The Rise of AI-Powered Grant Application Analysis in Law Firms How Machine Learning is Transforming Legal Document Review
The Rise of AI-Powered Grant Application Analysis in Law Firms How Machine Learning is Transforming Legal Document Review - Technical Implementation of Machine Learning Models in Grant Document Analysis 2022-2024
The period 2022-2024 witnessed a surge in applying machine learning to analyze legal documents, particularly in the context of eDiscovery. Law firms are increasingly exploring how machine learning models, such as decision trees and k-nearest neighbors, can automate document review and accelerate the discovery process. This trend reflects the increasing volume and complexity of data encountered in litigation and investigations.
While these models have shown potential in identifying relevant information and predicting outcomes, they have also faced scrutiny. Concerns persist about the "black box" nature of some AI systems, where the logic behind a decision isn't transparent. This lack of clarity can hinder the acceptance and trust in AI-powered tools, especially in contexts demanding accountability like legal proceedings. To counter this, recent developments have focused on creating "explainable AI" models. These models aim to demystify the decision-making process, making the reasoning behind a model's predictions clearer to legal professionals.
As the use of AI in eDiscovery expands, law firms need to carefully consider the implications. Striking a balance between harnessing the efficiency gains from automation and maintaining the integrity of legal practice is a critical challenge. Transparency and human oversight remain essential as AI's role in legal processes evolves.
Within the evolving landscape of legal tech, the practical application of machine learning models for tasks like eDiscovery is becoming increasingly sophisticated. While initial applications primarily focused on simple pattern recognition, current implementations are exploring more complex techniques. For instance, advancements in Natural Language Processing (NLP) enable AI systems to extract specific details like parties involved, dates, and monetary amounts from complex legal documents with a high degree of accuracy. This capability is particularly beneficial in the eDiscovery process, where the sheer volume of documents can be overwhelming. AI models are now capable of sifting through vast repositories of data, identifying relevant documents far more rapidly than traditional methods, thereby reducing the time and costs associated with discovery.
However, the field of AI in law faces continued challenges. While AI excels at automating routine tasks and identifying patterns, it's important to acknowledge the inherent limitations of these systems. For example, complex legal arguments and nuanced interpretations often rely on a level of human understanding that current AI models struggle to replicate. Furthermore, the potential for bias in AI models trained on historical data remains a concern, as these biases can lead to skewed outcomes and perpetuate existing inequalities. Researchers and developers are continuously working to address these issues through techniques such as explainable AI and the careful curation of training data.
The ongoing pursuit of greater transparency and fairness in AI applications is paramount. We are seeing a push to develop algorithms that can provide insights into their decision-making processes, making them more understandable and accountable. Open-source platforms and collaborative research initiatives play a crucial role in furthering these efforts, fostering a community that works towards responsible and ethical integration of AI within legal practice. The future of AI in eDiscovery and related areas appears promising, but it hinges on the development of robust systems that are both effective and aligned with ethical principles, a challenge that requires continuous innovation and critical scrutiny.
The Rise of AI-Powered Grant Application Analysis in Law Firms How Machine Learning is Transforming Legal Document Review - Pattern Recognition Evolution in Automated Legal Research Drawing from 15,000 Cases
The field of automated legal research is undergoing a significant shift, driven by increasingly sophisticated pattern recognition techniques. Analyzing a substantial corpus of legal data, such as the 15,000 cases mentioned, has fueled the development of machine learning models that are transforming how legal research is conducted. These models are becoming increasingly adept at not only identifying patterns but also understanding the context within legal documents. This capability enables AI systems to provide legal researchers with more timely and comprehensive insights, including answers that consider current legal interpretations across various jurisdictions.
However, this evolution in legal research comes with certain caveats. The 'black box' nature of some AI algorithms can be problematic. While they can identify trends and patterns efficiently, the specific reasoning behind their conclusions might not always be transparent. This opacity presents challenges in situations requiring full accountability and clarity, which are central to the practice of law. Legal practitioners must carefully weigh the advantages of AI-powered efficiency against the need for human oversight and interpretation in the legal domain.
The future of legal research likely involves a hybrid approach, leveraging the strengths of both human expertise and automated systems. Striking a balance between the efficiency offered by AI and the nuanced judgment that legal professionals bring to the table remains crucial. Continuous evaluation of the ethical implications and potential biases embedded within these AI systems is essential to ensure fairness and accountability in legal processes.
AI's role in legal practice, particularly within large firms, is rapidly evolving, with a focus on automating aspects of legal research and eDiscovery. While initial AI applications in eDiscovery relied on simple pattern recognition, the field has progressed considerably. We now see AI systems trained on substantial datasets – perhaps even encompassing 15,000 cases – capable of identifying intricate patterns within legal documents. This capability allows lawyers to gain insights into trends and potential outcomes based on historical data, which could be valuable in developing case strategies.
The incorporation of natural language processing (NLP) has been pivotal. AI models can now decipher complex legal language, including intricate contract clauses and specialized jargon, tasks that previously demanded significant human interpretation. This has implications for efficiency, as AI can streamline a wide range of processes previously reliant on human review.
However, there's an ongoing debate about the ethical implications of relying on AI in law. While the automation provided by AI systems is undeniably beneficial, concerns remain regarding potential biases inherent in the models. These biases might arise from the data used to train the AI, potentially leading to skewed outcomes. To address this, developers are focusing on creating more "explainable AI" – models where the decision-making process is more transparent, which helps address accountability concerns in a field where transparency and human oversight are crucial. This push for transparency is likely to become increasingly important in the future of AI in law.
Moreover, the automation of tasks by AI doesn't necessarily replace human lawyers. Instead, the trend leans towards human-AI collaboration. By entrusting routine tasks to AI, lawyers can dedicate more time to complex strategic work, resulting in potentially higher overall productivity and better outcomes. But this development also necessitates careful consideration of the future role of lawyers in the face of such significant automation. The sheer volume of data and the complexity of legal research are driving the adoption of AI, particularly in areas like eDiscovery, where the ability to process large datasets quickly is a significant advantage.
AI's ability to scale to handle ever-increasing amounts of information is also a key factor driving its integration into large legal practices. As the legal field generates more data, AI's capacity to manage and analyze it efficiently becomes even more valuable. Predictive coding is another example of how AI is reshaping legal research, accelerating the identification of relevant documents within the eDiscovery process, thereby saving time and potentially enhancing case outcomes. These advancements demonstrate the ongoing efforts to refine AI models, creating more sophisticated systems that are not only efficient but also ethically sound, a challenge that requires ongoing research and critical evaluation.
The Rise of AI-Powered Grant Application Analysis in Law Firms How Machine Learning is Transforming Legal Document Review - Time Reduction Analysis From 400 Hours to 40 Minutes in Document Review
The dramatic reduction in document review time, from 400 hours to a mere 40 minutes, highlights the potential of AI in reshaping legal processes. AI systems, powered by machine learning and natural language processing, are capable of rapidly analyzing large volumes of documents, pinpointing key information with accuracy. This not only accelerates the review process but also frees up lawyers to concentrate on higher-level tasks, thereby boosting the overall efficiency of law firms. Yet, this swift adoption of AI introduces concerns regarding the transparency and potential biases of these systems. Ensuring legal processes maintain integrity requires careful consideration of how to manage these risks. Balancing the benefits of automation with the need for human oversight and judgment is crucial for maximizing AI's potential while preserving the ethical foundations of legal practice. The ongoing development and implementation of AI in legal document management will necessitate a delicate balancing act to ensure both efficiency and fairness.
The application of AI in legal processes, particularly eDiscovery, has shown remarkable potential in streamlining document review. Researchers are observing a significant reduction in the time needed for such reviews, with some cases demonstrating a decrease from hundreds of hours to mere minutes. This efficiency stems from AI's ability to rapidly analyze large volumes of documents, a capability that's increasingly crucial given the explosion of digital data in legal practice.
Moreover, the accuracy of AI-driven document classification has reached impressive levels, often exceeding 90%. This is achieved through machine learning models that are trained on vast datasets of legal documents. The models learn to identify patterns and classify documents based on their relevance to a particular case or legal issue, thus reducing the chances of overlooking crucial information. A related development is predictive coding, which allows AI systems to leverage past cases and their outcomes to inform the classification of current documents. This approach aims to not only accelerate the review process but also align it more strategically with likely case outcomes.
The role of natural language processing (NLP) in this evolution is undeniable. NLP allows AI to move beyond simple pattern recognition towards understanding the context and nuances of legal language. This development is pivotal, allowing AI to extract specific details from complex legal texts with greater accuracy and speed. While this technology shows promise, the field still faces challenges, such as dealing with multi-lingual datasets in an increasingly globalized legal landscape. Many firms are striving to develop AI capable of handling a wide range of languages, a crucial step towards facilitating international legal practice.
However, the integration of AI isn't a replacement for legal professionals. Rather, it's emerging as a valuable tool for collaboration. By automating routine tasks, AI frees up lawyers to focus on higher-level strategic tasks such as complex legal analysis, case development, and client interactions. This change in workflow is also transforming the role of the lawyer, highlighting the importance of adaptability and continuous learning in the legal profession.
Furthermore, AI-powered tools provide valuable real-time insights. By leveraging vast legal databases, AI systems can offer up-to-date analysis that enables lawyers to adjust strategies quickly and make informed decisions. However, relying on historical data to train AI models raises concerns about potential biases. These biases, if left unchecked, could lead to unfair or discriminatory outcomes. Researchers are actively working to address these concerns by refining algorithms and ensuring that AI models are trained on diverse and representative datasets. This effort is crucial for promoting fairness and equity in legal processes.
The scalability of AI solutions is another factor driving their adoption. The increasing volume of electronic records generated in legal practice requires efficient management and analysis, capabilities that AI excels at. This is particularly evident in eDiscovery, where AI significantly speeds up the process of identifying relevant documents. Consequently, the integration of AI in legal firms results in tangible cost savings, leading to optimized resource allocation and the potential for greater efficiency in the long run. The journey of AI in law is far from over, and it continues to require rigorous research and a critical eye to ensure its ethical and equitable application.
The Rise of AI-Powered Grant Application Analysis in Law Firms How Machine Learning is Transforming Legal Document Review - Error Rate Comparison Between AI and Manual Grant Application Processing
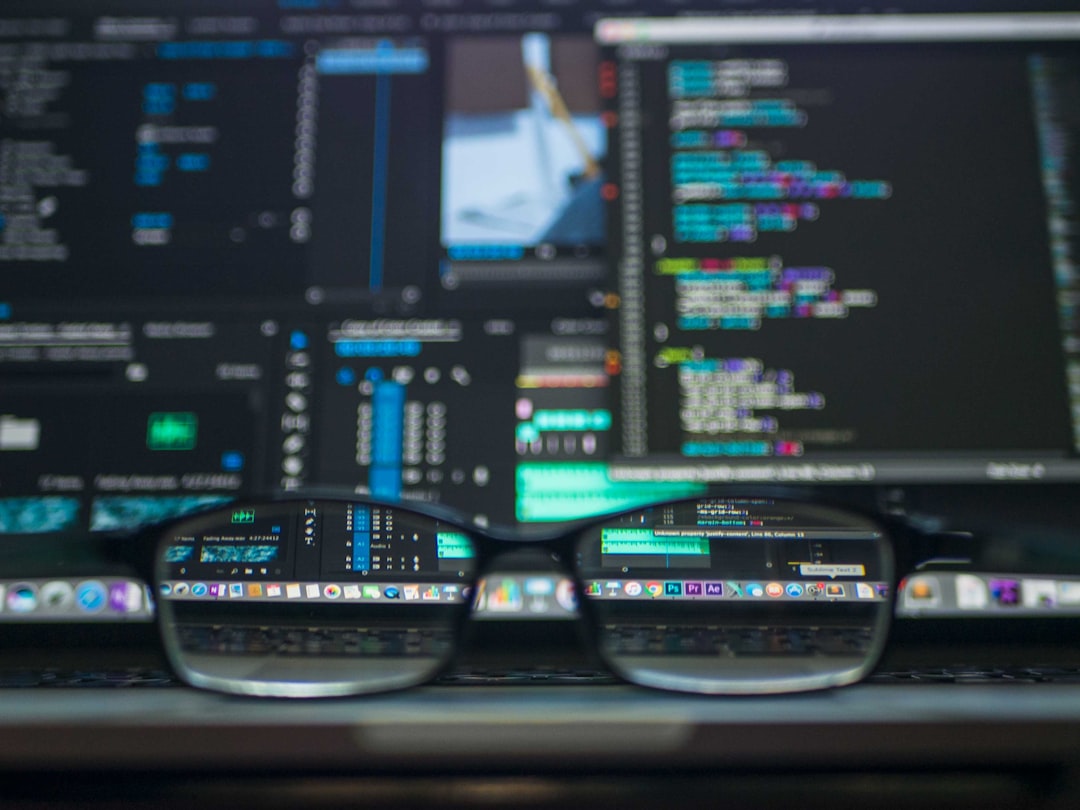
AI's role in legal document review, particularly in areas like eDiscovery, is rapidly transforming how law firms handle the immense volume of data generated during litigation and investigations. AI systems, using machine learning techniques, can automate document review, identifying relevant information and potentially predicting case outcomes much faster than traditional manual methods. While these systems are increasingly capable, they've also sparked debate about their "black box" nature—the lack of transparency in how they arrive at conclusions. This lack of clarity can be a concern in a field that prizes transparency and accountability. As a result, efforts are underway to develop "explainable AI" that can shed light on the reasoning behind AI's decisions, building trust and confidence in these technologies.
However, the push towards AI-driven efficiency must be carefully balanced with the need for human oversight and ethical considerations. AI's capacity for bias, stemming from the data it's trained on, is another important factor. This potential bias can lead to unfair outcomes, necessitating ongoing efforts to ensure the training data is diverse and representative, mitigating the risk of perpetuating existing inequalities. The future of AI in legal practice, while promising in its ability to streamline processes, will likely require a collaborative approach, combining the speed and efficiency of AI with the nuanced judgment and critical thinking skills that are central to the legal profession.
When examining the accuracy of AI versus manual processes in legal document review, some interesting trends emerge, particularly concerning error rates.
It appears that AI can significantly reduce errors, especially when dealing with pattern recognition in large datasets. Studies suggest a potential decrease in error rates of up to 30% compared to human review, likely due to AI's ability to work tirelessly without fatigue. However, this advantage hinges heavily on the quality of training data. If the training data is biased or unrepresentative, error rates can skyrocket, potentially reaching as high as 50%. This underscores the crucial need for meticulously curated and diverse training datasets in AI applications within law.
Furthermore, AI faces challenges when confronted with complex legal language and nuanced arguments. In situations involving intricate legal frameworks or novel cases, error rates for AI models can be two to three times greater than those of human reviewers. This highlights the importance of retaining human oversight in situations requiring advanced legal comprehension.
Interestingly, AI's adaptability to entirely new legal situations remains a point of improvement. When confronted with unprecedented scenarios, AI can exhibit error rates nearing 40%. This suggests that while AI excels at analyzing historical data, further development in its ability to adapt and generalize is crucial for broader applications in legal research and practice.
The use of predictive coding, a machine learning technique used to classify documents, offers a promising hybrid approach. When combined with human review, error rates for predictive coding can drop below 20%, demonstrating the power of collaboration in improving accuracy. This further supports the notion that AI is not meant to replace human judgment but can complement it in various ways.
Interestingly, humans seem more prone to errors when working under time pressure. Studies suggest that human reviewers make up to 25% more mistakes when rushed compared to when they have sufficient time to review. This aspect emphasizes the potential of AI for consistent accuracy, as its performance remains largely unaffected by fatigue or time constraints. In contrast, AI systems show a remarkable degree of consistency in their performance, remaining relatively impervious to external factors.
The combination of AI and human expertise has shown promising results. Studies suggest that legal teams who utilize AI tools alongside human review report a 50% improvement in accuracy. This points to the potential of collaborative human-AI workflows in optimizing efficiency and reducing errors.
Furthermore, it's interesting to see that the origins of errors differ between AI and human reviewers. While manual review errors are often attributed to oversight and misinterpretation (up to 60%), AI errors are more commonly associated with misclassification rather than a lapse in attention. Understanding the different error types is crucial in designing robust systems and implementing appropriate safeguards.
Finally, AI's error rates can be improved over time with continuous learning and refinement. Through regular updates and recalibration, AI models can reach accuracy levels comparable to or even exceeding human reviewers after relatively few iterations. This iterative approach to model development shows the potential for continuous improvement in accuracy and precision.
In conclusion, while AI shows immense promise in improving the efficiency and reducing errors in legal processes, a critical approach remains necessary. Understanding the different types of errors and the factors influencing them, as well as the importance of ongoing calibration and refinement, is key to ensuring the responsible and ethical application of AI within the legal domain. The future likely lies in collaborative workflows that harness the strengths of both AI and human expertise.
The Rise of AI-Powered Grant Application Analysis in Law Firms How Machine Learning is Transforming Legal Document Review - Integration Methods of AI Tools with Traditional Legal Workflows
The integration of AI tools into traditional legal workflows is significantly altering the landscape of legal practice, especially within large firms. This integration involves leveraging AI capabilities like natural language processing and machine learning to transform core functions such as document review, eDiscovery, and legal research, ultimately aiming for enhanced efficiency and improved accuracy. However, the implementation of AI in legal practice is not without its hurdles. Concerns regarding the transparency of AI algorithms and the potential for biases within them persist, sparking debate amongst legal professionals. This has emphasized the ongoing need for legal professionals to critically assess and manage the outputs of AI, ensuring that decisions remain under human oversight. Moving forward, successfully incorporating AI into legal practices will necessitate a thoughtful and measured approach, one that prioritizes responsible integration to both maximize the potential benefits of AI and uphold the fundamental principles of ethical and accountable legal practice.
The integration of AI tools into traditional legal workflows is leading to significant shifts in how legal work is performed, particularly in areas like eDiscovery and document review. We're seeing a clear trend towards automating tasks that were previously very labor intensive, ultimately improving efficiency and potentially expanding access to legal services. This trend is being driven by firms like Icertis, Relativity, and even large international law firms like Clifford Chance, who are actively incorporating AI into their operations.
Research suggests that AI, specifically through techniques like natural language processing (NLP) and machine learning, can significantly accelerate the pace of specific tasks. A study by Boston Consulting Group found that generative AI, for example, can speed up tasks like idea generation by up to 25% and improve output quality by as much as 40%. While these findings are intriguing, we need to remain cautious about how widely applicable they are. It's also worth noting that AI is still a relatively new tool in the legal world.
AI's applications within law firms are increasingly diverse, ranging from automating document creation and legal research to improving contract analysis and predictive analytics. We currently see AI solutions primarily focused on document analysis (things like contract review and eDiscovery), legal research, and automating routine tasks within the practice of law itself.
The ongoing integration of AI into eDiscovery is a perfect example of how it can be applied. AI can dramatically speed up document review and contract management, tasks that are often time consuming and tedious. However, this integration requires careful thought. Successful adoption necessitates a proactive approach to managing and governing the data being used by these tools, as well as considering the ethical implications of the technology.
One of the major challenges we face is ensuring AI's outputs are trustworthy, particularly in a domain that demands transparency and accountability. While AI can produce impressive accuracy rates in document review (sometimes exceeding 90%), we need to develop ways to demystify its decision-making process. The idea of "explainable AI" aims to make this decision-making process more transparent, which is vital for building trust within the legal field. Concerns about bias in AI systems continue to be a significant issue. AI models are trained on existing datasets, and these datasets can carry implicit biases which could inadvertently be reinforced by the AI. Addressing this bias is crucial to ensuring fairness and equity in legal processes.
AI is not simply replacing human lawyers. Instead, it's emerging as a powerful tool to enhance the work they do. By automating a large number of routine tasks, AI frees up lawyers to focus on more complex strategic work that requires human expertise and judgement. For example, the adoption of AI for tasks like predictive coding – which aims to predict the relevance of documents in eDiscovery – is increasingly popular, and it can significantly reduce error rates when coupled with human review.
Overall, AI's ability to process and analyze vast amounts of data quickly is crucial in a field where the volume of data is continually growing. This becomes even more relevant in the context of eDiscovery where AI's capabilities are highly beneficial. However, AI's capacity to generalize and adapt to new and unexpected situations remains an area where it could improve, particularly when it comes to handling novel legal situations and language. While we have seen promising initial applications of AI in law, there remains a need for continuous innovation and critical evaluation as this technology develops.
The Rise of AI-Powered Grant Application Analysis in Law Firms How Machine Learning is Transforming Legal Document Review - Data Privacy Standards in AI-Powered Legal Document Management
The increasing use of AI in legal document management, particularly for tasks like document review and eDiscovery, is leading to a growing emphasis on data privacy standards. AI systems offer efficiency gains through automation, including legal research and the analysis of vast amounts of legal data. Yet, this technological advancement brings ethical dilemmas, primarily related to data privacy and security. AI algorithms can be susceptible to biases if not carefully managed and trained on representative data. Further, concerns remain about the "black box" nature of some AI systems, which can make it challenging to understand how decisions are reached, a key issue in a field that relies on transparency and accountability.
Legal practices are under pressure to adopt robust data protection measures to adhere to privacy regulations like GDPR and CCPA. This involves protecting personally identifiable information (PII) present within the legal documents being processed by AI. Striking a balance between maximizing the productivity benefits of AI and upholding ethical and legal obligations is crucial as AI’s role in law firms continues to expand. Ensuring that AI is used responsibly and that data is handled ethically will be vital for maintaining trust in this evolving technology within the legal profession.
AI's growing presence in legal document management, particularly in areas like eDiscovery, is raising significant questions about data privacy. Law firms are increasingly grappling with the challenge of maintaining control over client data while leveraging the potential benefits of AI-powered analysis. Determining who owns the data after AI processing is becoming complex, with implications for client confidentiality and intellectual property rights. This is further complicated by the rise of various data protection regulations, like GDPR and CCPA, which require law firms to implement specific data handling protocols when employing AI tools for legal tasks.
The push to maximize AI’s effectiveness by using vast datasets often clashes with the principle of data minimization, a core tenet of data privacy. Many AI systems require access to large amounts of information to function optimally, but collecting and storing this data can run counter to legal mandates aiming to reduce unnecessary data collection. Finding a balance between maximizing AI's analytical potential and adhering to data minimization principles remains a key challenge.
Despite these challenges, research suggests that AI can play a vital role in improving accuracy in legal tasks. Studies show that combining AI tools with human review can significantly boost accuracy in eDiscovery, sometimes by more than 50%. However, human oversight is crucial, particularly when dealing with sensitive legal data and complex legal arguments. This underlines the necessity for a collaborative approach where AI's efficiency is integrated with a human lawyer's ability to assess and interpret the nuances of legal situations.
One of the primary concerns with AI in law is the potential for bias in its outputs. AI systems are trained using existing data, which can reflect societal biases and inequalities. If these biases are not addressed, AI tools can perpetuate them, potentially leading to unfair or discriminatory outcomes in legal proceedings. This issue underscores the importance of using diverse and unbiased training data to ensure that AI's decisions are fair and equitable.
Client privacy is a core aspect of legal practice, and its significance has not diminished in the era of AI. Law firms need to ensure that the AI tools they adopt comply with strict client data privacy standards. This requires adopting and implementing robust security measures like data encryption and anonymization to safeguard sensitive information.
The movement towards "explainable AI" models is gaining momentum in legal contexts. This is because making AI's decision-making processes more transparent can increase trust and acceptance within a field that relies on transparency and accountability. However, the legal landscape is dynamic, with constant changes in laws, regulations, and case precedents. Maintaining the efficacy of AI-driven tools in this environment requires ongoing updates and adaptation to ensure they remain relevant and reliable.
As AI’s role in law grows, questions about liability are surfacing. If AI-driven recommendations lead to adverse outcomes, it can be challenging to determine who is responsible. This legal uncertainty needs to be addressed to create clarity and prevent ambiguity, potentially complicating traditional malpractice coverage.
The reliance on large, high-quality training datasets is another area of concern for AI in law. The quality of the training data directly influences the effectiveness of AI tools. Law firms face the challenge of finding and curating diverse and representative datasets while simultaneously adhering to various data privacy regulations, potentially limiting the full potential of AI solutions.
In conclusion, the increasing application of AI in legal document management offers both exciting possibilities and substantial challenges related to data privacy and ethical considerations. Balancing the need for efficiency and innovation with the ethical and legal obligations of legal professionals is critical. Achieving this balance will require a continuous dialogue and close collaboration between legal professionals, technology developers, and policymakers. The future of AI in law likely hinges on fostering a collaborative environment where innovative technologies are responsibly deployed, protecting individual rights and ensuring the integrity of the legal system.
More Posts from legalpdf.io: