Automate legal research, eDiscovery, and precedent analysis - Let our AI Legal Assistant handle the complexity. (Get started for free)
Analyzing the Impact of Critical Race Theory on AI Contract Review Practices
Analyzing the Impact of Critical Race Theory on AI Contract Review Practices - Understanding Critical Race Theory's Origins and Evolution in Legal Contexts
Critical Race Theory (CRT) took root within the Critical Legal Studies movement, which gained momentum in the late 1970s. This movement challenged the conventional understanding of American law by examining the power imbalances that shaped it. CRT's development also drew upon Critical Racing Law, a field that delved into the interconnectedness of race and gender within legal frameworks. Key figures like Kimberlé Crenshaw played a critical role in shaping CRT's focus on the intersectional aspects of race and gender.
CRT's core aim is to dissect how historical events and legal systems have inadvertently perpetuated racial inequality. Its evolution has involved scrutinizing historical narratives to understand how racial biases have become embedded in legal frameworks. Since 2020, CRT has become a contentious topic, with proponents seeing it as essential for comprehending America's legal heritage while critics sometimes misinterpret its core tenets.
A central tenet of CRT is the need to bring racial considerations to the forefront of legal discourse. Traditional legal scholarship, CRT proponents argue, often fails to adequately address the multifaceted impacts of racial issues on society. By centering race, CRT has broadened discussions around civil rights and expanded the notion of legal equality to include racialized citizenship. The growing body of CRT-related research demonstrates its continued influence in diverse academic and societal fields, driving reassessment of diversity and inclusion efforts across various sectors. Notably, criminal justice and related legal systems have seen increased application of CRT to understand the effects of systemic racism on minority communities.
Critical Race Theory (CRT) arose from the dissatisfaction with conventional civil rights strategies, particularly their perceived failure to fully grasp and dismantle the deeply ingrained nature of racism within legal systems. Its origins can be traced back to the late 1970s and early 1980s, emerging from the Critical Legal Studies movement, a school of thought that examined law through the lens of power structures.
Further contributing to CRT's development was the field of Critical Race Law, which integrated insights from critical race feminism and explored how race and gender intertwine within legal contexts. This perspective, particularly highlighted by scholars like Kimberlé Crenshaw, led to a more nuanced understanding of how these identities intersect to shape lived experiences and legal outcomes.
Crenshaw's work played a vital role in shaping CRT's focus on understanding how race intersects with other identities, leading to the development of frameworks that analyze the layered impacts of social categories and systems of power. This intersectional approach is central to CRT's critique of traditional legal narratives, which often fail to fully acknowledge and address the complex ways that various forms of oppression overlap.
Since the early 2020s, CRT has become a subject of intense public debate, often with misunderstanding and misrepresentation of its central concepts. This debate has highlighted the theory's enduring relevance to examining historical and contemporary inequalities rooted in racism. Proponents emphasize the necessity of centering race within legal discourse, arguing that traditional legal scholarship frequently overlooks or downplays its impact on society.
One of CRT's core goals is to dissect how narratives and legal frameworks have perpetuated systemic racism over time. Historical analysis serves as a critical lens through which to unpack how these frameworks function. CRT argues that racial considerations are essential to discussions of law and justice, pushing back against the tendency to ignore or minimize racial disparities.
CRT extends its scope beyond racism itself to analyze the concept of racialized citizenship. This expansion broadens the scope of inquiry within legal discussions, bringing into sharper focus the fight for equal rights and legal equality for all.
The influence of CRT grew significantly from 2011 to 2019, evidenced by a surge in academic work that used CRT as an analytical framework. These studies have placed racial issues at the core of inquiry and considered various perspectives related to race.
The application of CRT extends beyond academia, impacting diversity and inclusion initiatives in numerous sectors, such as the workplace and educational institutions. These efforts have prompted a thorough reevaluation of how institutions address race and diversity, fostering a more critical examination of existing systems.
Finally, within criminal law, CRT has sparked investigation into its implications for racial minorities within the legal system. This research sheds light on the ways in which systemic disparities manifest within the legal system, contributing to inequalities in outcomes.
Analyzing the Impact of Critical Race Theory on AI Contract Review Practices - The Intersection of AI and Contract Review in Modern Legal Practices
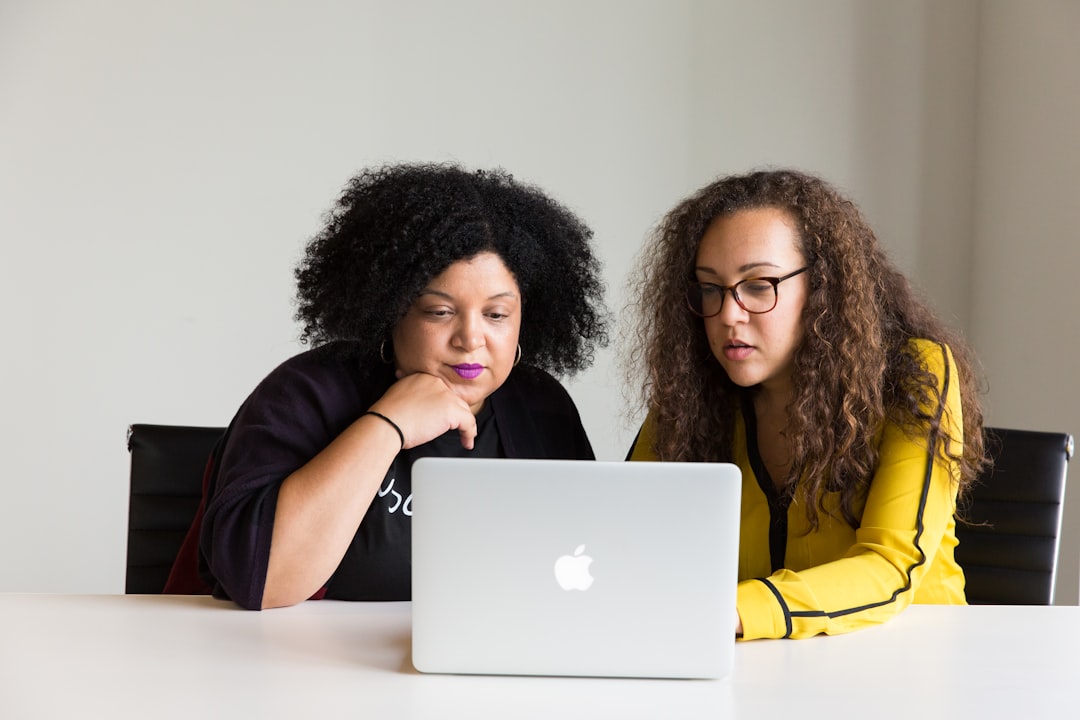
The intersection of AI and contract review has brought about a notable shift in modern legal practices. AI's ability to analyze contracts with speed and precision offers a new level of efficiency and accuracy, potentially streamlining legal workflows and improving decision-making. While these advancements are undeniable, they also introduce concerns about over-reliance on technology and the potential for inherent biases within AI algorithms to perpetuate or even worsen existing inequalities. AI's growing role in legal tasks is fundamentally changing how legal work is performed, prompting a reassessment of traditional skills and the need for legal practitioners to adapt to this changing environment. It's crucial that we critically examine how this technological evolution intersects with broader social and legal contexts, particularly those addressed by critical race theory. Finding the appropriate balance between harnessing AI's potential and retaining the indispensable human element in legal practice—with its nuanced understanding of ethical and contextual complexities—is paramount for the future of the legal profession. This includes considering how the technology might inadvertently reinforce or exacerbate existing systemic biases and inequalities, a crucial aspect of the ongoing discussion surrounding AI's place within legal practice.
The convergence of artificial intelligence (AI) and contract review is reshaping legal practices. AI's ability to quickly sift through complex documents, potentially reducing review times by a significant margin, is freeing up lawyers to concentrate on more intricate, strategic tasks that require human intellect and judgment. However, this increased efficiency also brings forth concerns. Studies suggest that the training data used to develop AI algorithms can inadvertently embed biases, potentially leading to skewed outcomes and possibly discriminatory practices.
Aligning AI contract review tools with the principles of Critical Race Theory (CRT) underscores the importance of scrutinizing the design of AI systems. The goal is to ensure that these systems don't inadvertently amplify existing systemic inequalities within the legal realm. Intriguingly, AI’s capacity to analyze extensive historical contract data can uncover concealed biases that might escape human notice, potentially promoting greater transparency in legal processes.
However, some worry that the rise of AI in contract review might inadvertently hinder the development of young lawyers. Traditionally, junior lawyers gain crucial experience through manual contract review. If AI automates much of this work, they might miss out on these formative learning opportunities, potentially impacting their future growth within the legal profession.
Moreover, the accelerated compliance with regulatory requirements that AI-assisted contract review seems to facilitate has also raised apprehensions. Some question whether reduced human oversight leads to overly hasty reviews that lack depth and nuance.
This intersection of AI and CRT highlights the need for clarity and accountability in algorithmic decision-making processes. By promoting transparency, law firms can better identify and address biases within contract language and related outcomes. It could lead to the development of fairer and more equitable legal tools.
Although AI can bring greater consistency to contract interpretation, conflicting views on bias might impede the effective implementation of CRT principles in AI systems. This creates the need for careful consideration and ongoing discussion.
As a consequence, jurisdictions that employ AI for contract review are progressively implementing standards and best practices, reflecting a move toward enhanced accountability within the legal arena.
The broader ethical implications of automated decision-making, especially the relationship between technological advancement and justice, form a crucial part of the evolving dialogue surrounding CRT and AI. It urges developers and legal practitioners alike to consider how these advancements can be used to uphold fairness and promote justice in legal practice.
While AI has the potential to revolutionize contract review, it’s imperative to remain mindful of the pitfalls and potential for perpetuating inequalities. The journey toward harnessing AI in legal practices demands ongoing consideration of its ethical implications and how it can be integrated responsibly within the framework of social justice and equality.
Analyzing the Impact of Critical Race Theory on AI Contract Review Practices - Examining Racial Bias in AI-Driven Contract Analysis Tools
The rise of AI in contract analysis presents a critical juncture where the potential for racial bias becomes acutely apparent. These tools, trained on historical data, can inadvertently perpetuate and amplify existing racial inequalities that have shaped legal systems and practices. Understanding how AI systems can reflect and even worsen racial disparities is crucial, and Critical Race Theory (CRT) provides a powerful framework for this examination. A major challenge arises from the lack of transparency within many AI algorithms, making it difficult to identify and address how bias might be shaping contract outcomes. This opacity raises serious concerns about accountability, especially when the impacts of these biases can have profound consequences on individuals and communities.
It is imperative that legal professionals actively question the assumptions and potential biases embedded in these AI-driven systems. The over-reliance on these technologies, without careful consideration of their implications, risks reinforcing the very systemic inequalities that CRT aims to dismantle. Moving forward, a clear need exists for interventions that prioritize ethical considerations in the development and deployment of these technologies. By incorporating a critical lens that acknowledges and challenges the potential for racial bias, the legal field can strive toward equitable contract analysis and a more just legal landscape.
AI-powered contract analysis tools, while promising in their ability to quickly process legal documents, might inherit biases embedded within the historical data used for their training. This means that if past contracts reflect existing societal racial inequities, the AI system might inadvertently learn and replicate those biases in its assessments and recommendations. This could manifest as different outcomes depending on the demographics reflected in the training data – contracts primarily from certain racial or socioeconomic groups could potentially lead the AI to overlook or misunderstand the needs of other groups.
One of the key challenges lies in the "black box" nature of many AI algorithms. Their internal workings are often opaque, hindering our ability to fully understand how they arrive at specific conclusions. This lack of transparency makes it difficult to identify biases that could negatively impact fair legal practices. Moreover, increasing reliance on AI for contract analysis can potentially stifle the professional development of young lawyers. Traditionally, a significant part of their training involved manually reviewing contracts, gaining valuable insights into legal nuances. If AI automates much of this process, a crucial learning opportunity could be lost, potentially impacting their capacity to spot and rectify bias in the future.
CRT highlights that race intertwines with other aspects of identity in intricate ways. Many AI systems may not yet fully incorporate this complex perspective, simplifying analyses related to multi-faceted identity. This simplification can have unintended consequences on legal decisions. However, the growing use of AI for contract review is prompting the development of standards and best practices aimed at increasing accountability and mitigating biased outcomes. This effort underscores a push towards ensuring fairness in the application of AI within legal frameworks.
The success of AI tools is heavily reliant on the understanding of subtle cultural aspects within the language of contracts. Without this contextual understanding, AI could misinterpret crucial terminology and potentially inadvertently favor specific racial or demographic contexts over others. Though AI can bring consistency to contract interpretation, human oversight remains crucial. Over-reliance on automated systems, particularly in areas requiring nuanced understanding of social and cultural factors, can lead to errors.
Interestingly, AI's capacity to examine a vast volume of historical data might reveal previously unseen patterns of discrimination in contract language. This could be an opportunity for legal reform, prompting changes that could foster greater equity within the legal system. However, the implementation of CRT principles within AI tools is uneven across legal sectors and jurisdictions. Some firms might adopt more rigorous frameworks to address bias, while others might lack a clear structure, leading to inconsistent levels of fairness in legal decision-making.
Analyzing the Impact of Critical Race Theory on AI Contract Review Practices - Integrating CRT Principles into AI Algorithm Development for Legal Applications
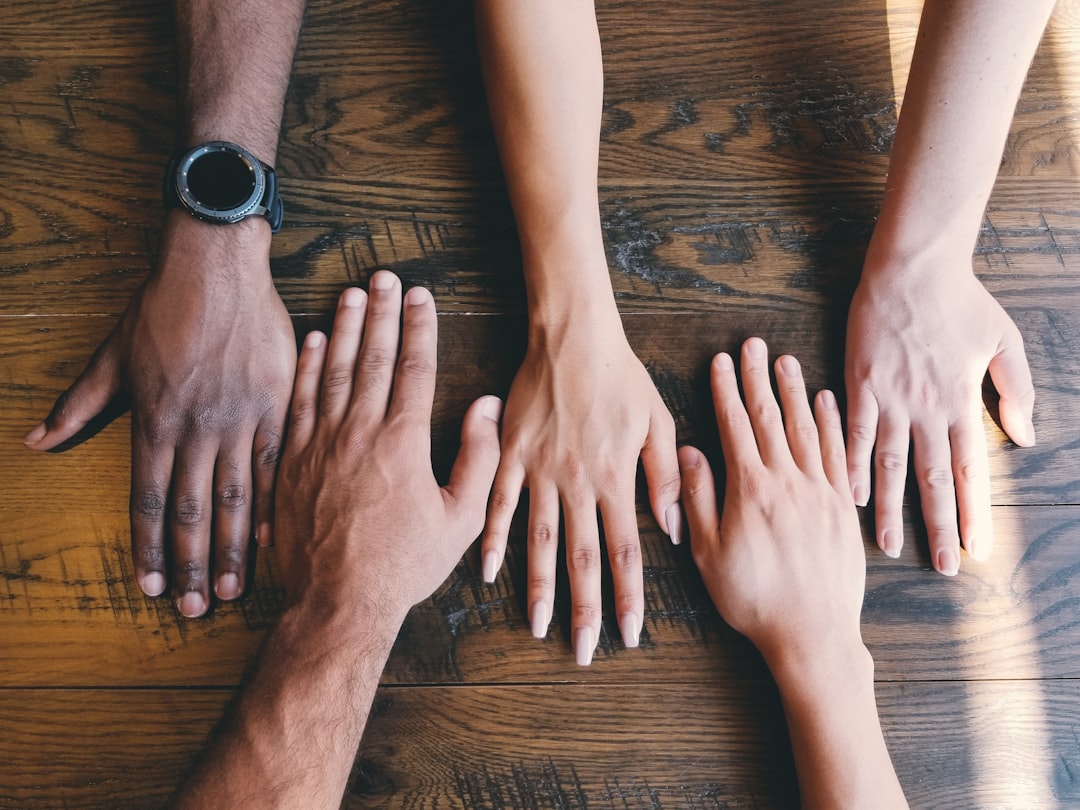
Integrating CRT principles into the development of AI algorithms used in legal settings is crucial for tackling the issue of racial bias and inequality. As AI becomes more prevalent in legal practice, we must carefully examine the data and methods used to train these algorithms to prevent the perpetuation of existing disparities. By incorporating CRT into the design and assessment phases of AI development, developers can identify and mitigate potential biases, aiming for more equitable outcomes in legal applications like contract review. This approach emphasizes transparency and accountability in how AI makes decisions, prompting a crucial review of the relationship between technology and deeply rooted social injustices. Ultimately, integrating CRT principles provides a pathway for creating AI systems that are both ethically sound and socially responsible within the legal field.
1. Incorporating CRT principles into how AI algorithms are built can help us spot biases in the results AI produces, which can then be used to directly challenge the systemic inequalities that CRT aims to fix.
2. It's interesting that while many AI algorithms are designed to be unbiased, their actions can sometimes reflect the biases present in society's history. For example, if an algorithm is trained on data that's biased, it might end up reinforcing racial inequalities in how contracts are evaluated.
3. In most places, the legal rules about transparency aren't developed enough to hold AI-powered contract analysis accountable for racial biases. This creates a big gap in legal protection and ethical considerations.
4. AI's ability to sift through massive datasets can unintentionally highlight historical biases in the language of contracts that might not have been noticed before, potentially leading to systemic changes in both form and function of legal practices.
5. If the data used to train AI systems isn't diverse enough, these systems may not be able to understand the nuances related to different racial and social groups, leading to uneven outcomes and interpretations in contract reviews.
6. Relying heavily on automated contract analysis could weaken the practical training of law students and younger attorneys. Important skills that are developed through manual review are at risk of being eliminated from legal education.
7. New ways of using CRT suggest that including racial equity metrics directly into the design of AI algorithms could significantly improve the quality and fairness of contract review results.
8. The "black box" nature of many AI algorithms makes accountability in legal practices more difficult. Human oversight needs to be combined with AI technology to make sure that diverse legal contexts are represented fairly.
9. Addressing racial disparities in AI-powered contract analysis isn't just about fixing algorithms; it's also about shifting the culture within legal practices to make equity and inclusivity a priority in decision-making.
10. While incorporating CRT into AI systems might improve their ability to predict equitable outcomes, it also requires ongoing evaluation to ensure that these systems don't inadvertently strengthen existing biases as they develop and change over time.
Analyzing the Impact of Critical Race Theory on AI Contract Review Practices - Challenges in Implementing CRT-Informed AI Systems for Contract Review
Implementing AI systems for contract review that are informed by Critical Race Theory (CRT) presents a number of challenges. A primary concern is the potential for these systems to unintentionally perpetuate existing racial biases embedded within historical data used for training the algorithms. While AI can offer improvements in efficiency and accuracy, the opaque nature of many algorithms makes it difficult to ensure accountability and transparency, increasing the risk of biases impacting contract outcomes. Furthermore, the current environment surrounding CRT discussions can create opposition or pushback against incorporating CRT principles into AI development, potentially hindering the movement towards fairer legal practices. It's crucial for legal professionals to thoughtfully analyze the potential racial implications of using AI tools, and to prioritize an organizational culture that emphasizes inclusion and ethical considerations in their deployment. Ultimately, the successful integration of CRT-informed AI into contract review practices will require ongoing dialogue, rigorous monitoring, and a persistent commitment to creating a more just and equitable legal landscape.
1. AI systems trained on historical contract data can inadvertently absorb and amplify existing biases, potentially perpetuating systemic inequalities in legal outcomes if not carefully addressed. This is a significant concern as AI becomes more prevalent in contract review.
2. Many AI algorithms lack transparency, operating as "black boxes" where their internal decision-making processes are difficult to understand. This opacity makes it challenging to identify and address any inherent biases, creating obstacles to accountability in legal situations.
3. Implementing CRT principles in AI development requires a shift in perspective, not just in the data used to train the algorithms, but also in the design process itself. This involves thinking critically about the intersectional ways race, gender, and socio-economic factors influence legal outcomes.
4. There's a risk that increased reliance on AI for contract review could diminish opportunities for junior lawyers to develop essential practical skills. Traditional manual review processes are a vital part of legal education, and AI automation could lead to their decline, hindering the development of future legal professionals.
5. The streamlined analysis offered by AI can potentially unveil hidden patterns of discrimination or bias in the language of contracts. This creates a chance for legal reform by exposing aspects of historical legal practice that have gone unnoticed, fostering a potential path towards greater equity.
6. Many jurisdictions still lack established legal frameworks that specifically address AI accountability and bias. This absence of clear guidelines creates a significant gap in the legal and ethical safeguards needed to ensure AI-driven contract reviews are fair and just.
7. Studies show that incorporating racial equity metrics into the design of AI algorithms can improve their ability to promote fair outcomes in contract analysis. This suggests a practical route to enhance the ethical functioning of AI in legal contexts.
8. Bringing CRT into the realm of AI necessitates a cultural change in legal practices. Merely implementing technological solutions isn't enough; instead, equity and inclusivity must become core values that drive decision-making within the legal field.
9. The use of AI in legal contexts raises crucial ethical questions regarding automated decision-making. It emphasizes the importance of continuous evaluation and monitoring of these AI systems to prevent the unintentional reinforcement of biases over time.
10. It's vital for legal professionals to actively engage with AI technologies and critically examine the underlying assumptions and potential biases embedded within the systems. This scrutiny is essential to ensure that AI applications in contract review are aligned with the principles of equity and justice advocated by CRT.
Analyzing the Impact of Critical Race Theory on AI Contract Review Practices - Future Directions for Equitable AI Contract Review Practices
The path towards equitable AI contract review practices necessitates a careful balancing act between technological advancement and a steadfast commitment to fairness. As AI's influence grows within legal domains, a critical concern arises regarding the potential for these systems to inadvertently perpetuate historical biases embedded in their training data. Proactive measures involve employing an algorithmic design approach that is informed by Critical Race Theory (CRT) principles, fostering a nuanced understanding of how race and other social factors can influence the outcomes of AI-powered contract analysis. Though AI offers significant advantages in terms of efficiency and the potential for exposing previously hidden discriminatory patterns, its increasing prominence could inadvertently hinder the development of legal professionals who traditionally learned valuable skills through manual contract review. Overcoming these challenges requires an ongoing conversation regarding best practices, emphasizing the incorporation of transparency, accountability, and a broader, more inclusive understanding of intersecting social identities into AI systems. Ultimately, this pursuit aims to guide the legal sphere towards a future characterized by greater justice and equity.
AI contract review tools are increasingly common, and while they promise efficiency and accuracy, they also risk perpetuating historical biases embedded in the data they're trained on. This can lead to unfair outcomes, potentially favoring some demographics over others.
Many AI systems used in contract review function as "black boxes," making it difficult to understand how they reach their conclusions and identify any biases built into their decision-making processes. This lack of transparency presents a challenge for ensuring accountability in legal settings.
Developing AI systems that incorporate Critical Race Theory (CRT) principles requires more than just diverse datasets. It calls for a fundamental shift in how these systems are designed, taking into account the interwoven influence of factors like race, gender, and socioeconomic status on legal outcomes.
There are worries that an over-reliance on AI for contract review could lead to a decline in the critical thinking and analytical skills traditionally developed through manual contract analysis. This could hinder the development of future legal professionals and their ability to identify and address bias in legal processes.
The ability of AI to analyze vast amounts of historical contract data could unearth previously hidden patterns of discrimination and bias. This has the potential to serve as a starting point for reforming legal practices and promoting greater equity in contract evaluation.
However, the absence of clear legal frameworks specifically addressing AI bias and accountability creates a significant void in ethical governance. This leaves many jurisdictions unprepared to deal with the ethical implications of AI-powered contract review.
Research suggests that incorporating measures of racial equity directly into the design of AI algorithms can help make contract analysis fairer. This points to a path for improving the ethical standards of legal technology development.
Moving forward, integrating CRT principles into legal AI will require more than just technology implementation. It necessitates a shift in the culture within legal practice, making equity and inclusion core values in how legal decisions are made.
Automated decision-making in legal matters raises important ethical questions, underlining the need for continuous monitoring and adjusting of AI systems to avoid inadvertently reinforcing biases.
Ultimately, legal professionals need to engage critically with AI systems. They must constantly examine the underlying assumptions built into these tools to ensure that their application in contract review aligns with the principles of justice and equity promoted by CRT.
Automate legal research, eDiscovery, and precedent analysis - Let our AI Legal Assistant handle the complexity. (Get started for free)
More Posts from legalpdf.io: