growing concern. Criminals are using sophisticated AI to create fake images and videos of landlords or properties, effectively constructing fraudulent identities that can lure unsuspecting renters. These scams can involve forged photos of properties, or even manipulated videos of a supposed landlord, making it difficult to differentiate truth from falsehood. While some deepfakes might have telltale signs of poor quality, the technology is becoming more advanced, making detection challenging. These scams can lead to substantial financial losses for victims who unknowingly enter into agreements with fake entities. The ease with which deepfake technology can be deployed, combined with its growing sophistication, highlights the urgent need for individuals to be educated about these threats and for the development of more sophisticated methods to detect them. It's crucial for renters to be vigilant and employ skepticism when encountering such materials in the rental process. The increasing prevalence of these AI-powered scams poses a significant threat to the integrity of the rental market, and proactive measures are essential to address this issue.
AI-powered image manipulation, including deepfakes, is becoming a major problem in rental scams. These tools, often built using neural networks, can make extremely realistic alterations to images with relatively little effort. This makes it easy to create fraudulent IDs or rental agreements, which can be tricky to verify. Deepfakes take this a step further by creating visuals and audio that closely mimic real people. Scammers use this to create trust, making victims more likely to fall for rental scams.
The speed at which generative adversarial networks (GANs) are improving is alarming. GANs can create not just realistic photos but also videos that could convincingly fake interactions, making it even harder to spot the fraud. In the world of rental scams, this translates to scammers modifying photos of properties, often making them look better than they are in real life to trick people into paying deposits.
Research shows that it can be tough for even experts to tell the difference between real and fake images. This makes the average person even more vulnerable, as they may not have the skills to spot manipulated images. Online platforms where people rent properties can sometimes be part of the problem because they don't always have good processes for checking images, which lets scammers slip through the cracks.
Image manipulation often goes hand in hand with social engineering. Scammers might use fake photos of themselves or create fake documents in online chats to appear more credible during rental discussions. This tactic increases their chances of being believed. The widespread availability of high-quality smartphone cameras makes it much easier for scammers to take photos and manipulate them quickly, creating convincing scams in a matter of seconds.
There's also a useful feature of photos called metadata, which can sometimes highlight inconsistencies if looked at carefully. But many people don't check it, giving scammers a head start if their manipulated images look real at first glance. The laws surrounding online fraud aren't keeping up with the sophistication of deepfake technology. This creates problems when it comes to prosecuting those responsible when the evidence is based on manipulated images and sounds. There's a clear need for better legal structures and methods to help prevent and prosecute these types of scams.
Unveiling the Anatomy of Scams Key Identifiers in AI-Assisted Contract Reviews - Advanced Phishing Tactics Using AI-Powered Chatbots
The integration of AI-powered chatbots into the world of phishing has ushered in a new era of sophisticated scams. These chatbots enable attackers to automate and scale phishing campaigns like never before, targeting individuals with personalized messages designed to manipulate them. This approach leverages social engineering principles, using AI to mimic human communication in a way that can be surprisingly persuasive.
One of the most concerning aspects of this development is the ability of these chatbots to interact with multiple victims concurrently. This expands the reach and efficiency of phishing attacks compared to the traditional methods, creating a more significant threat. Furthermore, AI chatbots can carry on conversations that appear indistinguishable from those initiated by real people, potentially leading victims to divulge sensitive information or click on malicious links without suspicion.
The effectiveness of these new AI-driven phishing methods raises significant concerns. Individuals who are typically well-versed in cybersecurity practices may still find it difficult to identify these sophisticated scams. This underlines the urgent need for increased awareness and the development of new strategies to combat this evolving threat. As AI technology continues to advance, the threat landscape will likely continue to evolve, necessitating a proactive approach to safeguard individuals and organizations against these increasingly complex attacks.
The integration of AI-powered chatbots in phishing attacks has significantly amplified both the volume and sophistication of these scams. These chatbots can now mimic human interactions with incredible accuracy, making it difficult to differentiate between genuine and fraudulent communications. They achieve this by employing social engineering tactics, crafting personalized messages based on information gleaned from data breaches and social media profiles. This personalization makes the scams appear much more authentic and targeted, tricking people into trusting them.
What's perhaps more worrisome is that these chatbots aren't static. They can learn from past interactions. If one approach fails to deceive a user, the chatbot can adapt and try a different tactic in the next attempt. This learning capability allows scammers to continuously refine their approach, potentially increasing their success rate over time. Moreover, AI-generated text often passes through traditional security filters, because it mimics natural language so well. This makes them harder to detect using standard anti-phishing methods.
These chatbots aren't just limited to text-based interactions either. They are able to incorporate elements like audio and images, making the experience appear even more realistic. The ability to weave together different communication styles adds a layer of complexity, blending the lines between real and fabricated interactions. Furthermore, the chatbots can establish a sense of urgency in their messages, pushing people to act quickly and potentially without proper critical thinking. This type of psychological manipulation is a well-known technique in traditional scams, and now it's being amplified by AI.
The automation afforded by chatbots enables scammers to run 24/7 phishing operations. They can launch numerous attacks simultaneously, inundating their targets with messages and making it harder to stay alert and catch the fraud. This shift is concerning as it can reduce the barrier to entry for scammers. Individuals with minimal technical knowledge can now effectively conduct highly sophisticated phishing attacks by simply leveraging readily available AI tools.
A troubling consequence is that existing laws and cybersecurity measures are struggling to adapt quickly enough to counter these new techniques. Regulations, often focused on combating conventional phishing methods, struggle to keep up with the evolving capabilities of AI. Even worse, some of these attacks don't merely impersonate individuals. They can effectively replicate official communications from companies, making it nearly impossible for victims to discern a genuine request from a scam. This highlights the need for more thorough verification and due diligence in situations where authenticity matters.
The rise of AI-powered chatbots in phishing represents a significant challenge in cybersecurity. The combination of learning abilities, personalization, and automation creates a more sophisticated threat landscape. It's clear that vigilance and critical thinking are crucial in protecting yourself from these scams. And as the technology evolves, the importance of adapting security practices and regulatory structures becomes more pressing.
Unveiling the Anatomy of Scams Key Identifiers in AI-Assisted Contract Reviews - Detecting Anomalies in AI-Generated Contract Language
As AI-generated content becomes more prevalent in contract drafting, the ability to detect anomalies in this language becomes increasingly important. AI's capacity to produce text can sometimes lead to outputs that deviate from expected patterns and norms. This raises concerns about the reliability and quality of AI-generated contracts, potentially impacting their integrity.
For example, AI-generated contract language might exhibit inconsistencies in terminology, unusual phrasing, or a style that clashes with established standards for contractual agreements. These inconsistencies can create confusion, and potentially weaken the legal standing of a contract. This emphasizes the need for thorough reviews of AI-generated contracts to ensure they meet the standards required for legal validity and to mitigate potential risks.
Unfortunately, there's currently a lack of specialized tools designed to detect these anomalies. This gap hinders the ability to quickly and effectively verify the authenticity and compliance of AI-assisted contract generation. There is a need for further development and research in this area, as it is a critical aspect of ensuring the responsible and reliable use of AI in legal and contractual situations.
The rapid evolution of AI's ability to generate human-like text, particularly in areas like contract drafting, makes it harder to rely on traditional methods for spotting manipulated or fraudulent content. AI language models are now able to create not only standard legal phrasing but also subtly alter word choices in ways that may escape cursory review. This raises concerns about how easily legal experts can pinpoint abnormalities in these contracts.
Sometimes, AI-generated contract language includes seemingly logical but internally inconsistent terms or clauses. While designed to appear coherent, these contradictions often betray a fundamental misunderstanding of complex legal principles by the AI.
It's becoming apparent that systems trained on historical contract data, while capable of identifying patterns, can also absorb and propagate unintended biases present in the original data. This leads to potentially unfair or flawed terms in newly generated contracts.
AI-generated scams might employ authentic legal language and references to real statutes, but they can manipulate these elements to misrepresent the law, effectively deceiving individuals into believing they are signing fair agreements.
Researchers have observed that some AI-generated contracts exhibit peculiar formatting inconsistencies. These may include misplaced punctuation or inconsistencies in bullet points, which, although seemingly trivial, can act as indicators of a lack of human review in the contract drafting process.
Unlike traditional contract scams, the use of AI can involve the creation of plausible, entirely fabricated scenarios cleverly interwoven into seemingly valid agreements. These fictional situations can introduce harmful clauses deep within the contract, making detection far more challenging.
While research is being done on how to use machine learning for detecting anomalies, current approaches are still in their early stages. Many of the existing models haven't proven very effective at differentiating between AI-generated and human-written legal texts.
It's also worth noting that many of the established cybersecurity defenses against contract fraud operate on a sort of "zero-sum game" principle. Implementing protections against one type of fraud can, paradoxically, introduce vulnerabilities to others, especially when AI is involved in creating both legitimate and fraudulent content.
AI-powered contract review tools are also prone to false positives. Software might erroneously flag valid contract language as problematic while simultaneously failing to detect subtle manipulations used for deception. This points to the crucial need for review processes that combine both AI and human expertise.
The growing use of AI in contract generation highlights the need for continuous vigilance and adaptation in detecting anomalies. The technology is continually evolving, presenting new challenges that require careful consideration and the development of more sophisticated methods to ensure the integrity and fairness of legal documents.
Unveiling the Anatomy of Scams Key Identifiers in AI-Assisted Contract Reviews - The Role of Machine Learning in Identifying Fraudulent Signatures
Machine learning (ML) has become increasingly important in the detection of fraudulent signatures. It leverages its ability to analyze complex patterns and subtle anomalies in data that might not be readily apparent to humans. ML techniques, such as deep learning and neural networks, are particularly well-suited to process vast quantities of data and detect irregularities that might indicate fraudulent activity. The accuracy and reliability of these ML-powered systems depend heavily on the quality of the data they are trained on. Furthermore, ML methods like ensemble learning, combining various models, can create a more comprehensive assessment of a signature's authenticity. While this automated approach offers significant improvements in efficiency and the capacity to scale, it's crucial to acknowledge that fraud techniques constantly evolve. This necessitates a continuous refinement of the ML models to stay ahead of the curve and ensure continued effectiveness in fraud prevention.
Machine learning is increasingly vital in spotting fake signatures by examining subtle patterns and irregularities in the way people sign their names. These are often hard for humans to see. AI's ability to detect fraud in finance has raised the bar, and this is now being applied to verifying signatures.
Techniques like deep learning and neural networks help process lots of signature data to identify patterns that hint at forgery. However, the quality of the data used to train these models is crucial. Good machine learning models rely on having many examples of real and fake signatures to learn from. Gathering this data, especially with a variety of different people and writing styles, can be a challenge.
Some machine learning approaches focus on analyzing the way a person signs, like how hard they press, how fast they move the pen, and the angle of the strokes. This adds a depth of understanding that just comparing pictures can't provide. There are also methods that look for specific traits in the signature like the angles, curve types, and connections between parts, often pinpointing over 40 unique features.
We're seeing significant progress in deep learning algorithms, which can now differentiate between authentic and fake signatures with accuracy rates over 99% in some cases. The ability to validate signatures instantly during transactions is quite useful. It speeds things up compared to older approaches that involved a lot of manual checks.
Machine learning algorithms are particularly adept at noticing outliers. They can use unsupervised learning methods to flag signatures that don't follow the usual patterns. This helps find possibly fraudulent signatures that a person might miss.
Even things like the pen and paper used when someone signs can affect the signature itself. Luckily, machine learning can be taught to factor these things in, which improves how reliably it can verify signatures.
The neat thing is that you can hook machine learning-based signature verification systems into other tools meant to detect fraud. This way you can have a more robust defense against various types of fraud in situations where multiple identifiers are used for verification.
As people's handwriting and signing habits evolve over time, machine learning can adapt to these changes. Systems can be designed to learn new 'true' signatures and thus maintain their accuracy.
The legal ramifications of using machine learning for signature authentication are still being worked out. As this technology gets more popular, we may see legal systems needing to update what's considered a valid and reliable signature verification method. It will be interesting to see how the law adapts to the changing landscape of AI-driven authentication.
Unveiling the Anatomy of Scams Key Identifiers in AI-Assisted Contract Reviews - Blockchain Integration for Enhanced Contract Verification
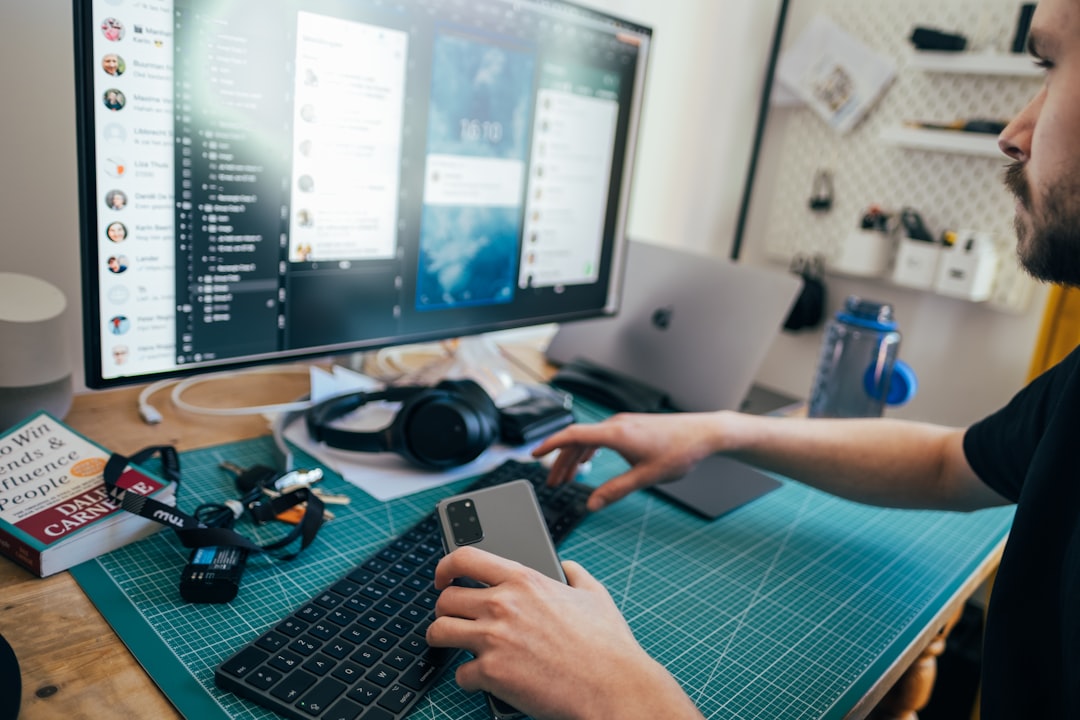
Blockchain technology, when integrated into contract verification processes, offers a compelling pathway to establish greater trust, especially in the realm of AI-assisted contract reviews. By leveraging smart contracts, the technology can automate the verification process, ensuring that contracts are genuine. However, this added layer of security can introduce complications such as potential delays in development and implementation due to the associated costs. Research suggests that the combination of blockchain and deep learning could play a valuable role in identifying potentially harmful schemes hidden within contracts, such as Ponzi schemes. This approach, however, calls for advancements in how we extract key features that signal such issues. While the prospect of a more secure and transparent contract environment is attractive, hurdles exist. Building systems that can effectively address the constantly evolving strategies of those involved in contract fraud remains a significant challenge. The confluence of blockchain and AI holds the promise of significantly improving the integrity of contracts in the evolving digital space, but realizing this potential will require ongoing innovation and refinement.
Blockchain's inherent ability to create an unchangeable record of transactions gives it a unique role in enhancing contract verification. When a contract is stored on a blockchain, it can't be altered without everyone involved agreeing. This makes it very hard for anyone to tamper with a contract after it's been signed, greatly reducing the chances of fraudulent activity.
Smart contracts, which are programs that run on a blockchain and automatically enforce contract terms, are also interesting. These reduce the need for middlemen, which can streamline things and minimize the opportunities for errors or fraud that can occur when a third party is involved.
The transparency of blockchain is useful for contract monitoring. Anyone can see a complete history of changes to a contract, so it's like a public audit trail. This adds to the trust between people involved in the contract, as everyone can see what has happened.
For areas where traditional notarization is slow and complicated, blockchain might provide a better solution. Digital signatures on a blockchain can serve as a type of legally recognized digital notary, potentially allowing people to do contract signing remotely.
Because of the cryptographic techniques used in blockchains, only people who are supposed to have access to contract data can see or change it. Each transaction is encrypted and needs agreement from several nodes in the network to be validated. This makes it practically impossible to change a contract without detection.
AI-based contract review tools are still working on understanding legal complexities, and integrating them with blockchain could help. Blockchain can provide a foundation for truth, helping to verify that contracts are legitimate and haven't been tampered with.
Blockchains also allow for continuous monitoring of contracts. The process of checking if contracts are being followed as agreed can be automated, which is unlike traditional, periodic manual audits. This can potentially detect any problems or signs of fraud quickly.
The decentralized nature of blockchain means that no single person or organization has full control over contract data. This helps prevent corruption or manipulation of contracts. Because everyone has equal access to the history of the contract, it makes everyone more accountable for their actions.
Studies have shown that people are more comfortable with contracts that use blockchain for verification because they believe it lowers the risk. This could make contracts more trustworthy and influence how businesses that heavily rely on contracts function.
While it has a lot of potential, some people in the traditional legal field are hesitant to adopt blockchain for contract verification. They may not understand how it works or how it can prevent fraud. More education and clear examples of how blockchain can fight fraud might be needed for more widespread acceptance.
Automate legal research, eDiscovery, and precedent analysis - Let our AI Legal Assistant handle the complexity. (Get started for free)
More Posts from legalpdf.io: