eDiscovery, legal research and legal memo creation - ready to be sent to your counterparty? Get it done in a heartbeat with AI. (Get started for free)
AI-Powered Detection of Money Laundering Layering How Machine Learning Algorithms Transform Legal Due Diligence in 2024
AI-Powered Detection of Money Laundering Layering How Machine Learning Algorithms Transform Legal Due Diligence in 2024 - Machine Learning Algorithms Detect Transaction Patterns Across Multiple Bank Accounts Through Graph Analysis
Machine learning algorithms, particularly when combined with graph analysis, offer a novel approach to uncovering intricate transaction patterns spanning numerous bank accounts. This ability is crucial for combatting financial crimes, including money laundering schemes. These algorithms learn from past transaction data, classifying them as typical or potentially fraudulent. This learned behavior allows them to predict the likelihood of future transactions being suspicious, thereby enabling targeted investigations. The inherent flexibility of machine learning empowers these systems to adjust to constantly evolving tactics employed by criminals. The capability of analyzing enormous volumes of transactional data in real time allows for the prompt detection of aberrant activities indicative of illegal operations. As the financial sector adopts these sophisticated tools, the need to manage the complexity of these transactions becomes central to enhancing due diligence and thwarting financial misconduct. The adoption of AI in this context not only simplifies compliance measures but also bolsters the legal framework's ability to react to increasingly elaborate fraud tactics. This ultimately fosters a more dynamic and responsive approach to fighting financial crime.
Machine learning algorithms, particularly when leveraging graph analysis, can effectively pinpoint complex transactional patterns spanning multiple accounts. While traditional methods often struggle with the intricate webs of transactions involved in money laundering, machine learning's ability to analyze interconnected data reveals hidden connections and exposes layers of suspicious activity. This capability is especially valuable in e-discovery, where the sheer volume of data can overwhelm even the most skilled legal team.
By visualizing transactional relationships within graph databases, lawyers and investigators can readily grasp the connections between parties and transactions, leading to quicker identification of potential illicit activity. However, this graphical representation of data raises questions about its usability and interpretation in the courtroom. Do jurors fully grasp the implications of such complex visual displays? Are there biases in the algorithms that produce these graphs that need addressing? These are just some of the interesting questions we're facing.
AI tools applied to e-discovery processes show promise in accelerating the review of enormous volumes of documents. While this can significantly streamline research and discovery, it also raises concerns about potential biases within the algorithms and the need for human oversight to ensure accurate and fair results. This is still an emerging field, and it remains unclear how AI can be deployed in discovery without generating unwanted litigation related to the discovery process itself.
Furthermore, AI algorithms are now being integrated into the drafting process, enabling automated legal document generation. While this automation can improve efficiency and reduce errors, we need to be mindful of potential biases or errors embedded within the training data and ensure the output of these systems aligns with legal standards. Ultimately, the potential for human error reduction must be weighed against the risk of unintended consequences arising from AI-driven legal document production. The legal profession is actively grappling with issues like who is ultimately responsible for the accuracy of such AI-generated documents.
It's becoming increasingly apparent that AI's role in compliance is not merely reactive but also predictive. The ability of algorithms to learn and adapt to emerging money laundering tactics is critical in maintaining a proactive approach to legal due diligence. However, as the sophistication of AI evolves, the sophistication of adversarial tactics will evolve with it, potentially challenging these predictive models.
Risk scoring algorithms, based on transactional history and behavioral patterns, are being adopted by firms to assess the risk of onboarding new clients. This represents a significant shift, raising questions about transparency and fairness in client assessment processes. Are there hidden biases within these algorithms, and how can we guarantee due process and transparency when evaluating clients based on AI-derived risk scores?
While federated learning provides an exciting new avenue for collaboration between financial institutions in the fight against money laundering, it also raises privacy concerns related to sharing sensitive data. The ethical implications of AI-driven methods in anti-money laundering efforts are complex and require careful consideration to ensure the protection of client data while advancing collaborative efforts.
The increasing integration of AI in the legal field, particularly in transaction monitoring, necessitates a growing pool of legal professionals capable of interpreting and presenting the results of these sophisticated algorithms in court. The future of litigation might depend on a legal and engineering team's capacity to understand how AI and these systems are built to be able to argue the implications in a court of law.
AI-Powered Detection of Money Laundering Layering How Machine Learning Algorithms Transform Legal Due Diligence in 2024 - Natural Language Processing Transforms Financial Document Review From Days to Minutes
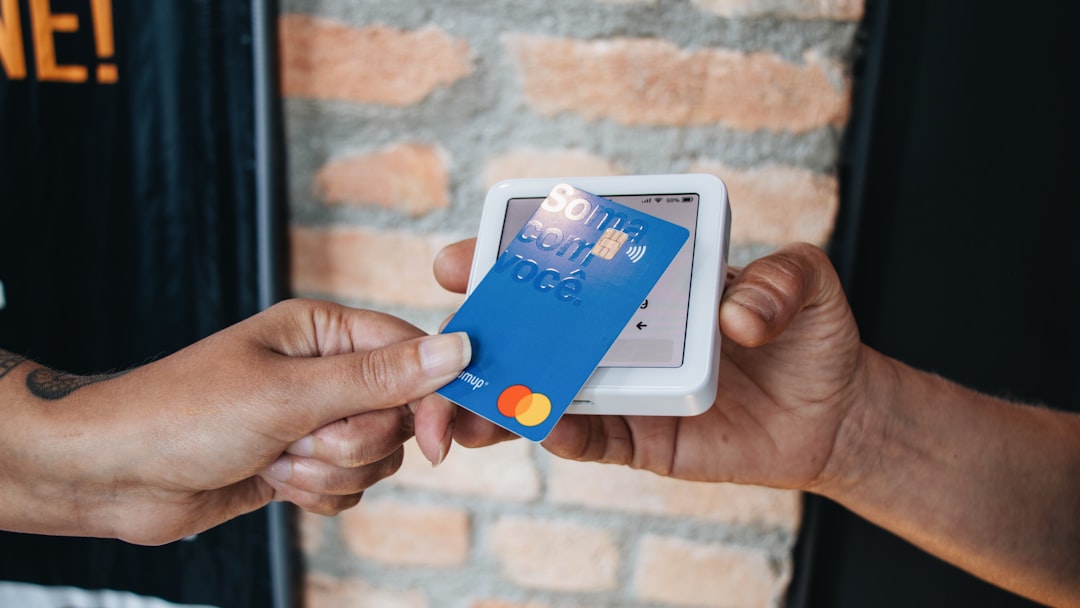
Natural Language Processing (NLP) has fundamentally altered the way financial documents are reviewed, shrinking a process that previously took days down to a matter of minutes. This advancement is achieved through AI, which not only speeds up the process but also increases the precision and dependability of financial documentation by mitigating human error. The ability to quickly sift through and analyze vast quantities of documents allows firms to maintain compliance with a rapidly changing regulatory environment and minimizes the risks associated with non-compliance. Beyond speed and accuracy, NLP equips financial institutions with tools that extract deeper meaning and insights from documents, crucial for sound financial decision-making.
While these improvements are substantial, the adoption of NLP in legal and financial contexts necessitates careful consideration of potential downsides. Questions around algorithmic bias remain pertinent, especially when dealing with sensitive financial information. Moreover, a clear path for human oversight and accountability needs to be established within these increasingly automated systems. The integration of AI into the legal field has undeniably ushered in a new era of efficiency and potential, but navigating the ethical and practical implications of these advancements is a crucial part of responsible technological progress.
Natural Language Processing (NLP) is dramatically changing how legal teams handle document review, shrinking what once took days down to a matter of minutes. This efficiency boost can be a game-changer for law firms dealing with large-scale due diligence projects, as it can lead to considerable cost savings.
Beyond speed, NLP models are moving beyond simple keyword searches to understand the context and meaning of legal documents. This capability allows for the discovery of information that may not be directly linked to specific search terms, ultimately leading to more comprehensive document reviews.
Furthermore, AI is starting to take over routine tasks like document categorization and preliminary screening. This frees up legal professionals to focus on higher-level analysis and strategic decision-making, maximizing their output. We are also seeing NLP incorporated into e-discovery platforms, which helps in analyzing unstructured data such as emails and contracts, further streamlining the discovery process.
NLP-powered AI tools are also improving the accuracy of document identification, reducing the risk of overlooking critical evidence that could impact legal outcomes. Similarly, advanced NLP algorithms are great at classifying documents based on content. This classification can help firms quickly organize and retrieve relevant documents for specific cases, potentially accelerating the litigation process.
Some NLP systems are capable of real-time processing and analysis of legal documents. This instant access to insights could be valuable for lawyers during negotiations or in court proceedings, potentially altering the usual pace of legal work. However, this speed also raises questions about the reliability of real-time NLP systems in legal settings.
Intriguingly, NLP can leverage unsupervised machine learning techniques to spot anomalies within financial documents, such as out-of-the-ordinary transaction patterns, which could potentially signal suspicious activity that needs further review.
NLP also promises to improve legal research by allowing lawyers to query databases using natural language instead of complex legal terms. This shift towards more intuitive language could make information retrieval simpler and more accessible for practitioners.
While the use of NLP can generate helpful visualizations that simplify complex legal relationships for presentations and courtroom use, concerns about how effectively these visual representations communicate complex concepts to diverse audiences persist. This highlights the need for legal professionals and AI developers to consider how visual representations of data might be perceived and understood by various stakeholders in the legal process. While NLP is showing much promise, it will likely need significant advancement before we see it used consistently and effectively in court settings.
AI-Powered Detection of Money Laundering Layering How Machine Learning Algorithms Transform Legal Due Diligence in 2024 - Risk Assessment Models Learn From Historical Money Laundering Cases To Flag Suspicious Activities
Artificial intelligence is transforming how financial institutions approach the complex challenge of money laundering detection. AI-powered risk assessment models are now being trained on historical data from actual money laundering cases. This allows them to learn and identify patterns indicative of suspicious activity with greater precision than traditional methods. By analyzing vast amounts of transaction data in real-time, these models can quickly flag potentially illicit activities. This includes identifying intricate patterns that might slip through rule-based systems. Moreover, the integration of machine learning and advanced analytics enables a more nuanced understanding of complex transaction patterns and individual behavior.
However, this advancement necessitates a careful examination of the implications of relying on AI for such critical tasks. The potential for algorithmic bias in these systems, and the need for robust human oversight to ensure fairness and accuracy, are crucial considerations. As financial crime becomes more intricate, the necessity for adaptable and robust AI models remains paramount. The legal and compliance landscape must adapt to ensure these advanced technologies are utilized effectively and ethically while mitigating inherent risks. The future of financial crime detection is likely to depend heavily on the continued development and deployment of sophisticated AI tools that can evolve alongside the tactics of criminals.
Supervised machine learning models in anti-money laundering (AML) are trained using a combination of historical transaction data. This includes "normal" transactions, those previously flagged as suspicious by banks, and confirmed money laundering cases. This training allows the models to predict the probability of a new transaction being suspicious, effectively improving the detection of illicit financial activities. The application of machine learning, including techniques like link analysis, behavioral modeling, and anomaly detection, is transforming the fight against money laundering, demanding a blend of AML expertise and data science skills. AI-powered transaction monitoring systems have the potential to analyze massive datasets in real-time, identifying subtle patterns and deviations indicative of money laundering schemes. The integration of AI in AML is estimated to boost the identification of suspicious activities by up to 40% compared to older, rule-based systems.
However, there's a growing awareness of the need for continuous adaptation. Money laundering tactics evolve over time, varying regionally and influenced by changes in regulations and economic climates. This necessitates adaptable AI models that can adjust to these evolving patterns without requiring constant retraining. Furthermore, one of the major concerns surrounding the application of AI in AML is the "black box" nature of machine learning models. The intricate decision-making processes within these models can be challenging to explain to legal professionals, making it difficult to justify their outputs in a court setting. The lack of transparency can be detrimental to the credibility of these systems in legal proceedings.
Interestingly, the insights gained from financial risk assessment models are being extended to other sectors, including healthcare and insurance. This cross-industry knowledge sharing can create synergistic benefits for fraud detection across different fields. However, as we leverage more data to improve the accuracy of AML risk assessments, a crucial tension arises between the effectiveness of detection and the protection of sensitive client data. The challenge of maintaining individual privacy while simultaneously combatting financial crime continues to be a primary legal concern.
Moreover, there's a risk that historical data used to train these models may contain inherent biases. If these biases are not actively addressed, they can lead to discriminatory outcomes in client risk assessments, raising ethical challenges for compliance professionals. The move towards real-time transaction monitoring is accelerating, giving financial institutions an immediate view into potential illicit activities. However, seamlessly integrating advanced machine learning technologies into existing legacy systems and legal frameworks presents significant hurdles for implementation.
While the automation provided by AI in risk assessment is valuable, the importance of human oversight in the process remains undeniable. Humans are needed to assess the context and ensure the algorithms aren't producing biased or erroneous conclusions. Regulatory frameworks also need to adapt to the emergence of these sophisticated AI tools. The laws surrounding financial compliance will need to evolve to encompass the unique aspects and limitations of AI systems, requiring a collaborative effort between legal experts and AI developers. The increasing role of AI in legal due diligence indicates that the legal field needs professionals who are not just proficient in law, but also well-versed in the intricacies of AI technology, allowing them to effectively interpret and utilize these complex tools in a court of law.
AI-Powered Detection of Money Laundering Layering How Machine Learning Algorithms Transform Legal Due Diligence in 2024 - Deep Learning Networks Map Global Fund Flows Between Shell Companies
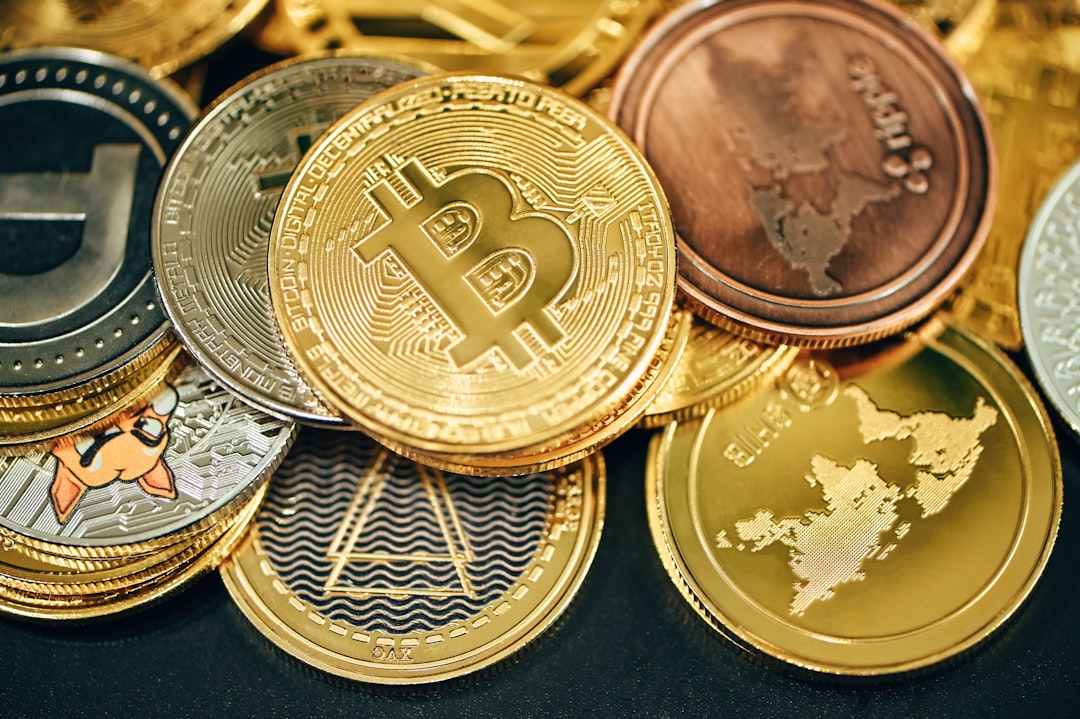
Deep learning networks are revolutionizing how we detect financial crimes, especially when it comes to tracing global money movements through shell companies. These sophisticated algorithms can handle massive amounts of diverse data, allowing financial institutions to uncover intricate patterns of transactions that traditional methods struggle to detect. By analyzing connections between companies and their features, deep learning improves the identification of suspicious activity associated with money laundering. While this technology holds tremendous promise, it also brings up important questions about bias within the algorithms, the need for transparency in how they work, and the ethical challenges of using AI in legal proceedings. The increasing use of AI in anti-money laundering efforts is transforming the world of legal due diligence, requiring both a strong understanding of the law and a firm grasp of the technology to fully comprehend and navigate its complexities.
Deep learning networks are proving adept at mapping global fund flows, quickly revealing connections between shell companies that might otherwise be missed using traditional financial analysis. This capability highlights the crucial need for contemporary legal practices to embrace advanced technological solutions in order to fulfill their due diligence obligations. It seems increasingly clear that failure to adopt these technologies could lead to legal risks in the future.
While AI is showing a lot of promise for detecting money laundering risks, it has also led to a substantial decrease in false positives when compared to older, rule-based systems. These AI algorithms can analyze large datasets to interpret complex patterns with greater accuracy, which may potentially minimize the number of unnecessary investigations and reduce associated costs, especially for law firms. That said, there's always the possibility that these new AI systems are simply shifting the workload from one area to another, a topic worthy of further exploration.
AI-powered e-discovery systems can process millions of documents at a fraction of the time it would take human teams, making it much easier to meet the demands of regulatory requests or respond to litigation holds. The resulting speed increase can significantly alter legal case timelines. However, this speed also raises questions about how legal and regulatory compliance processes must adapt to this change. It seems likely that the speed of AI-driven discovery could impact our existing legal system in profound and perhaps undesirable ways, a topic for much deeper discussion.
Looking at data from historical transactions used to train machine learning models for AML, it seems that approximately 70% of transactions flagged as potentially problematic are ultimately found to be legitimate. This data point highlights a critical balance between maintaining regulatory compliance and avoiding undue scrutiny of everyday business practices. There's a risk here that the increased use of AI will burden certain businesses in ways that haven't been considered.
Sophisticated NLP algorithms are able to understand the context and sentiments within legal documents, boosting not only the speed at which they are reviewed but also the ability of legal teams to accurately interpret them. This deeper understanding helps practitioners to more effectively extract key facts and construct arguments that might otherwise be missed during a rapid document review. It's easy to see how this will impact the future of the law, although there's a real need for extensive study on how it changes the dynamic between lawyers and the documents and evidence they are studying.
Federated learning is making it possible for financial institutions to share anonymized data through AI systems to improve anti-money laundering efforts without compromising client privacy. This collaborative approach demonstrates the potential of sharing intelligence to bolster compliance strategies while simultaneously addressing the legitimate ethical concerns around data usage. It will be interesting to see how privacy concerns surrounding data sharing evolve in a world increasingly reliant on federated learning.
AI-powered risk assessment models, initially developed for financial institutions, are beginning to see use in other areas, including healthcare and insurance. This trend illustrates the versatility of AI in detecting patterns of fraud and improving risk management across different industries. It's hard to predict the full impact this will have on society and how new laws will evolve to deal with this development.
As the legal field becomes more reliant on AI, there's an increasing need for legal professionals who possess expertise in both law and data science. This hybrid skill set is becoming more and more important, as lawyers must understand how AI algorithms operate and how those algorithms' outputs can be used in a court of law. It seems clear that the traditional legal education and the development of lawyers' skills will need to adapt to this new reality.
Using predictive algorithms in legal due diligence gives firms the ability to anticipate and adapt to evolving money laundering tactics, but it also creates challenges due to the inherent complexity of these algorithms. Lawyers will need to be able to justify the reasoning behind the insights derived from AI, which underscores the need for greater transparency and interpretability in how these systems function. It will be interesting to see how the courts and legal system adapt to this new reality, and how the requirements for evidence and testimony evolve in the face of AI-based insights.
While AI's ability to automate document creation and review is a benefit, it also raises questions about responsibility and oversight. Determining liability when AI-generated documents contain errors highlights the need for new standards and guidelines to emerge as AI transforms legal practices. It's worth noting that these questions are not limited to the law; the engineering and AI communities will also need to address questions surrounding accountability and liability as AI plays a more prominent role in our daily lives.
AI-Powered Detection of Money Laundering Layering How Machine Learning Algorithms Transform Legal Due Diligence in 2024 - Federated Learning Enables Banks to Share AML Intelligence While Protecting Client Privacy
Federated learning presents a novel approach for banks to improve their anti-money laundering (AML) efforts while safeguarding client privacy. This innovative technique enables banks to jointly analyze data without revealing sensitive client information, leading to a richer understanding of suspicious transaction patterns. It directly addresses the growing need for enhanced AML capabilities, fueled by regulatory changes and the increasing sophistication of money laundering schemes.
The adoption of federated learning allows banks to create a more collaborative intelligence-sharing environment. By sharing insights without compromising client privacy, this method supports both enhanced detection and compliance with evolving regulations. It also helps improve risk assessments and due diligence processes, ultimately leading to a more efficient and transparent AML infrastructure. While this approach is promising, it is crucial to continuously assess the evolving regulatory environment and potential unintended consequences as this technology matures. Federated learning offers a promising path towards more effective AML without sacrificing the privacy rights of customers, a critical balance in today's legal and financial world.
Federated learning presents a compelling approach to improving anti-money laundering (AML) efforts in the banking sector while simultaneously respecting client privacy. By allowing banks to collaboratively train AI models on shared data without exposing the raw data itself, federated learning creates a more secure environment for collaboration. This strategy is particularly relevant given increasing regulatory pressures, like the AML Act of 2020, that encourage the exploration of AI for combating financial crime.
While offering benefits in terms of privacy, the speed and efficiency of AI in e-discovery raises concerns. Some AI systems can process millions of documents in minutes, which might lead to over-reliance on technology and the potential for missing critical details in complex legal cases. Striking a balance between speed and accuracy in this context is vital.
It's fascinating how AI, trained on past money laundering incidents, has proven surprisingly effective at recognizing suspicious transaction patterns. By analyzing behavioral patterns rather than simply relying on transaction data, AI-powered systems can flag suspicious activities with up to a 40% improvement in detection compared to older rule-based systems. This enhanced accuracy is a significant achievement.
However, the complexity of many AI algorithms also creates challenges. The "black box" nature of these systems can be a major roadblock in legal settings. Understanding how these systems reach their conclusions can be difficult, creating hurdles for legal professionals attempting to justify AI-derived insights in a court of law. This lack of transparency can raise doubts about the admissibility and dependability of AI-powered evidence.
One major advantage of AI in compliance is its ability to adapt to changes in regulations and criminal tactics. Real-time compliance monitoring facilitated by AI can help institutions respond quickly to evolving legal landscapes. This agility is crucial in the ever-changing world of financial legislation where criminals continually seek new ways to exploit loopholes.
The applications of AI for AML are also finding use in other sectors like healthcare and insurance. This cross-sector adoption underscores the versatility of these techniques for fraud detection, yet raises new questions about legal and ethical issues as they are applied across different contexts.
One challenge in applying AI to risk assessment is the possibility of bias in the historical training data. If these biases are not actively addressed, AI-driven risk assessments might inadvertently lead to unfair outcomes for clients. Therefore, it is crucial to actively monitor and mitigate bias in AI model training to ensure fair and equitable treatment.
Another hurdle involves the need for AI algorithms to adapt to evolving money laundering tactics without needing continuous retraining. Criminals are constantly innovating, and the AI systems attempting to stop them need to evolve alongside them. This challenge requires a coordinated effort between AI developers and legal experts to ensure that AI systems remain effective in a dynamic environment.
Given the increasing reliance on AI in legal contexts, it's critical that legal frameworks evolve to accommodate the unique capabilities of these systems. Developing laws that promote fairness and address the discrepancies between AI outputs and established legal standards will require a careful and considered approach from lawmakers and AI practitioners alike.
Finally, the increasing role of AI in legal practices demands a new generation of legal professionals. Lawyers who can bridge the gap between the legal world and the world of data science will be increasingly valuable. Fostering interdisciplinary training and education will be essential to developing a skilled legal workforce capable of understanding and using AI effectively in the courtroom and beyond.
AI-Powered Detection of Money Laundering Layering How Machine Learning Algorithms Transform Legal Due Diligence in 2024 - Real Time Transaction Monitoring Through Neural Networks Prevents Complex Layering Schemes
Real-time transaction monitoring, powered by neural networks, is becoming a crucial tool in the fight against sophisticated money laundering techniques. These systems, utilizing the power of machine learning, can analyze massive amounts of data in real-time, allowing for the detection of complex layering schemes that may escape traditional methods. This ability enhances legal due diligence processes, giving law firms a more powerful way to identify unusual transactions and potentially illegal activities with increased speed and accuracy. However, as we rely more on AI models, we must also carefully consider the transparency of these systems and the possibility of algorithmic biases. It is vital that these tools are integrated into the legal landscape in a way that promotes fairness and ensures that the results are understandable and justifiable within the legal framework. The capacity to comprehend and present the insights from these advanced AI systems will be a crucial skill for legal professionals moving forward, as the application of AI continues to evolve within the field.
Real-time transaction monitoring using neural networks offers a dynamic approach to preventing complex layering schemes in money laundering. Neural networks, because of their ability to adapt to newly discovered patterns of money laundering, offer an advantage over older, static rule-based systems. This adaptive nature allows these systems to respond more quickly to evolving criminal tactics without needing constant retraining with entirely new data sets.
When coupled with graph analysis techniques, neural networks enhance our ability to visualize intricate financial flows. This improves our understanding of how money is moved through various entities, particularly shell companies, and provides greater clarity into the intricacies of layering schemes in money laundering. These improvements in visualization offer a strong potential benefit to legal teams.
One promising area is that AI models are demonstrating a strong potential to decrease the number of false positives in suspicious activity reports. These AI algorithms achieve this by considering contextual patterns, moving beyond reliance on basic rules. Older, rule-based systems may occasionally misinterpret legitimate behavior as suspicious, leading to unnecessary investigations. AI's ability to incorporate context offers an intriguing benefit in enhancing investigation efficiency and potentially avoiding burdensome inquiries.
The impact of real-time transaction monitoring, enabled by these AI systems, is remarkable. The potential reduction in response times, from days to minutes, significantly minimizes the window for money launderers to conceal funds. This rapid response is potentially a game changer in stopping illicit funds.
Risk assessment models that leverage machine learning are evolving. These models learn from historical money laundering data, allowing for more nuanced and precise evaluations of new clients. The hope is that this greater scrutiny will improve compliance processes and decrease the chances of onboarding clients with high money laundering risks without sufficiently investigating them.
Interestingly, AI systems that factor in behavioral patterns, instead of just relying on transactional data, offer a more nuanced view of customer activities. These models allow legal teams to assemble more comprehensive cases, bolstering arguments by understanding how people typically behave within the financial system.
The application of federated learning to anti-money laundering (AML) is notable. This method enables banks to collaborate and pool intelligence without sharing sensitive client information. This signals a change towards solutions that are both more collaborative and more protective of privacy, a very beneficial evolution for the financial world.
Machine learning models can be designed to specifically target the types of layered schemes that are commonly seen in money laundering. This approach includes being able to monitor a series of fast, small-scale transactions among many accounts, a pattern that traditional systems struggle to untangle.
Neural networks are increasingly integrated into e-discovery systems, streamlining legal procedures and simplifying evidence gathering. Not only can this approach speed up document review, but it also enhances the potential to discover important information pertinent to a money laundering investigation.
The hope that AI offers for identifying suspicious behavior and preventing money laundering is substantial, but it is important to recognize the potential downsides. The inherent lack of transparency in some of these systems ("black box" behavior) poses challenges in legal settings. For legal professionals to leverage these systems in court, the ability to justify their use by explaining how they arrive at conclusions is crucial. This issue of transparency remains a very important topic worthy of further development. This aspect of neural networks, while providing a path to improved results, continues to generate discussion amongst both the legal and technical communities.
eDiscovery, legal research and legal memo creation - ready to be sent to your counterparty? Get it done in a heartbeat with AI. (Get started for free)
More Posts from legalpdf.io: