Automate legal research, eDiscovery, and precedent analysis - Let our AI Legal Assistant handle the complexity. (Get started for free)
AI-Powered Investigation Tools Analyzing Discrimination Claims in White v
University of Idaho
AI-Powered Investigation Tools Analyzing Discrimination Claims in White v
University of Idaho - AI Pattern Recognition Reveals Historical Bias Patterns in University Admissions
AI's increasing presence in university admissions processes is prompting a closer look at how historical biases might be ingrained within the selection procedures. AI's ability to sift through massive amounts of data can expose patterns suggesting that existing inequalities may be inadvertently reinforced by these tools. This raises concerns regarding the potential for AI to perpetuate discrimination against certain groups, such as women, people with disabilities, and ethnic minorities. Studies have shown that AI algorithms can misclassify academic performance, particularly for Black students, compared to White students, highlighting the need to mitigate such disparities.
The goal is to leverage AI for improved fairness and efficiency in admissions while simultaneously acknowledging and addressing the potential for bias. Carefully monitoring how AI is implemented in admissions is crucial to avoid replicating historical patterns of prejudice. As universities embrace AI in this critical area, it's imperative that they take steps to minimize human biases while boosting the objectivity of the decision-making process. This involves continuous evaluation and adjustments to ensure AI doesn't inadvertently contribute to discriminatory practices.
AI's ability to analyze extensive university admissions data goes beyond standard statistical methods, potentially revealing ingrained discriminatory patterns that may have been previously hidden. This capability is particularly relevant in legal cases, where AI algorithms are increasingly used to analyze historical data and predict legal outcomes, providing valuable insights into potential biases embedded in admissions processes.
The application of AI in e-discovery, a crucial stage in legal proceedings, is proving transformative. AI can automatically sift through vast volumes of documents, including applications, transcripts, and communications, rapidly identifying elements indicative of bias that would typically require extensive manual review by legal teams. This has the potential to significantly expedite the discovery process and reduce the time and resources spent by lawyers on manual document review.
However, the subtle influence of algorithm design cannot be overlooked. Minor tweaks to algorithm parameters can drastically alter the representation of bias in the data, highlighting the paramount importance of transparent and carefully designed algorithms in any legal investigation concerning discrimination. This requires a thoughtful approach to ensure fairness and equity in AI's role in uncovering bias.
AI can also unearth more nuanced patterns within applicant data, such as the correlation between socioeconomic status or geographic location with long-term admission trends. By uncovering these connections, AI can assist in understanding how subtle factors can perpetuate bias across generations of students.
AI's ability to conduct automated legal research can unearth precedents that support arguments of discrimination, ensuring that legal teams have a broader understanding of the legal landscape for their cases. Moreover, the quantitative metrics that AI can provide on bias within admissions processes empower universities to take a data-driven approach to enacting fairer admissions practices.
Yet, the rise of AI in law also presents complex ethical considerations, particularly concerning the privacy of student data. It is crucial to develop a framework that balances the innovative potential of AI with the need to safeguard individual rights and protect sensitive information.
Furthermore, AI can analyze the sentiment expressed in application essays and letters of recommendation, thereby identifying potential biases that might unduly favor certain demographics during the evaluation process. These insights can provide a more complete picture of how bias can manifest in otherwise seemingly objective parts of the admissions process.
The adoption of AI tools for legal document creation within law firms is increasing efficiency, but raises concerns about the quality of the outputs and the possibility of these tools inadvertently reinforcing pre-existing biases in legal language. This highlights the need for ongoing critical evaluation and human oversight in the application of AI within legal frameworks.
AI-Powered Investigation Tools Analyzing Discrimination Claims in White v
University of Idaho - Machine Learning Analysis of Document Collections Shows Discriminatory Language Evolution
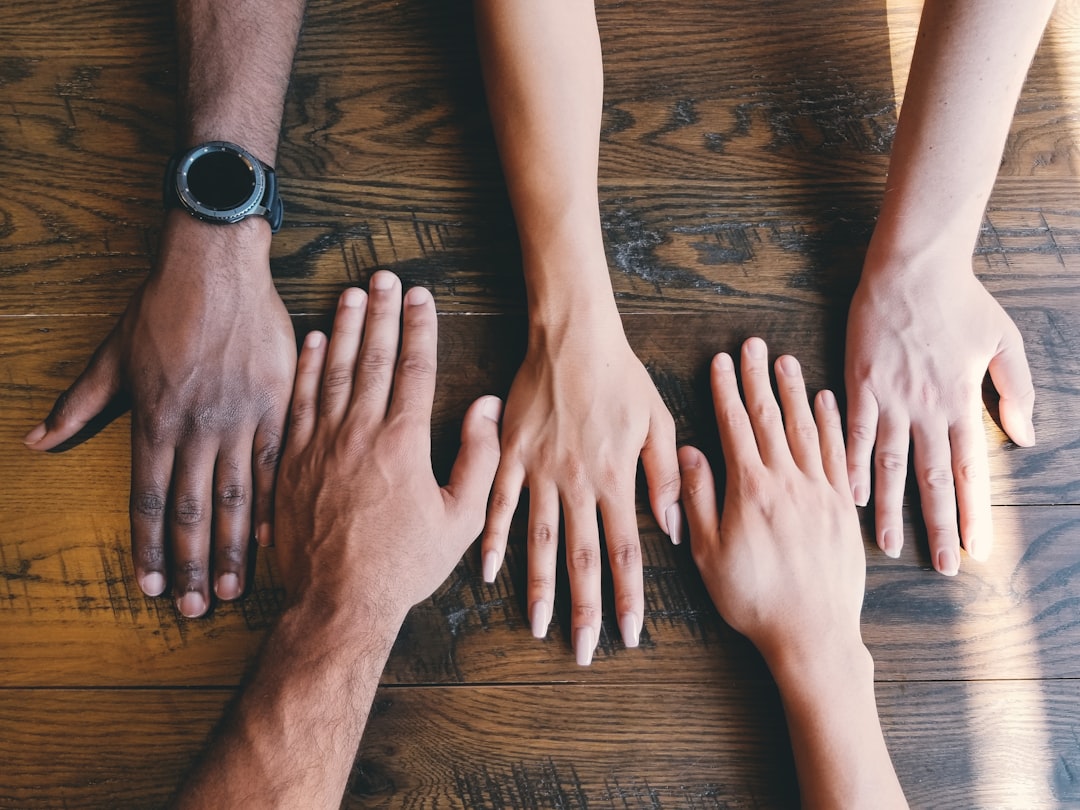
The use of machine learning to analyze large collections of documents is revealing how discriminatory language has changed over time within the legal field. This capability is particularly relevant in cases like White v. University of Idaho, where AI tools can help identify patterns and biases within the documents related to the claims of discrimination. While these AI-driven investigations can uncover hidden biases, it's crucial to acknowledge that the algorithms used can themselves introduce or exacerbate biases present in the data they are trained on.
The growing reliance on AI in legal discovery and research raises important ethical questions about its role in upholding fairness and equity. It's essential to carefully evaluate the design and application of these AI tools to ensure they don't unintentionally perpetuate existing inequities. As AI becomes more integrated into legal processes, including e-discovery, legal research, and even the creation of legal documents, careful consideration of ethical implications and potential biases must remain at the forefront. This balance between leveraging the power of AI for efficient and thorough investigations while mitigating its potential to reinforce discriminatory practices is a critical challenge facing the legal sector today. This critical examination of how AI is employed in legal contexts is vital to prevent the unintended consequences of algorithmic bias and maintain integrity in legal outcomes.
AI's growing role in legal processes, especially in document analysis within e-discovery and legal research, is revealing how historical biases can be subtly embedded within legal language and practices. While AI can significantly speed up the review of vast document collections, potentially reducing the time spent on manual tasks by a substantial margin, there's a risk of overlooking the implications of algorithmic decision-making.
The capability of AI to process natural language opens up new avenues for uncovering discriminatory language patterns that might otherwise go unnoticed. By analyzing documents at a scale previously unimaginable, AI can highlight how biases can affect legal arguments and even case outcomes. Furthermore, the ability of AI to conduct automated legal research doesn't just stop at predicting outcomes based on past cases but also enables lawyers to identify potential gaps in legal frameworks that may perpetuate discriminatory practices. This allows them to more effectively build their case and, perhaps, initiate legal reforms that address identified biases.
However, the integration of AI in law firms, particularly its role in document creation and compliance strategy adaptation, presents some interesting challenges. While AI can potentially synthesize legal changes and case law in real-time, keeping firms abreast of evolving legal landscapes, it raises questions about the accuracy of these AI-driven interpretations. Similarly, while AI can offer valuable insights into the emotional weight and sentiment of legal arguments or applicant statements, highlighting potential biases, relying heavily on AI without human oversight can lead to a lack of nuanced understanding and potentially exacerbate historical biases within the legal system.
The effectiveness of AI in combating discriminatory practices can also be seen in its capacity to analyze inconsistencies in admissions processes and provide quantitative data that supports claims of bias. However, ensuring fairness and transparency in these processes is essential. Small adjustments to the algorithms used for document analysis can significantly change the identification and classification of biased language, emphasizing the need for ongoing scrutiny and external audits to ensure accountability. As AI becomes increasingly integrated into legal practice, the legal community must remain vigilant, critically evaluating AI outputs and ensuring that AI tools serve the pursuit of justice and fairness rather than inadvertently perpetuating systemic biases. This also necessitates continuous training and education for legal professionals to effectively utilize and understand the limitations of AI in legal contexts.
AI-Powered Investigation Tools Analyzing Discrimination Claims in White v
University of Idaho - eDiscovery Tools Map Communication Networks in White v University of Idaho Case
In the White v. University of Idaho case, eDiscovery tools are proving instrumental in uncovering potential discriminatory practices by mapping communication networks within the university. These tools, used in the context of legal discovery, involve the collection and analysis of electronic communications like emails and social media data. By analyzing these communication networks, lawyers can build a more comprehensive understanding of interactions and potential discriminatory patterns that might otherwise remain hidden.
The incorporation of artificial intelligence into eDiscovery is accelerating the process of reviewing vast quantities of documents. AI-powered systems can quickly identify potentially problematic language or patterns within the collected data, significantly reducing the time and resources needed for manual document review. This efficiency is particularly useful in discrimination cases where a comprehensive understanding of communication dynamics can be crucial.
Despite these benefits, using AI in legal proceedings necessitates caution. AI algorithms, if not properly designed and monitored, can inadvertently amplify biases present within the data they are trained on. Therefore, ensuring the fairness and transparency of AI-driven eDiscovery processes is vital. The legal field needs to continuously assess the ethical implications of employing AI tools, striving to achieve a balance between harnessing the advantages of AI and mitigating its potential to perpetuate discriminatory practices. The continued use of AI in legal cases like White v. University of Idaho emphasizes the need for careful consideration and rigorous evaluation of the methods and outcomes of AI-driven investigations.
In cases like White v. University of Idaho, eDiscovery tools are playing a crucial role in mapping communication networks and analyzing data related to discrimination claims. These tools, now increasingly powered by AI, can handle the sheer volume of electronically stored information (ESI) generated in modern legal contexts, which includes everything from emails and databases to social media content. This efficiency is significant, as AI can process millions of documents in a fraction of the time it would take human reviewers.
One interesting aspect is how AI can identify patterns across large datasets that humans might miss. It can analyze communication networks and spot biases within university admissions processes that might otherwise go unnoticed. However, this capability also brings to light the critical importance of algorithm design. The way AI models are trained can inadvertently introduce or exacerbate biases present in the training data, requiring careful consideration, especially when dealing with sensitive issues like discrimination.
Furthermore, AI's natural language processing (NLP) capabilities are proving valuable in understanding the subtle nuances of legal language. It can analyze the evolution of discriminatory language over time, potentially influencing future legal arguments. This historical context, revealed through AI, helps to understand how past discriminatory practices may still impact current policies. Additionally, advanced e-discovery tools can cluster documents based on similarity, allowing legal teams to focus their efforts on the most relevant information.
The use of AI is also providing valuable quantitative insights into admissions trends and potential biases. This quantitative data can support claims of discrimination and potentially help universities implement fairer admissions practices. However, the use of AI in legal proceedings necessitates the development of robust accountability frameworks. We need to constantly evaluate the fairness and transparency of the algorithms employed to avoid inadvertently reinforcing existing biases.
AI is also enabling law firms to adapt to changes in the legal landscape in real-time, processing new laws and case law. While this offers opportunities for enhanced legal compliance, it also raises questions about the accuracy and reliability of AI-generated legal interpretations. Ultimately, the most effective approach is to leverage AI's strengths, its ability to process vast amounts of information, in collaboration with human expertise. AI can provide insights, but nuanced understanding and ethical considerations require human oversight to ensure fairness and avoid the perpetuation of biases within the legal system. The careful balance between AI's power and human judgment will be crucial in promoting fairness and integrity in legal outcomes.
AI-Powered Investigation Tools Analyzing Discrimination Claims in White v
University of Idaho - Natural Language Processing Identifies Key Discrimination Evidence Across Email Servers
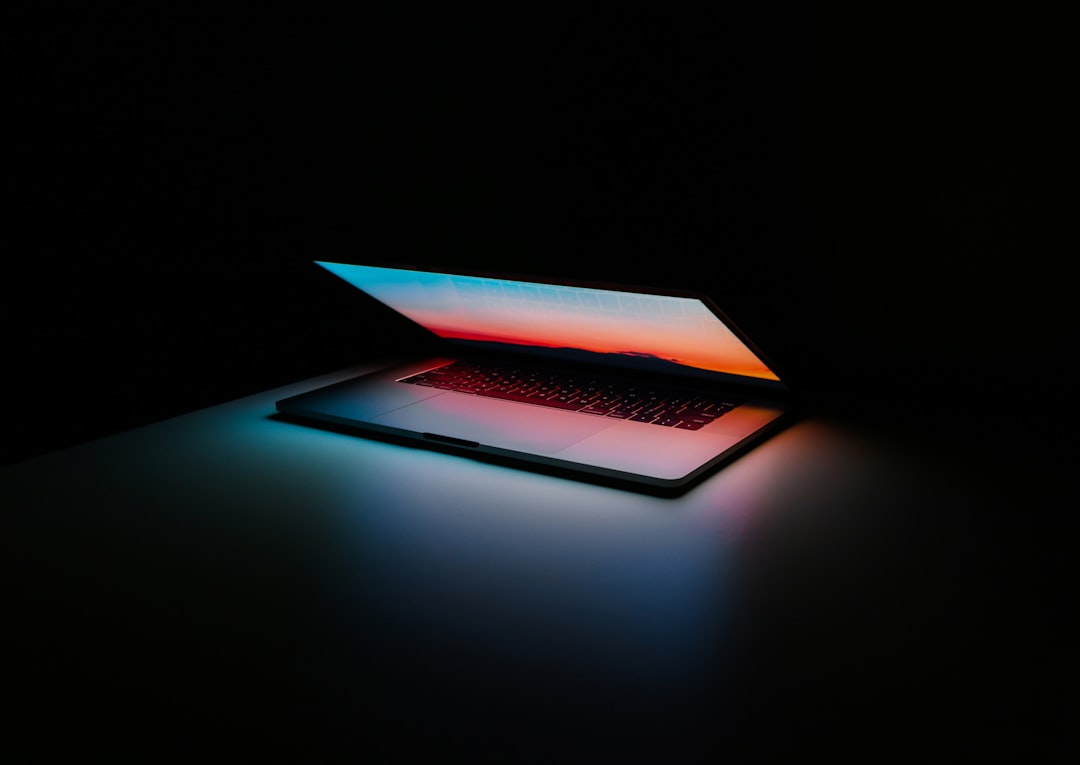
In legal contexts involving claims of discrimination, like the **White v. University of Idaho** case, Natural Language Processing (NLP) is increasingly utilized to unearth critical evidence. NLP can delve into massive email repositories, identifying subtle patterns and language that may indicate discriminatory practices. This capability offers a powerful tool for e-discovery, allowing lawyers to efficiently process large volumes of data and discover previously hidden biases.
However, relying on AI in such sensitive areas brings ethical considerations to the forefront. NLP systems, like other AI tools, can inadvertently mirror and even intensify pre-existing societal biases embedded within the data they're trained on. Consequently, there's a growing concern that the application of NLP could potentially perpetuate discrimination rather than combat it. To mitigate this risk, it's imperative that the design and implementation of these AI tools are approached with a keen awareness of potential biases and a strong commitment to fairness and transparency. The legal field must strike a balance between leveraging the speed and analytical power of NLP and ensuring the integrity of the legal process, preventing the unintentional reinforcement of unfair or discriminatory outcomes. A careful examination of how NLP is applied within legal frameworks is crucial for ensuring these tools contribute to a more just and equitable legal landscape.
1. **Accelerated Document Review**: AI's ability to sift through massive volumes of documents in eDiscovery has revolutionized the speed of legal discovery. This rapid processing allows legal teams to shift their focus from tedious manual reviews towards interpreting the data and uncovering meaningful patterns.
2. **Visualizing Communication Networks**: AI-powered tools are revealing the hidden connections within communication networks, especially pertinent in cases like White v. University of Idaho where understanding organizational dynamics is critical. This visual mapping can uncover potential patterns of discriminatory behavior that might otherwise go undetected.
3. **Understanding Language Nuances**: Natural language processing within these AI systems is proving useful in deciphering the subtle nuances of legal language. By analyzing the evolution of language over time, we can gain valuable insights into how discriminatory language has shifted and persisted within the legal realm.
4. **The Delicate Balance of Algorithm Design**: The impact of even subtle changes in AI algorithm design can significantly alter the identification of biased language, highlighting the importance of rigorous oversight in their development. We must be cautious of how algorithms are trained to ensure they don't inadvertently reinforce existing societal prejudices.
5. **Quantifying Bias**: AI-powered analysis provides a unique opportunity to quantify patterns within admissions trends and provide evidence for claims of discrimination. This quantitative approach strengthens legal arguments and provides universities with data-driven insights to enact fairer admissions policies.
6. **Navigating the Ethical Landscape of AI**: The increasing use of AI in law raises complex ethical questions, especially regarding data privacy and the potential for unintentional bias in algorithmic decision-making. Striking a balance between utilizing AI's capabilities and safeguarding ethical considerations is crucial for maintaining the integrity of the legal system.
7. **Historical Context in Discrimination Cases**: AI allows us to delve into the historical context of discrimination cases, providing a broader understanding of how past biases might still influence current practices. This historical perspective can inform legal arguments and inform policy changes aimed at promoting fairness.
8. **Adapting to a Changing Legal Landscape**: The ability of AI to process new laws and case law in real-time allows law firms to maintain a more dynamic and responsive approach to legal compliance. However, relying on AI-generated legal interpretations requires careful consideration of their accuracy and reliability.
9. **AI's Potential for Bias in Legal Drafting**: While AI assists in generating legal documents, we must be mindful of the possibility that it might inadvertently perpetuate pre-existing biases in legal language. Human review and oversight remain essential to ensure fairness and equity in the outputs of AI-driven document creation.
10. **Wider Applicability Beyond the Legal Arena**: The insights gleaned from using AI in cases like White v. University of Idaho can be valuable across various sectors. These techniques could be adopted to enhance fairness in other contexts where discrimination claims are prevalent, leading to more equitable practices across various domains.
AI-Powered Investigation Tools Analyzing Discrimination Claims in White v
University of Idaho - Automated Legal Research Systems Track Similar Cases and Legal Precedents
Automated legal research systems are now a cornerstone of legal practice, using AI to quickly find similar cases and related legal precedents. These systems employ powerful techniques like natural language processing and machine learning to sift through mountains of legal data, pulling out crucial information much faster than traditional methods. This not only speeds up the process but also frees up lawyers to spend more time crafting legal strategy and interacting with clients.
However, as we increasingly rely on AI in legal research, we must be mindful of the potential for bias embedded within these AI systems themselves. How these algorithms are designed and the data they're trained on can subtly influence their results, potentially leading to skewed outcomes. Striking a balance between embracing the efficiency AI offers and mitigating the risk of perpetuating bias is crucial. The goal is to ensure that while AI empowers lawyers with quicker access to precedent, it doesn't unintentionally skew the fairness and equity of the legal process. The application of AI in law must be carefully managed to promote a just and equitable legal system.
1. **Leveraging Historical Legal Trends**: AI-powered legal research tools can delve into vast repositories of historical case law, identifying patterns of bias that might have influenced past legal decisions and could potentially affect current ones. This capability allows legal professionals to present a more robust and contextually informed argument by drawing on broader historical trends.
2. **Streamlining E-Discovery with Automation**: AI-driven systems can dramatically accelerate the e-discovery process by automatically sifting through enormous document collections. Instead of weeks spent manually reviewing documents, lawyers can potentially access relevant information in a matter of minutes. While this efficiency is undoubtedly beneficial, it also raises concerns about the potential for overlooking subtle details during the automated process.
3. **Detecting Bias Within Legal Language**: AI's natural language processing capabilities allow for a more in-depth examination of legal texts. It can identify patterns of biased language used within legal documents over time, revealing how subtle biases may have perpetuated discriminatory practices in the past and possibly in the present.
4. **The Challenge of Algorithmic Bias**: The efficacy of AI systems relies heavily on the datasets they are trained on. If those training datasets contain inherent biases, there is a risk that the output of the AI model will reflect and amplify those biases. Thus, it becomes critically important to carefully curate and evaluate the training data used to develop AI tools for legal purposes, in order to ensure ethical and equitable outcomes.
5. **Predicting Legal Outcomes with AI Models**: AI models trained on massive legal databases can provide predictions regarding the potential outcomes of legal disputes. In cases similar to White v. University of Idaho, this predictive capability can offer valuable insights, helping to inform case strategy and resource allocation. However, overreliance on these predictive models without a full understanding of their limitations could be problematic.
6. **Mapping Interconnections Within Legal Networks**: Certain AI tools in legal research can visualize relationships between cases, lawyers, and parties involved in legal actions. These visualizations can expose previously hidden patterns that might suggest systemic biases or cooperative efforts amongst individuals influencing legal outcomes. However, it's crucial to interpret such visualizations with caution, acknowledging the complexity of legal systems and the potential for misinterpretations.
7. **Keeping Up with Evolving Legal Landscapes**: Some AI tools can continuously monitor changes in laws and legal precedents, providing law firms with real-time updates. This constant monitoring allows for a more dynamic and responsive approach to legal practice. Nonetheless, it remains crucial to critically evaluate the reliability and accuracy of these real-time updates, as even AI systems can make errors in interpretation.
8. **Gaining Insights from Sentiment Analysis**: The integration of advanced sentiment analysis techniques allows for the assessment of emotional tones within communication relevant to legal cases. This can be incredibly useful in discrimination claims where the motives and sentiments of parties play a pivotal role. Yet, interpreting sentiment can be complex, and caution is needed to avoid misinterpreting subtle nuances or imposing biases onto communication.
9. **Adapting Research to Specific Case Requirements**: AI can tailor legal research tools to the specific needs of a particular case, allowing users to input parameters and questions that reflect their unique circumstances. This customized approach can potentially lead to more targeted and relevant research results. However, ensuring that the customization options are well-designed and do not introduce biases in their own right is crucial.
10. **Enhancing Legal Compliance with AI**: As regulatory environments become increasingly complex, AI-driven tools can assist law firms in maintaining legal compliance. By processing large volumes of documents, they can identify potential risks of non-compliance, enabling proactive measures to address potential legal obligations. However, AI tools are only as good as the data and algorithms they employ, and continued human oversight is necessary to ensure the accuracy and fairness of the outputs.
AI-Powered Investigation Tools Analyzing Discrimination Claims in White v
University of Idaho - AI Document Review Platforms Process Witness Statements and Administrative Records
AI document review platforms are playing a growing role in legal processes, especially when it comes to handling witness statements and administrative records. These platforms utilize AI to streamline the review of large document sets, accelerating the discovery process and reducing the time spent on manual tasks. They can analyze documents to find key issues, potentially revealing hidden biases or compliance violations that might be missed during manual review. This enhanced speed and accuracy can improve the quality of legal investigations. Yet, it's essential to remember that AI systems can, at times, reflect existing biases in the data they are trained on. This means that the design and implementation of these tools need constant scrutiny to avoid inadvertently promoting unfair or discriminatory outcomes. As AI adoption in law becomes more widespread, striking a balance between leveraging its power and ensuring human oversight is crucial. This is necessary to avoid potential biases within algorithmic decision-making and maintain fairness throughout the legal process.
AI document review platforms are transforming how legal teams handle large volumes of documents, particularly witness statements and administrative records. These platforms can drastically reduce the time spent on manual review, potentially shrinking a weeks-long task into a matter of days. This efficiency is particularly valuable in cases where quick turnaround is crucial.
One notable capability of these AI tools is their ability to identify patterns in language, such as the subtle evolution of discriminatory language within historical records. This type of pattern recognition can be extremely helpful in building a stronger case based on historical context, something that would be significantly harder to achieve through purely manual review.
Furthermore, these systems often include features that automatically group similar documents together, based on content. This means lawyers can quickly focus their analysis on the most relevant information, rather than manually sorting through thousands of files.
AI’s advancements in natural language processing (NLP) are enhancing the ability to understand the context and sentiment behind witness statements. This enables a more nuanced interpretation of potential biases or discriminatory intentions, potentially revealing evidence that might otherwise go unnoticed.
These platforms also allow for a more data-driven approach to legal analysis, offering both qualitative and quantitative insights into trends within administrative records. This capability could uncover systemic biases that haven't been readily apparent through traditional means, such as within admissions processes or university policy enforcement.
However, the legal field must remain cautious regarding the inherent potential for bias within AI systems. The design and implementation of these algorithms are critical, as seemingly small changes in their parameters can significantly affect how they identify biased language. Transparency and constant monitoring are essential to ensure fairness and prevent any unintended amplification of existing biases within the data.
By analyzing historical trends within administrative records, AI can provide a crucial context for understanding how current discrimination claims fit into a larger picture of institutional practices. This broader perspective can offer valuable insights for developing future legal strategies.
AI's ability to process massive datasets can also help detect implicit biases that might exist in communication patterns amongst university staff and officials. These biases, often subtle and easily missed by human auditors, can be highlighted through AI's analytical capabilities.
Keeping lawyers up-to-date on changes in case law and legal precedents is another area where AI tools shine. Real-time updates provided by these systems eliminate the typical delays associated with traditional legal research. This continuous flow of information can be especially relevant when evaluating discrimination claims in light of evolving legal standards.
Ultimately, the deployment of AI in legal document review highlights the growing importance of ethical considerations in the design and application of these technologies. While they offer significant benefits, algorithms that lack proper checks and balances can unintentionally perpetuate biases embedded in the data they are trained on. This, in turn, can negatively impact the fairness of legal outcomes if not proactively addressed through a robust ethical framework.
Automate legal research, eDiscovery, and precedent analysis - Let our AI Legal Assistant handle the complexity. (Get started for free)
More Posts from legalpdf.io: