Tata Elxsi Employee Ruling Spotlights AI's Growing Influence in Legal Discovery and Labor Rights
Tata Elxsi Employee Ruling Spotlights AI's Growing Influence in Legal Discovery and Labor Rights - AI's expanding footprint in electronic discovery processes
Artificial intelligence continues to deepen its integration into electronic discovery processes within the legal sector as of mid-2025. This evolution fundamentally alters how legal professionals, particularly in larger firms, manage the often overwhelming volume of digital information pertinent to litigation. The technology is being leveraged to accelerate tasks like document review and the initial identification of potentially relevant materials, aiming to free up human capacity for higher-level strategic work rather than exhaustive manual analysis. While the promised gains in efficiency and potential cost reduction are significant drivers, this reliance on algorithmic processes also raises critical questions. Concerns persist regarding the transparency and potential biases embedded within these tools, which could inadvertently affect the scope or fairness of discovery. Furthermore, the increasing automation necessitates an ongoing examination of the implications for traditional legal roles and the broader labor landscape within the profession, prompting a necessary dialogue about ensuring the integrity and ethical application of these evolving capabilities.
Here are a few observations about AI's increasing presence in legal electronic discovery workflows and related processes:
1. Systems leveraging artificial intelligence are consistently demonstrating the capability to identify potentially relevant electronic documents with reported accuracy rates often exceeding what might be typically expected from conventional linear human review processes, though the efficacy still relies heavily on careful model training and iterative refinement.
2. Tools powered by AI are extending their reach beyond just document sorting, analyzing extensive corpora of legal precedent and historical litigation data to provide statistical insights or 'predictions' about potential case trajectories, influencing strategic considerations derived from discovery findings.
3. Initial experimentation shows AI models are being applied to generate drafts of more standardized legal texts, including certain discovery requests or basic components of filings, potentially streamlining tasks that previously consumed significant human drafting time, freeing up practitioners for more analytical work.
4. The capacity of large language models and other AI techniques to scan enormous datasets, such as employee communications or internal records gathered during discovery, to detect non-obvious patterns or anomalies indicative of systemic issues, like potential discrimination or collusion, offers a scale of analysis beyond traditional methods.
5. Automation driven by AI is increasingly integrated into managing data sensitivity within discovery, assisting with tasks like identifying and redacting personal or confidential information to help meet evolving data privacy obligations, and assessing the compliance footprint of large datasets targeted for collection and review, albeit requiring robust validation and oversight.
Tata Elxsi Employee Ruling Spotlights AI's Growing Influence in Legal Discovery and Labor Rights - Navigating discovery challenges in labor law matters with AI tools
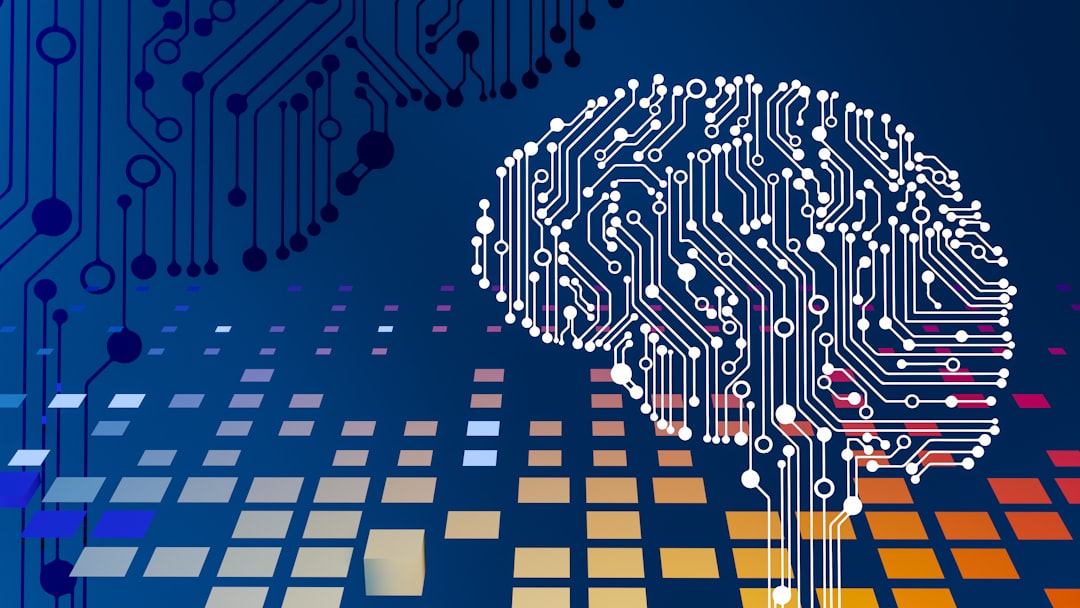
AI technologies are increasingly being brought to bear on the complex discovery processes inherent in labor law disputes. Given the often sensitive nature and high volume of electronic data involved – from internal communications to policy documents – these tools are seen as potentially useful for sifting through information. However, deploying artificial intelligence in this arena introduces distinct challenges. Concerns around algorithmic bias are particularly acute in the labor context, where biased systems could inadvertently disadvantage employees or employers by misidentifying relevant evidence or perpetuating discriminatory patterns present in the training data. Ensuring genuine transparency in how these tools select, categorize, or flag information is difficult but vital. As legal practitioners and firms incorporate AI into navigating labor discovery, continuous, critical evaluation of the tools' impact on fairness and their adherence to principles safeguarding labor rights is imperative. The drive for operational improvements must be balanced carefully against the potential for unintended negative consequences on the substantive rights of parties involved.
Navigating discovery challenges in labor law contexts presents a complex landscape where the integration of advanced computational tools is becoming increasingly apparent. As of mid-2025, several distinct applications of artificial intelligence are being explored or deployed to grapple with the specific data characteristics and strategic demands of these disputes.
Algorithmic systems are being engineered to perform iterative analysis on incoming document streams, allowing data-driven feedback to inform the ongoing refinement of search parameters and data ingestion criteria. This adaptive capacity is intended to enhance the precision of identification in fast-evolving labor disputes, attempting to focus human review effort on potentially high-value information streams rather than relying solely on static rule sets.
The application of advanced natural language processing techniques is increasingly leveraged to detect subtle but potentially significant shifts in communication patterns within extensive datasets. The hypothesis is that these nuanced changes in language usage might signal evolving power dynamics or coordinated behaviors relevant to labor rights contexts, providing a granular layer of analytical observation often missed by traditional keyword searching or linear review, though interpreting the significance of such patterns remains a human task.
In the context of large-scale labor litigation involving vast digital footprints from numerous individuals, statistically driven predictive coding models represent a key application area. Their reported efficacy in identifying document relevance across massive corpuses with reduced human review time is a significant factor, potentially altering resource allocation in managing discovery burdens inherent in complex, multi-party employment actions compared to manual review paradigms.
The technical exploration extends beyond traditional text analysis to encompass other data modalities frequently encountered in labor contexts, including metadata trails and multimedia formats. Capabilities under development or in pilot phase include algorithmic processing of audio transcripts to analyze spoken language cues and the potential for pattern recognition within visual data like surveillance footage – aiming to cross-reference or provide alternative data points against testimonial accounts, albeit with inherent challenges in contextual interpretation.
Efforts are underway to automate routine, high-volume discovery tasks such as the algorithmic generation of draft privilege log entries or preliminary summaries for document categories identified as potentially sensitive or confidential. While requiring stringent human validation and oversight, the goal is to offload repetitive classification and documentation processes associated with managing privileged or protected information, balancing the need for thoroughness with the practicalities of scale in large datasets.
Tata Elxsi Employee Ruling Spotlights AI's Growing Influence in Legal Discovery and Labor Rights - Ethical considerations for AI use in evidence collection and analysis
As artificial intelligence tools are increasingly deployed in the critical functions of gathering and analyzing evidence for legal proceedings, a significant ethical dimension demands careful attention. The shift towards relying on algorithmic processes to manage and interpret potentially case-critical information introduces fundamental questions about fairness, transparency, and accountability. Ensuring that these automated systems operate free from biases that could unfairly impact outcomes, particularly in disputes involving sensitive personal or employment data, is paramount. Furthermore, the ability to comprehend *why* an AI flagged certain evidence or drew a specific conclusion is crucial for maintaining trust and the integrity of the legal discovery process. Upholding justice in the age of AI necessitates a commitment from legal practitioners and the wider system to rigorously evaluate these tools, address their limitations, and establish clear ethical standards to prevent technological capabilities from undermining fundamental rights.
From an engineering viewpoint, the application of artificial intelligence within the process of collecting and analyzing electronic evidence, particularly in fields like labor law discovery, brings a fascinating set of technical and ethical puzzles. As of mid-2025, here are some observations on these considerations:
1. While regulatory bodies are pushing for increased insight into how AI models operate, achieving genuine algorithmic transparency in complex systems used for document classification or relevance ranking in e-discovery remains a significant technical hurdle. Explaining the precise "reasoning" behind a model's output for millions of documents is inherently difficult, even with post-hoc analysis tools, creating a gap between regulatory ambition and current AI capabilities.
2. Efforts to build 'fairer' AI are ongoing, with research exploring techniques like generative adversarial networks (GANs) or differential privacy during model training to mitigate biases potentially embedded in historical legal data. However, these methods are not silver bullets; they introduce complexity, can sometimes reduce model performance, and fundamentally rely on assumptions about what constitutes "fairness," which itself can be context-dependent and hard to define mathematically for legal outcomes.
3. Addressing the privacy concerns when training AI models on sensitive legal data involves exploring decentralized architectures. Techniques like federated learning allow models to be trained locally on disparate datasets within law firms without centralizing or directly sharing the raw confidential information. This offers a privacy advantage but presents engineering challenges related to model convergence, communication overhead across networks, and ensuring consistent model quality across diverse data distributions.
4. AI is pushing the boundaries of legal research by attempting to map and analyze the intricate logical relationships between legal precedents rather than just retrieving relevant keywords or documents. Systems are being designed to identify supporting arguments, potential counterarguments, and even analyze patterns in judicial language, but capturing the full nuance of legal reasoning and the subtleties of judicial interpretation in code is a complex, ongoing task with inherent limitations.
5. There appears to be a conscious effort in system design within legal AI to frame the technology as an augmentation tool for legal professionals rather than a replacement. From a user interface and workflow perspective, this means designing tools that facilitate human oversight and validation of AI outputs, particularly in areas with high ethical stakes like evidence analysis, acknowledging that critical judgment and ultimate ethical responsibility reside with the human practitioner.
Tata Elxsi Employee Ruling Spotlights AI's Growing Influence in Legal Discovery and Labor Rights - The role of emerging AI regulations in shaping legal discovery
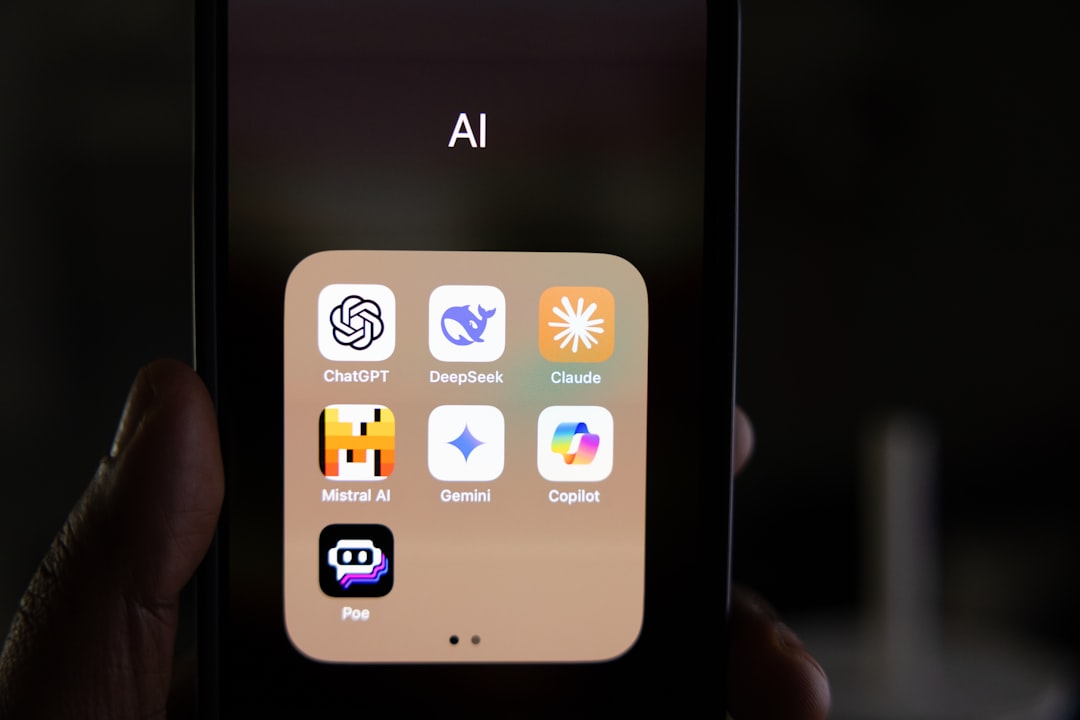
The increasing integration of artificial intelligence into legal workflows is undeniably being shaped by emerging regulations, which are proving crucial in addressing the fundamental ethical and accountability concerns tied to AI's use in processes like discovery, where accuracy and fairness are paramount. As AI tools are applied in document review and relevance analysis, the developing regulatory frameworks aim to impose requirements for greater transparency and mitigation of algorithmic bias, a critical step for safeguarding the integrity of discovery and preventing potential harm, particularly in matters impacting labor rights and equitable treatment. Navigating this complex intersection of technology and law requires continuous critical evaluation of AI's function in discovery, always considering the evolving regulatory landscape, to ensure public confidence and maintain the pursuit of justice.
Emerging AI regulations are actively influencing the landscape of legal discovery processes, prompting shifts that are intriguing from a technical standpoint as of May 22, 2025.
1. Regulatory emphasis on transparency is inadvertently stimulating demand for specialized technical consultants who can audit and document the operational mechanics of AI models used in document review or analysis. This stems from courts and regulators increasingly expecting legal professionals to articulate *how* an AI arrived at a particular outcome, a requirement that often exceeds the typical lawyer's technical expertise and the inherent opacity of complex machine learning systems.
2. The growing legislative focus on algorithmic fairness is driving changes in how AI models are being trained for legal applications. There's a noticeable move away from solely relying on historical legal data, which can perpetuate societal biases. Instead, researchers and developers are exploring data augmentation techniques and debiasing algorithms, grappling with the complex engineering challenge of computationally defining and achieving 'fairness' in a legally meaningful context, while simultaneously preserving model efficacy for relevance identification.
3. A significant area of debate among legal technologists and privacy advocates involves applying principles from data protection laws, such as the 'right to an explanation,' to the outputs of AI used in discovery. This raises challenging questions about whether parties in litigation have a right to understand the precise factors or data points that led an AI to flag specific documents as relevant or irrelevant, and the technical feasibility of generating such explanations in a comprehensible manner for complex model decisions.
4. The necessity of navigating a fragmented global regulatory environment for data privacy is pushing innovation in 'privacy-preserving' AI techniques. For instance, federated learning architectures are gaining traction in cross-border discovery scenarios, enabling AI models to learn from data distributed across different jurisdictions without requiring sensitive information to be centralized, offering a technical workaround to comply with diverse and sometimes conflicting data residency and processing regulations.
5. Increasingly, law firms are deploying AI systems to perform internal audits and risk assessments of their *own* AI tools used in discovery. This proactive approach is driven by the anticipation of regulatory scrutiny regarding potential algorithmic bias or unpredictable behavior in legal processes. The technical challenge lies in developing reliable metrics and monitoring frameworks to evaluate the performance and fairness of these systems against evolving ethical and legal standards, essentially using AI to help govern AI use.
Tata Elxsi Employee Ruling Spotlights AI's Growing Influence in Legal Discovery and Labor Rights - Adapting legal practice models for AI integration
Integrating artificial intelligence is prompting a fundamental rethinking of how legal services are delivered, particularly within large law firms. New models are emerging to incorporate AI across core functions such as managing discovery processes, conducting legal research, and assisting in the creation of legal documents. This evolution, however, is not straightforward. It forces critical consideration of significant ethical questions, including how to prevent or manage algorithmic bias and the difficulty of understanding why an AI reached a certain conclusion. Maintaining adequate human oversight remains vital. Successfully embedding AI within legal workflows necessitates a continuous, critical assessment to ensure technology serves to strengthen, rather than compromise, the foundational principles of justice and professional integrity.
Here are some observations on how legal practice models are starting to adapt with the integration of artificial intelligence, framed from the perspective of someone exploring the underlying technology as of May 22, 2025:
From a technical perspective, adapting legal practice models for AI integration is less about simply plugging in tools and more about redesigning workflows and interaction paradigms. It's intriguing to see where the friction points and unexpected developments are emerging as computational capabilities meet established professional practices.
1. One notable shift is in how legal professionals are learning to query vast legal knowledge bases. Moving beyond structured Boolean searches, the adaptation involves interacting with generative AI models capable of synthesizing information from diverse sources. The engineering challenge here lies in ensuring these models remain factually grounded within the specific, often subtly nuanced, context of legal precedent and statutory language, minimizing the risk of hallucination or misinterpretation that could lead to malpractice.
2. Generating complex legal documents is evolving beyond template filling. While AI can draft boilerplate, the adaptation involves building systems that can generate original clauses or modify existing ones based on highly specific client needs, jurisdictional variations, and evolving case law. This demands AI architectures capable of handling long-range dependencies in text, maintaining logical consistency across hundreds of pages, and adhering to sophisticated stylistic and formal requirements, a non-trivial technical feat.
3. Firms are exploring AI not just for finding documents but for analyzing the logical structure of arguments within briefs and judicial opinions, aiming to build more robust strategic frameworks. The adaptation here involves teaching lawyers to leverage AI outputs that map counter-arguments or identify potential weaknesses in their own reasoning. From an engineering standpoint, developing AI that truly understands abstract legal reasoning and can assess rhetorical effectiveness remains a complex, ongoing research area with significant performance variability.
4. A critical bottleneck in adapting existing practice models is the sheer difficulty of integrating disparate AI tools and legacy legal software. Law firms often operate with systems that weren't designed for seamless data exchange. Building middleware, establishing secure data pipelines, and standardizing data formats to allow AI models to access information securely and efficiently across discovery platforms, document management systems, and financial tools is proving to be a substantial engineering and operational hurdle.
5. There's increasing focus on building what might be termed 'AI-assisted explanation' for lawyers themselves. As AI tools provide insights into case strategy or suggest contractual language, the technical demand is growing for the AI to not just provide the output but to offer a comprehensible rationale or point to the specific legal sources that informed its suggestion. This is challenging because explaining complex neural network decisions in a way that aligns with human legal reasoning is an active area of research with no simple solutions yet widely deployed.
More Posts from legalpdf.io: