How AI-Powered Document Analysis Uncovered Key Evidence Patterns in Hengle v
How AI-Powered Document Analysis Uncovered Key Evidence Patterns in Hengle v - Machine Learning Models Detect Financial Fraud Patterns in Hengle Discovery Data
Within the evolving field of legal technology, machine learning models have proven invaluable in the identification of financial fraud, especially when applied to the Hengle discovery data. These advanced algorithms uncover intricate patterns associated with the components of the Fraud Pentagon, delving into the subtleties of financial transactions. Traditional, rule-based fraud detection systems frequently struggle to keep pace with ever-changing fraud schemes. In contrast, deep learning models demonstrate a greater aptitude for handling extensive datasets and adapting to new and evolving fraud patterns, making them more capable of identifying fraudulent activity. This has clear implications for e-discovery and legal research, potentially impacting the quality and scope of legal investigations. However, it's imperative to approach the use of these AI tools with a balanced perspective. It's crucial to acknowledge their limitations, particularly when navigating challenges associated with limited historical data of actual fraudulent events, ensuring that these advancements contribute to the overall integrity of the legal system.
Machine learning models are increasingly being used to identify patterns of financial fraud within the complex data unearthed during e-discovery. These models can process massive datasets of financial transactions much faster than human reviewers, helping to pinpoint potentially fraudulent activities in a fraction of the time. While traditional fraud detection methods rely on predefined rules, they struggle to keep pace with the ever-evolving tactics of fraudsters. This is where machine learning excels; its algorithms can dynamically learn and adapt to new patterns, identifying subtle relationships within the data that might evade human detection. This ongoing learning process enhances the models' ability to recognize and flag suspicious activity with increasing accuracy.
The capability of machine learning to analyze large volumes of data efficiently has significant implications for legal discovery, particularly in complex cases involving extensive financial records. However, as with any powerful tool, there are limitations. The challenge lies in the scarcity of readily available examples of fraud within historical data, making it more difficult for AI models to learn and accurately identify new fraudulent patterns. Additionally, there's a constant need to balance the speed and efficiency provided by AI with the necessity of human oversight and legal expertise to ensure the integrity and fairness of the process. This delicate balance is crucial, particularly as the use of AI in legal decision-making expands.
The implementation of AI in the legal sphere raises important ethical considerations. As AI models become more sophisticated, we must remain mindful of potential biases within the datasets they are trained on. It's crucial to develop robust methods for evaluating and mitigating any potential biases in order to maintain the fairness and impartiality of the legal process. There's a need for further research and development in this area to ensure that these powerful tools are used responsibly and effectively. Ultimately, the goal is to leverage AI to enhance efficiency and transparency in legal proceedings, while upholding fundamental principles of justice and fairness.
How AI-Powered Document Analysis Uncovered Key Evidence Patterns in Hengle v - AI Document Classification System Maps Violation Clusters Across 50,000 Case Files
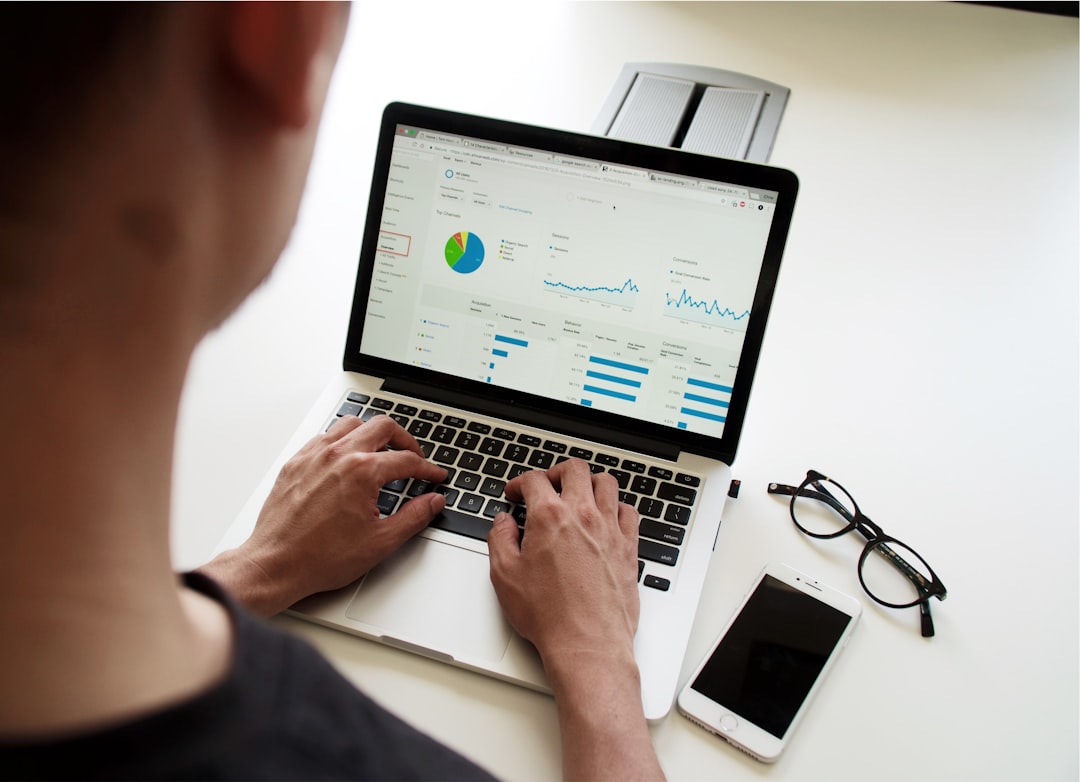
In the realm of legal practice, AI-powered document analysis tools are increasingly demonstrating their value, especially within the context of e-discovery. One notable example involves an AI document classification system that effectively categorized 50,000 case files, identifying recurring patterns of violations within the data. This system, applied to the Hengle case, successfully highlighted significant evidence patterns buried within a massive document pool. This ability to quickly and accurately categorize and analyze vast quantities of documents accelerates the process of e-discovery and legal research, making it more efficient and thorough.
The use of AI in this context can streamline legal workflows and potentially lead to faster and more accurate case analysis. However, it's crucial to recognize that these advanced technologies are not without their limitations. AI models rely on the data they're trained on, and biases present in that data can influence their results. Therefore, careful consideration and human oversight are needed to ensure the integrity and fairness of the process. Ultimately, the integration of AI in the legal profession holds significant promise for enhancing the overall practice of law, but the responsible implementation and continuous evaluation of these tools are crucial for realizing their full potential.
AI systems are increasingly being used in legal contexts to process and analyze large volumes of documents. In one recent application, an AI document classification system analyzed a dataset of 50,000 case files, successfully identifying clusters of violations. This demonstrates the potential of AI to uncover hidden patterns within complex legal data.
This particular AI system, which can be viewed as a type of Document AI, leverages advancements in deep learning and machine learning models to categorize documents, a task that would take legal teams significantly longer using traditional methods. This ability to sift through thousands of documents quickly helps streamline the process of discovery and e-discovery. The system uses a combination of optical character recognition (OCR) and natural language processing (NLP) techniques to understand the content of documents and can even be enhanced by incorporating generative AI, further refining the speed and precision of classification and data extraction from the raw, unstructured documents.
While the technology offers significant benefits in terms of speed and efficiency, it's important to understand how this system works in order to assess the reliability and overall utility. The classification model at the heart of the system is essentially a complex algorithm that assigns categories to documents based on features learned from training data. A key step in this process is to establish a pipeline that not only classifies but clusters related documents, a process that in this instance reveals clusters of violations. This type of clustering is a newer technique that is now finding relevance in legal investigations. Additionally, the system incorporates a process for verifying the confidence level of classifications, offering a safeguard against erroneous categorizations.
While the system holds immense promise for improving efficiency and potentially leading to faster resolution of legal disputes, it also presents challenges. One such challenge is the potential for bias in the data used to train the AI model. If the data reflects existing societal biases, the AI system may perpetuate those biases, leading to unfair or inaccurate conclusions. Another challenge is the need to ensure transparency and interpretability in AI decision-making. In legal proceedings, understanding the rationale behind AI-generated conclusions is vital for maintaining confidence in the system and ensuring that it doesn't inadvertently introduce unfairness into the legal process. It's crucial to strike a balance between maximizing the benefits of AI-driven analysis while mitigating potential risks.
Platforms such as LexisNexis are already beginning to incorporate AI capabilities to analyze and process legal documents, reflecting a broader shift in the legal sector towards integrating AI tools for more efficient and effective case management. It's fascinating to see how AI is transforming this field, particularly in the context of legal discovery. The ability to map complex relationships between documents and reveal hidden patterns has the potential to reshape how we approach legal research and, ultimately, how justice is served.
How AI-Powered Document Analysis Uncovered Key Evidence Patterns in Hengle v - Natural Language Processing Reveals Communication Networks Between Key Defendants
In the evolving landscape of legal practice, Natural Language Processing (NLP) is proving invaluable in revealing hidden communication patterns within complex legal cases. This AI-powered technique meticulously analyzes vast quantities of text, unearthing the intricate relationships and networks among key defendants. By discerning subtle connections within legal documents, NLP can illuminate crucial aspects of a case that may be obscured from human analysts. This capability is particularly relevant in cases involving financial fraud or complex corporate structures, where understanding the flow of communication is key to uncovering illicit activities.
While the ability of NLP to expedite legal research and analysis is undeniable, we must acknowledge potential downsides. The algorithms underlying NLP are trained on existing datasets, which may carry inherent biases. This necessitates a careful approach, incorporating human oversight and critical evaluation to mitigate the risk of skewed outcomes. Striking a balance between leveraging AI's speed and analytical prowess while maintaining the integrity and fairness of legal proceedings is crucial as NLP and other AI-driven tools gain wider acceptance within the field of law. The future of legal research and the application of justice may be profoundly influenced by the careful and responsible integration of AI, like NLP, into the discovery process.
Natural language processing (NLP) is becoming a crucial tool in legal analysis, particularly in uncovering hidden connections within complex communication data. AI algorithms can now map out intricate networks of communication among defendants, a task that would be extremely time-consuming and difficult for humans to accomplish. This ability to visualize how frequently and in what context key figures interacted can be pivotal, surfacing relationships that might otherwise go unnoticed and potentially influencing legal strategies.
One of the major advantages of AI-powered document review is the ability to rapidly process massive quantities of communication data, like emails and messages. This is a boon in cases where the sheer volume of documents can be overwhelming. Instead of wading through mountains of data, legal teams can prioritize evidence based on AI's assessment of importance, making the e-discovery and subsequent analysis much more efficient. However, this efficiency relies on the assumption that the training data used by the NLP models is unbiased and accurately reflects the reality of communications.
Beyond mere volume, NLP can identify subtle patterns and changes in communication that humans might miss. These could include shifts in language, the frequency of communication around particular events, and other indicators that can provide unique insights into a case. The potential for these insights to shape legal arguments and potentially influence outcomes is substantial. However, it's important to remember that AI's understanding is dependent on the data it's trained on. Biases in the underlying communication data could lead to skewed insights, highlighting the need for careful oversight and validation when relying on these powerful tools.
The integration of NLP with e-discovery platforms is accelerating the evolution of legal workflows. This isn't just about speeding up the document review process; it's about allowing legal teams to gain a deeper understanding of the contextual relationships within documents. NLP can help pinpoint evidence that's relevant to a specific legal argument much faster and more effectively than traditional methods. It's worth noting that this application of AI is helping to reimagine how legal strategies are crafted. Previously, legal arguments relied heavily on manually reviewing and synthesizing evidence. Now, AI provides a unique lens into the tapestry of communications, which could lead to more focused and potentially more successful litigation tactics.
It's crucial to recognize that while NLP tools can provide remarkable insights, they should not be viewed as a replacement for human judgment. Legal professionals still play a critical role in interpreting the results and understanding them within the larger context of legal principles and precedent. AI is a tool that can augment human capabilities, but it can't entirely replace the nuances of legal reasoning and experience. Additionally, AI can now be applied in real time as new information comes to light during litigation, enabling strategies to adapt quickly to evolving circumstances. This dynamic application of AI in a legal context is a significant shift from the traditional slower-paced methods of document review.
Looking ahead, the role of AI in legal research will likely continue to expand. We're likely to see more AI tools that leverage historical patterns of communication, coupled with past legal precedents and outcomes, to offer predictive insights into cases. This might seem like a radical shift in the way we think about legal research, and the ramifications of potentially using AI to forecast the outcome of a case are considerable and still under discussion. As the technology matures and our understanding of its capabilities expands, we will need to carefully evaluate both the benefits and potential risks to the fairness and integrity of the legal system.
How AI-Powered Document Analysis Uncovered Key Evidence Patterns in Hengle v - Automated Timeline Analysis Identifies Critical Evidence Sequence in Lending Practices
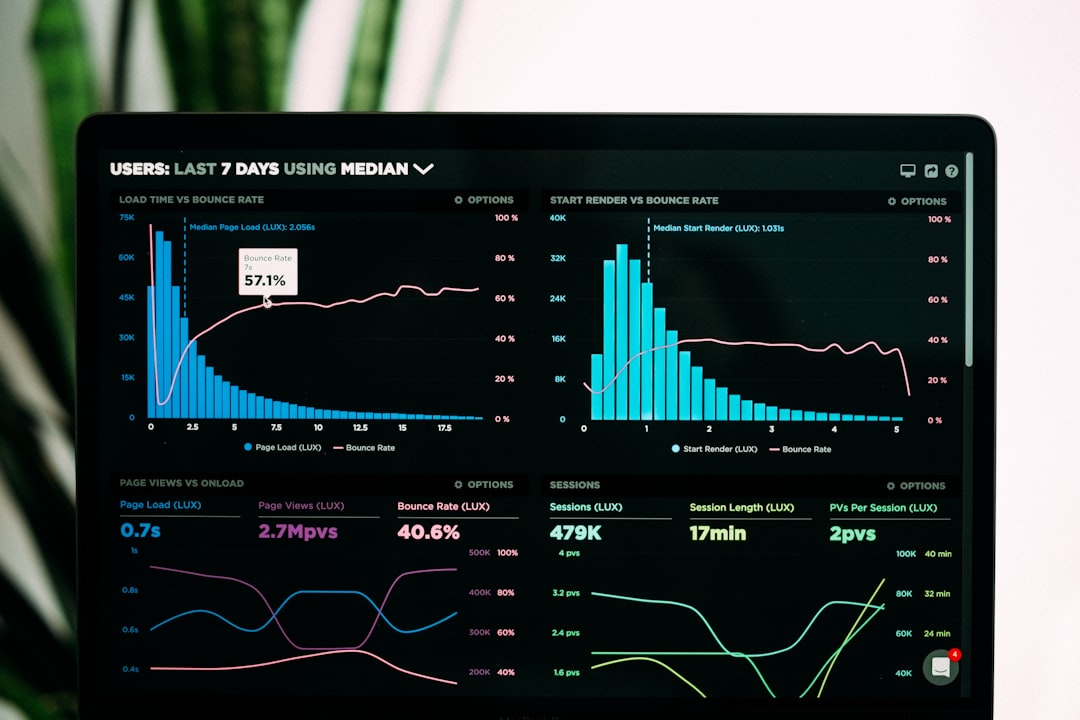
Automated timeline analysis is becoming increasingly valuable in legal contexts, particularly when investigating financial matters and digital forensics. AI-powered tools are able to sift through massive quantities of data, including timestamps from various digital sources, reconstructing the order of events and helping identify potential irregularities. This can be incredibly useful in lending practice cases, where identifying a sequence of events related to fraudulent activity is crucial. The ability to generate timelines automatically helps streamline investigations and builds stronger, more coherent evidence for legal proceedings.
While the potential for enhancing efficiency in legal research and e-discovery through AI is exciting, there are important considerations. AI models are only as good as the data they are trained on, which can introduce biases or blind spots. A critical aspect is to find the right balance – using automated timeline analysis to optimize efficiency, while simultaneously ensuring human oversight and expert review to guarantee fairness and integrity in the legal process. As with any technology that automates aspects of legal analysis, we need to be mindful of potential biases and ethical implications in order to safeguard the impartiality of the legal system.
Automated timeline analysis, especially in the context of legal cases, is increasingly showing its value. AI-powered systems can sift through massive volumes of digital evidence, like emails, documents, and transaction records, far more quickly than humans. This allows for the rapid creation of detailed timelines, which can then be analyzed for key patterns and sequences of events that are crucial to understanding the context of a case. Traditional approaches often struggle to cope with the sheer volume of digital data involved in modern legal cases, leading to time-consuming and potentially incomplete investigations.
However, while AI speeds up the identification of relevant evidence, it is not without its issues. For instance, AI systems can be prone to biases, particularly if trained on datasets that reflect pre-existing prejudices or skewed views. It's vital for legal professionals to ensure that the underlying data used to train AI models is as unbiased as possible. Moreover, the interpretation of the resulting timelines must be coupled with human legal expertise. While AI can highlight potential anomalies and unusual sequences, the task of determining the legal implications of these findings requires the analytical skills and knowledge of lawyers and legal researchers.
It's fascinating how AI can help reveal previously hidden connections between seemingly unrelated events. For example, in a complex fraud case, AI might be able to link seemingly isolated transactions that collectively point to a larger pattern of illicit activity. Similarly, in corporate disputes, the analysis of emails and documents could reveal communication sequences that suggest breaches of confidentiality or hidden agendas. These insights would be nearly impossible for humans to extract from large, unstructured datasets.
Furthermore, AI tools can assist with pattern recognition that extends beyond merely building a timeline. By recognizing recurring patterns within the timeline itself or other evidence, AI can start to form hypotheses that might help legal professionals develop more effective strategies. For example, a pattern of suspiciously timed transfers between accounts might lead a lawyer to dig deeper, which might never have been noticed in a traditional manual review.
One potential future development in this area is the integration of these tools with generative AI. This could lead to a system where AI could automatically create summaries of a timeline and flag significant deviations, ultimately speeding up the process even further. However, this comes with its own set of concerns, as this can quickly veer into problematic territory if proper guidelines and oversight are not in place. It will be essential to address issues of accuracy and trustworthiness of generative outputs if such approaches are to be employed in the legal context.
In general, the legal field, like many other sectors, is undergoing a transformation driven by advancements in AI. The use of automated tools for timeline analysis is just one illustration of how AI is impacting traditional legal processes. As we continue to see rapid improvements in AI capabilities, it's crucial that we think critically about how these tools are being used, to ensure that the legal system remains transparent, impartial, and just in this new era.
How AI-Powered Document Analysis Uncovered Key Evidence Patterns in Hengle v - Deep Learning Algorithms Track Digital Evidence Trail Through Multiple Jurisdictions
Deep learning algorithms are transforming the way legal professionals handle digital evidence that stretches across different jurisdictions. These algorithms excel at analyzing extensive datasets, uncovering connections and patterns within digital evidence that might cross geographical borders. This enhances the efficiency of investigations that involve multiple jurisdictions. However, questions about the reliability and accuracy of evidence produced by AI continue to surface, underscoring the need for human oversight to safeguard the integrity of the legal process. This integration of AI in electronic discovery compels a critical look at ethical considerations, including the possibility of biases present in the training data, which could influence legal decisions. The legal technology landscape is constantly changing, and the balance between harnessing the benefits of deep learning and applying careful scrutiny is crucial to uphold justice in our increasingly digital world.
In the evolving landscape of legal technology, deep learning algorithms are playing an increasingly significant role, particularly in the realm of digital evidence analysis and e-discovery. These algorithms offer the potential to analyze massive datasets with unprecedented speed and accuracy, potentially revolutionizing legal practices across multiple jurisdictions.
One of the key advantages of deep learning in this context is its ability to track digital evidence across borders. In cases of fraud or other crimes that involve multiple legal territories, deep learning models can sift through data from various sources and jurisdictions, making it easier to identify intricate fraud patterns. This capability overcomes limitations associated with traditional methods and provides a more holistic view of complex situations.
Another significant implication lies in the streamlining of e-discovery. Traditional e-discovery methods often involve lengthy and labor-intensive manual review processes. Deep learning models, on the other hand, can process millions of documents at a time, greatly accelerating the review and analysis stages. This can shorten discovery timelines from months to potentially just a few weeks, enabling law firms to deliver faster and more efficient results.
Deep learning models have a knack for recognizing complex patterns that might escape even the most experienced human analysts or conventional algorithms. They can, for example, uncover hidden connections within digital communications or financial transactions that might indicate fraudulent activity or otherwise be difficult to discern. This level of intricate pattern recognition holds immense potential for legal investigations.
Moreover, the application of deep learning has enabled advancements in automated data classification. Document AI systems leveraging natural language processing (NLP) can quickly categorize legal documents, assisting with the prioritization of crucial evidence and overall case management efficiency. This kind of automation streamlines the processing of massive document datasets and allows legal professionals to focus on the most critical aspects of a case.
Furthermore, deep learning can uncover biases in data, which is particularly useful in cases involving potential discrimination. By analyzing patterns in transactions or other data, deep learning algorithms can identify systematic biases embedded within lending practices or other business processes. This capability can help legal teams highlight potential areas of concern in a more precise and impactful way.
We also see increasing adoption of AI technologies by larger law firms, extending beyond document review and into the realm of predictive analytics. Leveraging historical data patterns and legal precedent, AI can assist in predicting the likely outcomes of various legal strategies, enabling firms to potentially refine their litigation tactics in more targeted and informed ways.
Deep learning algorithms enable real-time analysis during legal proceedings, which represents a significant departure from conventional static approaches. Legal teams can adapt their strategies as new evidence emerges, benefiting from immediate insights and responses. This dynamic capability provides a significant advantage in complex cases where evidence can shift rapidly.
However, the increasing use of AI in evidence gathering has led to critical discussions about ethical implications. Questions of algorithm transparency and bias in datasets used to train AI models are pivotal considerations for maintaining the integrity of legal proceedings. Ensuring the fairness and reliability of AI-driven evidence remains a challenge and requires thoughtful discussion.
Beyond litigation, AI can also play a role in automated compliance monitoring. AI-powered systems can be utilized to detect potential legal issues in real-time, aiding in the prevention of compliance violations before they escalate into more serious problems. This proactive approach to compliance can be beneficial for companies seeking to minimize legal risks.
Finally, AI is reshaping traditional legal research processes. The ability to quickly analyze vast amounts of jurisprudence and statutes through AI can inform legal strategies and provide lawyers with insights that would take significant time and effort to discover through traditional methods. This enhancement to legal research is likely to continue to evolve, changing how legal strategies are developed in the years ahead.
In conclusion, while the use of deep learning in the legal field offers tremendous advantages, it's essential to consider both the potential benefits and the ethical considerations carefully. As AI continues to develop and become increasingly integrated into the legal system, striking a balance between innovation and ethical considerations will be crucial for maintaining the integrity and fairness of the justice system.
How AI-Powered Document Analysis Uncovered Key Evidence Patterns in Hengle v - Pattern Recognition Software Uncovers Previously Hidden Links in Settlement Documents
AI-powered pattern recognition software is reshaping legal document analysis, particularly within the context of settlement agreements. These sophisticated programs, employing machine learning, can identify subtle links and evidence patterns previously hidden within complex documents. This ability to uncover connections often missed by human review significantly enhances the effectiveness of e-discovery and legal research. Cases such as Hengle v. serve as examples of how such technologies can revolutionize the process of uncovering critical information, improving the speed and comprehensiveness of investigations.
However, the use of AI in legal contexts isn't without its caveats. Ensuring the reliability and accuracy of AI-generated insights remains paramount. Furthermore, the inherent risk of bias in the data used to train these algorithms demands careful oversight to prevent the perpetuation of unfairness in the legal process. As AI continues to mature and become more integrated within legal practices, a delicate balance needs to be struck: maximizing the efficiency of AI-driven tools while safeguarding the principles of fairness and impartiality inherent to the legal system. The future of law will likely be influenced profoundly by these advancements, requiring continuous evaluation and thoughtful integration to ensure justice remains at the forefront.
AI-driven document analysis has proven increasingly valuable for legal professionals, particularly in accelerating the pace of discovery and revealing hidden connections within large datasets. For instance, AI can rapidly process and categorize thousands of documents in a matter of hours, substantially shortening the timeline of e-discovery processes. This speed advantage stems from sophisticated algorithms that can identify complex patterns and relationships within the data, unveiling connections that may evade manual review. This is particularly useful for cases involving multiple jurisdictions, where AI can track digital evidence across geographical boundaries and aid in developing cohesive legal strategies.
Furthermore, AI is being used to identify biases embedded in datasets, a crucial feature in cases involving discrimination claims. It allows for a more precise understanding of systemic issues within data, potentially leading to fairer outcomes. Automated timeline analysis is another application where AI shines, generating comprehensive timelines from large data volumes that can assist with clarifying the sequence of events related to a case, especially in the complex world of financial fraud.
Natural Language Processing (NLP) is playing a key role in uncovering hidden communication networks and relationships among individuals in legal cases, offering unique insights that can influence litigation strategies. While the promise of generative AI to summarize and suggest subsequent actions in legal analysis is compelling, there are concerns surrounding the reliability and accuracy of its outputs, especially in legally consequential contexts.
However, despite the efficiency gains provided by AI, human oversight remains essential. Legal professionals need to critically assess and interpret AI-generated insights within the larger context of established legal principles to maintain fairness in legal processes. The ability of AI to analyze evidence in real-time as new information emerges during litigation provides a significant advantage over traditional methods. There is also growing interest in applying AI to predictive analytics, utilizing historical data and legal precedents to forecast likely legal outcomes, allowing law firms to strategize with greater precision and potentially enhance their success rates in litigation.
While AI-powered document analysis presents several benefits, we must acknowledge the potential for unintended biases in the datasets used for AI training. Careful consideration and human validation are crucial to mitigate these risks. The legal field continues to grapple with the ethical implications of AI, specifically related to the reliability and transparency of evidence generated by these systems. Striking a balance between utilizing the strengths of AI and safeguarding the integrity of the legal system is a vital challenge that requires continuous discussion and refinement.
More Posts from legalpdf.io: