The Evolution of E-Discovery in AI-Assisted Contract Review A 2024 Perspective
The Evolution of E-Discovery in AI-Assisted Contract Review A 2024 Perspective - AI-Driven Document Review Slashes Time from 27 to 5 Days
Artificial intelligence is dramatically altering the way legal teams manage the document review phase of e-discovery. Instead of the typical 27-day review period, AI-powered systems can now complete the same task in just 5 days. This remarkable reduction in time is a result of AI's ability to swiftly process large datasets, including diverse file types like emails, texts, and multimedia. We've moved beyond simply discussing the potential of generative AI in e-discovery; it's now being actively used in real-world scenarios. This shift necessitates that legal professionals adapt, developing stronger critical thinking and collaborative skills to work alongside these powerful new tools. As efficiency increases, law firms are considering moving away from traditional billing models based on hours worked and exploring alternative approaches like flat fees or subscriptions. This efficiency boost isn't limited to e-discovery; the ability to quickly sift through massive datasets is also proving invaluable in areas like corporate due diligence during mergers and acquisitions. Overall, AI is fostering a profound shift in how legal tasks are conducted, significantly improving speed and effectiveness.
Recent advancements in AI are dramatically reshaping document review in e-discovery. We've seen a remarkable shift, with some cases demonstrating a reduction in review time from a typical 27 days to a mere 5 days using AI. This isn't just a matter of speed; it signifies a fundamental change in how we approach legal tasks. The potential for generative AI to handle real-world e-discovery scenarios is becoming increasingly apparent, moving beyond theoretical discussions.
This shift is evident in firms, some even within the Am Law 200, experiencing over 90% reductions in review times. AI excels at sifting through diverse data sources – emails, messages, multimedia – streamlining the process in ways previously unimaginable. While AI drives the initial review, it's important to remember that human review remains crucial. AI evaluations are often followed by human checks to ensure accuracy, reflecting an iterative process. It's this collaborative approach that's helping refine the technology and its integration into the legal field.
This rapid change in e-discovery practices is also sparking discussions about how law firms operate. The efficiency gains could lead to a transition from traditional hourly billing models to fixed fees or subscriptions. In addition to e-discovery, the benefits of AI are being seen in other areas, like corporate due diligence for mergers and acquisitions, where AI's ability to handle massive volumes of documents is incredibly valuable. However, this rapid shift also requires us to be mindful of developing a critical understanding of AI and its place in the legal landscape. We need to develop skills in critical thinking, alongside technical expertise, to ensure that these powerful tools are used responsibly and effectively.
The Evolution of E-Discovery in AI-Assisted Contract Review A 2024 Perspective - GenAI Achieves 70% Recall Rate Comparable to Traditional TAR
Generative AI (GenAI) has shown promising results in e-discovery, achieving a recall rate of roughly 70%. This is comparable to what established Technology-Assisted Review (TAR) methods can deliver, demonstrating its potential as a viable alternative. Some suggest GenAI can potentially reach even higher recall rates, possibly hitting the 90% mark with further development. This faster route to accuracy, compared to traditional TAR, is noteworthy.
However, the increased adoption of GenAI has brought about worries about its environmental impact, highlighting the urgent need for more sustainable AI implementations. Legal professionals are starting to adopt these tools and, as this continues, it's crucial to approach them with a focus on balancing efficiency with ethical considerations, data security, and proper governance. This evolution showcases a broader movement in legal tasks where AI takes on an increasing role in streamlining and improving processes, but careful attention to these aspects will be important as these tools become more integral.
Recent developments in generative AI (GenAI) for e-discovery are quite interesting. We've seen that these models can achieve a recall rate around 70%, which is roughly on par with traditional Technology-Assisted Review (TAR) methods, at least initially. This is promising, but it also means a sizable portion of relevant documents (potentially 30%) might be missed. It highlights that while AI can be helpful, human oversight during review is still essential.
Interestingly, TAR versions 2 and 3 sometimes still perform better than GenAI in some recall tests. This suggests that while GenAI has potential, it's not yet a clear-cut improvement over all existing methods in every situation. It appears that GenAI’s performance is heavily reliant on the specific algorithm and the type of training data used. This variability can lead to wide swings in results across different cases, so it's crucial to carefully evaluate and choose the right AI tool for each job.
The data itself seems to influence GenAI's performance. Very sensitive or specialized legal content can be trickier for AI to analyze, which means it's important to remember that AI's capabilities aren't always universal and understanding the context of the legal material is critical. Also, despite the benefits, we can't overlook the importance of maintaining data privacy and complying with all the necessary legal regulations when using AI for document review. It’s a balancing act between speed and responsibility.
One of the reasons GenAI is improving is advancements in how these models are trained. Using bigger, more diverse datasets and more advanced model architectures helps improve their ability to learn. The ongoing refinement through feedback mechanisms also plays a key role. It's worth mentioning that while AI can help make review faster, integrating these systems into existing workflows can be costly. Firms need to consider these costs when weighing the potential time savings.
It seems that we sometimes get so focused on high recall rates that we overlook the importance of getting things right. A very high recall without a strong focus on precision can create a different type of problem: more work for legal teams sifting through false positives.
A big benefit of GenAI is its ability to predict relevance, which can further streamline the process. This is very helpful, but it depends on continual model training and tuning based on feedback. Overall, it looks like we're moving towards a future where humans and AI collaborate in e-discovery. While GenAI can be a huge help for handling vast amounts of data, human expertise is still required to ensure complex legal matters are understood and to help avoid the pitfalls that come with relying solely on algorithms.
The Evolution of E-Discovery in AI-Assisted Contract Review A 2024 Perspective - Iterative Review Process Refines AI Models for Enhanced Accuracy
The iterative review process is a key driver in the evolution of AI models, especially within AI-assisted contract review. This continuous cycle of evaluation and refinement ensures that AI outputs become progressively more accurate, which is crucial for effectively identifying relevant documents during e-discovery. The process involves scrutinizing the AI's performance and using that feedback to improve the algorithms, ultimately boosting the reliability of AI-driven results. Generative AI significantly contributes to this refinement process, but it's important to acknowledge that human oversight still plays a critical role. This collaboration between humans and machines is vital to ensure that efficiency gains from automation are balanced with the need for meticulous accuracy and comprehensive understanding, particularly in the intricate world of legal contracts. As the reliance on AI within e-discovery grows, it's essential to be mindful of potential biases and their influence on the decision-making process. We need to ensure these evolving technologies are used responsibly and thoughtfully.
The iterative review process is essentially a cycle of continuous improvement for AI models. By repeatedly evaluating the outputs of AI systems, like those used in contract review, we can refine their accuracy over time. This process involves making small adjustments to model parameters with each iteration, leading to gradual improvements in how effectively the system identifies relevant documents. This fine-tuning is particularly important when dealing with the complexities of legal language and context.
Human feedback plays a crucial role in this process. Not only does it help correct errors in AI outputs, but it also helps to train the model on specific legal terminology and the nuances of particular cases. This leads to better recall – capturing more relevant documents – and higher precision – reducing false positives.
One intriguing aspect of this iterative approach is its ability to uncover subtle patterns in document relevance that might be missed by more traditional review methods. This can allow legal teams to predict which documents are most likely to be relevant, even before the entire review process is complete. This can potentially save a significant amount of time and resources.
Furthermore, the iterative process can highlight anomalies and outliers in the data. These outliers can reveal weaknesses in the AI's understanding of certain document types or legal frameworks. Identifying these weaknesses allows for modifications to either the model itself or the way it is trained.
To further ensure that the models generalize well to different types of legal documents, statistical methods like cross-validation are commonly used within this iterative framework. This helps to prevent overfitting to a single specific dataset, making the AI system more adaptable and reliable.
We can even incorporate diverse types of data sources into the iterative review, making the AI more robust. By being exposed to a wider range of document formats, legal jargon, and legal practices from various jurisdictions, the AI becomes more resistant to unexpected variations in the documents it encounters.
This cycle of evaluation and refinement also builds trust and transparency. The constant feedback loop makes the impact of adjustments easily visible, showing how changes in the model affect the outcomes and the decision-making processes.
For law firms to successfully integrate iterative review processes, there needs to be a shift in culture. A collaborative environment, where human legal expertise and AI systems work hand-in-hand, is essential. It's a move away from a skeptical viewpoint and towards a more synergistic approach to technological advancement.
The fascinating thing about this iterative refinement is that it transforms AI from just a tool into something more dynamic. It evolves and adapts to legal practices over time, potentially changing how legal documents are understood and managed in the long run. It’s still early days in this evolution, but the potential is significant.
The Evolution of E-Discovery in AI-Assisted Contract Review A 2024 Perspective - AI Addresses Keyword Search Limitations in eDiscovery
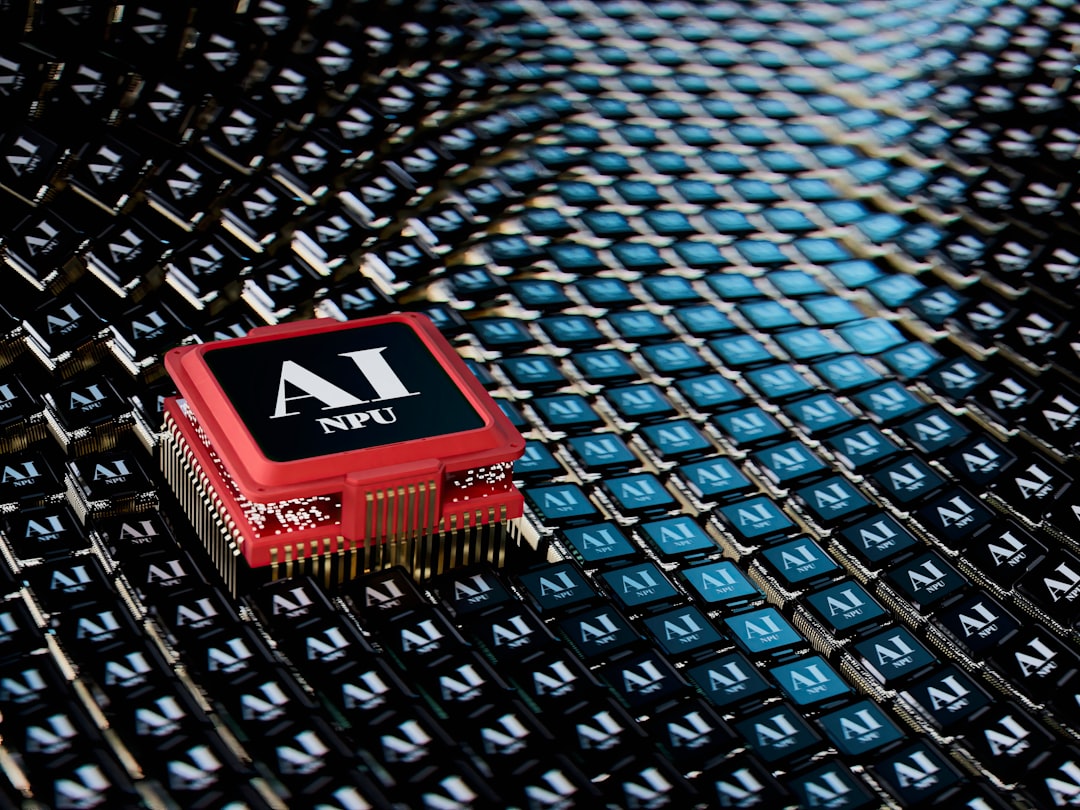
Traditional keyword searches in eDiscovery, while seemingly simple, often fall short, leading to the exclusion of crucial documents. AI is changing this by incorporating methods like predictive coding and data analytics, improving the accuracy and overall quality of document review. These tools help lawyers better grasp the context surrounding digital documents, allowing for a more comprehensive understanding than keyword searches alone can provide. Technology-assisted review (TAR) reflects this shift, utilizing AI algorithms to enhance the discovery process by more effectively identifying relevant materials. This, in turn, translates to faster review cycles and reduced costs. As AI becomes more integral to eDiscovery, the legal community needs to thoughtfully consider the accompanying challenges and ethical implications this evolving technology brings.
In the realm of e-discovery, the traditional approach of keyword searches often falls short, potentially missing up to 30% of relevant documents. This limitation arises from keyword searches' rigid reliance on pre-defined terms, which can fail to capture the nuances and context embedded within legal documents.
AI systems, however, have the capacity to learn and adapt throughout the document review process. By analyzing both successful and unsuccessful classifications, these systems can progressively refine their understanding of legal language and context, ultimately enhancing the precision and accuracy of document identification.
Interestingly, the recall rate of generative AI in e-discovery displays variability depending on the specific algorithms employed and the quality of the training data used. While some implementations surpass established methods like traditional TAR, others may lag behind. This underscores the need for careful consideration and evaluation when choosing an AI tool for a particular legal task.
Though AI can dramatically reduce the time spent on document review, it's essential to remember that implementing and maintaining these systems requires investment. The initial setup and ongoing training necessary can sometimes offset the time-saving benefits achieved through rapid data processing.
The human-AI collaboration is crucial for effective AI-assisted review. Human reviewers not only correct errors in AI classifications but also serve as the primary trainers for refining algorithms. Human expertise is particularly valuable in conveying the complex and often nuanced legal context that AI alone may struggle to decipher.
While AI offers significant advantages, it's important to acknowledge that it doesn't inherently eliminate biases. In fact, if the training data isn't diverse and representative, AI systems can unknowingly amplify existing biases in the decision-making process.
The ongoing nature of AI learning ensures that errors from previous document classifications inform and improve future performance. This iterative process creates a dynamic learning environment where continuous refinement is paramount for achieving desired results.
Legal contexts can vary considerably across jurisdictions and specialized fields. AI systems can struggle with certain legal nuances, particularly those found in specialized areas. Therefore, tailoring the AI's training to include specific legal terminology and document types becomes critical for optimal performance.
One of AI's strengths in e-discovery is its ability to analyze vast datasets and identify patterns and correlations that might be missed by human reviewers. This capability boosts the predictive power of document relevance, improving the overall efficiency of the discovery process.
As AI becomes more deeply integrated into e-discovery workflows, legal teams will need to invest in training beyond simply using the tools. Understanding the limitations of AI is equally important, fostering a collaborative approach that appreciates both the strengths of technology and the crucial role of human legal expertise.
The Evolution of E-Discovery in AI-Assisted Contract Review A 2024 Perspective - Neural Network Deep Learning Tools Identify Critical Documents
Neural network deep learning is transforming how we find critical documents during e-discovery. These advanced AI tools can analyze huge amounts of data, going beyond basic keyword searches to more accurately pinpoint relevant documents. While neural networks show promise in this area, their ability to handle new and different types of data is still a concern. This means it's crucial to keep humans in the loop to fine-tune the systems and ensure they truly understand the complex language and context of legal matters. As deep learning tools become more integrated into legal workflows, it's vital to manage the pace of change carefully. This includes considering the ethical implications and ensuring these technologies are used responsibly and thoughtfully to complement, not replace, human expertise in the legal field.
Neural networks, with their layered structures, are proving quite effective at pinpointing crucial documents. Each layer in these networks analyzes data at progressively higher levels of abstraction, helping them grasp the intricate nature of legal language and uncover subtleties often overlooked by traditional methods.
Some of the current neural network models achieve impressive accuracy in document categorization, with some even exceeding 90% in certain instances. This capability is truly remarkable, showcasing their power to quickly sort through vast quantities of documents, potentially millions, in a fraction of the time it would take humans.
One of the neat things about neural networks is their ability to adapt. Continuous training allows them to fine-tune their performance based on new data, making them especially valuable in a constantly evolving field like law where terminology and case law change over time.
The use of semi-supervised learning methods is gaining popularity, a smart way to leverage unlabeled documents in datasets. This approach is attractive as it reduces the need for manual labeling while still boosting model performance by using a wider range of data.
Despite these advances, there are some issues with understanding exactly how neural networks arrive at their decisions. The "black box" nature of these complex systems presents challenges in legal contexts. It's not enough just to know that a document is tagged as critical; understanding *why* it's flagged is sometimes equally, if not more, important.
Neural networks are very good at recognizing intricate patterns in data, leading to improved predictive coding methods. These methods can anticipate which documents might be relevant based on past review patterns, leading to a potential reduction in review cycles while maintaining accuracy.
However, there's a growing concern about the possible introduction of bias from the training data used to create these models. If the training data isn't diverse and reflective of the real world, these systems could inadvertently perpetuate or amplify existing biases in how documents are classified. This issue needs careful consideration.
The quality of training data is crucial. Neural networks are only as good as the information they're trained on. If the training data is poorly curated, it can result in erroneous document classifications, highlighting the need for robust data management procedures when creating AI systems for legal use.
Neural networks can be designed to handle a variety of document types, such as images and text, simultaneously. This broad capability is especially useful in legal scenarios where multimedia evidence can be important.
Integrating neural networks into legal processes isn't just about saving time; it fundamentally reshapes how legal teams work together. By taking on time-consuming tasks, AI allows legal professionals to focus on more complex, strategic aspects of cases and judgment.
The Evolution of E-Discovery in AI-Assisted Contract Review A 2024 Perspective - Legal Profession Adapts with Soft Skills Training for AI Integration
The integration of AI into legal practices, particularly in areas like e-discovery and contract review, is prompting a shift in how legal professionals operate. With a large majority of law firms expecting AI to significantly influence the legal profession, it's clear that adaptation is crucial. This means lawyers need to develop and enhance their soft skills – particularly collaboration, critical thinking, and effective communication – to successfully partner with AI technologies.
While AI can streamline tasks like document review and potentially boost accuracy, it's not without its challenges. The potential for bias in AI systems and the need for human oversight to maintain ethical standards and legal accuracy are critical concerns. The legal field is navigating a delicate balance between harnessing AI's potential for efficiency and ensuring that the human element remains essential for navigating the complex nuances of legal matters.
This evolution requires a forward-thinking approach to legal education and professional development. Lawyers must embrace a mindset of continuous learning, developing a critical understanding of AI's capabilities and limitations. The goal isn't to replace human expertise with AI, but to create a collaborative environment where AI tools effectively support and augment the intricate work of legal professionals. As AI's role in the legal field continues to expand, a robust understanding of ethical considerations and best practices will become ever more important.
The legal field is starting to understand that, alongside the technical aspects of AI, skills like empathy, collaboration, and communication are becoming increasingly important. These "soft skills" are essential for building a smooth workflow between lawyers and AI tools during the document review process.
Studies have shown that when lawyers receive training in these areas, they become more effective at managing the AI systems used in document review. They can better translate what the AI is outputting into real-world legal strategies. This leads to smoother workflows, better outcomes, and higher productivity. It also seems that soft skills can help to reduce the likelihood of human biases affecting decision-making when AI-generated insights are part of the equation. Lawyers can be trained to think critically about the output of AI instead of just taking it as fact.
This focus on human skills is also critical for building and maintaining relationships with clients. The use of AI is still relatively new, and being able to clearly communicate both the potential benefits and limitations of AI is vital for establishing trust. The ability to connect with clients and convey this information in a way they can understand is becoming more crucial in the current landscape.
I think one of the most interesting aspects is how soft skills can help lawyers provide better context to AI systems. This could, in turn, lead to more precise document analysis in tasks like e-discovery. This interplay between human context and AI algorithms might ultimately mean that we move away from relying solely on purely technical solutions, moving towards a more hybrid approach.
These soft skills are also important from an ethical perspective. They're necessary for responsible AI usage, ensuring it aligns with the existing legal and ethical norms of the profession. With rapid changes in technology, the legal world is transforming at a quick pace, so the ability to adapt to changes quickly is going to be extremely useful.
Additionally, encouraging a more diverse range of thought processes within legal teams can lead to more creative solutions to integrating AI. It's likely that this will be essential for tackling challenging legal problems in the future. I think that a greater emphasis on communication also helps to improve the feedback loops between lawyers and AI, which is important for the iterative process that helps to train and refine these AI systems over time.
It seems clear that if the legal field wants to stay current with the evolution of AI, lawyers will need to constantly develop their soft skills, alongside technical expertise. This ongoing investment in human skills will not only enhance current AI systems but also prepare the legal field for future advancements. It’s a change that will be important for navigating both challenges and opportunities in the future of the legal profession.
More Posts from legalpdf.io: