eDiscovery, legal research and legal memo creation - ready to be sent to your counterparty? Get it done in a heartbeat with AI. (Get started for free)
Understanding AI Contract Analysis in Workers' Compensation Law A 2024 Technical Perspective on Claim Documentation Reviews
Understanding AI Contract Analysis in Workers' Compensation Law A 2024 Technical Perspective on Claim Documentation Reviews - Natural Language Processing Enables Automated Review of Medical Reports and Legal Documentation
The application of Natural Language Processing (NLP) is gaining traction in automating the review of both medical and legal documents. This is especially pertinent in workers' compensation, where the volume and complexity of documentation can be overwhelming. NLP's capability to extract data from unstructured sources, such as clinical notes and operative reports, allows for a more streamlined approach to case management. Essentially, it offers a path to automation for tasks previously done manually, leading to quicker processing and potentially more reliable insights.
While traditional methods of systematic review were often laborious and time-consuming, NLP provides a more scalable solution for managing the increasing amount of healthcare information. This is crucial in a field where information overload can hinder efficient decision-making. As AI technologies continue to refine their ability to interpret and analyze complex text, the intersection of NLP and legal fields like workers' compensation is likely to see even greater advancements, especially in the pursuit of improved accuracy and efficiency. However, it's important to keep in mind that while this approach shows promise, it also introduces concerns regarding data security and potential biases within algorithms, which warrant continued scrutiny.
Natural Language Processing (NLP) presents a compelling approach to automatically sift through the deluge of medical reports and legal documents that often accompany workers' compensation cases. It's remarkable how quickly NLP can analyze unstructured text, potentially handling thousands of documents in seconds—a task that might take human reviewers weeks to accomplish.
The latest NLP algorithms are surprisingly adept at spotting subtle discrepancies within text, including inconsistencies in medical terms or legal definitions, which might escape the attention of a human reviewer. While promising, there's a need for continued scrutiny and evaluation of the accuracy and robustness of such detection methods.
Studies have shown that NLP-powered systems can substantially reduce human error when reviewing complex documents, sometimes achieving error reduction rates exceeding 30%. This precision can be a game-changer in enhancing the dependability of claim analysis. It's worth noting that this has to be compared to the error rates of humans on such complex tasks and how much that error rate matters for different use cases.
By grasping the context of language, NLP models can effectively categorize and prioritize claims based on urgency and relevance, thereby streamlining the review workflow for workers' compensation cases. While the potential is intriguing, more empirical evidence is needed to truly understand the effectiveness and reliability of such prioritization across diverse claim scenarios.
NLP systems can employ semantic parsing techniques to bridge the communication gap between legal and medical jargon and plain language, fostering improved communication between claimants and insurance providers. The accuracy of these translations needs to be carefully assessed, as misinterpretations could lead to significant misunderstandings and complications.
One of the exciting aspects of NLP is its capability to learn and adapt. Machine learning techniques allow NLP models to refine their accuracy and efficiency over time as they process more documents. However, it's crucial to understand how these models learn and what kind of biases could be encoded in their learning process.
While some studies suggest that NLP applications in healthcare might improve patient outcomes, it's crucial to establish a direct causal link and consider other factors influencing patient care outcomes. It would be valuable to analyze real-world data and explore specific clinical use cases where NLP demonstrably improves patient outcomes.
Legal professionals are finding NLP to be a helpful tool in their practice. NLP can rapidly scan vast libraries of case law, legal updates, and regulations, effectively assisting in due diligence and staying current on relevant legal developments. However, the legal field is complex and requires careful consideration when relying solely on automated systems for critical decisions, so caution and human oversight are warranted.
The prospect of reduced operational costs through NLP-driven document review is appealing, as it necessitates fewer personnel to manage the heavy volume of paperwork associated with medical and legal claims. Nonetheless, it's important to account for the implementation costs of these systems and ensure they provide a net benefit to the organization.
The integration of NLP into existing workflows can demonstrably enhance overall productivity, enabling professionals to concentrate on more complex, strategic decision-making, instead of labor-intensive manual review of documents. However, ensuring a smooth and effective integration necessitates meticulous planning and careful consideration of the potential changes to existing practices and human roles.
Understanding AI Contract Analysis in Workers' Compensation Law A 2024 Technical Perspective on Claim Documentation Reviews - Machine Learning Models Analyze Historic Claims Data to Predict Settlement Values
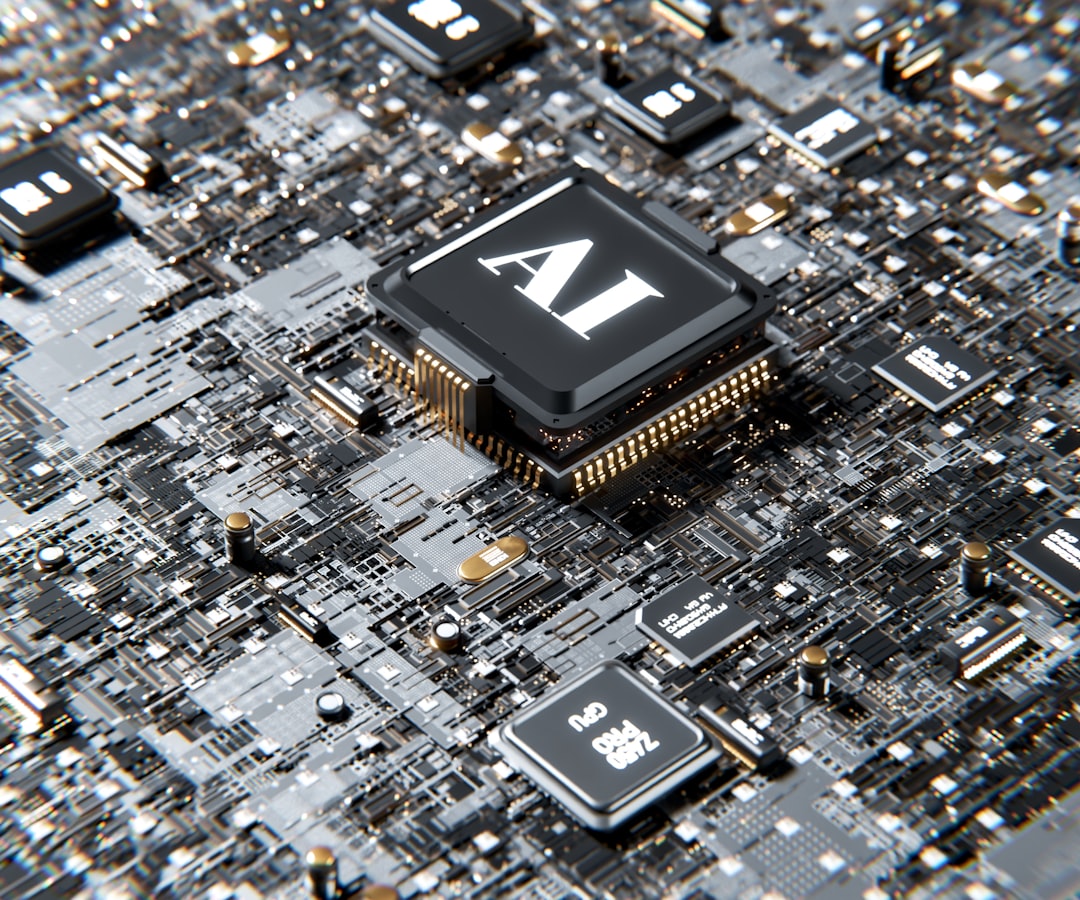
Machine learning models are now being used to examine historical workers' compensation claims data to forecast settlement values. These models, by using techniques like deep learning and supervised learning, can analyze large volumes of claim information to identify trends and predict future outcomes. This predictive capability allows for more accurate settlement estimations and helps in managing risks related to claim payouts. Furthermore, these models can handle data from different sources, making them more broadly useful in managing and optimizing claims processes overall. Despite the potential benefits, it's vital to critically examine how these models are built and used to ensure they don't introduce bias or inadvertently compromise data integrity. There's a constant need to evaluate the reliability of these predictive models and assess their long-term impact on claims management.
Machine learning models are increasingly being used to analyze historical workers' compensation claims data to predict settlement values. These models can potentially achieve surprisingly high accuracy rates in estimating settlement outcomes, surpassing traditional methods that rely heavily on human intuition and experience. It's interesting to see how they're able to pick up on subtle patterns within the data, like the duration of medical treatment, specific types of injuries, and even a claimant's age, which can all contribute to a more accurate prediction.
However, the sheer volume of data used to train these models can be mind-boggling – potentially millions of claim records. While this provides a robust foundation for predictions, it also brings up questions about data quality, security, and management. Interestingly, some models are also able to classify claims into different types with a remarkable level of precision, which allows for more tailored approaches to settlements based on historical patterns.
Furthermore, these models have the ability to analyze claims in real-time, which is quite different from traditional methods where reviews can take time. This rapid analysis can lead to quicker insights and more proactive case management, a big benefit in environments where legal proceedings move quickly. They're also quite good at flagging outlier claims, which could be indicators of unusual case circumstances or even potentially fraudulent activity.
But there are some things to keep in mind. Models that learn from past data can unintentionally pick up on biases that exist within that data, raising concerns about potential fairness and ethical implications in sensitive legal matters like workers' compensation. Another interesting facet is how these models can capture complex relationships between different factors that traditional approaches may overlook. For example, the relationship between the severity of an injury and the claimant's history of prior claims could have a substantial influence on settlement predictions.
Some researchers are using these models to study settlement trends over time. It's fascinating how this historical analysis could allow legal teams to adapt their strategies to evolving patterns. The introduction of machine learning in the claims process significantly impacts workflows, shifting from decisions based primarily on experience to those driven by data. This change forces us to reexamine how human roles are involved in the legal process. We need to carefully consider how human expertise can best be used alongside machine-learning insights for better outcomes.
Understanding AI Contract Analysis in Workers' Compensation Law A 2024 Technical Perspective on Claim Documentation Reviews - Pattern Recognition Technology Maps Treatment Coverage Gaps Across Geographic Regions
AI-powered pattern recognition is increasingly used to map out disparities in healthcare coverage across different geographical areas. By combining geographic information systems (GIS) with artificial intelligence (AI) techniques, we gain a clearer picture of treatment availability and outcomes in various regions. Essentially, this approach allows researchers to create visual maps that highlight areas where access to certain medical services is limited. This is particularly valuable in pinpointing healthcare disparities that may disproportionately affect specific population groups or areas with limited resources.
While this technology has immense potential for improving healthcare access, it's not without its challenges. For example, integrating the unique characteristics of a specific geographic location into the models can be tricky, raising questions about the accuracy and applicability of the results. Therefore, it's essential that researchers critically evaluate the data used and the methods employed in the analyses. Moreover, as with any AI system, there's a risk that existing biases within the data may be perpetuated or amplified by the algorithms. This means it's important to remain mindful of the potential for biases to affect the resulting insights and to design and implement solutions that address these issues, promoting equitable access to healthcare for all.
In the realm of workers' compensation, the use of pattern recognition technology, particularly methods like self-organizing maps, is shedding light on stark geographical disparities in the availability of treatment. We're seeing that access to necessary medical care following workplace injuries varies wildly across different regions. Rural areas, for instance, seem to be hit harder by these treatment coverage gaps compared to more urban locations. It prompts us to question whether everyone is getting a fair shot at quality care, regardless of where they live or work.
These technologies can leverage sophisticated algorithms to comb through mountains of claims data, digging out hidden patterns in treatment coverage gaps that might slip through traditional methods. It's exciting to see how this enhanced capability gives healthcare providers and insurers a powerful tool to identify areas that need improvement or more targeted action.
Interestingly, research hints at a strong connection between local regulatory environments and these treatment disparities. Places with more stringent medical protocols might be experiencing a wider range of access issues. This suggests a complex relationship between the rules and regulations around healthcare delivery and the actual availability of necessary services.
The insights provided by pattern recognition extend further to identifying trends in claim denials. For example, we can see if certain types of injuries or medical conditions lead to unusually high denial rates. These patterns could offer crucial clues for changes to insurance policies or adjustments in training protocols that would help to reduce inappropriate claim denials.
Furthermore, examining claim data over time reveals that treatment access issues can fluctuate throughout the year or even in response to new laws. It's quite fascinating to see how these temporal patterns could potentially forecast the effects of upcoming policy shifts on healthcare availability.
One very practical application of this technology is in resource allocation. By anticipating where treatment gaps might appear, health agencies can strategize and deploy resources more effectively to underserved areas. This proactive approach is especially useful in sectors with higher injury rates where prevention is paramount.
It's also interesting that pattern recognition can seamlessly integrate things like income levels and education data, the so-called "social determinants of health," into the analysis of treatment gaps. This helps to get a deeper understanding of the barriers that some groups of people face when trying to get healthcare.
Advanced mapping techniques offer a visual way to represent where treatment gaps are most pronounced. This visualization capability empowers decision-makers in government and insurance to target resources and policy changes to the areas that need it most.
One of the potential benefits of these technologies is how they can bring stakeholders together. Insurers, healthcare providers, and even government agencies can start collaborating more effectively when they have a better understanding of where the gaps exist.
Ultimately, by effectively identifying and addressing gaps in treatment coverage, we can see long-term improvements in public health, potentially reducing the frequency and severity of workplace injuries, ultimately leading to lower overall claim costs and improvements to the wellbeing of employees. It highlights that these technologies have the potential to contribute meaningfully to both individual and societal well-being.
Understanding AI Contract Analysis in Workers' Compensation Law A 2024 Technical Perspective on Claim Documentation Reviews - Automated Risk Assessment Protocols Flag High Risk Claims for Early Intervention
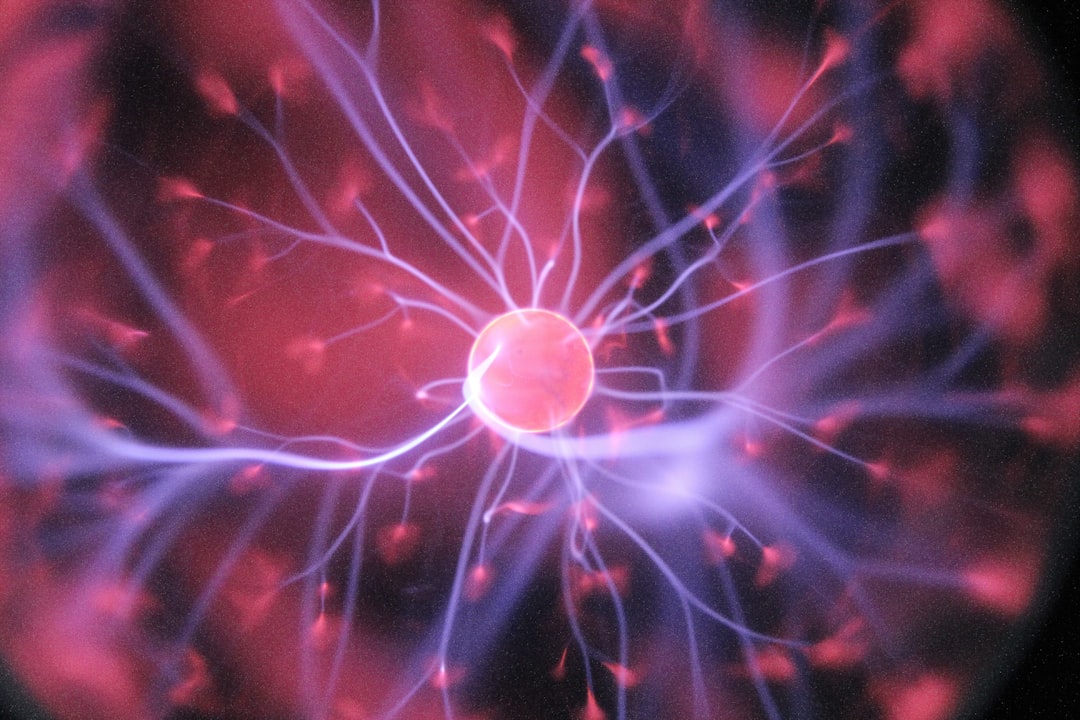
Automated systems are increasingly being used to evaluate workers' compensation claims and flag those with high risk factors for early attention. These protocols rely on artificial intelligence to analyze various data points within a claim, aiming to assist in informed decisions around claim approval and financial payouts. Organizations are adopting risk management frameworks like the one offered by NIST to help guide the integration and oversight of AI within their operations, recognizing the need to balance automated decision-making with human input and judgment. The evolution of these protocols is likely to streamline claims processing, though concerns about algorithmic bias and data privacy necessitate careful consideration and ongoing monitoring. It's hoped that with proper development and deployment, these AI-powered risk assessment tools will ultimately improve the efficiency and accuracy of decision-making within the complex world of workers' compensation, ultimately leading to better outcomes.
Automated systems are increasingly being used to analyze workers' compensation claims and identify those with a higher risk of complications or costs. These systems, using various data points, can potentially flag a large portion of high-risk claims, allowing for early interventions. This approach could be quite impactful in mitigating potential issues like fraud or unnecessary costs.
These systems are not static. They are designed to adapt as they process new data, refining their ability to identify risks over time. This means they can potentially respond to changes in claim patterns or even shifts in workplace injury trends. It's intriguing to consider how quickly they could react to new information.
Furthermore, the predictive capabilities of these systems are quite impressive. They're not just identifying risk factors but trying to forecast future claim behaviors, such as the likelihood of a claim escalating to litigation. This ability could potentially influence legal strategies and hopefully improve outcomes in terms of settlements or disputes.
These risk assessment protocols draw data from a wide range of sources, including medical histories, demographic information of claimants, and even geographical factors. The breadth of information considered can potentially provide a more comprehensive risk assessment than a human evaluation alone. However, whether this comprehensive approach is truly effective or not remains to be fully understood.
One potential application of this technology is in the area of fraud detection. By analyzing past claim patterns, these systems can potentially identify characteristics typical of fraudulent activity. While this is an area of significant potential, we still need to consider the reliability and accuracy of these fraud detection techniques in practical settings.
Some research suggests that early identification of risk can lead to a reduction in the overall duration of claims. This is certainly plausible, considering that addressing issues early on could streamline the claims process. While the idea is attractive, we should be critical about generalizing these conclusions as different claims and situations could lead to variations.
These systems are often proposed as a way to reduce operational costs in managing claims. This could be achieved by reducing the manual effort needed for evaluating claims. While potentially beneficial, careful planning and consideration are needed to avoid unintended consequences or a shift away from important aspects of claims management.
By leveraging data to examine trends across geographical regions, these automated systems can highlight potential disparities in claim frequency or type. This information is potentially quite useful for tailoring interventions or even considering policy changes to address specific risk factors within different areas.
There's evidence that early interventions facilitated by these systems may lead to more favorable settlements or outcomes. It's interesting to consider the mechanisms by which this occurs and how much of this is driven by the ability to prepare a better defense as a result of the early intervention.
One of the benefits often cited for these automated risk assessment systems is their potential to minimize human bias in claim evaluation. If implemented carefully, they could improve fairness and consistency in handling claims. It's essential to remember that these systems are trained by people and therefore could potentially inherit existing biases from the training data. Ongoing scrutiny and review of system performance are crucial to address this.
It's a complex field with the potential for significant impact on the workers' compensation landscape. While promising, we must continue to explore the implications and limitations of these systems. Research on the efficacy and ethical considerations remains crucial as we further integrate AI in legal and insurance domains.
Understanding AI Contract Analysis in Workers' Compensation Law A 2024 Technical Perspective on Claim Documentation Reviews - Real Time Analytics Drive Faster Communication Between Stakeholders and Medical Providers
Real-time analytics are becoming increasingly important for improving communication and collaboration between those involved in healthcare and medical professionals. The use of AI-powered systems enables near-instantaneous data sharing, which can lead to quicker decisions related to patient care and managing claims. This faster access to data can help medical providers better coordinate patient treatment plans and identify problems earlier, which may improve patient outcomes. Furthermore, having the capability to look at medical data as it's created allows healthcare organizations to react much quicker to issues that arise with claims, which can be helpful for both patients and the medical providers who are treating them. This continuous flow of information is vital for understanding the complex landscape of workers' compensation laws and for creating better claim documentation review processes. However, it's important to note that this approach also raises questions about data privacy and potential biases in algorithms, which require careful consideration.
The use of real-time analytics is rapidly changing how information flows between patients, medical professionals, and other involved parties. It's remarkable how these tools can condense information from a variety of sources, creating a comprehensive picture of a patient's situation. This integrated view is proving beneficial in allowing for quicker, more collaborative decision-making, particularly during crucial moments in a patient's treatment journey.
The potential to predict trends, such as a patient's likelihood of complying with their treatment plan, is a major advantage of real-time analytics. By spotting warning signs early, healthcare professionals can step in proactively, potentially altering treatment plans or providing additional support to prevent complications. This shift towards prediction, rather than just reaction, appears to be leading to better overall outcomes.
The impact of real-time analytics also extends to efficiency gains. For example, researchers have shown a reduction in needless repetitions of tests or procedures, which can translate into both cost savings for healthcare providers and a better experience for patients who avoid unnecessary interventions. It's important to consider whether these gains are large enough to justify the cost of implementation and if they are having the desired impacts.
Another interesting aspect is the improved patient follow-up. Using real-time data, healthcare providers seem to be having better success in keeping patients engaged with their care plans, which contributes to the overall efficiency and continuity of treatment. This increased engagement could potentially reduce the total cost of care over time.
Real-time analytics also offers the potential to make healthcare more equitable. By digging into the data, researchers can see disparities in treatment access and tailor interventions to help those groups that are facing hurdles. This capacity is vital in ensuring that everyone, regardless of socioeconomic background, receives the care they need.
While the application of real-time analytics seems to have enhanced the connection between patients and their doctors, it's important to understand if there are potential unintended consequences to the increased data collection and use. There's a growing sense that patient satisfaction is increasing, as they receive faster and more transparent updates about their care. But it's crucial to verify if this feeling of trust is sustainable and if patient privacy concerns are being addressed properly.
The positive effects are not limited to clinical interactions. Real-time analytics can improve administrative tasks, like claims processing, resulting in faster approvals and a generally more streamlined process for all participants. It's a reminder that this type of technology can be beneficial across a variety of operational areas.
The speed at which new healthcare challenges arise—whether a new disease or the rapid spread of an infectious outbreak— necessitates more flexible and adaptable healthcare systems. Real-time analytics is seen as an engine for this change, allowing healthcare providers to respond more swiftly to these emergencies. However, it's important to keep in mind that this rapid adaptability also presents new hurdles to address regarding issues like data integrity and ethical considerations.
In conclusion, real-time analytics is reshaping the landscape of healthcare communication and management. The potential for increased efficiency, enhanced communication, and improved patient outcomes is undeniable. But, it's vital to maintain a healthy dose of skepticism about its claims, keeping a critical eye on ethical aspects and the long-term impacts. Only through continuous exploration and critical analysis can we maximize the benefits of this evolving field and ensure it benefits both patients and the broader healthcare system.
Understanding AI Contract Analysis in Workers' Compensation Law A 2024 Technical Perspective on Claim Documentation Reviews - Regulatory Compliance Verification Through Automated Document Classification Systems
Within the intricate landscape of workers' compensation law, regulatory compliance verification is a crucial aspect, especially given the constant changes in regulations and the significant volume of documentation involved. Automated document classification systems powered by artificial intelligence (AI) offer a promising path forward. These systems can analyze contracts and other documents to automatically identify potential compliance issues, such as outdated language or missing required clauses. This automation can significantly enhance the efficiency of compliance audits, helping to ensure that all parties involved in a claim adhere to the agreed-upon terms and regulations.
The benefits of these systems extend beyond faster audits. They can create a more transparent and readily auditable trail for regulatory reviews, thereby minimizing the risk of penalties for non-compliance. Furthermore, the streamlined processing of claims that results from these systems can optimize overall operations within a field that requires increasingly detailed documentation and strict compliance standards.
While offering many potential benefits, it is essential to be cautious about relying solely on these automated solutions. It's crucial to consider the potential for bias within the AI algorithms used in these systems, which could lead to unfair or inaccurate outcomes. Moreover, the use of these systems raises legitimate questions about the security and privacy of sensitive claim data. Ongoing scrutiny and evaluation of these automated solutions are vital to ensure they are used responsibly and in a way that benefits all stakeholders involved in workers' compensation cases. Only through careful implementation and oversight can the promise of AI-driven regulatory compliance be fully realized.
AI-powered systems for classifying documents are becoming increasingly important in verifying regulatory compliance, especially within the complexities of workers' compensation. These systems offer a lot of promise, but also raise some interesting questions.
For example, dealing with laws about data ownership and control (data sovereignty) is tricky. Regulations vary across different regions, so designing a system that complies everywhere can be challenging. This is particularly important when dealing with sensitive claim data.
But it's not just about classifying documents; these systems can also follow the entire history of a claim document (its lifecycle). This means tracking when it was created, reviewed, changed, or archived, which creates a more transparent and accountable process.
Interestingly, these systems can also adjust their rules based on the latest legal changes or regulations. This dynamic adaptation contrasts with the more static compliance rules of the past. This flexibility could be really beneficial as laws change rapidly.
Furthermore, some researchers are exploring using blockchain technology to validate documents within these systems. This could reduce the risk of fraud in the claims process by ensuring the authenticity of records.
Another notable feature is the inherent scalability of these systems. This means they can easily handle a larger volume of claims without requiring more staff. This potential for reshaping claims management is worth keeping an eye on.
These systems also offer some advanced features like recognizing error patterns. By analyzing past mistakes in documents, the systems can improve their accuracy over time, helping ensure greater compliance in the future.
Another interesting area is the potential to benchmark compliance across different organizations. If data from various organizations is aggregated, we might be able to see common issues, which could lead to better industry practices.
AI can improve how different stakeholders communicate within the claims process. Having access to real-time updates on compliance status could smooth out the audit process, benefitting everyone involved.
It's important to be aware of the potential for bias within AI systems. However, if organizations continuously monitor and audit the systems, they can identify and correct biases in the classification processes, promoting fairness.
Finally, these systems generate very detailed audit trails. Not only do these trails document compliance activities, but they can also help organizations proactively identify potential risks. This allows for more strategic decision-making from a legal and operational perspective.
It seems that automated document classification systems offer a powerful set of tools for ensuring regulatory compliance. While they present new opportunities, it's crucial to continue to evaluate the impact of these technologies on existing processes and evaluate if they are truly beneficial in terms of increased fairness, reduced errors and the broader impact on the individuals whose claims are managed with these systems.
eDiscovery, legal research and legal memo creation - ready to be sent to your counterparty? Get it done in a heartbeat with AI. (Get started for free)
More Posts from legalpdf.io: