Legal Requirements for AI-Powered Lease Termination Agreement Validation A Technical Analysis
Legal Requirements for AI-Powered Lease Termination Agreement Validation A Technical Analysis - Core Legal Requirements for Automated Lease Analysis Under US Law 2024
The use of AI in lease analysis within the US legal landscape is rapidly evolving in 2024. Tools employing Natural Language Processing (NLP) and Machine Learning (ML) can now delve deeper into lease documents, recognizing key elements like obligations and potential hazards. This enhanced understanding can help ensure compliance with established legal norms. But alongside these benefits, concerns linger. AI systems can, by their nature, reflect and amplify existing biases, especially within crucial decision-making processes in contracts or legal disputes. This adds a layer of complexity to implementing AI solutions within legal workflows. The federal government's efforts to regulate AI development are gaining momentum, putting a spotlight on the need for legal professionals to navigate this emerging regulatory environment. The goal is to harness the power of AI in a way that ensures both the trustworthiness and validity of lease analysis. This move towards automation in legal practices is becoming undeniable, requiring continuous adjustment to keep pace with these transformative technologies.
Within the US legal landscape of 2024, the increasing adoption of automated lease analysis tools necessitates a keen awareness of various core legal requirements. For instance, the Fair Housing Act looms large, demanding that AI-driven tenant selection processes are free from any inherent biases. Transparency becomes a key issue, as algorithms used in automated lease agreements need to be comprehensible and open to scrutiny by regulators. The Uniform Commercial Code (UCC) weighs in on the topic of electronic contracts, placing importance on the authenticity and integrity of digital signatures within automated lease processes.
The Electronic Signatures in Global and National Commerce Act (ESIGN) ensures that automated lease terminations using electronic signatures maintain the same legal validity as traditional handwritten ones. Even though it originates in the EU, the General Data Protection Regulation (GDPR) influences US companies operating globally, necessitating robust data protection measures within automated lease analysis systems handling personal information. The Americans with Disabilities Act (ADA) introduces a new dimension, requiring accessibility in user interfaces for automated lease systems to serve individuals with disabilities.
Furthermore, the legal principle of "Caveat Subscriptor" highlights the importance of user understanding. Automated systems must guarantee that users comprehend the lease terms they agree to, suggesting a need for clear and transparent output from the algorithms. The legal landscape is also fragmented with state-specific landlord-tenant laws, which can significantly impact compliance parameters for lease analysis, depending on location.
A major hurdle for developers is creating systems that can effectively interpret contextual nuances. Legal terminology can vary in meaning depending on location and the most recent court decisions, demanding advanced NLP capabilities within the system. As a result of the potential for incorrect lease terminations, the call for insurance or warranties that safeguard those relying on these tools is growing louder. It's important to recognize that relying on AI for critical legal tasks may increase the risk of future liability claims and these tools will require a more robust system of safeguards.
Legal Requirements for AI-Powered Lease Termination Agreement Validation A Technical Analysis - Technical Framework for Notice Period and Tenant Rights Detection
The "Technical Framework for Notice Period and Tenant Rights Detection" is crucial for ensuring legally sound lease termination processes, especially given the diverse legal landscape across different states. This framework emphasizes the need for AI systems to accurately identify and process key elements within lease termination notices. This includes correctly recognizing the stated termination date, adhering to the legally mandated notice periods specific to each state, and correctly including any relevant legal justifications for the termination, such as local landlord-tenant laws or specific lease clauses.
The legal complexities surrounding lease terminations, particularly in the context of AI-driven lease analysis, necessitate systems capable of adapting to the unique requirements of each state. The interpretation of legal language can shift depending on location and recent legal decisions. This adaptability is critical, as misinterpretations can lead to significant legal issues and potentially harmful consequences for tenants. The framework stresses that accurate and compliant interpretation of lease termination notices, including the proper notice periods and tenant rights, is paramount.
In essence, this technical framework emphasizes that the intersection of AI and legal processes requires careful consideration. As AI systems become increasingly integrated into lease agreements, it is crucial that they are built with a high degree of flexibility and legal precision. Developers must ensure these AI systems are capable of interpreting complex legal language with accuracy and adapting to the ever-changing requirements of state-specific landlord-tenant laws. Only then can we build trust and ensure that automated lease termination processes are both legally sound and fair to all parties involved.
A key challenge for AI in lease analysis is adapting to the wide variations in landlord-tenant laws across different US states. The meaning of legal terms can change dramatically depending on location and recent court decisions, which means an AI system trained on a national dataset might struggle to interpret specific state regulations accurately. For example, "breach of contract" can have significantly different implications in different states, depending on local legal precedent and statutes. This highlights the need for AI systems to be flexible and capable of adapting dynamically to these specific nuances, or they risk leading to incorrect or legally unsound lease terminations.
Furthermore, the temporal element of lease agreements is often overlooked in standard legal analyses. Lease clauses can become invalid over time due to shifting economic conditions or new regulations, requiring AI to be aware of the potential for terms to expire or change in applicability. This kind of temporal logic isn't usually built into traditional legal AI applications, posing a unique challenge.
Bias within existing housing data is another major concern. AI models, if trained on historical data, might unintentionally perpetuate existing biases within the housing market, potentially leading to discriminatory outcomes for certain communities. This indicates that strong bias-mitigation techniques are crucial for AI-driven lease analysis to ensure fair and unbiased application.
The ever-changing nature of judicial precedents poses a further challenge. AI tools must be able to track recent court decisions, which can change the interpretation of lease terms significantly. This highlights the need for ongoing monitoring and integration of these legal changes into the models to maintain accuracy.
Data privacy standards are also a major hurdle. While federal laws like ESIGN provide a framework for electronic signatures, states have different requirements for handling personal data, and a "one-size-fits-all" approach by AI systems might violate local regulations. Developers need to consider these specific data sovereignty concerns within each jurisdiction.
The design of the user interface is also crucial for user acceptance. AI-driven systems should present complex legal information in a clear and comprehensible way. This becomes particularly challenging when lease terminology might be interpreted differently in specific locations, potentially leading to user confusion and a lack of trust in the system.
Building truly effective AI for lease analysis in this environment requires region-specific training datasets. Using datasets trained predominantly at a national level might miss crucial state-specific nuances. Therefore, it's beneficial to explore localized datasets that reflect the unique language and regulations in individual states.
Beyond federal laws like ESIGN, AI systems must contend with a multi-faceted regulatory landscape. Laws like the Fair Housing Act and individual state's tenant protection regulations all need to be considered, requiring precise and nuanced compliance for the systems to be valid.
The quick pace of change within housing laws adds to the complexity. If AI models are not regularly updated, they may quickly become outdated and unable to keep pace. This implies a need for continuous legislative monitoring and integration within the machine learning pipeline to maintain compliance and accuracy.
Lastly, the principle of "Caveat Subscriptor," which emphasizes tenant comprehension of the lease terms, is crucial in this context. AI systems should provide clear, easy-to-understand outputs. Failure to do so could compromise the reliability of automated lease analysis and lead to disputes over misinterpreted agreements.
Overall, creating adaptable AI for lease termination analysis across different states is a complex and continuing task. It requires a combination of advanced AI techniques, expert legal knowledge, and a strong understanding of the constantly changing legal environment within each jurisdiction. It is an area where ongoing research and refinement are needed.
Legal Requirements for AI-Powered Lease Termination Agreement Validation A Technical Analysis - Data Privacy Standards and GDPR Compliance in AI Lease Processing
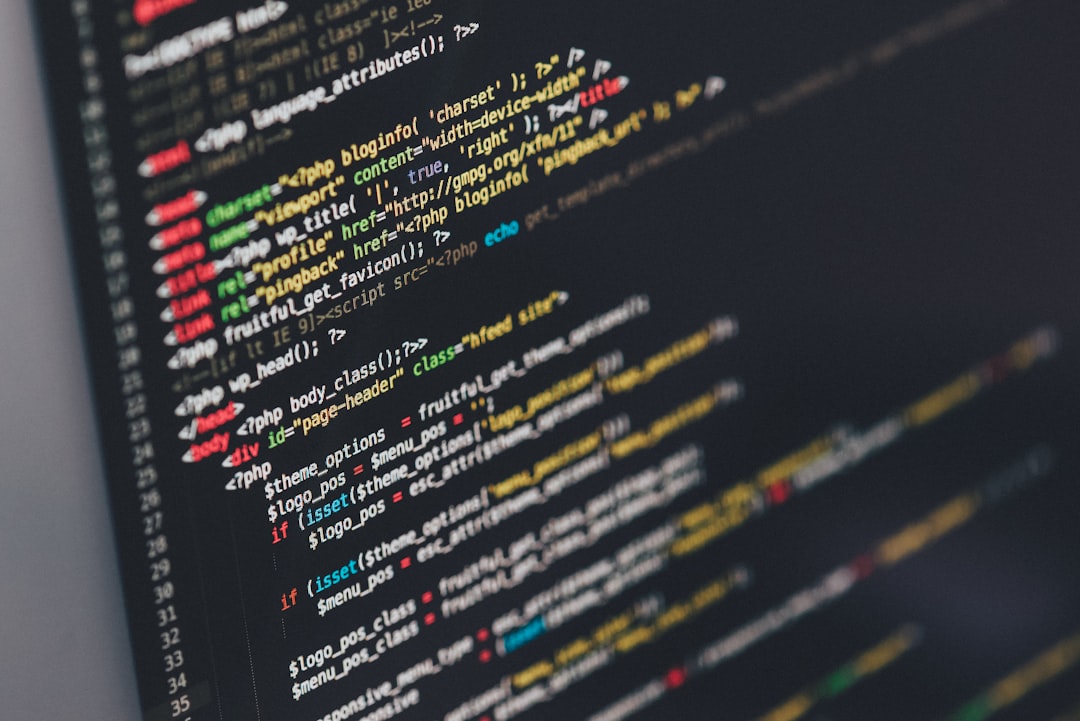
When AI is used to process lease agreements, data privacy and compliance with regulations like the GDPR become paramount, especially given the increasing reliance on sensitive personal data within these systems. The GDPR, which took effect in 2018, seeks to safeguard personal data of individuals, encompassing any processing that involves algorithms. This means that AI systems handling personal information related to leases are subject to the GDPR's stipulations, including the requirement to only process the minimal amount of data needed for a specific purpose. Furthermore, there are strict limitations imposed on how much data is collected, how long it's stored, and who has access to it.
Developers and businesses using AI for lease processing can cultivate user trust and safeguard sensitive data by adhering to GDPR guidelines and other relevant data privacy standards. This is not just about legal compliance; establishing clear and ethical data handling practices is essential. This also includes developing standards that address ethical considerations, ensuring that developers incorporate them into the design of the AI systems.
Furthermore, fundamental security measures are a must to prevent unauthorized access or data extraction from the AI systems. Compliance in this space also necessitates the creation of clear data usage policies, a thorough review of any third-party AI providers involved, and the development of technical safeguards to maintain the integrity and accuracy of the data being processed. A crucial aspect of this process is establishing a detailed plan for data retention and deletion, ensuring adherence to GDPR's requirements.
Ultimately, the convergence of AI and GDPR highlights the significant responsibility that organizations have beyond just complying with the letter of the law. They must prioritize ethically sound data practices throughout the lifecycle of their AI-powered lease processing. This includes continually evaluating their data management procedures and consistently reviewing their technical safeguards to ensure the protection of individuals' personal data. It's a constant challenge that requires ongoing attention.
The General Data Protection Regulation (GDPR), introduced in 2018, significantly impacts how organizations handle personal data, including those using AI in lease processing. While it originated in the EU, its influence extends to US companies handling data of EU residents, making it a global concern. It's interesting to note that GDPR requires any processing of personal data by algorithms, meaning AI systems used for lease processing must adhere to its principles. This includes limiting the data collected to only what's strictly needed for a specific purpose, controlling the quantity and duration of storage, and restricting data access.
One of the major hurdles the GDPR creates is obtaining meaningful consent for data processing. AI developers have to design user interfaces and systems that clearly communicate data rights, making it harder for a system to operate without the user's complete understanding of the data exchange. Also, adhering to "Privacy by Design" is important, meaning data privacy has to be central to AI development from the very beginning. It's a shift in how we design AI systems, emphasizing protection throughout the system's lifecycle.
The "Right to Explanation" within GDPR adds a level of complexity for AI systems that can often function as "black boxes." In lease processing, where decisions need to be clear, AI models must explain their reasoning, potentially requiring new types of algorithms. Data breaches have to be reported to affected parties within 72 hours under GDPR, increasing the need for robust security protocols and systems that can react quickly to security incidents. Transferring personal data outside the EU also requires special consideration. If AI systems used in lease processing are based in the US, developers have to carefully navigate the rules to ensure compliance.
Another challenge for AI lease processing developers is meeting the audit requirements of the GDPR. AI systems have to be regularly checked to ensure they collect, store, and use personal data in accordance with GDPR's rules. The heavy fines associated with GDPR violations—up to 4% of global revenue—serve as a strong motivator for companies to comply. This will have an impact on how AI algorithms for lease processing are trained. It will become even more crucial to anonymize the training data or to generate synthetic datasets to avoid violations. These are tough issues for the developers of these systems, but ones they must consider and address.
In essence, the intersection of AI and GDPR brings a new set of challenges to AI lease processing systems. It appears the legal framework of GDPR creates a heightened need for better security practices, more careful data management, and potentially the need for more transparent AI systems. How developers will react to these new demands in the coming years will be very interesting to follow.
Legal Requirements for AI-Powered Lease Termination Agreement Validation A Technical Analysis - Error Rate Benchmarks and Manual Override Protocols
Within the context of AI-driven lease termination agreement validation, establishing clear benchmarks for error rates and implementing robust manual override protocols is crucial. Defining acceptable error rates helps measure the accuracy of AI systems in interpreting lease language, especially when navigating the complex and varied regulations across different states. However, recognizing the limitations of current AI technology is key. Manual review protocols are essential to address situations where AI systems may fail to accurately identify critical elements within lease agreements, particularly those related to state-specific landlord-tenant laws. This combination of AI-driven analysis and human oversight promotes accountability and fosters user trust. It ensures that the potential legal consequences of lease terminations are appropriately managed. As AI's role in legal applications continues to grow, integrating strong error management strategies into AI systems is paramount for ensuring the integrity and legal soundness of lease termination processes.
When we look at how well AI performs in analyzing lease agreements for termination, we find that the error rates can change quite a bit. It depends on how complex the lease is. Simpler leases might have error rates around 5%, but more complicated ones can see errors jump to over 20%. This difference underscores the importance of making sure our AI systems are really good at dealing with the wide range of legal situations we see in leases.
Interestingly, even the most advanced AI models for lease processing benefit from human intervention, especially when things get tricky. We've seen that letting humans step in and make decisions, or override the AI's suggestions, can cut down errors by as much as 50%. This makes sure legal rules are followed and tenant rights are protected.
Setting up systems to track the error rates and give humans a way to take over can be expensive. It's not uncommon for these costs to take up over 30% of the total money spent on deploying the AI. This cost includes building the AI itself, but also the ongoing costs of people reviewing the results and making sure the AI is doing things correctly.
Something we've observed is that the way AI is trained can lead to biases in the outcome. If the training data comes from past lease agreements, it can unknowingly build those past biases into how the AI interprets new leases. This bias can show up in how it recognizes tenant rights or calculates notice periods, leading to possible legal fights. We need careful ways to check for and eliminate bias in the models.
Laws around lease agreements are constantly changing, which makes it tricky for the AI. The AI designed for lease terminations today might be out of date tomorrow because the laws have changed. The things that were legally correct last year might not be valid this year. This means that the way we design the AI system has to be very flexible and adaptable so that it can keep up with those changes.
It's surprising to find that a large portion of the mistakes AI makes in lease analysis – about 70% – comes from not taking into account things specific to each case. This might be something as simple as local tenant laws or some particular clause in a lease agreement. The AI's performance improves when we tailor each AI application to the specific legal environment it's working in.
How we design the interface for users has a big effect on how effective those manual override systems are. If we make it easy and intuitive for users to step in, then we can reduce the chances of human errors when they do. This makes the whole system more compliant with the law and protects its integrity.
When it comes to lease terminations, a mixed approach seems to be best. Using AI for the first look at the lease, then having human experts review the results, turns out to be far more accurate than just relying on the AI. Studies show we can get a 60% reduction in errors this way compared to fully automated systems.
As AI becomes more and more a part of legal practices, questions about who is responsible if something goes wrong become a serious concern. If the AI wrongly decides to end a lease, is it the AI company, the people who built it, or the lawyers who used it that are responsible? That is something that's still being worked out.
Developing and implementing AI for lease termination needs people with skills in both AI and law. AI teams are now working closely with legal experts to create training data that reflects how complex and diverse lease laws are. This partnership helps improve the quality of the AI's assessments.
Legal Requirements for AI-Powered Lease Termination Agreement Validation A Technical Analysis - Court Precedents Regarding AI Generated Lease Termination Notices
The use of AI for generating lease termination notices is a relatively new development, and as such, there's a growing need to understand how courts are approaching this practice. While the number of specific cases is still limited, recent court cases have started to address the use of AI-generated documents in legal proceedings. A key concern raised in these cases is the adherence to due process. Judges and courts are paying attention to whether AI-generated termination notices provide adequate information and follow the established procedures required by law. One area of concern is the accuracy of information presented by AI tools. Some instances have shown that AI-generated documents have included inaccurate or fabricated legal information, leading to questions about the reliability of these tools. This has sparked a debate about the potential ethical challenges of relying on AI in sensitive legal situations like lease terminations. The broader legal landscape surrounding AI is also still evolving, making it essential that individuals involved in developing and deploying such tools stay updated with evolving legal requirements and interpretations. There's a growing focus on how to ensure that AI-powered systems are designed in a way that doesn't unintentionally perpetuate bias against certain groups of tenants. Ultimately, the legal questions surrounding AI's role in lease terminations are likely to become more frequent and significant as AI adoption increases in legal and real estate fields. The legal community and the developers of AI tools need to engage in an ongoing discussion about the legal liabilities associated with these tools, the responsibilities of those using them, and how to ensure that they operate in a way that's both effective and ethically sound.
AI's application in generating lease termination notices is facing a complex legal landscape, especially given the wide variation in state-specific laws. The way AI systems handle lease terminations needs to adapt to differing local interpretations and court rulings, as these legal standards can change frequently.
Interestingly, AI's accuracy seems to be tied to the complexity of the lease itself. Simpler leases often see error rates around 5%, but as lease terms get more involved, the error rates can climb past 20%. This highlights the difficulties inherent in interpreting legal language, even for sophisticated AI.
Perhaps surprisingly, it appears that human intervention can significantly improve the reliability of AI in this context. Systems where humans can override AI decisions have shown a reduction in errors of up to 50%. This indicates that fully automated systems may not be the most reliable option for legally sound lease terminations.
However, ensuring that human intervention is possible comes at a cost. Implementing the proper error-tracking systems to keep things compliant with regulations can lead to a substantial portion of project budgets – often more than 30% – being spent on manual review processes. This is a less-visible expense that adds to the cost of AI implementation.
Another interesting aspect is the potential for biases within AI systems. If the AI is trained using past lease data that reflects biases, it can inadvertently build those biases into its decision-making process. This can lead to unfair outcomes for certain tenants, particularly when determining tenant rights or calculating proper notice periods, underscoring the need for better methods to detect and remove such biases from the models.
The dynamic nature of housing laws presents a continuous challenge. AI models need to be designed to adapt to ongoing changes in legislation, as a system designed today could be outdated quickly due to a new law or regulation. This highlights a need for flexibility and adaptable AI models if they are to remain useful in lease terminations.
Many of AI's errors in this context seem to stem from not considering the specific nuances of individual lease cases. Researchers have found that a remarkably large portion, approximately 70%, of errors occur due to a failure to correctly account for local regulations or particular lease terms. This indicates that a 'one-size-fits-all' AI approach may not be the most effective. The accuracy can be enhanced by customizing the AI to the specific legal context it will operate within.
The way we design the user interface seems critical for manual review systems to be successful. Making it easy for users to take over and review AI-driven decisions helps reduce user error and improves the overall reliability of the process.
Combining the insights of AI with the expertise of human review seems to be the most effective approach currently available. Studies show that hybrid systems which employ AI for initial assessments and then bring in human reviewers can lower error rates by up to 60% when compared to fully automated systems.
The increasing role of AI in making lease termination decisions raises complex questions about legal liability. If AI misjudges a termination and results in a wrongful termination, it's still unclear if the responsibility would lie with the AI developers, the companies utilizing the AI, or the legal professionals relying on the AI's output. This is a very important area that needs to be addressed as we move forward.
Successful development of AI for lease termination appears to require the collaboration of AI engineers and legal professionals. AI teams are increasingly working alongside legal experts to develop training data that reflects the intricacies of lease law, enhancing the accuracy of the AI's analysis.
It seems that the path forward for effective AI-driven lease terminations will require ongoing research and development to address these challenges and ensure legal accuracy and fairness.
More Posts from legalpdf.io: