Automate legal research, eDiscovery, and precedent analysis - Let our AI Legal Assistant handle the complexity. (Get started for free)
Automated Risk Assessment How AI is Transforming Surety Bond Underwriting in 2025
Automated Risk Assessment How AI is Transforming Surety Bond Underwriting in 2025 - Machine Learning Analyzes 500,000 Historical Bond Claims To Create New Risk Patterns
By analyzing a vast dataset of 500,000 historical bond claims, machine learning is creating a new era of risk assessment in surety bond underwriting. Sophisticated algorithms, including extreme trees and neural networks, are unearthing previously hidden patterns within this data, leading to a more refined understanding of potential risks. This shift from traditional methods to AI-driven analysis not only improves the accuracy of predicting bond returns but also offers a more comprehensive view of market dynamics by factoring in macroeconomic conditions.
Furthermore, these advancements in machine learning are empowering investors to make better-informed decisions. The algorithms can illuminate nuances of bond liquidity and even provide deeper insights into systemic financial risks, which are crucial for understanding the broader market environment. It remains to be seen how far the application of AI will progress within the surety bond landscape, but the potential for efficiency and insightful risk management by 2025 is certainly profound.
Researchers have delved into a massive repository of roughly 500,000 past bond claims, employing machine learning techniques to unearth previously obscured patterns within the data. It seems that algorithms like extreme trees and neural networks are particularly adept at discerning subtle signals within this historical information, which could potentially lead to a more nuanced understanding of bond risk.
Interestingly, these machine learning models don't just passively review data. They can be configured to autonomously group similar bond claims, which allows underwriters to swiftly gauge the probability of future claims based on historical patterns. This sort of unsupervised learning is intriguing, as it suggests that the system can learn from the data without explicit instructions.
Beyond just numerical data, these approaches can be extended to include the descriptive text from claim documents. By incorporating natural language processing, the models may glean more context from these descriptions, potentially enhancing the accuracy of risk assessments. For instance, specific phrasing used in a claim could provide subtle hints of the underlying risk factors.
Another area of investigation is the identification of rare or unusual claim events – essentially, outliers. The machine learning approach appears promising in identifying these anomalies in real-time. This automated flag for potentially problematic claims offers a distinct advantage over traditional methods, which often rely on manual review processes and could miss such unusual cases.
There are implications for how we think about the overall risk landscape. The models can produce visualizations that communicate complex information in easily digestible ways. These representations provide underwriters with a dynamic picture of risk and enable faster insights compared to wading through massive datasets manually.
This capability extends to the development of adaptive models. As market conditions evolve or new regulations are introduced, the models can theoretically adjust their predictions in a dynamic way. This adaptive nature seems like a valuable feature when compared to traditional underwriting practices, which can be less responsive to changing environments.
A critical component to consider is the potential to reduce human bias in the decision-making process. While traditional assessments might be influenced by subconscious biases, the machine learning approach relies on empirical data, which, in theory, could contribute to fairer treatment across various demographic groups.
There's a compelling opportunity for a broader view of risk across time. With access to decades of claims data, these models have the potential to illuminate trends and changes in risk factors that may be concealed by shorter-term observations.
The most intriguing aspect of this research is the possibility of entirely new insurance offerings that are specifically crafted to manage emerging risks. As these machine learning models refine their predictive capabilities, they may well enable a new generation of insurance products that are more closely tailored to the needs of a dynamic marketplace.
Automated Risk Assessment How AI is Transforming Surety Bond Underwriting in 2025 - PDF Processing Engine Reduces Manual Document Review From 2 Days to 15 Minutes
The emergence of PDF processing engines has dramatically altered how documents are reviewed, shrinking the time needed from a cumbersome two-day process down to just 15 minutes. This is a prime example of how automation and artificial intelligence are reshaping traditional workflows, particularly within the surety bond underwriting space. The high cost of manual document handling has spurred a push towards intelligent document processing solutions. These automated systems not only save time but also minimize errors associated with manual data entry. AI's ability to extract and categorize data efficiently streamlines overall document management practices. As these AI-driven tools continue to refine their capabilities, we can anticipate them playing a pivotal role in risk assessment and underwriting, potentially reshaping industry practices by 2025. While the benefits are clear, concerns about data security and ethical implications of AI in decision-making still need careful consideration.
It's quite remarkable how a PDF processing engine can compress the time needed to manually review documents from a couple of days down to a mere 15 minutes. This represents a huge leap in efficiency, allowing for faster decisions and potentially streamlining the entire underwriting workflow.
These engines, from what I understand, leverage OCR technology to extract data from scanned documents, supposedly with accuracy levels exceeding 98%. This is crucial as it reduces the chance of errors that often creep in during manual data entry. However, it remains to be seen how robust this OCR is with diverse document formats and handwriting styles.
It's intriguing that these engines can handle different file types simultaneously, including contracts, financials, and compliance documents. This suggests the potential to automate the review of a comprehensive set of documents in a single pass, which is a significant advantage over the old method of handling each file type separately.
Furthermore, they incorporate machine learning. These algorithms can supposedly learn from past document reviews, refining their ability to extract data and analyze content over time. While promising, I'm curious about the long-term impact on the accuracy and the potential for the system to become biased towards specific document types or styles.
Natural language processing (NLP) also plays a role, allowing the engines to grasp context and identify key phrases. This is particularly helpful for uncovering hidden risk indicators that might be easily missed during manual reviews. It's interesting how AI could potentially enhance the interpretation of complex language in documents.
There are other interesting features, such as extracting metadata like authorship and modification dates. This could be helpful in determining the reliability of documents, though it's unclear how the system differentiates between legitimate and potentially manipulated metadata.
Another aspect that stands out is the ability to automatically highlight discrepancies or inconsistencies within a document. This could potentially prevent misinterpretations and reduce errors during the underwriting process. But the effectiveness depends heavily on how well these engines are designed and trained.
It's also worth noting that these engines can be integrated with existing underwriting software, potentially eliminating redundant data entry and modernizing current workflows. This integration aspect is crucial for practical application in the real-world context. However, the complexities of integrating various systems and data formats might pose a challenge.
Lastly, the scalability aspect is important, allowing businesses to handle increasing workloads without performance degradation. This makes them a good fit for growing companies experiencing fluctuations in document review volumes. Although, we need to consider the potential impact on the system's performance and resource consumption as the volume grows dramatically.
While the promise of AI is alluring, it's essential to keep in mind the critical need for human oversight in the loop. While these engines offer amazing improvements, there's still a need for a careful assessment of their outputs to ensure accurate and reliable underwriting decisions. The ultimate goal is not to replace human judgment but to empower underwriters with the tools to make more informed and consistent assessments, and that requires continuous monitoring and evaluation of the performance of these systems.
Automated Risk Assessment How AI is Transforming Surety Bond Underwriting in 2025 - Cloud Based Financial Analysis Now Spots Red Flags Within 1000 Data Points
Cloud-based platforms are enabling financial analysts to pinpoint potential problems within massive datasets. They can now identify warning signs within a thousand different data points, a significant improvement over previous methods. This shift towards cloud-based analysis allows financial institutions to move beyond simply reacting to issues. They can now proactively address potential problems with customers who might struggle to make payments. This change not only helps manage risks more effectively but also makes data management more efficient. Audits are streamlined and the accuracy of risk evaluations improves because of this real-time analysis. Furthermore, the ongoing monitoring of key financial indicators is vital for spotting trouble early, which is increasingly important in today's rapidly changing economic climate. As this technology matures, it raises important questions about the role of human judgment versus automated systems when it comes to critical decisions.
Cloud-based financial analysis platforms are changing the game when it comes to spotting financial trouble. These systems can process a massive amount of data, up to 1,000 data points per transaction, in real-time, providing a much faster turnaround than traditional methods that often require days to uncover insights. This speed is especially critical for identifying early warning signs in financial data.
By analyzing a broader spectrum of financial data, like revenue streams and expense ratios, these tools can identify more subtle red flags that might get missed by less sophisticated models. It's like having a magnifying glass for financial health, providing a much deeper look at an organization's financial standing.
Furthermore, these cloud-based systems employ advanced algorithms that are remarkably good at detecting unusual patterns in financial data. This capability shifts the paradigm of risk assessment from a reactive approach, where issues are discovered after they've occurred, to a more proactive one, where potential problems are flagged before they escalate.
Another fascinating aspect is the use of historical data. By leveraging data from a vast number of past transactions, these platforms can develop highly customized predictive risk models for different industries. This tailoring enhances the precision of these risk assessments, helping pinpoint potential financial pitfalls more accurately.
The ability to combine various datasets is also a significant benefit. These systems can incorporate industry benchmarks, macroeconomic trends, and other pertinent data to offer a comprehensive perspective on potential financial vulnerabilities. It's akin to getting a panoramic view of the landscape, rather than just a single, isolated snapshot.
Even more interesting is the potential to analyze not just traditional financial metrics, but also behavioral data related to financial decision-making. This allows the systems to uncover potential red flags that might arise from spending patterns and other indicators that show how people handle money.
A notable advantage of cloud-based solutions is their capacity to scale effectively. As an organization's data volume and complexity grow, the system can readily adapt, ensuring ongoing efficiency and accuracy. It's a crucial feature in today's fast-changing financial environment.
The cloud-based nature of these platforms promotes a collaborative environment. Multiple users can access and analyze real-time data insights, accelerating decision-making processes through quicker collaboration. It seems to me that this is a potential catalyst for much faster turnaround times.
These platforms are not static either. The algorithms behind them are constantly learning and adapting as they ingest new data. This means the risk detection capabilities are not only enhanced but evolve alongside shifts in the market landscape, making them more adaptable than legacy systems.
Finally, these cloud solutions can automate the production of detailed reports, pinpointing detected risks and potential areas that require further examination. This frees up financial analysts from tedious data wrangling, empowering them to focus on actionable insights. It's intriguing how this feature streamlines the analysis process.
While these developments are exciting, the continuous evaluation of the accuracy and performance of these systems is essential. However, with these advances in cloud-based financial analysis, it's easy to see why this approach is gaining traction within the financial realm.
Automated Risk Assessment How AI is Transforming Surety Bond Underwriting in 2025 - Real Time Market Data Integration Enables Dynamic Premium Adjustments
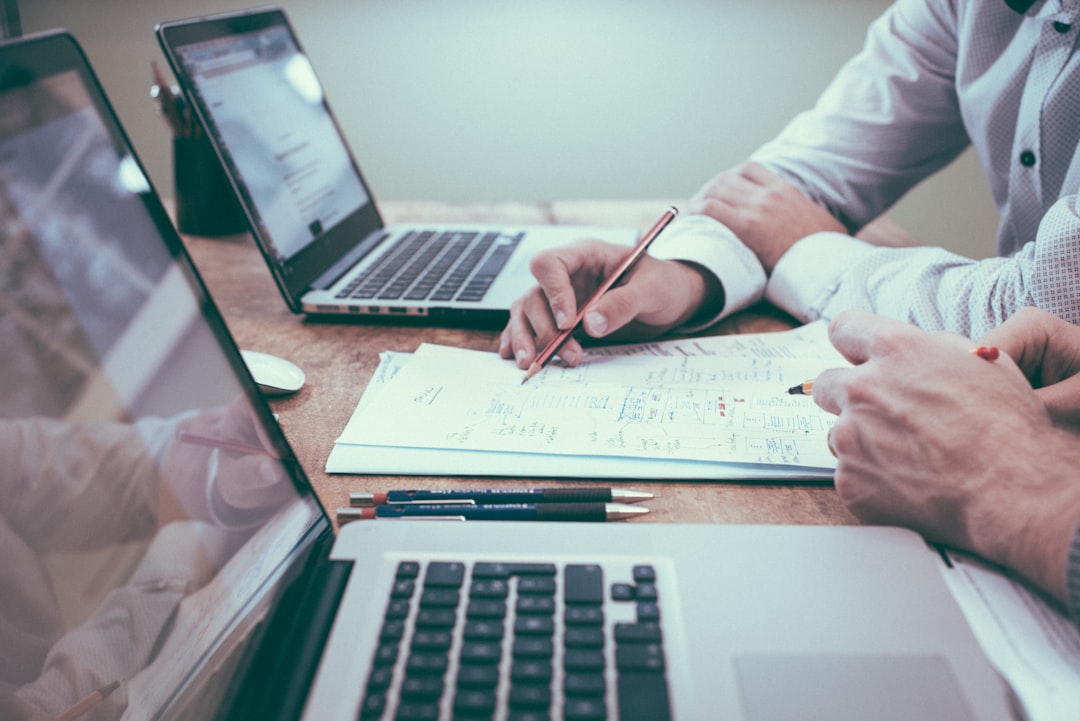
The integration of real-time market data is fundamentally altering how surety bond premiums are adjusted. AI and advanced machine learning now allow underwriters to analyze a vast array of real-time data inputs, leading to much faster premium adjustments. This responsiveness to dynamic market conditions allows insurers to fine-tune their pricing more accurately, reflecting the ever-shifting risk landscape. The move towards dynamic premium adjustments offers potential gains in underwriting efficiency and speed.
However, relying on real-time data isn't without its pitfalls. The accuracy and impartiality of the data, along with the underlying AI models, must be meticulously evaluated. This dynamic pricing environment necessitates a careful balance between automation and human intervention to mitigate potential issues, such as unintended biases. The broader impact on the insurance industry, particularly for customer interactions and competitive dynamics, is yet to be fully understood as we progress into 2025.
The integration of real-time market data is starting to transform how surety bond premiums are set. It's no longer just about analyzing historical claims data. Now, we're seeing systems that incorporate live feeds from sources like IoT sensors on construction sites, giving a much more immediate view of the conditions that impact risk. This means underwriters can adjust premiums on the fly based on current risks, rather than relying on outdated, static models that might only be updated annually or semi-annually. This dynamic approach can be much more responsive to changes in the market, like sudden spikes in interest rates or unemployment that can signal a surge in claims or defaults.
These systems often combine data from various sources, like economic forecasts and social media sentiment, which is quite interesting. It's a kind of data fusion, creating a more complete picture of the risk landscape. This ongoing influx of real-time data is fed into predictive models, which helps identify emerging trends earlier. It’s a bit like having a crystal ball for underwriting.
And it’s not just about data collection, it's about how we analyze it. Advanced AI techniques are becoming increasingly central to these dynamic adjustment calculations. These algorithms can sift through complex datasets, helping automate risk inference. While this can be helpful, there's always a need for human oversight to ensure the AI doesn't go rogue with its assessments.
Furthermore, we can integrate insights from behavioral economics into the mix. This might give us a better way to assess how clients’ behaviors influence risk, which allows for more tailored premium adjustments. Another application is that real-time data is useful in spotting fraudulent claims by comparing it to different sources and flagging deviations from normal patterns.
This push for real-time data also seems to have implications for the way organizations function. The shared, up-to-the-minute information available from this integration promotes better collaboration across teams like underwriters, data scientists, and financial analysts. This can potentially lead to faster decision making and a smoother workflow. Also, with regulations constantly changing, real-time data enables insurance companies to swiftly adapt underwriting practices to comply with new requirements, which mitigates some of the legal risks associated with non-compliance.
It’s all quite fascinating, but it does beg some questions: Are we too reliant on algorithms? Can we guarantee the integrity and fairness of AI-driven premium adjustments? These are all valid concerns as these methods become more common in underwriting. Nonetheless, the potential benefits of real-time market data integration for surety bonds appear to be immense, potentially leading to a more responsive, accurate, and adaptive risk assessment process in the years to come.
Automated Risk Assessment How AI is Transforming Surety Bond Underwriting in 2025 - Automated Verification System Cuts Bond Application Processing to 60 Seconds
A new automated verification system has drastically changed how bond applications are processed, slashing the time it takes from submission to assessment down to a mere 60 seconds. This system uses artificial intelligence to automate parts of the underwriting process, speeding things up without sacrificing the quality of the evaluation. The ability to process a high volume of applications quickly not only makes operations more efficient but has the potential to completely alter the way risk is assessed in the surety bond market. However, there are legitimate concerns about over-dependence on these automated systems since the careful application of human judgment remains crucial for effective underwriting. As the technology advances, it will be important to maintain a careful balance between automation and human oversight to ensure accurate and unbiased underwriting decisions.
It's fascinating how automated verification systems are streamlining the bond application process. These systems can process applications in a remarkably short timeframe, just 60 seconds. This is a significant leap forward from the traditional method, where reviewing applications could take days or even longer. The speed is achieved through a combination of optical character recognition (OCR) and natural language processing (NLP) which enables the system to digest various document types, extract key details, and minimize human error that’s common with manual reviews.
The system's capability extends beyond basic document parsing. It can dig into applicant backgrounds and financial history, identifying potential risks that might be overlooked in a quick scan. This deeper analysis is built into the system through advanced algorithms. The process also seems to be designed with integration in mind. It can plug into existing databases and automatically check for regulatory compliance, adding a layer of security and accuracy to the assessments.
Furthermore, the speed of the automated system makes it a valuable tool for managing inconsistencies and discrepancies in application materials. These systems can flag issues almost immediately, giving underwriters a faster response time to emerging concerns. This, in turn, supports more dynamic underwriting operations, shifting the focus from handling repetitive paperwork towards strategic decision-making. This could be a productivity boost for underwriters.
One key characteristic is the scalability of the automated verification system. It can apparently handle increases in the volume of applications without a slowdown, something that is often a challenge with conventional methods. Additionally, these systems can leverage historical data through predictive analytics, potentially illuminating risk factors that would normally take considerable effort to unearth.
However, the reliance on historical data raises some interesting questions. How well does the historical data truly represent future trends or unique situations? The effectiveness of the system relies on its ability to accurately predict risk based on past trends, but what happens when circumstances change? This highlights the ongoing need for human oversight and continuous refinement of the underlying algorithms to maintain accuracy.
This shift toward automated verification seems poised to fundamentally change how customers interact with underwriters. Applicants could expect much faster approvals and perhaps even more personalized service. These changes could also lead to opportunities to nurture more meaningful client relationships, a positive side effect that was not necessarily envisioned with older underwriting practices. While the automation is a key development, it's clear that careful monitoring and a human-in-the-loop approach are crucial for ensuring that this technology provides long-term benefit.
Automated Risk Assessment How AI is Transforming Surety Bond Underwriting in 2025 - Blockchain Integration Creates Tamper Proof Digital Bond Certificates
Blockchain's emergence offers a compelling path to improved surety bond operations through the creation of immutable, digital bond certificates. These certificates, stored on a distributed ledger, ensure a secure and transparent record of bond transactions that can be verified across multiple parties. This approach fundamentally alters how bond data is managed, moving away from potentially vulnerable centralized systems.
Integrating blockchain with AI promises further benefits. Real-time data analysis and automated risk assessments become feasible, potentially speeding up the decision-making processes in bond underwriting. This could lead to quicker and more efficient operations and a decrease in the reliance on traditional, often slow, intermediaries. However, this path isn't without its challenges. The question of how to manage and regulate the use of blockchain technology for surety bonds, especially regarding data privacy and the implications of automated decision-making, remain a critical area of concern. It will be vital as this technology progresses to find a good balance between the benefits of automation and the need for human oversight in managing bond-related risks. The surety industry faces a significant decision point in figuring out how these technological advances can be properly integrated into established practices and regulatory frameworks.
Blockchain's potential in surety bonding is fascinating, particularly in the realm of creating digital bond certificates. The technology's core strength, a decentralized and immutable ledger, essentially makes tampering with a bond certificate practically impossible. Imagine a digital record that's cryptographically linked to previous entries, making it incredibly difficult to alter without widespread agreement across the network. This offers a significant boost in security compared to traditional paper-based systems, which are vulnerable to counterfeiting or manipulation.
One of the intriguing aspects of this is how AI integrates with blockchain to automate the assessment of risk. By recording bond transactions and related data on the blockchain, we have a verifiable history of every interaction. This real-time data stream, coupled with AI, could potentially automate a lot of the tasks currently handled by underwriters. This includes assessing a contractor's performance history, determining their creditworthiness, and ultimately determining the risk associated with issuing a bond.
However, it's not just about securing data. Blockchain can also streamline operations and potentially reduce costs. With a decentralized and tamper-proof record, we could eliminate the need for intermediaries and simplify processes like transferring ownership. It would be interesting to see if this could cut down on the paperwork and delays typically associated with bond transactions.
Further, the use of smart contracts in conjunction with blockchain could automate specific conditions of bond agreements. For example, specific payments could be triggered automatically upon verification of project milestones. This automatic execution of agreed-upon terms reduces friction and creates greater clarity in the bond issuance process.
The ability to track bond transactions in real-time and grant selective access to specific parties is also a notable feature. This enhanced transparency could create a more efficient ecosystem for bond issuance. Think of it like a collaborative, auditable record that everyone involved can access when needed.
There are also some exciting implications for things like fractional ownership. The ability to break down bond certificates into smaller units could create more accessibility for smaller investors. This democratizes investment opportunities, allowing more people to participate in bond markets that were previously inaccessible.
Of course, there are still a few hurdles to consider. The question of ensuring data privacy and the overall adoption by different stakeholders within the industry will play a critical role in how effective blockchain becomes. But, from an engineering standpoint, the potential for this technology to change how we approach risk management, automate tasks, and enhance transparency in surety bonding seems promising, and it'll be interesting to see how these developments unfold over the next few years.
Automate legal research, eDiscovery, and precedent analysis - Let our AI Legal Assistant handle the complexity. (Get started for free)
More Posts from legalpdf.io: