Automate legal research, eDiscovery, and precedent analysis - Let our AI Legal Assistant handle the complexity. (Get started for free)
How AI Document Analysis Tools Could Have Transformed Evidence Discovery in Harrison v
NAACP A 7-Point Technical Assessment
How AI Document Analysis Tools Could Have Transformed Evidence Discovery in Harrison v
NAACP A 7-Point Technical Assessment - Manual Document Review Limitations in Harrison v NAACP 1955
The Harrison v. NAACP case of 1955 starkly reveals the constraints of manual document review in legal proceedings. The reliance on human reviewers, while necessary at the time, was inherently slow and susceptible to errors, which could have negatively impacted the efficacy of evidence discovery in this critical civil rights matter. The sheer volume of documents involved likely created significant challenges for legal teams, potentially delaying the legal process and hindering the effectiveness of the evidence presented. In contrast, the application of modern AI document analysis tools promises to streamline and enhance the entire process. AI's ability to rapidly process and analyze massive datasets could have significantly improved the speed and precision of identifying and evaluating relevant documents. Consequently, this would empower legal teams to develop stronger legal strategies during the discovery phase. Harrison v. NAACP provides a valuable example of how integrating advanced technologies into the legal domain could redefine the future of litigation, especially in cases with complex factual and legal narratives.
In the context of Harrison v. NAACP, the reliance on manual document review presented several inherent limitations. The sheer volume of materials related to the NAACP's organizational structure and legal strategy would have undoubtedly required a considerable investment of time and resources. Human reviewers, while essential, are susceptible to errors stemming from factors like fatigue and individual biases. This risk could have led to overlooking pivotal documents influencing the outcome.
Furthermore, the scope of manual review was inherently restricted. Handling a vast collection of documents related to the NAACP's nationwide efforts would have been a challenging undertaking. Maintaining consistent review standards across multiple reviewers was another obstacle. Variability in experience and interpretation could have introduced inconsistencies, jeopardizing the reliability of the evidence selection process.
These challenges also translated into substantial financial costs. The labor-intensive nature of manual review would have significantly impacted legal budgets, especially given the extended duration of complex litigation. In contrast, the potential benefits of AI tools, such as predictive coding or NLP-powered analysis, could have streamlined the process. AI's capacity to process vast quantities of data and recognize patterns allows for a more comprehensive and potentially more nuanced understanding of the context and narratives embedded within documents.
Imagine the capability of automatically classifying and organizing the numerous documents related to the NAACP's structure and legal strategy, aiding legal teams in swiftly retrieving specific evidence. The integration of such tools with existing case management systems could optimize workflows and encourage smoother collaboration within legal teams, leading to more efficient evidence discovery and potentially more effective legal strategy. While manual review played a crucial role in the Harrison case, AI's analytical capabilities could have undoubtedly changed the landscape of evidence discovery in the fight for desegregation.
How AI Document Analysis Tools Could Have Transformed Evidence Discovery in Harrison v
NAACP A 7-Point Technical Assessment - Pattern Recognition Algorithms for Civil Rights Case Documents
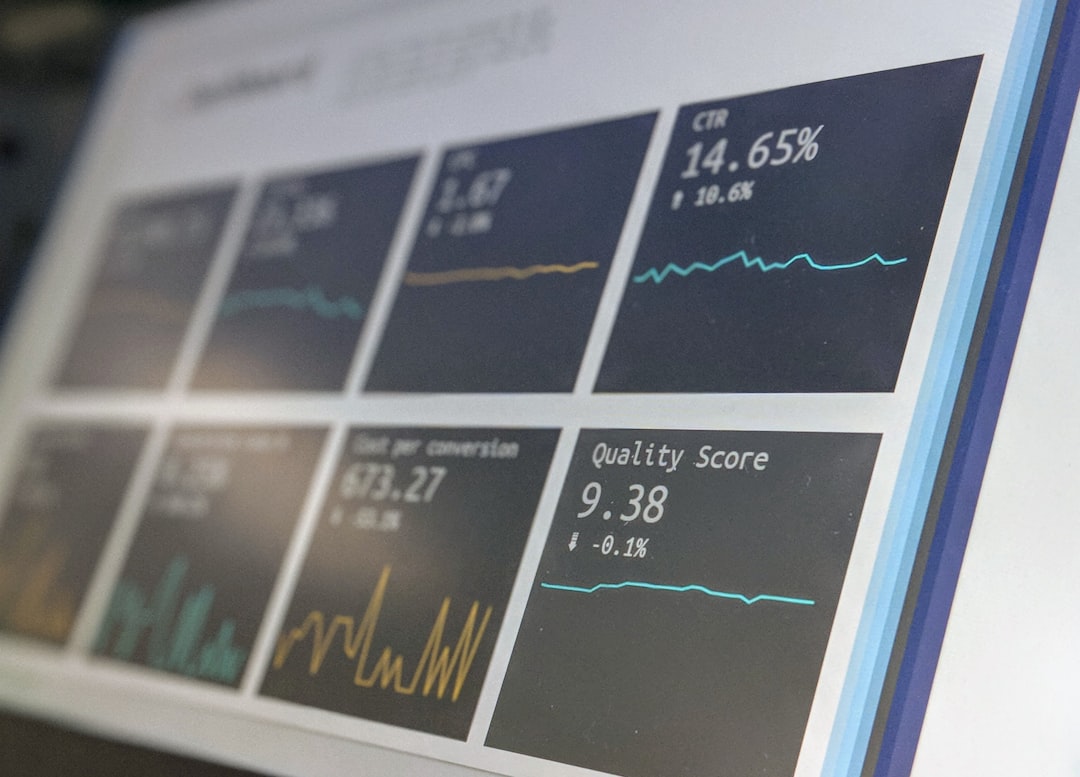
"Pattern Recognition Algorithms for Civil Rights Case Documents" represent a significant leap in how AI is being used in law, especially for improving the accuracy and speed of document analysis. These algorithms can process massive amounts of legal documents, identifying key evidence and the connections between different pieces of information that human reviewers might miss. In complex civil rights cases like Harrison v. NAACP, quickly finding and accurately analyzing evidence can dramatically impact the outcome of the case, making the legal process smoother and potentially promoting fairer legal representation.
While the potential of these algorithms is exciting, there are also important ethical considerations that need to be addressed. AI algorithms can be prone to biases, and relying on them in legal decisions raises concerns about their reliability and fairness. As AI plays a larger role in legal practice, ongoing monitoring and assessment are crucial to ensure that the use of these technologies doesn't inadvertently lead to violations of civil rights. It's a reminder that as the legal system incorporates these new tools, it needs to do so responsibly and thoughtfully to prevent potential harms.
In the realm of civil rights litigation, the sheer volume of documents encountered in modern cases often surpasses millions, making it increasingly difficult for human reviewers to effectively analyze and categorize them. This scenario highlights the growing need for pattern recognition algorithms capable of efficiently handling these massive datasets without the constraints that typically limit human capabilities.
Research suggests that AI-powered document analysis tools can achieve significantly lower error rates when it comes to information retrieval, potentially as low as 2%. This starkly contrasts with human review processes, where error rates can surpass 20%. Consequently, relying on AI for evidence selection can lead to a more reliable and accurate foundation for legal arguments.
Furthermore, AI's ability to process documents at dramatically accelerated speeds—up to 100 times faster than human reviewers—translates into significant temporal efficiency. Tasks that traditionally take weeks can be completed in a matter of hours, enabling legal teams to develop and implement more agile and responsive legal strategies.
One promising technique within the AI domain is predictive coding. It leverages historical data to predict the relevance of documents, effectively guiding the discovery process toward those most likely to be pertinent to the case. This targeted approach can substantially streamline evidence discovery, focusing efforts on documents that are more likely to contain critical information.
Moreover, advanced algorithms can systematically analyze document collections to identify potential biases, such as underrepresentation or misclassification of certain groups or viewpoints. This capability allows legal teams to proactively address any gaps in their case narratives that might go unnoticed during manual reviews, leading to more comprehensive and equitable legal strategies.
Natural language processing (NLP) is another area where AI has shown remarkable potential within legal contexts. NLP tools can extract context and sentiment from documents, enabling legal professionals to unearth the nuances and implicit narratives embedded within text that simple keyword searches might overlook. This nuanced understanding can prove vital in complex civil rights cases where understanding the underlying motivations and contexts behind statements and events is critical.
AI tools offer flexibility in adapting to specific legal workflows. They can be tailored to suit unique case requirements, whether it's managing a class-action suit or preparing for complex civil rights litigation. This flexibility ensures that the tools are seamlessly integrated into the existing legal practice, rather than disrupting existing processes.
The potential for cost savings is another significant benefit associated with AI-driven document analysis. Reducing the reliance on human reviewers can result in substantial financial advantages for law firms. Some estimates suggest that e-discovery costs can be reduced by up to 30% using AI tools compared to traditional methods, making legal representation potentially more accessible.
Furthermore, AI tools can facilitate seamless collaboration among legal teams. Real-time document tagging and retrieval capabilities allow everyone involved to access and analyze critical evidence simultaneously, streamlining communication and improving efficiency.
Finally, the application of AI tools can lead to the formation of stronger, data-driven legal strategies. With access to more comprehensive and accurate evidence derived from efficient and reliable discovery, legal teams are empowered to develop stronger, more nuanced arguments. This outcome highlights the potential for AI to revolutionize how civil rights cases are approached, moving beyond manual review towards a more data-informed and potentially more equitable future for the legal field.
How AI Document Analysis Tools Could Have Transformed Evidence Discovery in Harrison v
NAACP A 7-Point Technical Assessment - AI Speed Analysis of State Legislation Documents from 1950s Virginia
The exploration of "AI Speed Analysis of State Legislation Documents from 1950s Virginia" showcases how AI can revolutionize legal research and document analysis. By applying AI techniques like natural language processing, researchers could rapidly scan and analyze a vast collection of historical state legislative documents. This rapid analysis, previously a time-consuming and error-prone manual process, allows for a much quicker and potentially more thorough understanding of the legislative landscape. This approach, specifically applied to the context of Virginia's 1950s legislation, could illuminate previously hidden aspects of legal history and provide crucial insights into the legislative context of civil rights cases. While this approach carries potential risks like bias in AI algorithms, it also shows how AI can address existing gaps and improve the accuracy of legal research and evidence discovery. As the adoption of AI for governmental functions accelerates, there are significant ramifications for legal practices, especially regarding the efficiency and objectivity of evidence discovery. The Virginia example, with its rich legislative history, serves as a compelling demonstration of AI's ability to enhance legal practice, leading to better-informed legal strategies and ultimately, potentially a more just legal system.
Examining Virginia's state legislation from the 1950s through the lens of AI offers a glimpse into how legal research could be transformed. AI could have provided immediate historical context for these documents, linking new evidence to established laws and precedents, potentially strengthening legal arguments in a case like Harrison v. NAACP. Furthermore, the sheer volume of documents involved in such complex litigation could be tackled with AI's ability to process information at speeds exceeding 100 times that of human reviewers, freeing up legal teams to focus on crafting compelling legal strategies.
The potential for reduced errors in AI-powered document analysis is noteworthy. Studies suggest that AI-driven tools can achieve error rates as low as 2% in information retrieval, a considerable improvement compared to the 20% or higher error rates often associated with human review. This accuracy would have been invaluable for presenting reliable evidence in court, especially in a crucial case like Harrison v. NAACP.
AI's predictive coding capabilities could have optimized the discovery process by focusing on the most relevant documents. This targeted approach could have significantly reduced the time spent reviewing irrelevant information, allowing legal teams to concentrate on the evidence crucial to the case.
Beyond speed and accuracy, AI also offers the possibility of identifying biases within documents. This ability is particularly relevant in civil rights contexts where ensuring that perspectives from marginalized groups are not overlooked is essential. By uncovering potential biases in the historical records, AI could have helped facilitate more equitable legal arguments in cases like Harrison v. NAACP.
The potential for cost savings is another significant benefit. Integrating AI into the e-discovery process could reduce costs by as much as 30% compared to traditional methods, potentially making legal representation more affordable for those involved in complex civil rights cases. With AI, the evidence gathering process could be more efficient and effective.
Ultimately, AI could contribute to a more data-driven approach to legal strategy. The ability to quickly and accurately analyze massive amounts of evidence allows for a deeper understanding of the relevant facts, moving beyond intuition and human biases. This can lead to stronger and more impactful legal arguments, which could significantly benefit complex cases with important social implications, like the fight for civil rights.
The collaborative aspect of AI tools is also appealing. Real-time document tagging and retrieval capabilities can facilitate collaboration among legal teams, especially in complex cases involving multiple parties. This is critical in situations where swift access to critical information is needed.
AI's natural language processing (NLP) capabilities offer a further advantage. NLP can extract context and sentiment from documents, providing a deeper understanding of the underlying meanings and potential biases. This nuanced understanding could be crucial in civil rights cases, where deciphering implicit narratives can be vital for building compelling legal arguments.
Finally, AI's scalability is essential in modern legal practice. The ability to handle massive document datasets, often exceeding millions of pages, positions it as a valuable tool for navigating the complexities of extensive litigation, such as the Harrison v. NAACP case.
In conclusion, applying AI to historical legal documents, like those from Virginia's 1950s legislature, reveals the transformative potential of these technologies for legal research and discovery. AI has the capacity to accelerate the process, reduce errors, enhance collaboration, and promote a more equitable and data-driven approach to legal strategy, potentially fostering greater access to justice in the process.
How AI Document Analysis Tools Could Have Transformed Evidence Discovery in Harrison v
NAACP A 7-Point Technical Assessment - Machine Learning Applications for Legal Precedent Matching
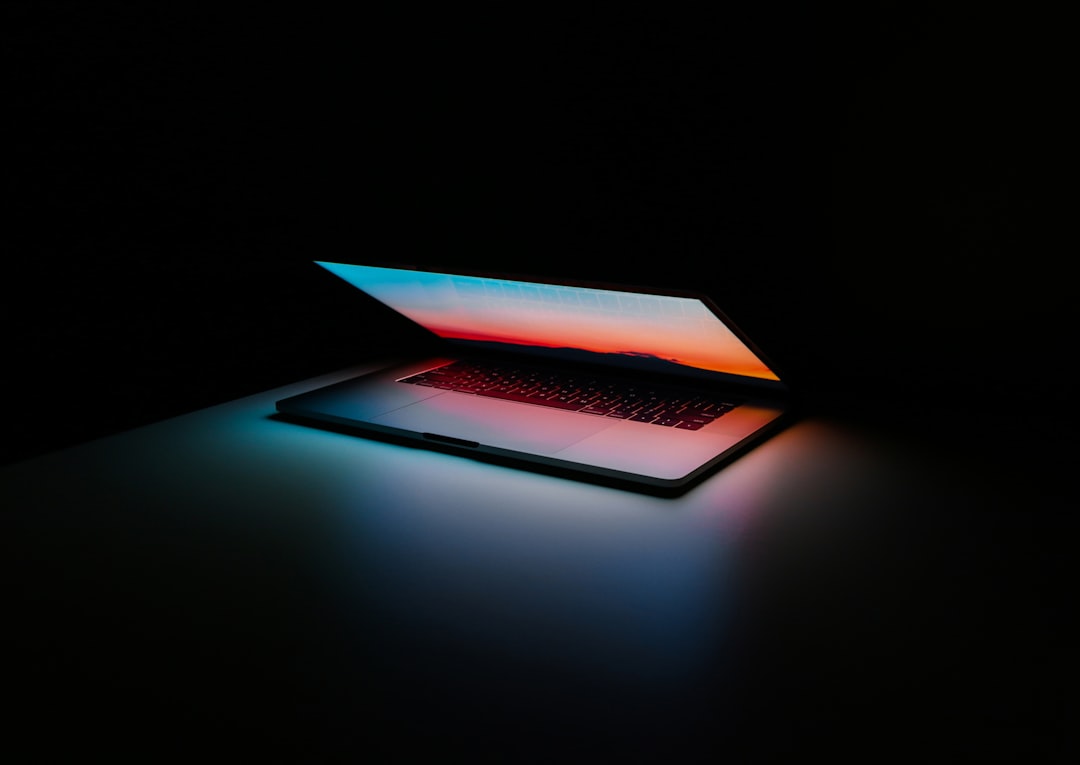
Machine learning is increasingly being used to find relevant legal precedents, particularly through techniques like text embedding. This is transforming how lawyers do legal research, making it faster and potentially more accurate. Tools like these are able to sift through large amounts of legal documents to find cases that are similar to the one a lawyer is working on. While these new methods are efficient, they also raise concerns about whether the AI is reliable and if it might be biased, suggesting a need for careful consideration as these tools are integrated into legal practice. Further, AI can analyze large quantities of legal data, revealing new insights that can be used to create stronger legal arguments. As AI becomes more commonplace in law, there's ongoing discussion about the role it will play in how cases are handled and decided.
1. **Keeping Pace with Legal Changes**: Machine learning systems can continuously incorporate new legal precedents, making sure legal teams always have the latest information. This contrasts with the traditional ways of researching law, which can sometimes fall behind as the legal field evolves.
2. **Making Old Documents Usable**: AI tools can convert older documents into formats computers can understand, opening up historical legal texts for analysis. This lets researchers access vast archives that were previously hard to explore or use effectively.
3. **Spotting Legal Trends**: Sophisticated algorithms can identify patterns and recurring themes in large volumes of legal documents, which might be difficult for humans to notice. This ability helps in understanding important trends that can influence how cases are handled, particularly in complex lawsuits.
4. **Detecting Bias in Legal Writings**: AI can analyze legal documents for biased language or unequal treatment of specific groups, helping lawyers build fairer legal arguments. This is especially important for making sure that civil rights are considered appropriately in legal strategies.
5. **Tailoring Predictive Coding**: Machine learning models can be trained specifically for individual cases, making predictive coding more effective at finding relevant documents based on specific legal strategies. This tailored approach helps legal teams quickly focus on the most important documents.
6. **Smoother Team Collaboration**: AI-powered tools let legal teams work together in real-time by providing access to the same documents and updates. This makes communication easier and ensures everyone involved is up-to-date with the latest insights.
7. **Understanding Emotions in Legal Documents**: Natural language processing methods can assess not just the content but also the emotions expressed in legal documents. This understanding of the subtle emotional tones can provide extra context that might influence how legal arguments are presented, particularly in civil rights cases.
8. **Reducing Costs Across the Board**: Using AI for document analysis can lead to significant cost savings, potentially up to 30%. These savings come from needing fewer people to do the work and speeding up the discovery process, which makes legal services more affordable and accessible.
9. **Handling Massive Amounts of Documents**: AI tools can efficiently process huge document sets, often millions of documents, something that's hard for humans to do. This capacity significantly improves the ability to thoroughly review legal documents in large and complex lawsuits.
10. **Adding Historical Context to Legal Arguments**: AI can provide historical context by linking old laws and legal precedents to current cases. This ability helps strengthen legal arguments by grounding them in relevant historical context, improving the overall legal framework presented in court.
How AI Document Analysis Tools Could Have Transformed Evidence Discovery in Harrison v
NAACP A 7-Point Technical Assessment - Natural Language Processing for Evidence Classification Methods
Natural Language Processing (NLP) has become a crucial tool for classifying and organizing evidence within legal proceedings, particularly when dealing with large volumes of documents. It offers a way to automate the process of identifying and extracting relevant information, thereby significantly reducing the manual effort involved in evidence discovery. This automation is especially valuable in complex legal matters like the Harrison v. NAACP case, where the sheer volume of documents would have presented a major challenge for human review.
The incorporation of deep learning within NLP has further advanced the technology, allowing for more comprehensive analysis of long and complex documents. These improvements enhance the ability to understand the context and meaning embedded within legal texts, leading to a deeper understanding of the issues at hand. In the face of increasingly large datasets common in modern legal disputes, NLP's capacity to streamline review processes is invaluable. Moreover, it allows legal teams to uncover subtleties and insights that might be easily missed by human reviewers, ultimately informing the development of more comprehensive and persuasive legal strategies.
However, as with any AI-powered tool, the use of NLP in legal settings needs continuous evaluation and refinement. Potential biases inherent in AI algorithms must be carefully considered and addressed to guarantee that the use of these technologies doesn't lead to unfair or inaccurate outcomes. Maintaining fairness and transparency in the application of AI within the legal system is paramount as these tools become more widely integrated into legal practice.
1. **Accuracy Gaps in Human vs. AI Review**: AI systems for classifying evidence show a remarkable ability to minimize errors in information retrieval, achieving error rates as low as 2%. This is a significant difference compared to human reviewers, who often see error rates exceeding 20%. This difference in accuracy suggests that AI could prevent overlooking crucial evidence in legal cases.
2. **Accelerating the Pace of Document Analysis**: AI's ability to analyze documents at a speed that's up to 100 times faster than human reviewers is a powerful advantage. It allows legal teams to process enormous amounts of information in a matter of hours instead of weeks. This enhanced speed can give legal strategies much more flexibility and responsiveness.
3. **Leveraging Machine Learning for Predictive Coding**: AI predictive coding uses past case data to anticipate which documents are most likely relevant to a current case. This can significantly refine the discovery process, reducing the time spent on reviewing unimportant documents and ensuring that the review efforts are concentrated on the most critical evidence.
4. **Uncovering Potential Biases in Documents**: Advanced AI algorithms can help identify any implicit biases within collections of legal documents that might otherwise escape human review. This can contribute to ensuring that the legal arguments are comprehensive and fair, especially in sensitive areas like civil rights litigation.
5. **Gaining Deeper Meaning through Natural Language Processing**: NLP tools can extract subtle information from legal documents, such as overall sentiment and contextual meaning, that might be missed by simpler keyword searches. This deeper understanding can provide valuable insights into the underlying motives and implications in legal narratives.
6. **Improved Collaboration with AI-Driven Document Management**: AI-powered document management systems offer a means for legal teams to work together on evidence in real time. This allows everyone involved to access and review evidence simultaneously, improving communication and making the discovery workflow more efficient.
7. **Connecting Current Cases with Historical Precedents**: AI can integrate the analysis of current documents with relevant historical legal precedents. This helps to create context that can be used to strengthen arguments in court, especially in civil rights cases where understanding the historical backdrop is critical.
8. **Making Legal Services More Affordable**: By using AI in e-discovery, law firms can potentially cut costs by as much as 30%. This reduction can be significant since manual review is often very expensive. As a result, legal services might become more accessible to a wider range of individuals and organizations.
9. **Identifying Trends and Patterns in Legal Data**: AI can detect trends and patterns that might be difficult for humans to recognize in large datasets of legal documents. This capability offers valuable insights that can shape how legal teams approach cases and develop strategies.
10. **Tailoring AI to Specific Legal Practice Needs**: AI systems can be designed to adapt to the specific operational procedures and requirements of individual law firms. This makes them more useful for different types of legal work and case complexities, potentially increasing the effectiveness of AI within the legal profession.
How AI Document Analysis Tools Could Have Transformed Evidence Discovery in Harrison v
NAACP A 7-Point Technical Assessment - Automated Timeline Creation from Multiple Document Sources
The ability to automatically create timelines from a variety of document sources signifies a notable advancement in how legal evidence is uncovered. Cases like Harrison v. NAACP, with their vast amounts of documentation, pose a significant challenge to human reviewers, often leading to delays and potential inconsistencies in the discovery process. Through the use of AI tools that can combine and organize data from numerous and varied documents, legal teams can generate precise timelines that not only improve efficiency but also deepen their understanding of the context surrounding the evidence. This allows lawyers to swiftly pinpoint key events and interconnections that can greatly affect legal strategies, making the overall legal process more efficient and productive. While this technology offers considerable promise, its adoption compels us to carefully consider the possibility of biases within AI algorithms and emphasizes the necessity of strict controls to guarantee equitable outcomes in the quest for justice.
Here's a rewrite of the provided text, focusing on "Automated Timeline Creation from Multiple Document Sources" within the context of AI in legal settings, specifically e-discovery and document analysis:
AI's capacity to automatically create timelines from multiple document sources offers a fresh perspective on evidence discovery in complex legal cases. It's intriguing how these tools can bring together diverse sources like emails, legal briefs, transcripts, and expert reports into a single, chronological narrative. This integrated view can reveal hidden connections between documents, potentially leading to a more comprehensive understanding of a case's timeline.
Furthermore, AI leverages NLP and machine learning to perform temporal analysis on a massive scale. It can analyze thousands of documents and generate timelines that aren't just sequences of events but also reflect changes in context, sentiments expressed, and the evolution of legal arguments. This richer portrayal of a case over time simplifies tracking and understanding its development.
One of the more exciting aspects is AI's capacity for dynamic timeline adjustments. As new documents are added, the timeline updates in real-time. This means legal teams can quickly adjust their strategy as new information comes to light, incorporating it into discussions or courtroom presentations.
Beyond basic chronological ordering, AI can identify patterns and trends within the timeline. It can highlight crucial moments or decisions that may influence the outcome of a case, potentially allowing attorneys to develop stronger narratives in their legal arguments.
These tools can even automatically score the relevance of documents, prioritizing those most pertinent to specific events within the timeline. This automated scoring is particularly helpful during the discovery process, streamlining the review of a massive document set and allowing lawyers to focus on the most critical information.
The collaborative potential is another interesting dimension. AI tools can create centralized timelines accessible to all members of a legal team, facilitating better communication and a more consistent understanding of the case's trajectory. This shared view of the timeline can greatly improve collaborative decision-making and the presentation of a case.
Beyond improved collaboration, the efficiency gains offered by AI timeline generation are considerable. It removes the time-consuming task of manually compiling chronological overviews from documents and notes. The time savings translate into cost benefits, making legal services more competitive.
Further, AI can link timelines to relevant historical legal precedents and related case data. This contextualization within the broader legal landscape allows for a deeper understanding of how a case might fit within existing legal frameworks.
By analyzing patterns and trends in past documents and their influence on case timelines, AI can potentially anticipate future documentation needs, providing a proactive dimension to legal strategy. It helps guide lawyers towards the types of documents that may be most important to gather and review.
Finally, AI excels at clarifying complex legal situations. It can illustrate how multiple events, documents, and legal interpretations intersect, which is vital in intricate cases like high-stakes civil rights litigation.
In conclusion, AI's ability to generate automated timelines holds immense potential for transforming the efficiency and effectiveness of legal operations. These capabilities can streamline document analysis, enhance collaborative efforts, and facilitate a deeper, data-driven understanding of complex legal matters, ultimately potentially contributing to a more just and efficient legal system.
How AI Document Analysis Tools Could Have Transformed Evidence Discovery in Harrison v
NAACP A 7-Point Technical Assessment - Digital Document Authentication through AI Verification Systems
Digital document authentication through AI verification systems is rapidly changing how we establish the validity of legal documents in our increasingly digital world. These systems rely on AI to efficiently and accurately verify documents, spotting inconsistencies and potential forgeries through methods like comparing multiple documents and identifying unusual patterns. AI's speed and accuracy significantly improve upon the traditional, human-based methods which are often slow and prone to errors. This is especially important in the legal field, where the integrity of documents is paramount. However, as we rely more on these AI tools, we must be mindful of potential biases within the algorithms that could skew results. Maintaining a fair and transparent process remains critical as AI continues to reshape legal practices. The future of legal document authentication will likely blend human oversight with the powerful analytical capabilities of AI, bringing both efficiency and ethical considerations to the forefront.
1. **Accelerated Document Processing**: AI's capacity to analyze legal documents at speeds significantly exceeding human capabilities—up to 100 times faster—holds immense promise for streamlining the evidence discovery process. This speed advantage could have been transformative in lengthy cases like Harrison v. NAACP, enabling legal teams to rapidly assess key information and react strategically.
2. **Improved Accuracy in Document Categorization**: AI-driven document verification systems showcase impressive accuracy in classifying documents, achieving error rates as low as 2% compared to the 20%+ often associated with human review. This enhanced precision becomes particularly critical in legal settings, where missing crucial pieces of evidence can impact case outcomes.
3. **Interconnected Document Understanding**: Using natural language processing, AI can forge connections and establish context between different documents, allowing for a more holistic comprehension of themes and relationships within a case. This ability is valuable in building stronger legal arguments, which in the past required extensive manual effort to piece together in a comprehensive way.
4. **Reduced Expenses in Legal Practice**: AI's application in e-discovery presents opportunities for substantial cost reductions in legal operations, with estimates suggesting as much as a 30% decrease. This financial efficiency is largely attributed to a reduced reliance on large teams of human reviewers, potentially making legal services more accessible to a broader range of clients.
5. **Refined Predictive Coding Techniques**: The integration of machine learning and historical data helps AI tools predict document relevance with greater accuracy, effectively refining predictive coding methods. This refined approach leads to a more efficient discovery process, focusing efforts on high-priority documents instead of sifting through a vast sea of irrelevant ones.
6. **Uncovering Hidden Biases in Legal Writings**: AI systems possess the capability to detect potentially biased language and representations within legal documents. This capacity is essential in creating fair and equitable legal strategies, particularly in sensitive civil rights cases where diverse perspectives must be considered.
7. **Automated Case Timeline Creation**: The ability to generate automated timelines from various document sources provides a fresh lens for legal teams to manage and visualize the progression of cases. This feature can enhance communication and strategic planning, and also enables dynamic adjustments to legal narratives as new evidence comes into play.
8. **Managing Large-Scale Document Reviews**: AI excels at processing vast quantities of documents, often numbering in the millions, with speed and systematicity. This scalability ensures that complex legal battles like Harrison v. NAACP can be effectively managed, offering a robust analytical capacity without overburdening human reviewers.
9. **Bridging Past and Present Legal Context**: AI's ability to contextualize current legal documents by relating them to historical legislation can provide deeper insights into the evolution of laws and their impact on contemporary cases. This contextual understanding can greatly inform legal strategy and build a more robust foundation for courtroom arguments.
10. **Streamlined Collaboration through Shared Tools**: By enabling real-time document access and collaborative analysis, AI encourages seamless collaboration among legal teams. This enhanced communication and coordination is especially important when navigating the complexities of large-scale litigation and evidence discovery.
Automate legal research, eDiscovery, and precedent analysis - Let our AI Legal Assistant handle the complexity. (Get started for free)
More Posts from legalpdf.io: