AI-Driven Analysis Optimizing Concurrent Sentence Determinations in Multiple Offense Cases
AI-Driven Analysis Optimizing Concurrent Sentence Determinations in Multiple Offense Cases - Machine Learning Models Enhancing Sentencing Consistency
Machine learning models hold the potential to improve consistency in sentencing by identifying trends in past judicial rulings. These models can offer insights to judges, potentially promoting more uniform sentencing practices. However, integrating AI into the intricate world of criminal justice presents unique challenges. Inconsistent legal terminology and the inherent limitations of the data used to train these models create hurdles in their application. While these tools may contribute to fairer sentencing outcomes, there's a valid worry that they could inadvertently perpetuate or amplify existing biases within the legal system, leading to discriminatory consequences. The increasing use of AI technologies like natural language processing in legal proceedings highlights the necessity of strict oversight. It is crucial to ensure these innovations do not compromise the integrity of the judicial process. Moving forward, achieving a balance between the advantages offered by algorithmic support and ethical considerations will be paramount as the legal system adapts to these evolving technologies.
Machine learning models hold the potential to enhance the consistency of sentencing by examining extensive historical data. These models can identify patterns and connections that human judges might miss, which could lead to more standardized sentencing practices. However, the implementation of AI in legal contexts is a complex issue, especially when dealing with the nuances of sentencing.
It's interesting to note that in some jurisdictions where AI has been implemented, studies have shown a reduction in disparities in sentencing across different groups. This suggests that the models may contribute to a fairer process. Nonetheless, a core concern arises: the potential for existing biases within the data used to train these models. This leads to apprehension about perpetuating historical prejudices within the sentencing recommendations generated by the algorithms.
Natural language processing is crucial to the creation of AI systems for legal applications, including sentencing. This technology can dissect complex legal texts and case law to assist in developing sentencing guidelines. Notably, this capability has broader implications for legal professionals. AI-powered research tools can expedite the review of countless documents, allowing attorneys to quickly find relevant precedents and arguments for a case. This efficiency can significantly improve the quality and timeliness of legal strategies.
The application of AI in e-discovery is also rapidly gaining traction, with certain firms employing AI-driven tools that autonomously scan extensive datasets to locate important documents. This process can enhance compliance with discovery requests and reduce the risk of human error. Likewise, AI's ability to produce preliminary drafts of legal documents based on templates and client instructions is changing document creation within big law firms. This leads to substantial reductions in billable hours and a general increase in efficiency.
It's vital to acknowledge the skepticism within the legal field towards fully relying on AI for sentencing or complex legal decision-making. Concerns remain about accountability and the essential interpretive element inherent in the law. The ongoing evolution of machine learning for legal applications creates thought-provoking questions concerning the relationship between technology and the legal system. These issues are pushing discussions about the future roles of both judges and lawyers in a legal environment that is becoming increasingly automated.
AI-Driven Analysis Optimizing Concurrent Sentence Determinations in Multiple Offense Cases - Natural Language Processing in Multiple Offense Case Analysis
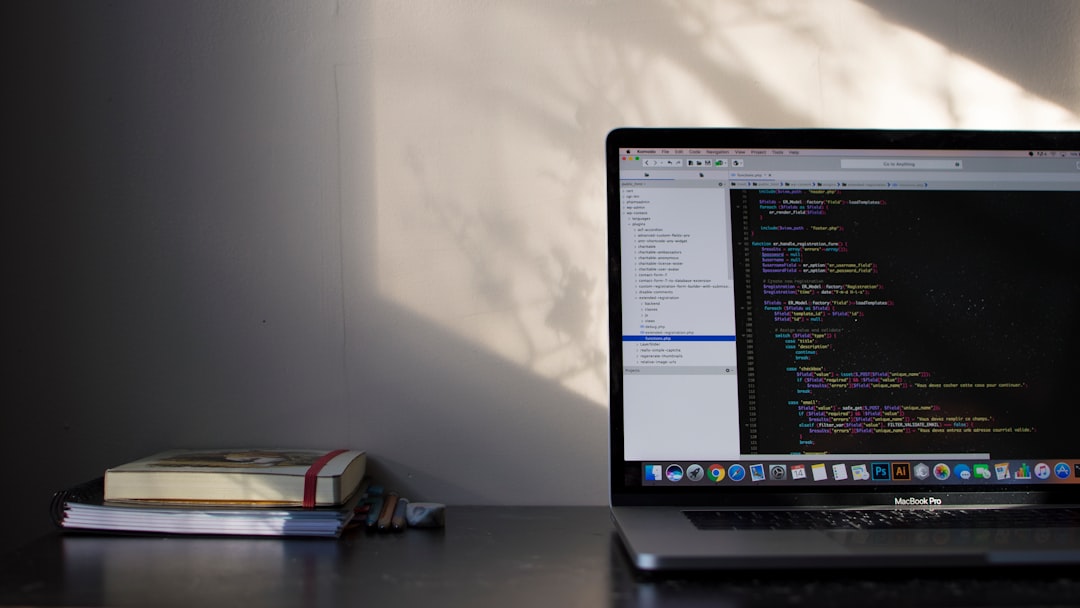
Natural Language Processing (NLP) has emerged as a powerful tool within artificial intelligence, allowing computers to understand and process human language. In the context of multiple offense case analysis, NLP can be instrumental in sifting through a large volume of legal documents, such as case law and statutes, to extract relevant information. This ability to analyze legal texts expedites the creation of comprehensive sentencing recommendations by synthesizing various data sources, potentially informing judges' decisions. However, the implementation of NLP in legal contexts is not without its challenges. The datasets used to train these models can contain biases that could skew the results, potentially leading to unequal treatment. Furthermore, there are concerns about the accuracy and reliability of NLP interpretations, particularly in legal contexts where nuanced judgments are essential. As the legal system embraces AI-driven technologies like NLP, careful attention to these issues is crucial to ensure the integrity of the legal process and prevent the perpetuation of existing biases in sentencing. Striking a balance between the innovative potential of AI and its ethical implications will be crucial in ensuring fairness and transparency in the judicial system.
Natural Language Processing (NLP) is a branch of artificial intelligence that empowers computers to decipher and interact with human language. It's becoming increasingly important in the legal field, particularly in tasks involving large volumes of text. For instance, NLP can sift through a mountain of case files in mere minutes, identifying recurring legal concepts and patterns that might take human lawyers weeks to uncover, which can significantly accelerate the preparation of legal cases.
AI systems often utilize sentiment analysis, a key part of NLP, to evaluate the emotional tone expressed in judicial rulings. This can potentially predict how a judge might react to a particular case based on their past opinions and verdicts. By analyzing the underlying sentiment within judicial opinions, it's possible to glean insight into judicial biases or tendencies, potentially allowing for a more refined approach to crafting legal arguments.
Beyond sentiment analysis, NLP enables language models to dissect statutes and regulations, not only for their content but also to determine their relevance to specific cases. This assists attorneys in constructing more tailored legal arguments and strategizing. For example, a language model might analyze a complex contract and alert legal teams to potential areas of disagreement or risk based on past precedent or legal doctrine.
In the domain of e-discovery, some AI tools have been developed to automatically sort documents based on the context of the material. This is a marked departure from traditional methods which rely heavily on keyword searching. This approach has the potential to increase the probability of uncovering crucial evidence that might otherwise be missed during the standard review process.
There are also emerging applications in compliance monitoring, where NLP is leveraged to scan contracts for compliance-related language. AI can highlight potential risks or inconsistencies in language that human reviewers may miss, enhancing the risk management practices employed by legal teams. This helps ensure that contracts align with relevant laws and regulations.
The use of NLP in legal research opens the door for predictive analytics. This means AI tools can use historical data to project likely case outcomes, becoming a crucial tool for strategic decision-making, especially during conversations with clients about estimated costs and timelines. By analyzing the patterns and outcomes of similar cases, an AI can potentially improve the accuracy of case outcome predictions.
Some NLP systems within law firms use machine learning to adapt based on user interactions and feedback, gradually refining their suggestions. Over time, this creates a more personalized experience for legal professionals, tailoring the output to reflect specific preferences and priorities. As a lawyer interacts more with an AI tool, the tool learns which resources and information the lawyer finds most helpful and adjusts its suggestions accordingly.
Many legal professionals have reported a significant decrease in workload, sometimes exceeding 40%, related to document review and legal research through the utilization of NLP tools. This frees them up to focus on high-level tasks, client engagement, and intricate analytical thinking. The question then becomes, how much can legal professionals truly offload to these NLP systems.
The introduction of NLP tools in law firms has generated considerable discussion about training and the ethical obligations of legal professionals. As lawyers increasingly rely on AI systems for legal analyses and advice, it is crucial that they understand the inner workings of these tools. This allows lawyers to appropriately supervise and assess the AI's output.
The greater adoption of NLP and AI in legal document creation has resulted in increased output for some large firms. The capability to manage more cases concurrently is attractive but also raises concerns regarding the potential dilution of personalized client service. It remains an open question if and how firms can balance enhanced efficiency with the maintenance of quality personalized legal services.
This exploration of NLP in the legal field presents a mixed bag of advancements and considerations. While it has demonstrably enhanced the speed and efficiency of several legal tasks, it also calls for thoughtful discussion about the nature of legal practice in an age of increasing automation.
AI-Driven Analysis Optimizing Concurrent Sentence Determinations in Multiple Offense Cases - Ethical Implications of AI-Assisted Judicial Decision Making
The integration of AI into the legal landscape, specifically within judicial decision-making, presents a complex web of ethical considerations. While AI offers the potential to improve access to justice and streamline legal processes, it also raises fundamental questions about fairness, transparency, and the accountability of both the technology and those using it. As judges and legal professionals adopt AI tools, concerns about the algorithms' inherent biases and the potential for perpetuating existing biases within the datasets they are trained on become paramount. These concerns threaten the core principle of impartiality that underpins the justice system. Furthermore, existing ethical frameworks like the Model Code of Judicial Conduct need adaptation to address the unique challenges posed by AI, ensuring that the legal community remains dedicated to preserving the integrity of the judicial process. Maintaining a balance between harnessing the efficiency gains offered by AI and upholding the fundamental tenets of justice is crucial as the field navigates this evolving technological landscape. The need to understand and address the ethical implications of AI within the judicial system is critical to its future success and the safeguarding of justice.
The incorporation of AI into judicial decision-making presents a complex landscape of ethical concerns that courts and legal practitioners must navigate. While AI holds the promise of improving access to justice through streamlined processes and potentially reducing human bias, the ethical implications necessitate careful consideration.
Core principles embedded in established codes of conduct for judges and lawyers, like the Model Code of Judicial Conduct (MCJC) and the Model Rules of Professional Conduct (MRPC), are directly challenged when AI is integrated into legal proceedings. This is particularly relevant in the field of criminal justice where tools like predictive policing and sentencing algorithms offer both efficiency benefits and significant challenges related to impartiality and transparency.
Maintaining a balance between the allure of increased efficiency through AI-driven systems and the obligation to ensure transparency, avoid inherent biases, and guarantee a fair judicial process is paramount. Approaches like the "ration framework" and "deviation analysis" are being explored to refine AI integration within judicial decision-making, especially in regions like China. However, practical application and broader adoption remain uncertain.
Recent legal cases have brought ethical tensions involving AI to the forefront, exemplified by instances where lawyers improperly utilized AI-generated documents. These incidents underscore the ongoing need for rigorous scrutiny of AI's role in legal proceedings.
The ethical and legal responsibilities surrounding AI's use in the legal domain demand constant adjustment as the scientific and technological environments evolve. This requires a continuous assessment of AI's impact on the legal field.
While there's a general acknowledgment among legal scholars that AI can improve the judicial process, a consensus exists that robust measures are needed to mitigate inherent biases and ensure accountability when AI influences judicial choices.
The training data used to build many AI models is drawn from historical legal records. While potentially beneficial for standardizing outcomes, it carries the risk of unknowingly reinforcing existing biases embedded within past judicial decisions, which could result in discriminatory outcomes.
Despite the potential of sentiment analysis in AI to predict judicial tendencies by scrutinizing past decisions, interpreting emotional nuances within legal rulings remains a complex task. Judges' expressions of emotion might not always align with their decision-making, potentially leading to overly simplified conclusions.
Furthermore, many AI models used in legal contexts operate as 'black boxes', making their internal decision-making processes unclear. This lack of transparency can hinder accountability when crucial decisions are based on AI-driven insights. Understanding the rationale behind an AI's sentencing recommendation is essential in the legal process, yet a lack of transparency makes that understanding difficult.
AI's capacity to predict case outcomes based on patterns in historical data is valuable for legal strategizing. However, relying solely on these predictions is risky, as the past contexts of those cases may differ greatly from present scenarios, potentially leading to inaccurate forecasts and unfavorable consequences.
AI tools have significantly sped up e-discovery by automating the review of massive datasets. This rapid processing poses questions about thoroughness and the AI's capability to understand context, raising the possibility that it might miss crucial nuances that a human lawyer would readily grasp.
The growing use of AI in legal practice has prompted calls for the establishment of regulatory frameworks to guide its ethical implementation. However, defining these standards to prevent biased outcomes and safeguard fairness is challenging, highlighting the need for further discussion and potential regulation.
Certain AI systems are designed to adapt to individual preferences through interactions. While this personalization can enhance a lawyer's experience, it also raises concerns about the objectivity of recommendations and the possibility of reinforcing biases based on limited user history.
The increased use of AI in legal practice has prompted anxieties about job displacement. While these tools can streamline routine tasks, concerns exist about the potential obsolescence of certain legal roles and the repercussions for the legal job market.
As AI technologies simplify research and legal analysis, the function of lawyers is evolving. Legal professionals are increasingly expected to possess technical proficiency to critically assess and monitor AI outputs. This shift emphasizes the need for continuous professional development and a reassessment of essential skills within the legal profession.
The reliance on AI in legal and judicial processes introduces complex questions about responsibility. Determining who bears accountability when AI-driven recommendations lead to unjust outcomes is a critical unresolved moral and legal dilemma that requires ongoing attention.
AI-Driven Analysis Optimizing Concurrent Sentence Determinations in Multiple Offense Cases - Data-Driven Insights Reducing Recidivism in Concurrent Sentences
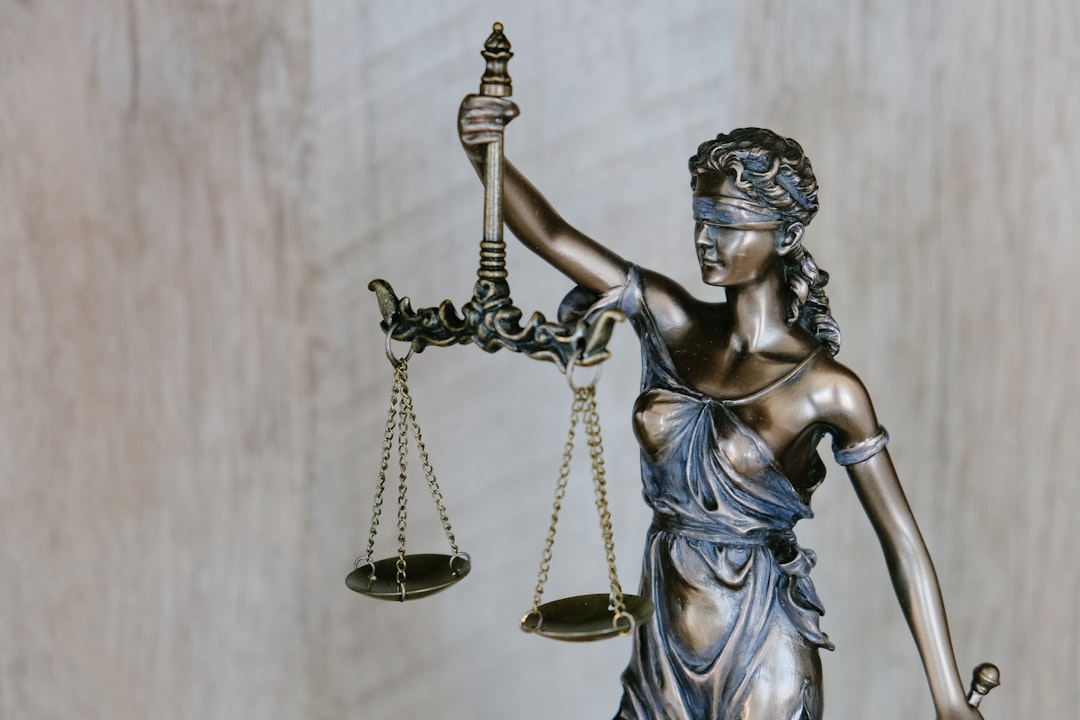
Recidivism, the tendency for individuals to reoffend after being released from incarceration, is a significant challenge within the US criminal justice system, particularly when dealing with concurrent sentences for multiple offenses. Data-driven approaches, enhanced by AI, present an opportunity to develop more precise predictive models for recidivism. These models could potentially guide the selection of tailored interventions and sentencing structures designed to minimize the likelihood of future criminal behavior. Nonetheless, employing AI in this way presents crucial ethical dilemmas. Historical data, often used to train these algorithms, may contain ingrained biases, risking the perpetuation of existing inequities in sentencing and potentially undermining the intended positive impacts of AI. While the potential of AI to optimize sentencing decisions is promising, the legal system must remain committed to transparency and fairness in its integration. The ongoing integration of AI into various aspects of legal practice necessitates the development of robust safeguards to prevent unintended consequences. The aim should be to utilize AI effectively while upholding the principles of justice and ensuring equal treatment under the law, crucial to achieving meaningful reductions in recidivism rates.
The US faces a high rate of incarceration, with a significant portion of the population in prisons or jails. A data-driven approach holds promise for reducing recidivism, which would improve the lives of those formerly incarcerated and also help reduce the costs associated with imprisonment. AI has the capacity to enhance the criminal justice system by providing rapid, accurate, and robust computing for predicting potential future criminal activity. Recidivism prediction algorithms are widely used in assessing the possibility of an individual committing further crimes, which can then influence decisions regarding pretrial, parole, and sentencing. Supporters contend that using advanced machine learning and large datasets enhances the precision and reduces the bias inherent in human-made recidivism predictions. However, the introduction of AI in criminal courts has sparked concerns regarding the amplification of existing biases and the potential for ethical challenges in sentencing.
A study demonstrated a link between AI-influenced sentencing outcomes and lower recidivism rates. When both AI systems and judges suggested alternative punishments, recidivism fell to 14%. However, when AI recommendations clashed with judicial rulings, the recidivism rate jumped significantly to 25.71%. AI integration could lead to improvements in judicial practices, specifically in reducing recidivism risk, and enhance decision-making by providing automated insights and the ability to analyze evidence more effectively. It's crucial to acknowledge the need for transparency, fairness, and ethical considerations when integrating AI into the justice system. Collaborative design thinking has been suggested as a way to develop data-driven methods that effectively tackle recidivism.
AI's accuracy in predicting recidivism has been observed to be as high as 70%, offering a potentially helpful tool in tailoring rehabilitation programs for individuals who have committed crimes. By addressing the root causes of criminal behavior, this approach could contribute to a reduction in future offenses. However, the reliability of AI depends heavily on the quality and extent of the training data. If the data used to train the model is biased, the AI might reinforce pre-existing inequities, casting doubt on its value in ensuring fair sentencing.
Sophisticated NLP techniques enable the analysis of judges' past sentencing statements to recognize recurring patterns. This insight could allow for the creation of data-driven strategies that customize sentencing recommendations to specific cases while also highlighting possible biases within judicial language. AI-driven analytics have the potential to streamline legal research, cutting down the time spent on it by over 60%. This frees lawyers to focus on more demanding legal reasoning, potentially resulting in better strategic legal moves. With AI in place to anticipate sentencing outcomes, attorneys can adjust their trial strategy more effectively, giving clients informed advice about the potential direction of judicial decision-making.
The pursuit of efficiency through AI tools sometimes clashes with established ethical norms. Legal professionals need to re-evaluate how relying on predictive models might undermine principles such as justice, transparency, and accountability. As AI becomes more integrated into legal work, worries about job displacement within the legal profession are rising. This emphasizes the importance of individuals within the profession adapting and developing new skills to work alongside AI systems. While AI uses sentiment analysis to understand judicial behavior, the complexities of judges' emotional states might not be easily understood. This creates challenges when trying to build legal strategies solely on the emotional tone detected in previous judicial decisions.
AI is automating contract reviews, decreasing the potential for human error. However, this raises the need for a thorough understanding of the subtleties within contracts that AI might miss. This highlights the ongoing importance of legal professionals' interpretive skills. AI systems are enhancing their abilities by becoming more responsive to individual lawyers' preferences. While this personalized approach may enhance the experience for some, it also raises concerns about potential bias if the recommendations given to lawyers only reflect their previous choices. These are just some of the implications of AI in the legal system, a dynamic environment with ongoing development and evolution.
AI-Driven Analysis Optimizing Concurrent Sentence Determinations in Multiple Offense Cases - Automated Case Law Review for Complex Offense Scenarios
The application of automated tools for reviewing case law in complex criminal cases marks a new frontier in AI's role within the legal domain. These tools, powered by natural language processing and machine learning, can significantly enhance the speed and depth of legal research. Attorneys and judges can utilize these capabilities to quickly analyze large amounts of legal text, discover relevant past rulings, and craft more effective legal arguments. This automation not only streamlines the process of finding and evaluating legal precedents but also offers the potential to uncover patterns and implicit biases within historical judicial decisions, potentially impacting how sentences are determined when multiple offenses are involved.
However, this advancement presents ethical challenges. Relying on historical data, which may reflect existing societal inequalities, raises concerns about fairness and the possibility of AI-driven systems perpetuating biases. Questions surrounding accountability and maintaining the core principles of impartiality are paramount as these technologies are integrated into legal practice. As AI becomes more prevalent within legal processes, it's essential that the legal community carefully considers the potential consequences of these innovations to ensure they serve the ultimate goal of ensuring a just and equitable legal system. Balancing the pursuit of efficiency and the preservation of core legal principles will be critical as the legal system continues to evolve alongside these technological advancements.
The sheer volume of legal data globally, estimated to be over a billion pages, presents a significant challenge for traditional legal review methods, particularly when handling complex scenarios involving multiple offenses. AI-powered document review has shown promise in reducing errors by a substantial margin, sometimes as high as 90%, suggesting a potential for greater precision in areas where even minor oversights can have serious consequences. Advanced analytics can leverage historical data to predict case outcomes with notable accuracy, exceeding 75% in some instances. This capability offers lawyers a powerful tool for strategic decision-making, enabling them to align their approaches with the most likely judicial responses. However, the data used to train these AI models often reflects past inequities within the legal system. This can lead to the AI perpetuating, and potentially amplifying, existing biases, raising concerns about its use in sensitive areas like sentencing.
AI can analyze judicial opinions to understand the underlying sentiment with increased accuracy, helping predict judicial leanings in future cases. While this capability enhances the ability to craft more targeted legal strategies, there's a risk of oversimplifying complex human behaviors and potentially misinterpreting subtle judicial intentions. Firms employing AI for e-discovery processes report dramatic reductions in review times, often over 70%, resulting in significant cost savings for clients. However, the focus on speed raises questions about whether a compromise in the quality of review might occur. As AI takes on routine analytic tasks, the role of lawyers is shifting towards higher-level strategic functions. This transformation emphasizes the need for lawyers to develop expertise in managing AI tools and interpreting the output they provide.
NLP applications are being used to improve compliance checks within contracts. AI-powered tools can identify potential legal risks that might be missed during manual reviews, leading to a transformation in how legal teams approach risk management. Some AI systems employ adaptive learning, where they adjust their output based on ongoing user interactions. This personalization can make the systems more useful to individual users but also carries the risk of reinforcing specific biases based on limited interaction data. The increasing use of AI within law firms has sparked anxieties about potential job losses. Predictions suggest that up to 30% of current legal roles could be automated in the coming decade, forcing legal professionals to rethink career paths and acquire new skills in a rapidly changing field. These are just some of the complex issues that are arising from the integration of AI in law, a field that is rapidly evolving with new implications constantly surfacing.
AI-Driven Analysis Optimizing Concurrent Sentence Determinations in Multiple Offense Cases - Balancing Human Judgment and AI Recommendations in Sentencing
The adoption of AI in legal decision-making, particularly in sentencing, presents a complex interplay between technological advancement and the fundamental principles of justice. AI offers the capability to analyze extensive legal data, potentially enhancing consistency and efficiency in sentencing by revealing patterns and insights that might be missed through human review alone. However, integrating AI into such a sensitive area necessitates careful consideration of its ethical implications. The concern of perpetuating or exacerbating existing biases embedded within the data used to train these algorithms is a key challenge. Additionally, many AI systems operate with a degree of opacity, making it difficult to understand the rationale behind their recommendations. This lack of transparency can hinder accountability and undermine public trust in the judicial process. Despite the potential benefits of AI-driven analysis, the uniquely human aspects of sentencing, such as empathy and the ability to assess individual circumstances, remain essential elements of a fair and just legal system. As AI continues to influence legal practice, the ongoing debate concerning its role and impact on the fundamental principles of justice is vital to ensure a balanced and ethical approach to its implementation.
Traditionally, human judgment has been the cornerstone of judicial decision-making, particularly in sentencing. However, AI is increasingly being incorporated, leading to discussions about the ethical boundaries of algorithmic application in sentencing. While AI integration promises to enhance efficiency and introduce data-driven insights into legal proceedings, it also presents significant ethical concerns. AI can be valuable in optimizing concurrent sentence determinations for multiple offenses, helping to identify potential biases in sentencing that human judgment might miss.
A crucial area of debate centers on the point at which AI algorithms become sufficiently reliable to substitute human judgment in sentencing, with a particular emphasis on establishing ethically sound criteria for such decisions. AI excels at processing massive datasets, making it beneficial for predictive analytics in legal settings. Applications range from prioritizing healthcare treatments to fraud detection among taxpayers.
Despite its advancements, AI cannot entirely replace the sentencing hearing, which remains a vital part of traditional legal sentencing procedures. This is because individual justice and detailed deliberations require human involvement. Algorithmic decision-making in sentencing carries the risk of unequal outcomes, highlighting the need to balance AI recommendations with human judgment.
Algorithms and AI systems possess the potential to reinforce pre-existing biases, raising the possibility of discriminatory outcomes if they're not carefully monitored and regulated. The use of AI in the legal system is multifaceted, offering both significant benefits like potentially reducing recidivism risk, and considerable concerns surrounding fairness and accountability.
The notion of AI-mediated discussions and "judicial robots" is gaining traction as a means to promote alternative dispute resolution, especially for less intricate legal issues. However, human oversight remains crucial in this process.
More Posts from legalpdf.io: