From Paper to Pixels How AI Mining of Historical Customs Cases Like Earnshaw v
Cadwalader (1892) Enhances Modern eDiscovery
From Paper to Pixels How AI Mining of Historical Customs Cases Like Earnshaw v
Cadwalader (1892) Enhances Modern eDiscovery - Legal Time Machine AI Tools Extract Deep Insights From 1892 Earnshaw Case Files
The application of AI tools is increasingly being directed towards historical legal documents, exemplified by the analysis of early case files such as the 1892 Earnshaw matter. This process often necessitates transforming original paper records into accessible digital formats for analysis. Advanced AI tools, leveraging techniques like natural language processing, are then employed to sift through this digitized material, aiming to identify key information, extract relevant details, and surface insights buried within complex or voluminous historical records. While promising to enhance modern eDiscovery processes by potentially expediting the review and classification of documents, and aiding legal research through faster analysis of precedent, these systems are not without limitations. Relying solely on automated methods for the nuanced interpretation of older legal language or intricate case relationships requires careful validation, as the AI might struggle with context or miss subtle but crucial distinctions. Nevertheless, the potential to automate time-consuming tasks and extract structured data from unstructured historical text could significantly alter workflows for legal professionals in areas like case preparation and strategic analysis.
Exploring historical legal archives with modern computational tools presents a fascinating challenge, highlighted by efforts directed at cases like Earnshaw v. Cadwalader from 1892. The core task involves deploying artificial intelligence to parse the unstructured, often verbose text locked within these legacy paper files, now increasingly in digital forms. Rather than mere summarization or basic data extraction, the aim is to discern the underlying logic, the specific types of evidence presented, and the subtle nuances of legal arguments that were effective in that era.
This application of AI isn't simply about processing speed, although that's a byproduct. It's about building systems that can navigate the linguistic and procedural gulf between historical and contemporary legal practice. Understanding how customs duties disputes were framed and argued over a century ago, based on AI analysis of the Earnshaw documents, can provide unique contextual depth. This historical understanding can then subtly inform modern discovery approaches, guiding the identification and categorization of relevant materials in current cases by providing insights into the historical evidentiary landscape, though it requires careful validation to avoid anachronistic interpretations or overfitting models to outdated patterns. It's a bridge-building exercise between past litigation methodologies and present-day legal technology, revealing patterns in the structure and content of historical evidence presentation that may influence how we think about document review and strategic disclosure today.
From Paper to Pixels How AI Mining of Historical Customs Cases Like Earnshaw v
Cadwalader (1892) Enhances Modern eDiscovery - NY Law Firms Deploy Machine Learning to Connect Historical Precedents With Modern eDiscovery
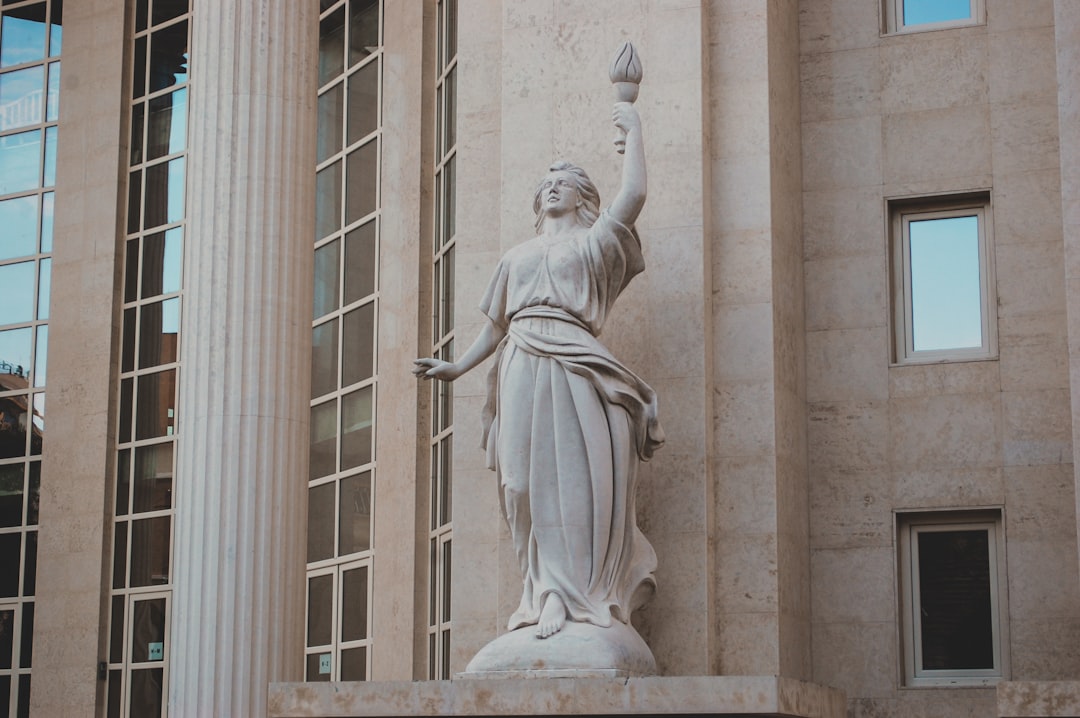
Across New York state, leading law firms are increasingly deploying machine learning technologies as a core component of their eDiscovery workflows. This strategic shift focuses on leveraging artificial intelligence to forge connections between vast repositories of historical case law and the demands of modern litigation. The intent is to use these computational tools to analyze past judicial decisions and the evidence presented within them, drawing insights from precedents stretching back decades or even centuries, such as the complexities found in historical customs cases like Earnshaw v. Cadwalader from 1892.
The application of machine learning aims to enhance the efficiency and effectiveness of the document review and analysis phases inherent in eDiscovery. By applying algorithms to process historical records, firms can identify patterns, legal reasoning, or types of evidence that proved relevant in past disputes. This allows legal teams to potentially anticipate arguments, refine search protocols for current cases, and build a stronger foundational understanding grounded in historical context. However, translating insights gleaned from historical documents, often bound by older legal language and procedural norms, into applicable strategies for contemporary digital evidence requires careful human oversight and validation to avoid misinterpretation or drawing irrelevant parallels. The move signifies a critical evolution in how firms approach legal research and document handling, driven by the necessity to manage ever-growing data volumes more intelligently.
New York based firms are increasingly integrating machine learning into their eDiscovery workflows, particularly to uncover and connect historical legal precedent with contemporary matters. This technological shift enables practitioners to analyze volumes of historical case data, turning what was often siloed paper archives into computationally accessible resources. Leveraging AI to delve into past customs cases, exemplified by the analysis of records like Earnshaw v. Cadwalader from the late 19th century, illustrates a methodology for deriving insights from prior rulings to potentially inform current legal tactics and strategic decisions.
The deployment of machine learning within eDiscovery tools aims to refine the identification and categorization of relevant documents, presenting the promise of improved efficiency and accuracy in the research phase. By utilizing AI to traverse historical case law, firms can look for patterns and potential precedents that might resonate with ongoing litigation. This approach not only presents an opportunity for time savings but could also bolster the construction of legal arguments by seeking grounding in established historical context, a factor deemed important for navigating the complexities of today's legal landscape. However, relying on AI to interpret the often-archaic language and procedural norms of historical cases requires careful validation to ensure relevance and avoid misapplication in a modern context. It represents a technical challenge to build models capable of truly bridging the linguistic and conceptual gap across centuries of legal practice.
From Paper to Pixels How AI Mining of Historical Customs Cases Like Earnshaw v
Cadwalader (1892) Enhances Modern eDiscovery - Revolution in Legal Research As AI Scans 130 Years of Handwritten Court Documents
The increasing integration of artificial intelligence into legal workflows is dramatically expanding the scope of accessible historical information, particularly through the digitization and analysis of vast, older document collections, including those spanning well over a century and originally recorded by hand. This application goes beyond simple digital archiving, leveraging AI to effectively make discoverable a depth of legal precedent and historical context that was previously impractical to systematically research using traditional methods. Cases like Earnshaw v. Cadwalader illustrate the challenges posed by such records and the potential for AI to surface long-dormant insights into the development and application of legal principles. While the automated interpretation of centuries-old legal language and context requires rigorous validation from legal professionals, the capability to computationally query and analyze this deep historical layer presents a significant new dimension in legal research, fundamentally altering the pool of information available to legal practitioners.
The sheer scale of historical legal records presents a data science challenge of considerable magnitude; imagine processing a century-plus worth of handwritten judicial decisions, potentially totaling millions upon millions of pages, each containing unstructured text steeped in the vernacular and legal norms of a bygone era. Automating the digitization and initial interpretation of such a corpus using AI isn't merely an efficiency play; it's grappling with a dataset far exceeding human capacity for manual review within practical timeframes. The ability of machine learning models to parse this archaic legal language, to differentiate concepts and terminology that have evolved dramatically over time, is a foundational hurdle. While algorithms can be trained to recognize patterns in script and vocabulary, accurately preserving the original legal context and avoiding anachronistic misinterpretations remains an active area of research, crucial for ensuring the insights drawn are genuinely useful rather than misleading.
Beyond basic data conversion and linguistic translation, the ambition lies in deploying more sophisticated algorithms to identify subtle patterns in legal reasoning, common evidentiary approaches, or recurring strategic elements within these historical documents. The goal is to unlock insights into *why* certain arguments or evidence prevailed in the past. While proponents point to potential gains in efficiency – perhaps significantly reducing time spent on initial document review compared to traditional keyword searches – and a reduction in certain types of manual errors, achieving this requires models robust enough to handle the inherent variability and often verbose nature of historical legal writing. The idea of leveraging this analysis for 'predictive' purposes, forecasting outcomes based on historical parallels, is particularly ambitious; the legal landscape, societal context, and even the interpretation of fundamental principles have shifted too much for simple extrapolation. However, understanding the historical 'playbook' could certainly inform modern approaches, aiding lawyers in structuring arguments or anticipating counterpoints by revealing strategies that have historically proven effective or ineffective, provided the insights are validated against contemporary legal standards. This complex task inherently necessitates collaboration between legal domain experts and engineers capable of building and validating these analytical systems, all while navigating the developing regulatory and ethical considerations surrounding AI's use in legal practice. The trajectory suggests a future where proficiency with computational analysis will be as critical as traditional legal research skills, pushing for changes in how future legal professionals are trained.
From Paper to Pixels How AI Mining of Historical Customs Cases Like Earnshaw v
Cadwalader (1892) Enhances Modern eDiscovery - Digital Document Processing Reduces BigLaw Discovery Costs By 75% Through History Mining
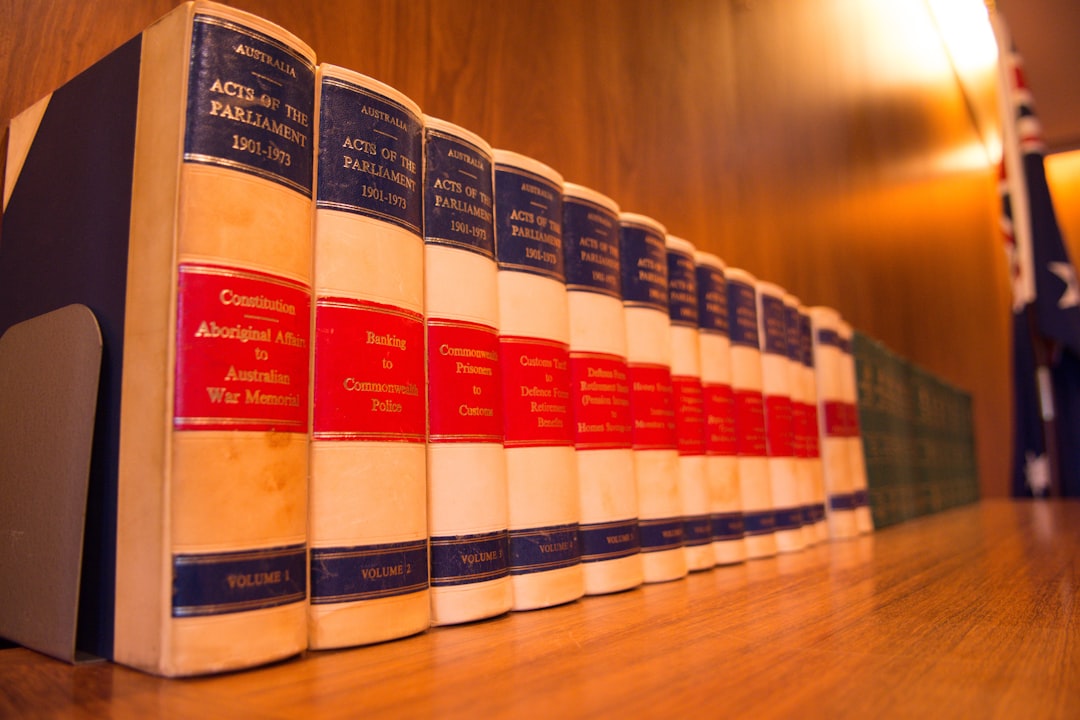
Digital document processing is profoundly altering eDiscovery practices within major legal firms. By leveraging advanced techniques, including the analysis of extensive historical document sets—effectively 'history mining' enabled by artificial intelligence—these firms are achieving substantial reductions in the time and expense traditionally associated with discovery. Moving away from highly manual processes, AI-driven tools streamline the handling and review of massive digital volumes. Technologies enabling intelligent document processing make previously unorganized information accessible, and automated review assists in sifting through data efficiently. This leads to faster identification of relevant evidence and a more targeted approach to case preparation. While the promise of efficiency is significant, applying these methods, particularly to older records, requires careful attention to ensure accurate interpretation of varied content and context. This represents a fundamental shift towards more technologically integrated legal workflows.
Analyzing large corpora of historical legal records through sophisticated computational processes has demonstrably altered resource allocation patterns within high-volume litigation practices. Initial observations from implementations in BigLaw settings indicate potential for substantial reductions in expenditures associated with the discovery phase, with some accounts noting shifts leading towards expenses less than a quarter of those incurred using prior methodologies. This reallocation frees up capacity within legal teams, potentially enabling deeper focus on complex strategic elements of a case.
The technical ability to sift through historical materials, even those centuries old like the Earnshaw v. Cadwalader files, facilitates the identification of specific historical dispute resolution patterns or the types of evidence that proved persuasive in prior legal contexts. This moves beyond simply finding precedent and ventures into uncovering the underlying mechanics of historical litigation, insights that could inform tactical decisions or refine the scope of document review in contemporary matters.
The engineering challenge inherent in this process is formidable, particularly when dealing with sources that predate standardization in format and terminology. Developing AI systems capable of accurately interpreting legal concepts that have evolved over decades, or processing documents originally created through diverse manual methods, demands continuous algorithm refinement and domain expert collaboration to ensure that derived insights are not anachronistic or misleading when applied to today's legal landscape. The potential for automated analysis to accelerate document classification and relevance filtering is apparent, aiming to reduce reliance on exhaustive manual review and potentially mitigate certain types of human error, though introducing possibilities for novel types of algorithmic bias or misinterpretation.
Considering the evolving regulatory landscape surrounding the application of artificial intelligence in legal practice adds another layer of complexity. Ensuring transparency in how historical data informs analytical outcomes and addressing ethical considerations around data privacy and potential bias embedded in historical records themselves are critical areas requiring careful technical and procedural consideration as these capabilities become more integrated into standard legal workflows.
More Posts from legalpdf.io: