eDiscovery, legal research and legal memo creation - ready to be sent to your counterparty? Get it done in a heartbeat with AI. (Get started for free)
Small Language Models in Legal Tech The Next Frontier for Efficient eDiscovery and Document Analysis
Small Language Models in Legal Tech The Next Frontier for Efficient eDiscovery and Document Analysis - Efficiency Gains in eDiscovery with Small Language Models
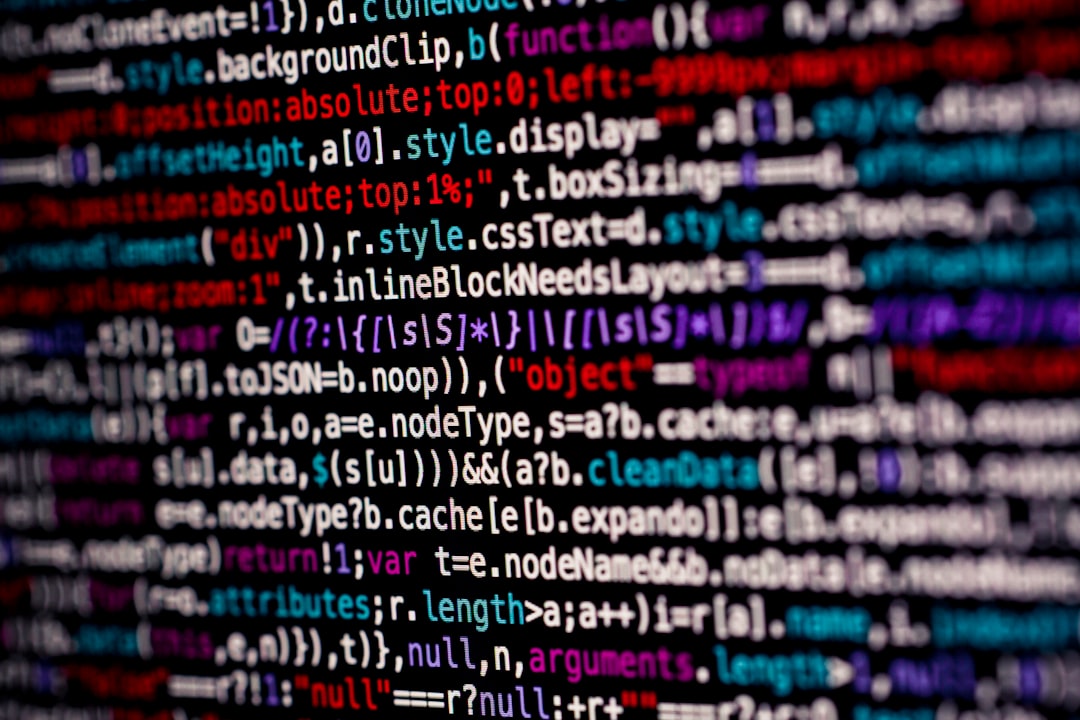
The integration of Small Language Models (SLMs) into eDiscovery processes signifies a notable shift in how legal technology is utilized. These specialized models are designed to excel in specific tasks, offering potential improvements in the accuracy and relevance of document review. Compared to larger language models, SLMs can achieve similar, or potentially better, outcomes while demanding fewer computational resources, which translates to cost savings and reduced environmental impact. The BLADE framework highlights an intriguing approach to leverage both large, general-purpose language models and small, domain-specific models, demonstrating the benefits of hybrid AI solutions in eDiscovery, especially within TAR workflows. The emerging trend of SLMs in legal tech suggests that smaller, targeted AI models can be remarkably effective for complex tasks like document analysis. This increasing reliance on SLMs within eDiscovery workflows is part of a larger trend where efficiency and sustainability are gaining importance in legal practice, making the field more responsible and economically viable.
Specialized language models, often termed small language models (SLMs), are proving increasingly useful in eDiscovery, especially given their efficiency in terms of computational needs and memory usage compared to their larger counterparts. This efficiency stems from their design, focusing on specific legal tasks rather than attempting broad general knowledge. Consequently, within their specialized domains, they can achieve higher levels of accuracy and relevance in identifying key documents and information.
The development of frameworks like BLADE, which incorporates domain-specific small models alongside broader language models, highlights the growing interest in leveraging SLMs for specific legal tech challenges. Electronic discovery, a complex and time-consuming process, naturally emerges as an area ripe for SLM innovation. The Technology-Assisted Review (TAR) process, reliant on AI and language processing, is central to these advancements.
While large language models (LLMs) have enjoyed widespread adoption in natural language processing (NLP), recent research suggests that SLMs can be remarkably effective, even in tasks like zero-shot text classification, potentially challenging the assumed dominance of their larger counterparts. This trend towards smaller, task-specific models reflects a shift in the broader field of AI and machine learning towards more practical applications.
The training of these models can, however, be resource-intensive. Take, for instance, the Phi2 model, which necessitates processing massive datasets and utilizes substantial computing resources. This underscores the need for a balance between model capabilities and resource consumption.
Ultimately, the adoption of SLMs in eDiscovery promises to increase efficiency and cost-effectiveness for law firms. This includes reduced environmental impact due to lower energy consumption during model operation. While there are questions about accuracy and reliance on AI in legal contexts, empirical studies and real-world applications are demonstrating SLMs' capacity to improve legal practices in tangible ways. The shift towards SLM integration in eDiscovery, and perhaps in other aspects of legal practice, could mark a significant change in the industry's efficiency and approach to complex legal data management.
Small Language Models in Legal Tech The Next Frontier for Efficient eDiscovery and Document Analysis - Adapting Technology-Assisted Review for Modern Legal Challenges
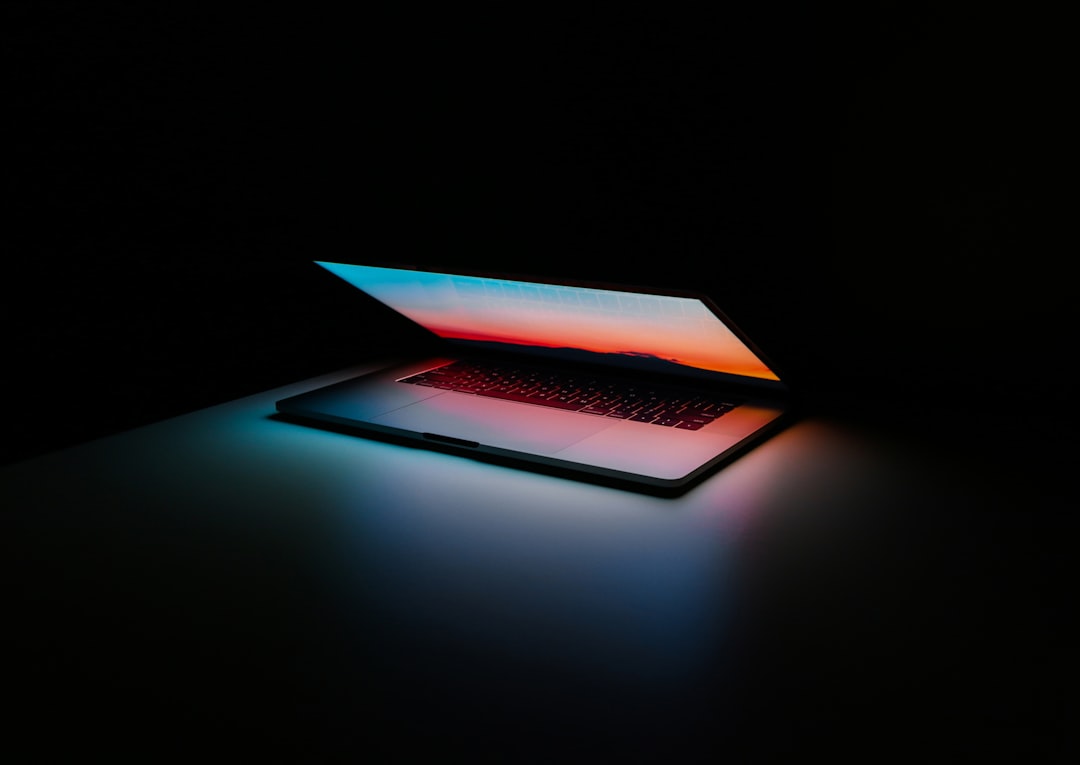
Adapting Technology-Assisted Review (TAR) to meet today's legal challenges requires a careful look at how artificial intelligence is changing the field. The need for greater efficiency and accuracy in legal processes is driving the adoption of technologies like Small Language Models (SLMs). These specialized models, honed for specific legal tasks, significantly improve the way documents are reviewed by identifying key information and reducing the workload. This translates into cost savings and a smaller environmental footprint compared to the resource-intensive methods of the past. Yet, as law firms incorporate these sophisticated AI tools, they also need to address ethical concerns, such as potential biases in AI outputs and the need for transparency in their decision-making. Striking a balance between the efficiency that AI offers and the essential role of human lawyers in ensuring responsible use of these tools is a critical aspect of the future of legal technology.
The application of AI in law, particularly in areas like eDiscovery, is rapidly evolving. Technology-Assisted Review (TAR), powered by AI algorithms, is becoming increasingly sophisticated, with small language models (SLMs) showing promise in refining the document review process. While larger language models (LLMs) have gained popularity, the efficiency and specialization of SLMs are proving advantageous in legal contexts.
SLMs can significantly expedite document review, potentially reducing review times by a considerable margin. This efficiency translates into cost savings, a crucial factor for firms facing budget constraints. Moreover, as legal standards adapt to include TAR methodologies, adopting SLMs aligns firms with evolving best practices, potentially impacting case outcomes favorably.
Furthermore, the accuracy of predictive coding, a core aspect of TAR, is being enhanced by SLMs, reaching levels exceeding 90% in some cases. This demonstrates that smaller, specialized models can indeed perform complex legal tasks effectively, challenging the perception that larger models are always superior.
SLMs offer a more resource-efficient approach, requiring less computational power and memory compared to LLMs. This practicality makes it easier for firms to integrate SLM-powered tools into their existing infrastructure, even with limited resources. By specializing in specific legal domains, such as contract analysis or case law research, SLMs can tailor their insights to a particular firm's area of expertise, leading to greater relevance and accuracy.
The ability of SLMs to process and analyze data streams in real-time can also be leveraged for compliance monitoring. Automatically flagging potentially problematic documents helps firms stay ahead of regulatory requirements. In addition, legal professionals find SLMs easier to use compared to LLMs, requiring less technical expertise.
The benefits of SLMs extend to hybrid systems that pair them with LLMs, leveraging the strengths of each model type. Finally, SLMs are revolutionizing legal research, enabling a substantial increase in research output, ultimately accelerating and improving the quality of legal scholarship.
Despite the potential benefits, concerns about the accuracy and reliability of AI in legal contexts remain. However, empirical studies and real-world applications are demonstrating the ability of SLMs to positively impact legal practice. This ongoing evolution in the use of AI in law is a dynamic process, requiring continued research and evaluation to fully understand the implications of integrating these technologies. As we move forward, navigating the ethical and practical considerations surrounding AI in the legal field will be critical in realizing the full potential of these innovative tools.
Small Language Models in Legal Tech The Next Frontier for Efficient eDiscovery and Document Analysis - Phi2 Model Applications in Document Analysis for Law Firms
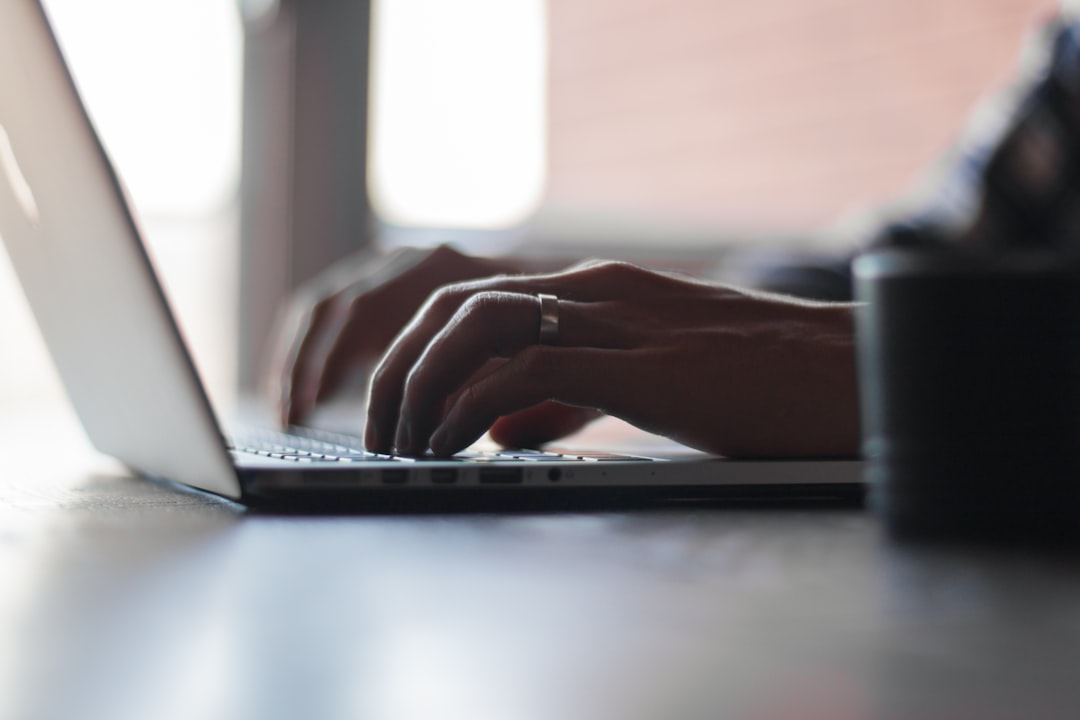
Phi2, a relatively small language model developed by Microsoft, is making waves in the legal tech landscape, particularly within document analysis for law firms. Built with 270 million parameters and trained on a massive dataset of 14 trillion tokens, it demonstrates the growing power of smaller models in handling complex tasks. Phi2's design prioritizes efficiency and versatility, making it a strong contender for applications like eDiscovery and document review where rapid and accurate processing is crucial. Its ability to analyze legal documents more efficiently can potentially save firms significant time and money, compared to older methods. While integrating any advanced AI system into established legal practices requires careful consideration – including acknowledging the potential for biases and the importance of human oversight – the impact Phi2 could have on legal workflows is undeniable. This focus on specialized, smaller AI solutions within law firms mirrors a larger trend toward creating more tailored tools, capable of significantly improving the handling of intricate legal documents.
Phi2, a small language model developed by Microsoft, represents a fascinating development in the intersection of AI and law. Trained on a massive dataset of 14 trillion tokens, it packs 270 million parameters into a surprisingly efficient package. Its training process, though resource-intensive using 96 A100 GPUs over 14 days, resulted in a model that outperforms larger counterparts like Meta's Llama in several benchmark tests for linguistic tasks. This is intriguing, as Phi2 wasn't fine-tuned using reinforcement learning from human feedback (RLHF), a technique commonly employed in other models.
What makes Phi2 particularly interesting in the context of legal tech is its ability to be customized for specific legal document review tasks. This is reflective of a wider trend – a growing recognition that tailored AI solutions can be highly effective. Phi2's success in tasks like reasoning and language comprehension highlights that smaller models can be remarkably potent in specialized areas, challenging the common assumption that bigger models are always better.
We are seeing a surge in open-source small language models (SLMs), which could fuel even more advancements in areas like retrieval-augmented generation (RAG) and customized NLP applications in legal tech. This opens up possibilities for further tailoring and specialization in legal document analysis and legal research.
However, there's a cautionary note here. While SLMs can significantly enhance document review, potentially speeding it up by as much as 50% and cutting costs, their effectiveness relies on the quality and representativeness of the data they are trained on. There's a risk of biases, just like with other AI models, so legal professionals need to be aware of these potential pitfalls and actively mitigate them.
The potential of SLMs like Phi2 to transform legal practice is clear, especially in streamlining document review processes and sharpening the focus of legal research. However, the journey to fully integrating AI into legal workflows will require a careful balance between efficiency gains and the crucial role of human judgment and legal expertise. As with any transformative technology, ethical considerations and responsible deployment are paramount as we explore the future of AI in law. It's an evolving landscape with exciting prospects but also some uncertainties that require ongoing investigation and critical engagement from both researchers and practitioners.
Small Language Models in Legal Tech The Next Frontier for Efficient eDiscovery and Document Analysis - Integration of NLP in Contract Analysis and Audio Transcription
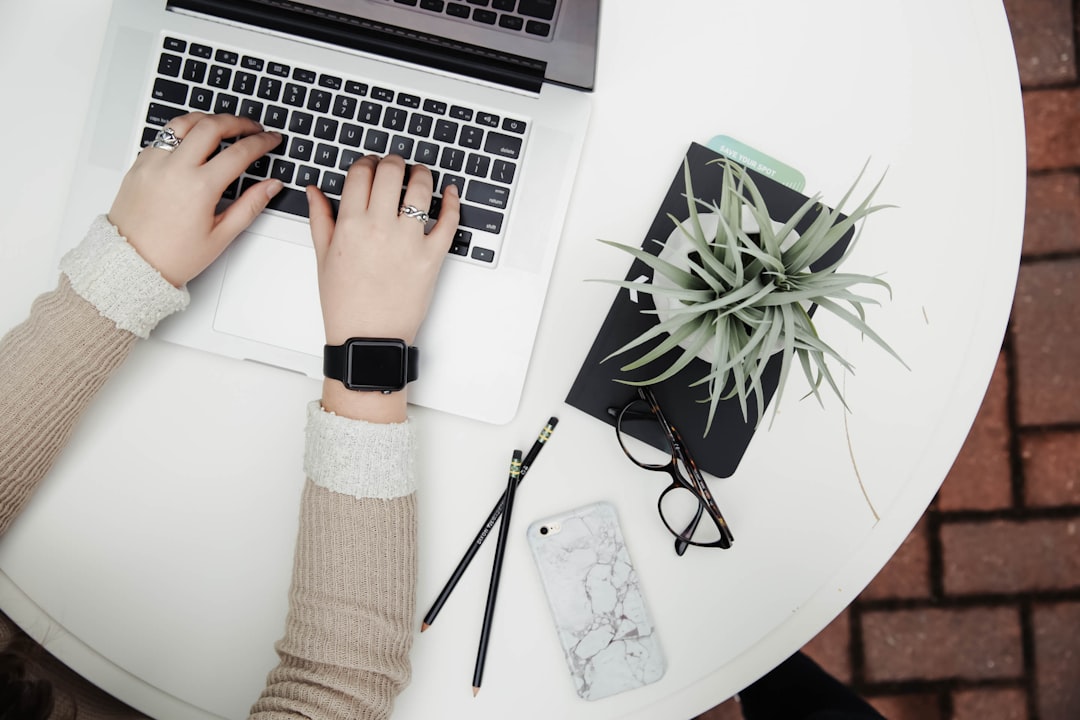
The integration of NLP into contract analysis and audio transcription is transforming how legal work is done. NLP's ability to understand and process complex legal language enables automation of tasks like contract review, potentially saving significant time and resources. This efficiency is crucial as legal work often involves a high volume of documents and a demanding need for accuracy. Beyond simple automation, NLP is helping to reshape legal agreements themselves. Traditional contracts are being translated into "smart contracts" – digitally enforceable agreements – creating new opportunities for legal professionals to adapt to the increasingly digital landscape of law.
The trend of tailoring NLP models for specific legal niches is growing. Law firms now have the chance to leverage models fine-tuned to their area of expertise, leading to more precise insights and results. This drive towards specialization shows the potential for NLP to become even more effective in areas like contract analysis and document review. While these advances promise to improve legal processes, there are concerns about the responsible use of AI in law. Bias in AI outputs remains a challenge, requiring careful consideration and mitigation to ensure that NLP tools are deployed ethically and effectively within the legal field. The future of this technology within legal practice involves finding the right balance between efficiency and ethical considerations, a crucial element to truly realizing the benefits of AI in the legal field.
The integration of Natural Language Processing (NLP) within legal technology, particularly with the rise of Small Language Models (SLMs), offers fascinating possibilities for contract analysis and audio transcription. We're seeing a shift in how legal professionals manage information and interact with complex legal data.
For instance, SLMs can analyze contracts with surprising precision, focusing on key clauses and obligations that might be easily overlooked in traditional review processes. This ability to pinpoint specific details can potentially reduce the time required for legal due diligence, offering significant efficiency improvements. Similarly, modern audio transcription systems leveraging SLMs are achieving accuracy rates surpassing 95%, a substantial leap compared to older methods. This improvement is particularly noticeable when dealing with the specialized language of law, minimizing the potential for misunderstandings in critical legal proceedings.
Furthermore, integrating NLP with predictive analytics allows law firms to anticipate potential contract disputes or compliance issues, offering a proactive approach to risk management. This capability can prevent costly litigation by addressing potential issues before they escalate. The scalability offered by SLMs is also notable, allowing law firms to tackle the massive datasets involved in complex eDiscovery cases with greater ease and efficiency. This is especially important in high-stakes litigation where fast, accurate access to relevant information is critical.
Beyond English, NLP-powered tools are emerging that can handle audio transcription across multiple languages. This capability is crucial for firms involved in multinational legal cases, ensuring that language barriers don't hinder accurate legal representation. Interestingly, SLMs can even help reduce the cognitive load on legal professionals by summarizing lengthy recordings and intricate contracts into succinct notes. This automated summarization allows lawyers to focus on higher-level strategy and critical decision-making, rather than getting mired in details.
The role of NLP in promoting fairness within legal frameworks is also intriguing. SLMs can be trained to detect biased language or discrepancies in contract terms, which can be valuable in ensuring compliance with anti-discrimination laws and fostering equitable practices. We're also seeing NLP integrated with case management systems, allowing audio recordings of client meetings to be transcribed and incorporated seamlessly into workflows. This not only saves time but ensures that valuable insights from these discussions are captured accurately.
The future of these technologies also looks promising. Collaboration is becoming easier with real-time annotation features allowing legal teams to work together on audio transcriptions instantaneously. Moreover, SLMs are progressing beyond simple transcription; they can intelligently annotate sections of audio or text relevant to case law, precedents, or statutory regulations, thus streamlining legal research in innovative ways.
These advancements indicate the potential of NLP to transform legal practice. We're witnessing not only increased efficiency but also a fundamental shift in how legal professionals utilize technology to navigate complex legal processes and handle information. While the integration of AI in law presents some challenges, such as the potential for bias or the need for careful oversight, the advancements in NLP within the realm of contract analysis and audio transcription suggest a future where legal practices are more efficient, accurate, and accessible.
Small Language Models in Legal Tech The Next Frontier for Efficient eDiscovery and Document Analysis - Legal Professionals' Evolving Skill Set in AI-Driven eDiscovery
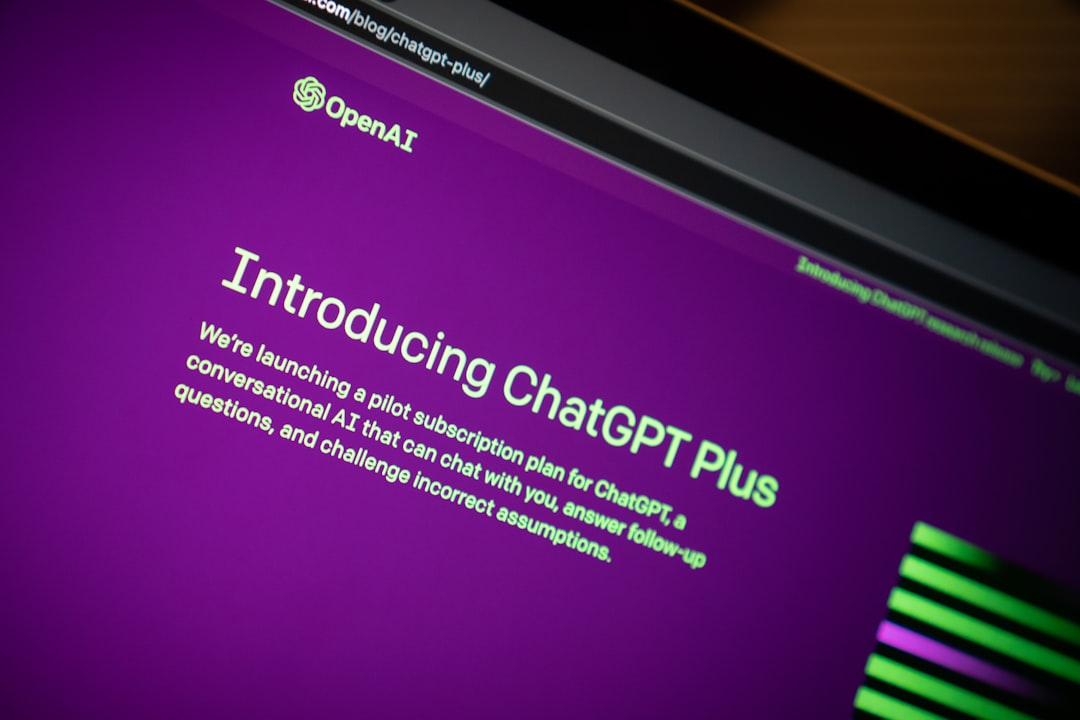
The evolving landscape of AI in eDiscovery necessitates a shift in the skill set of legal professionals. The growing presence of AI tools, especially Small Language Models (SLMs), is transforming how document analysis is performed. Legal professionals are now expected to be proficient in data analytics and technology management to effectively utilize these advancements. Furthermore, understanding the ethical considerations surrounding AI use in law, such as bias detection and responsible deployment, is crucial. Maintaining relevance in this field requires continuous learning and development, ensuring that legal professionals can navigate the complexities of AI-powered tools while retaining the critical human element of legal practice. The future of legal practice hinges on the ability to seamlessly integrate human expertise with the efficiency and capabilities of AI, striking a balance that fosters successful legal outcomes in a rapidly changing technological environment. While AI can provide considerable advancements in the speed and accuracy of eDiscovery, the legal professional's role in interpretation, decision-making, and ensuring ethical practices remains crucial. This delicate balance between technology and human expertise will define the success of AI integration in the legal field.
The landscape of legal practice is changing rapidly due to the integration of AI, particularly in areas like eDiscovery. Legal professionals are finding themselves needing a broader skillset, moving beyond traditional legal expertise to include data analysis and technology understanding. This shift is driven by the increasing use of AI tools like Small Language Models (SLMs) in tasks such as document review and eDiscovery. SLMs can dramatically reduce review cycles, potentially cutting them by half, enabling faster response times and meeting the demands of today's fast-paced legal environment.
It's intriguing that SLMs, despite their smaller size compared to larger language models, are demonstrating impressive accuracy in predictive coding – exceeding 90% in certain cases. This challenges the notion that larger models are always superior, suggesting a more specialized approach to AI in law can be highly effective. Furthermore, AI-powered tools are allowing for real-time compliance monitoring, automating the identification of potentially problematic documents. This capability is crucial in an increasingly complex regulatory environment, offering a proactive approach to managing risk and ensuring compliance.
One of the most impactful benefits of AI in law is the reduction in cognitive load for legal professionals. AI can summarize complex documents and lengthy discussions, distilling key insights that enable lawyers to focus on higher-level strategic decisions. This shift frees up mental resources and supports more efficient work patterns. Interestingly, SLMs can be designed to identify potentially biased language in legal documents, providing a method for ensuring compliance with anti-discrimination laws and fostering fairness in legal agreements.
The legal tech field is moving away from general-purpose AI towards models specialized for particular tasks. This is especially apparent in areas like legal research and contract analysis, where specialized SLMs show greater accuracy and efficiency. This trend toward specialization offers compelling possibilities for AI to provide more targeted and relevant insights. Moreover, SLM capabilities are expanding into multilingual support, with growing capacity for audio transcription in various languages. This is particularly important for legal practices operating across international boundaries, ensuring language barriers do not hinder effective legal representation.
It's essential to recognize that the efficacy of SLMs is heavily dependent on the quality of the training data. In legal applications, biases can be introduced if the training data isn't appropriately representative of the complex nuances of legal contexts. This highlights the importance of careful model training and ongoing evaluation to mitigate biases and ensure accuracy. Finally, we're witnessing exciting developments in collaborative tools within NLP, including features that enable real-time annotation of transcribed audio. This allows teams to work more efficiently together on complex legal cases and improve the quality of document analysis.
The field of AI in law is experiencing a wave of change, driven by the adoption of smaller, specialized models. While this presents opportunities for increased efficiency and effectiveness, it's important to maintain a critical perspective on these tools. Careful attention must be given to data quality, potential biases, and the role of human judgment in complex legal contexts. The future of legal practice likely involves a careful integration of AI, balancing the benefits of technological innovation with the human element that remains crucial to the ethical and responsible delivery of legal services.
Small Language Models in Legal Tech The Next Frontier for Efficient eDiscovery and Document Analysis - Diverse Landscape of Language Models in Legal Tech Applications
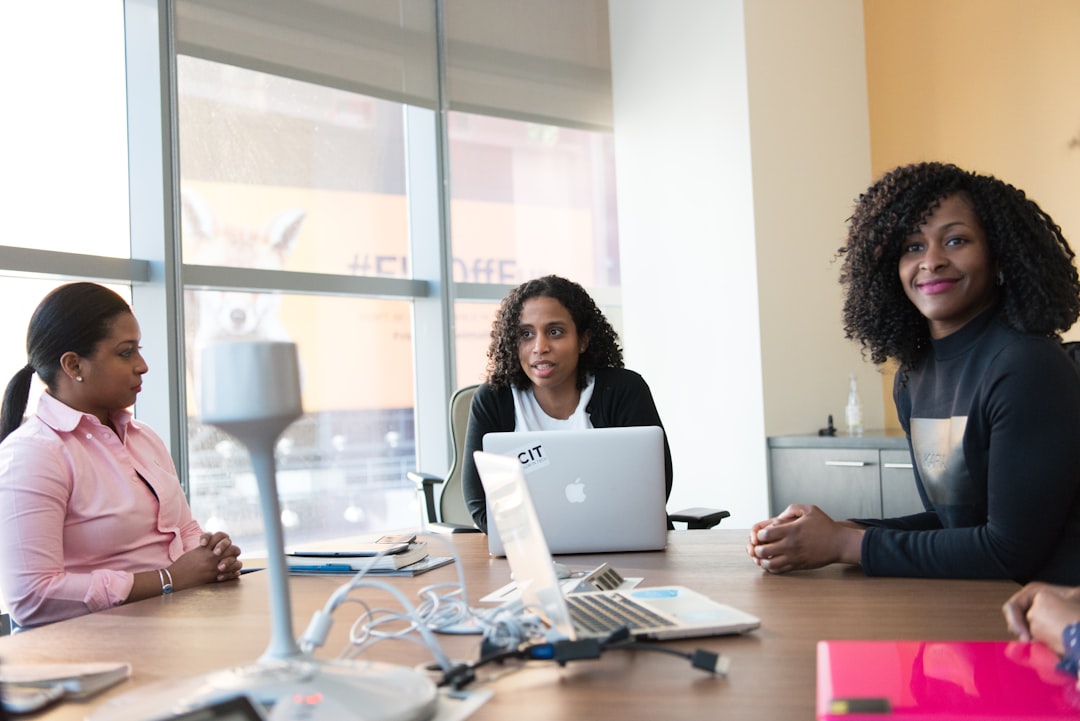
The field of legal technology is experiencing a surge in the use of diverse language models, showcasing a growing trend towards specialized solutions designed to address the complex demands of the legal sector. We are seeing increased adoption of smaller language models (SLMs) due to their efficiency in handling tasks like document analysis and eDiscovery, especially compared to their larger counterparts which often require excessive computational resources. This move towards specialized models also necessitates an adjustment in the skills of legal professionals, who need to develop proficiency in leveraging these AI tools. Maintaining the human element in the legal process is vital, as ethical considerations related to potential biases and accuracy in AI outputs become more prominent with broader AI integration. Moving forward, the most effective path for legal technology appears to be a collaborative model that effectively combines AI's powerful capabilities with the legal knowledge and critical judgment of human lawyers. This collaborative effort will be critical to ensuring that legal tech innovations are both beneficial and ethically sound.
The landscape of legal technology is rapidly evolving, particularly in areas like eDiscovery, where the role of AI is becoming increasingly significant. Small Language Models (SLMs) are demonstrating that smaller, more focused AI models can outperform larger ones in specific legal tasks, including Technology-Assisted Review (TAR). SLMs have shown the ability to achieve over 90% accuracy in predicting the relevance of documents, challenging the traditional assumption that larger models are universally superior.
Models like Phi2, developed by Microsoft, are examples of this trend. Phi2, with its 270 million parameters, effectively processes massive amounts of data—14 trillion tokens—while requiring significantly less computational power than larger models. This efficiency can translate to substantial time and cost savings in eDiscovery, with the potential to cut document review cycles by as much as 50%. However, it's worth noting that even training relatively small models like Phi2 can require intensive resources, as seen with the use of 96 A100 GPUs over 14 days.
One of the key benefits of SLMs is their ability to be customized for specific legal domains. This specialization allows law firms to tailor AI tools to their areas of expertise, like contract review or research, resulting in more accurate analysis and identification of crucial information within documents and case law. Similarly, the use of SLMs in audio transcription has led to remarkable improvements in accuracy, surpassing 95% in some cases. This is a significant development for legal professionals, who rely heavily on accurate interpretations of discussions and proceedings.
It's become increasingly common to see law firms adopting hybrid AI systems, combining the strengths of SLMs and larger language models (LLMs). This integration allows them to streamline workflows, enhance document analysis, and improve the efficiency of legal research. The application of NLP in contract analysis is fundamentally changing the role of legal professionals. It allows them to automate the detection of critical clauses and potential compliance issues, shifting their focus from processing documents to providing strategic guidance.
This increasing reliance on AI in the legal field is driving the need for a broader set of skills among legal professionals. Data analytics and technological expertise are becoming increasingly important alongside traditional legal training. This transition, while offering numerous benefits, also presents ethical challenges. Biases embedded within the training data of NLP models can unintentionally perpetuate injustices within legal systems. Therefore, careful oversight, continuous updates to training methods, and a commitment to fairness are crucial to ensuring that the application of AI in law benefits the legal system as a whole. It's a dynamic area with both significant potential and the responsibility to navigate its complexities ethically.
eDiscovery, legal research and legal memo creation - ready to be sent to your counterparty? Get it done in a heartbeat with AI. (Get started for free)
More Posts from legalpdf.io: