Automate legal research, eDiscovery, and precedent analysis - Let our AI Legal Assistant handle the complexity. (Get started for free)
Legal AI Analytics How Dashcam Evidence and Digital Forensics Led to Swift Arrests in the Baker-LebronDevila Case
Legal AI Analytics How Dashcam Evidence and Digital Forensics Led to Swift Arrests in the Baker-LebronDevila Case - AI-Enhanced Video Analysis Uncovers Key Evidence in Dashcam Footage
The application of AI in analyzing video evidence, particularly dashcam footage, has significantly impacted legal proceedings. Cases like Baker-LebronDevila demonstrate how AI can unveil crucial details hidden within video recordings, leading to faster resolutions. Tools like cloud-based OCR have streamlined the process of extracting information from video, transforming vast amounts of visual data into usable evidence.
However, the burgeoning use of AI-enhanced evidence raises important questions about its validity and dependability in the courtroom. Recent judicial decisions, where AI-enhanced video evidence was deemed inadmissible, underscore the need for caution. The legal system faces a challenge in integrating these new technologies while maintaining the integrity of the judicial process. Balancing the potential benefits with the need for fairness and security is crucial. As AI's role in legal evidence continues to grow, courts must carefully examine the implications and adapt procedures accordingly, recognizing that this shift may necessitate substantial adjustments within the legal profession.
AI's role in legal proceedings is expanding rapidly, particularly in the domain of eDiscovery and legal research. AI-powered tools can process massive datasets from various sources, like dashcam footage and document repositories, much faster than human analysts. For instance, AI algorithms are increasingly adept at identifying nuanced patterns in video, such as subtle changes in driver behavior or vehicle movement, which might go unnoticed by a human reviewer. This capability can drastically reduce the time required to find critical evidence within large video files.
Furthermore, the integration of AI in eDiscovery has fundamentally altered the discovery process within law firms. By automating the initial stages of document review, AI can accelerate the process, potentially leading to a 50% reduction in review time while maintaining accuracy. This advancement is particularly relevant in large-scale cases with enormous volumes of documents.
However, the use of AI in the legal field also necessitates a critical examination of its implications. While AI can streamline legal research by swiftly identifying relevant case law and legal arguments, it is crucial to consider the potential biases that may be embedded within these AI systems. Concerns regarding privacy and surveillance biases, especially when AI is deployed in law enforcement applications like facial recognition, need to be addressed carefully. As AI's influence in law continues to grow, the legal system faces the challenge of developing robust ethical guidelines and standards to ensure fairness and protect individual rights while harnessing the considerable benefits that these technologies offer.
The question of the admissibility of AI-generated evidence in court continues to be debated. The judiciary grapples with the challenge of determining the reliability and validity of evidence generated through AI-enhanced analysis. A recent Washington state case serves as a compelling example, illustrating the judiciary's cautionary approach when evaluating AI-enhanced evidence. Ultimately, the legal profession must adapt to this evolving technological landscape and formulate clear standards that guide the responsible and equitable use of AI-driven evidence in the pursuit of justice.
Legal AI Analytics How Dashcam Evidence and Digital Forensics Led to Swift Arrests in the Baker-LebronDevila Case - Machine Learning Models Extract Critical Speech Patterns From Digital Audio
The application of machine learning models in analyzing digital audio is transforming the field of digital forensics, especially within legal contexts. These models can extract subtle speech patterns and emotional cues hidden within audio recordings, offering a powerful tool for uncovering evidence that might be overlooked through traditional methods. This includes the identification and differentiation of voices, which can be vital in legal investigations. However, the rise of AI-driven audio analysis also presents significant ethical and legal concerns. The potential for voice cloning and manipulation of audio evidence raises questions about the reliability and integrity of this type of evidence in legal proceedings. As the use of AI in law expands, the legal profession needs to carefully consider the implications for courtroom procedures and ensure that advancements in AI do not compromise the fairness of trials or the accuracy of evidence. This includes developing clear guidelines and standards to responsibly integrate AI-powered audio analysis into the legal system, while also safeguarding against potential misuse and biases. Just as with other emerging AI applications in law, a balanced approach is critical, leveraging the benefits of AI while mitigating the risks.
Machine learning models are increasingly capable of extracting crucial speech patterns from digital audio, a development that could greatly impact digital forensics and eDiscovery within the legal field. This ability to analyze subtle cues within audio recordings opens up new possibilities for understanding the context and nuances of spoken words. For example, AI can dissect the acoustic environment of a recording, identifying the type of recording device used and potentially even revealing details about the location. Furthermore, a combination of Convolutional Neural Networks (CNN) and Long Short-Term Memory (LSTM) networks can automatically isolate relevant audio features. Features like the Zero Crossing Rate (ZCR), which gauges energy patterns within speech, help distinguish audio types.
Another critical application of AI in this domain is Speech Emotion Recognition (SER). SER focuses on extracting emotional nuances from speech, regardless of the actual words being spoken. This has implications for legal proceedings, potentially offering insights into witness credibility or revealing emotional states that might otherwise be obscured. However, the increasing reliance on these AI technologies raises complex ethical and legal concerns, especially in areas like voice cloning and the integrity of audio recordings. We must carefully consider the potential misuse of such technologies.
Interestingly, AI's influence isn't limited to audio analysis in legal cases. It's also being employed to expedite legal research. By analyzing vast repositories of legal texts, AI algorithms can identify and summarize patterns, ultimately leading to quicker and more efficient legal research. This has the potential to transform the discovery process, accelerating the review of large volumes of documents. Additionally, AI can sometimes uncover hidden clues within digital evidence that might be missed by human investigators. While this can be valuable, it also highlights a growing challenge in the legal system: ensuring the reliability and integrity of AI-generated insights. This concern is particularly relevant considering instances where AI-enhanced evidence has been deemed inadmissible in court.
The broader implications of AI's growing role in law are profound. It’s not just about enhancing efficiency; it’s also about influencing the future of justice. As AI-powered tools continue to develop, we need a rigorous discussion of fairness, bias, and privacy within the context of these technologies. As the legal profession grapples with AI's implications, establishing clear ethical guidelines and standards will be critical for ensuring that the promise of AI-driven insights is realized while protecting the integrity of legal processes and individual rights. The evolving landscape of legal evidence and AI is undeniably exciting, but it's vital to approach it with careful consideration and a commitment to responsible innovation.
Legal AI Analytics How Dashcam Evidence and Digital Forensics Led to Swift Arrests in the Baker-LebronDevila Case - Digital Forensic Teams Track Vehicle Movement Through GPS Metadata
Digital forensic investigators are leveraging GPS data embedded within various digital records, like those from dashcams or vehicle systems, to meticulously trace the movement of vehicles. This capability is proving increasingly important in legal proceedings, helping reconstruct events and establish timelines based on the digital trail left by vehicles. The rise in popularity of dashcams, paired with AI-powered analytics, provides even more nuanced insights into vehicle movement and behavior. This can help reveal subtle details that might otherwise be missed by a human review of the footage. However, the increased reliance on such technologies also raises complex questions regarding the accuracy and reliability of the evidence derived from this data. These concerns necessitate careful consideration of its admissibility in court and the development of clearer guidelines to ensure integrity within the legal process. As the role of AI expands in digital forensic analysis, the legal system must carefully examine and adapt to the implications of these technologies. It’s vital to strike a balance between utilizing the potential benefits and adhering to the core principles of justice and fairness in the pursuit of truth.
Digital forensic investigators are increasingly leveraging GPS metadata embedded within vehicle systems to reconstruct timelines and pinpoint locations in legal investigations. The precision of modern GPS, capable of achieving accuracy within a few meters, if not centimeters, greatly enhances the reliability of this data as evidence. However, the sheer volume of data generated by a vehicle's GPS—potentially several megabytes daily—presents both opportunities and challenges. Analyzing this wealth of timestamped location, speed, and direction data requires sophisticated tools and efficient methods.
Interestingly, AI is emerging as a promising approach to handle the deluge of GPS data. By applying machine learning algorithms, forensic teams can potentially identify patterns and predict vehicle behaviors based on historical GPS traces, offering deeper insights into investigations. This includes using techniques like time series analysis to correlate vehicle movements with other evidence, like dashcam recordings or witness statements. The result is a more comprehensive reconstruction of events.
The legal ramifications of this data are significant. Courts are increasingly relying on GPS metadata to resolve cases involving vehicular crimes. Decisions regarding liability, sentencing, or even acquittals are now influenced by these location-based datasets. Yet, this reliance on GPS data prompts important discussions around individual privacy. Questions regarding the limits of lawful surveillance through GPS tracking and the need for warrants to access this data are crucial, particularly given the growing capabilities of real-time location monitoring.
In addition to privacy concerns, data integrity is paramount. Forensic teams must ensure the authenticity and reliability of GPS metadata, which can be susceptible to manipulation. Techniques like cryptographic hashing are now being used to authenticate the data extracted from vehicles. Moreover, the evolving field of vehicle system forensics demands an interdisciplinary approach. Analyzing the complex datasets from modern vehicle telematics and infotainment systems requires collaboration between forensic experts, legal professionals, and data scientists, highlighting the technical specialization increasingly demanded within the legal profession.
The analysis of GPS data can also reveal subtle patterns that might escape human observation. Using machine learning, investigators can identify anomalies in driving behavior, potentially uncovering criminal intent or supporting pre-existing hypotheses. However, these advanced analytics also raise ethical and legal considerations. The potential biases inherent within machine learning models, especially when used in sensitive areas like criminal justice, must be carefully examined to ensure fair and equitable outcomes. The complex interplay of GPS data analysis, AI algorithms, and legal frameworks highlights the evolving nature of legal investigations, making it essential for the legal profession to continually adapt to the ever-changing landscape of technology.
Legal AI Analytics How Dashcam Evidence and Digital Forensics Led to Swift Arrests in the Baker-LebronDevila Case - Neural Networks Identify License Plates and Vehicle Models in Low Light Conditions
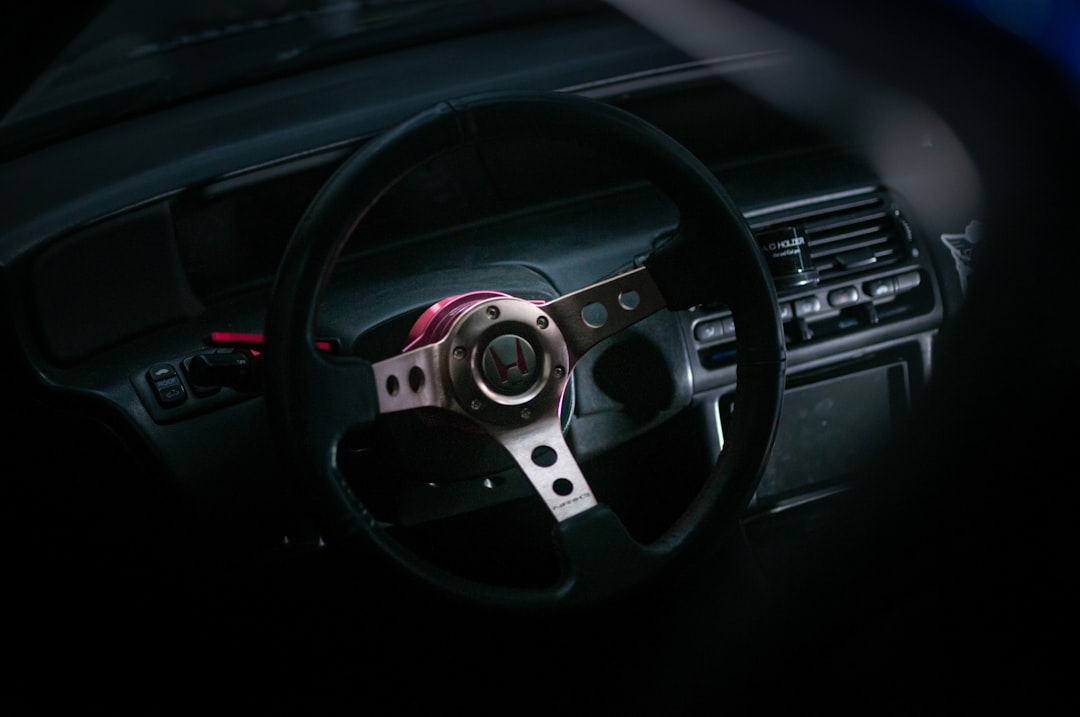
Neural networks are enhancing the ability of systems to automatically identify license plates and vehicle types, especially in situations with poor lighting. Techniques like deep learning, specifically with models such as YOLOv5 and the LIVDN, are improving the accuracy of these systems in complex or low-light conditions. This advancement allows law enforcement and investigators to quickly gather crucial evidence during investigations, similar to how the Baker-LebronDevila case highlighted the importance of timely and correct vehicle identification in facilitating swift arrests. However, the expanding use of AI in these critical areas requires continuous evaluation of the technology's reliability and potential biases, and also requires a careful examination of its impact on individual privacy. As AI integration within the legal system expands, finding the balance between fostering innovation and addressing ethical concerns is paramount to ensuring the continued fairness and integrity of legal proceedings.
AI is increasingly being used in legal discovery, specifically in the realm of eDiscovery, where large volumes of data need to be sifted through quickly and accurately. One area gaining traction is using neural networks for tasks like identifying and extracting information from documents or audio files. These networks, especially deep learning models like YOLOv5, have shown remarkable accuracy in identifying license plates and vehicle models, even in low light conditions, which could be relevant for various legal contexts involving dashcam footage or surveillance videos.
This ability to identify vehicles in diverse conditions is crucial in a field like eDiscovery, where analyzing video footage can be a time-consuming process. However, these systems are constantly under development. For example, the LIVDN (Low Illumination Vehicle Detection Network) is specifically engineered to handle situations with poor lighting. These kinds of specialized models underscore the ongoing need for refining and improving AI models to better suit real-world legal scenarios. Furthermore, many models are being developed using techniques like data augmentation, where they learn to analyze images with slightly altered conditions to improve performance.
A critical aspect of this is that these systems need to be robust enough to operate on everyday hardware found in law firms or used by investigators. The development of lightweight deep learning models allows for deployment on standard computer systems and smaller, resource-constrained devices. However, there are challenges. The accuracy of the systems can vary depending on environmental factors, lighting conditions, or even slight variations in license plate designs across different states or countries. The resulting variations highlight the need to tailor these systems to specific geographical locations.
The use of such AI within law also raises important questions about evidence discovery. As AI is generating insights in a more automated fashion, courts are struggling to decide on the admissibility of this data as evidence. There are legitimate concerns about potential biases in AI-powered systems. If a training dataset isn't diverse enough or includes embedded human biases, the resultant system may produce unfair or discriminatory outcomes. In addition, the sheer volume of data being generated by these technologies presents its own challenges. The legal system will need to grapple with what kinds of AI-generated insights will be discoverable during a legal case and establish rules around access.
Ultimately, the role of AI in eDiscovery is evolving rapidly. It provides a remarkable capability to expedite the discovery process by enhancing document review, but we must tread carefully. There's a need to understand the limitations, including potential bias in the models, and how these systems interact with existing legal frameworks. The continuous development of new models for diverse contexts, such as specialized architectures for different kinds of lighting conditions or geographical locations, indicates the importance of ongoing development and research within this field. While the promise of AI-powered legal research and document analysis is significant, it's essential to develop clear standards and address ethical considerations to ensure fairness and justice within the legal system.
Legal AI Analytics How Dashcam Evidence and Digital Forensics Led to Swift Arrests in the Baker-LebronDevila Case - Automated Timeline Construction Maps Criminal Activity Sequence
Automated timeline construction has emerged as a valuable tool in digital forensics, enabling the reconstruction of criminal activity sequences in a structured manner. This method leverages the wealth of timestamped data embedded within digital evidence, such as electronic communications, device logs, and video recordings, to create a detailed chronological account of events. This process, while powerful, can be complex, especially when dealing with the massive amounts of data often generated in modern investigations.
AI's role in this process is becoming increasingly important. AI-driven systems can sift through vast quantities of data, identify duplicates, and pinpoint crucial events or patterns that may otherwise be missed. This ability to streamline and refine the timeline creation process significantly improves efficiency and reduces the risk of human error. It also enables non-expert investigators to more easily interpret complex datasets.
Despite the benefits, the legal implications of AI-generated timelines are still being debated. The courts are grappling with the question of admissibility and reliability of evidence generated through AI. Concerns surrounding potential bias within AI models, data integrity, and the preservation of due process are paramount. As we rely more heavily on digital evidence in investigations, clear standards and guidelines for using AI in this context will be vital for the continued integrity and fairness of legal proceedings. While the technology offers promising solutions to challenges in modern criminal investigations, it is critical to balance its adoption with ethical considerations and legal oversight.
1. **Automating Event Sequencing for Legal Cases**: AI-driven systems are increasingly used to build timelines of events in criminal cases by connecting different pieces of digital evidence. These systems are particularly effective at organizing and connecting seemingly disparate data, such as GPS tracking data and dashcam footage, to construct a narrative of criminal activity, impacting court decisions and shaping legal strategy.
2. **Tackling the Data Deluge**: Legal teams often deal with massive amounts of data in major investigations. AI algorithms are able to analyze terabytes of information in a short period, significantly shortening the time it takes human teams to process and organize data. This can reduce eDiscovery time from months to just days in complex cases.
3. **Evolving eDiscovery Capabilities**: AI is altering eDiscovery by moving beyond simple keyword searches. It can now detect complex patterns in data, which can lead to the discovery of crucial evidence that traditional techniques might miss. This increased capability is vital for legal teams to build stronger cases based on hidden insights within massive datasets.
4. **Moving Beyond Static Events**: AI systems can go beyond simply identifying isolated events within digital evidence. They are increasingly capable of recognizing behavioral patterns within that data, which can reveal potential criminal intent. By analyzing how someone moves or interacts in a series of events leading up to an incident, these systems may be able to contribute to a deeper understanding of the crime's context.
5. **Real-Time Decision Support**: AI algorithms can analyze live video feeds or real-time data from dashcams, providing immediate insights during ongoing investigations. This is especially valuable in scenarios that demand quick decisions, potentially influencing police responses and emergency procedures.
6. **Adapting the Legal Landscape**: The integration of AI into legal proceedings is forcing legal frameworks to evolve. The admissibility of AI-generated evidence is a central question, requiring a careful examination of the underlying technology and how it impacts court procedures and the overall integrity of the judicial process.
7. **Addressing Potential Bias in AI Systems**: The reliance on AI in legal settings raises concerns about potential bias. If the data used to train AI systems is not representative of the real world or contains embedded human biases, then the system's output can be skewed, leading to unfair outcomes in areas like racial profiling or suspect identification. The legal system is beginning to consider how to prevent and mitigate these biases.
8. **Enhanced Audio Analysis and Integrity Challenges**: AI is revolutionizing the way audio evidence is analyzed, allowing investigators to identify subtle emotional cues and context hidden within recordings. However, this also increases the potential for manipulation of audio evidence through voice cloning, raising questions about audio integrity and reliability in court.
9. **Predictive Analytics in Legal Strategy**: AI systems are now capable of leveraging historical data to predict future legal outcomes. This ability can potentially give legal teams insights into case management and how judges might respond to certain arguments or evidence, offering strategic advantages. However, questions surrounding the reliability and fairness of such predictions need careful consideration.
10. **The Need for Collaboration Across Disciplines**: Effectively using AI in legal settings requires collaboration between lawyers, data scientists, and forensic experts. This interdisciplinary approach is crucial to navigate the technical complexities of AI-driven insights while maintaining the fundamental values of fairness and integrity that underpin the legal system.
Legal AI Analytics How Dashcam Evidence and Digital Forensics Led to Swift Arrests in the Baker-LebronDevila Case - AI Pattern Recognition Validates Witness Statements Against Digital Evidence
AI's capacity to recognize patterns within digital evidence is transforming how we evaluate witness accounts in legal proceedings. This ability to compare witness statements with objective data found in digital sources, like dashcam footage or electronic communications, provides a powerful tool for uncovering inconsistencies and potentially strengthening the validity of evidence presented in court. This new approach offers a more nuanced understanding of events by cross-referencing subjective testimony with objective digital data.
However, the expanding use of AI in legal evidence raises concerns about transparency and potential biases. When AI algorithms are employed as a decision-making tool, especially within a "black box" model, it can be difficult to understand exactly how the AI arrived at its conclusions. This lack of transparency can erode public trust and raise questions regarding the reliability of AI-driven insights in the courtroom. Furthermore, the algorithms used in these AI models could inadvertently perpetuate or amplify existing biases present in the data used to train them, leading to potentially unfair or discriminatory outcomes.
Therefore, as the legal field incorporates these powerful new AI tools, it's crucial to develop clear guidelines and standards for ensuring fairness and due process. There's a need to establish a balance between harnessing the potential benefits of AI-powered analysis and mitigating the risks associated with these new technologies. This evolving landscape necessitates a careful approach, ensuring that while AI enhances our ability to find and evaluate evidence, it doesn't compromise the principles of fairness and justice that underpin the legal system.
1. **Predicting Legal Outcomes**: AI is increasingly capable of analyzing historical legal data to predict the potential outcomes of cases. By identifying patterns and trends in past verdicts, these systems can help legal teams better assess the strengths and weaknesses of their arguments and potentially refine their strategies. It's a fascinating area, though I wonder how reliable these predictions truly are. There's always a risk of overfitting to past data and not capturing the complexities of each unique case.
2. **Evaluating Witness Reliability**: AI models are being developed that can evaluate the reliability of witness statements by examining speech patterns, inconsistencies in narratives, and even subtle emotional cues. This application of AI has the potential to provide new insights into witness credibility, but also raises questions about the objectivity of these assessments. How do you ensure that biases in the training data don't influence the outcome?
3. **Connecting Evidence Automatically**: Machine learning algorithms are now being used to automate the process of correlating different pieces of evidence within a case. For instance, connecting GPS location data to witness statements or matching images found on a suspect's device with other visual evidence. While incredibly helpful for faster investigation, it's crucial to ensure the validity of these connections and verify them rigorously, as any automated system can be prone to errors.
4. **Contextualized Legal Research**: AI-powered legal research tools are becoming more sophisticated, capable of dynamically adjusting their search parameters based on the context of the findings. This adaptive approach can lead to a more nuanced and comprehensive understanding of relevant legal precedents and arguments, speeding up the legal research process significantly. However, I do worry that relying solely on AI for research could limit the breadth of knowledge gained, as humans are adept at spotting unexpected connections.
5. **Real-time Courtroom Analytics**: There's an increasing trend towards integrating AI systems into courtrooms that can analyze testimonies and evidence in real-time. These systems aim to provide judges and lawyers with quick access to relevant information as a case unfolds, potentially increasing the efficiency of trials. This raises intriguing possibilities, but I can't help but question if this real-time analysis might sway decision-making unfairly, especially if there isn't enough time for careful scrutiny.
6. **The Authenticity Challenge**: The increased reliance on digital evidence has highlighted the importance of data integrity. AI tools can generate extremely realistic deepfakes and manipulate audio, making it harder to verify the authenticity of digital evidence. Developing robust and reliable methods to authenticate digital evidence is a critical challenge. How can we ensure that we can trust what we see and hear in the digital realm?
7. **Image Recognition's Impact**: AI's advancements in image recognition are transforming investigations by facilitating the rapid identification of objects, individuals, and license plates. This capability has implications for various aspects of legal cases, including speeding up the identification of suspects or correlating visual evidence with other data points. This is a powerful tool, but I also think about the potential for misuse, particularly in surveillance applications, where it can easily become invasive of privacy.
8. **Tracking Fleeting Evidence**: Social media and online communication often produce evidence that is ephemeral – it disappears quickly. AI tools can help to capture and analyze this fleeting data before it vanishes, allowing legal teams to preserve and utilize it as evidence. It's fascinating how AI is adapting to the modern digital landscape, but this type of capability also reinforces the need for clear laws around data retention and privacy.
9. **Bridging Jurisdictional Gaps**: AI is enabling the seamless integration of legal data across different jurisdictions, fostering better collaboration between law enforcement agencies and creating a more comprehensive picture of criminal activity. This increased connectivity is a positive development, but it also highlights the need for clear protocols for data sharing across borders and careful consideration of privacy and data protection laws.
10. **Guiding Ethical Use**: As AI increasingly integrates into the legal system, there's a growing awareness of the need to establish ethical guidelines for its deployment. These guidelines will be essential for ensuring that AI systems are used responsibly, minimizing risks such as bias and upholding the principles of justice. The question of how to create truly unbiased AI remains a huge challenge in the field, as human biases can seep into algorithms in unexpected ways.
Automate legal research, eDiscovery, and precedent analysis - Let our AI Legal Assistant handle the complexity. (Get started for free)
More Posts from legalpdf.io: