Automate legal research, eDiscovery, and precedent analysis - Let our AI Legal Assistant handle the complexity. (Get started for free)
Analyzing AI's Role in Environmental Impact Assessments Lessons from Standing Rock
Analyzing AI's Role in Environmental Impact Assessments Lessons from Standing Rock - AI-Enhanced Data Analysis in Environmental Impact Assessments
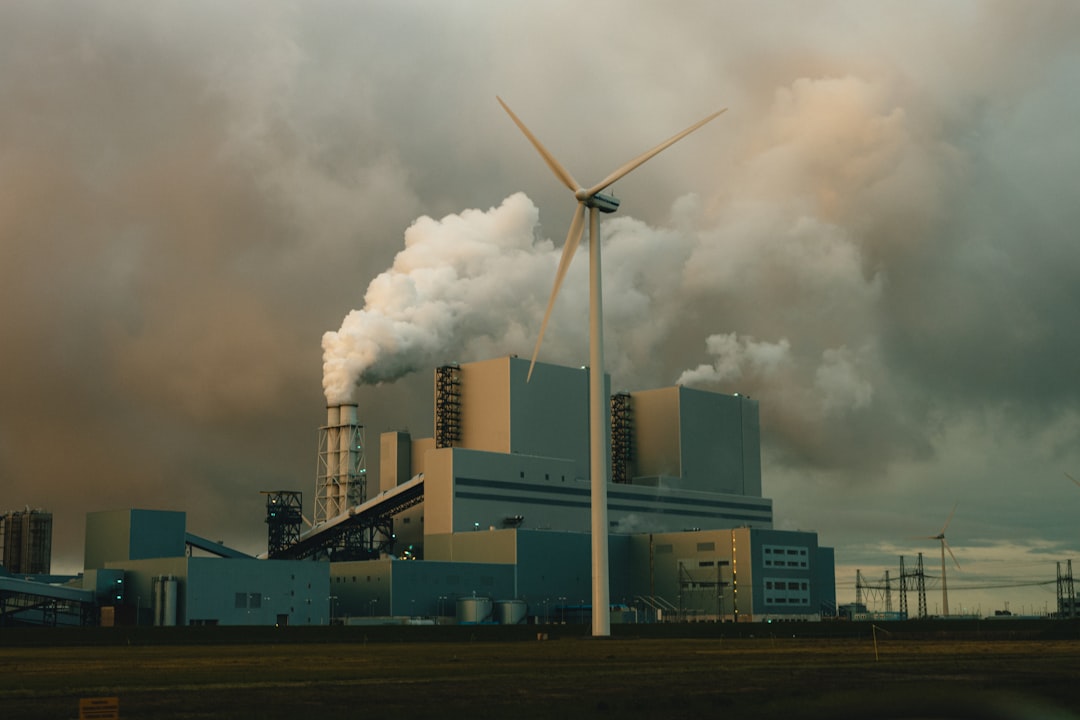
AI is being used to analyze environmental data in new and powerful ways. This is helping to improve the accuracy and scope of Environmental Impact Assessments (EIAs). By using AI to analyze vast amounts of information, we can better understand the risks and impacts of environmental changes, such as climate fluctuations that can worsen natural disasters.
However, this reliance on AI comes with some important environmental concerns. The development and operation of AI systems require significant energy and resources, which can have a negative impact on the environment. For example, the large data centers needed to run AI require a substantial amount of freshwater to cool their servers. It's crucial to consider these trade-offs and ensure that AI is used responsibly in environmental assessments. While AI has the potential to significantly improve our understanding of the environment, its environmental impact must be carefully managed to ensure that its benefits don't come at the cost of environmental degradation.
It's fascinating how AI is changing the landscape of environmental impact assessments. We're seeing algorithms analyze satellite imagery with incredible detail, picking up subtle changes that traditional methods miss. This is key for anticipating potential environmental issues before they become major problems.
The ability of machine learning to spot patterns and anomalies in massive datasets is also impressive. It's like having an extra set of eyes that can see things we might overlook, which can be vital for understanding the risks associated with projects.
Natural language processing has a big role to play too, letting us sift through a mountain of scientific papers and regulations with speed. This is crucial for keeping up with the ever-changing landscape of environmental standards.
AI-powered predictive models can also project the long-term consequences of development projects, allowing us to explore different scenarios and make more informed decisions.
But it's not just about crunching numbers. AI can be used to bring communities into the process, too. Some tools use crowdsourced data from citizen reporting to provide a more grassroots understanding of local environmental conditions.
That said, there are also some real concerns. The potential for bias in AI models needs careful attention. If the data used to train these models isn't diverse and representative, the assessments could be skewed. We need to ensure that AI isn't amplifying existing inequalities, but instead contributes to a more just and sustainable future.
Analyzing AI's Role in Environmental Impact Assessments Lessons from Standing Rock - Integrating Indigenous Knowledge through AI-Powered Community Engagement
Integrating Indigenous Knowledge (IK) into environmental impact assessments through AI-powered community engagement holds promise. By combining the wisdom of traditional knowledge with the power of AI, we can build a more inclusive and effective approach to environmental decision-making. This partnership can help bridge the gap between scientific knowledge and the intimate understanding Indigenous communities have of their lands and resources.
AI can serve as a powerful tool for Indigenous communities to share their knowledge in ways that are accessible and engaging to a wider audience. This can include using AI to create digital platforms for sharing traditional ecological knowledge, translating Indigenous languages, and developing interactive visualizations of environmental data. By leveraging AI, communities can better communicate their knowledge and ensure that it is incorporated into environmental assessments.
However, as with any technology, there are potential risks associated with integrating AI into Indigenous knowledge systems. One significant concern is the potential for data misuse and exploitation. It is crucial to ensure that control over data remains in the hands of Indigenous communities, protecting their cultural knowledge from misinterpretation or appropriation. This means developing AI technologies that are transparent, accountable, and respectful of Indigenous sovereignty.
Overall, the integration of Indigenous knowledge through AI-powered community engagement presents both opportunities and challenges. If implemented thoughtfully and responsibly, it has the potential to create more robust environmental impact assessments, foster greater understanding between cultures, and contribute to the preservation of Indigenous knowledge systems.
The idea of integrating Indigenous knowledge into AI-powered environmental impact assessments is exciting, but it's also quite complicated. Indigenous communities hold a wealth of knowledge, often passed down through generations of observation and experience. This knowledge can offer valuable insights that AI might miss, especially when it comes to long-term environmental trends and local ecosystems.
One challenge is that Indigenous knowledge is often communicated through storytelling and oral traditions, which are difficult to translate into the structured data formats that AI systems rely on. It's not simply about capturing the data; it's also about understanding the cultural context and nuances that inform those observations.
AI can definitely help us analyze patterns in traditional ecological knowledge. But simply translating local languages and culturally-specific concepts into data can lead to a loss of meaning. The unique way Indigenous communities view their relationship with the environment, often emphasizing interconnectedness and relational understanding, may not be fully captured in the binary decision-making frameworks of many AI algorithms.
Another concern is the potential for bias in AI models. If the training data isn't diverse and representative, it could skew assessments, potentially reinforcing existing inequalities. We must be careful that the integration of Indigenous knowledge doesn't just lead to the adoption of AI systems that further marginalize these communities.
Despite these concerns, there are clear benefits to bringing Indigenous knowledge into the fold. Traditional knowledge systems often contain data on local species, habitat conditions, and environmental changes that might not be documented in scientific records. By integrating these insights, AI models can become more accurate and effective.
But, as with any technology, the use of AI must be considered in light of its ethical implications. Data ownership and control are critical issues when incorporating Indigenous perspectives into AI. We need to be mindful of the potential for knowledge appropriation and misrepresentation without proper consent or acknowledgment.
Ultimately, the success of integrating Indigenous knowledge with AI hinges on a constant dialogue and collaboration between developers, researchers, and Indigenous communities. Technological solutions need to be developed alongside these communities, ensuring they are culturally relevant and contextually appropriate.
Analyzing AI's Role in Environmental Impact Assessments Lessons from Standing Rock - Addressing Bias in AI Models for Fair Environmental Assessments
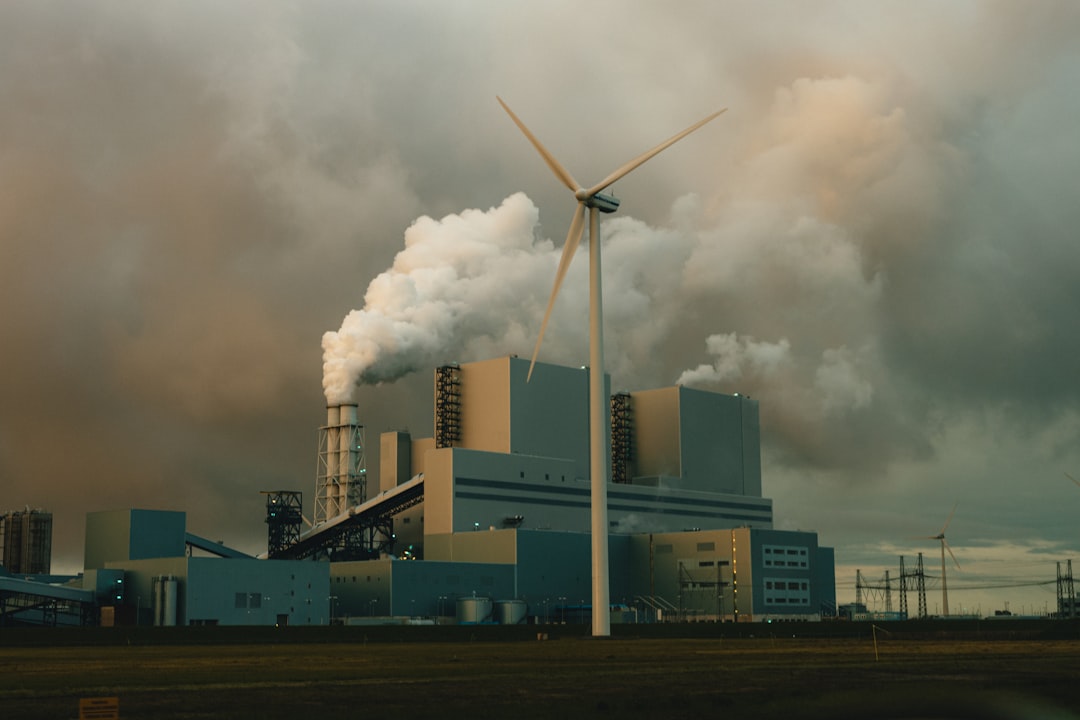
AI has the potential to transform how we conduct environmental impact assessments (EIAs), offering a more accurate and detailed approach. But this advancement comes with a crucial caveat: bias in the AI models used to analyze environmental data can have serious consequences. If the data used to train AI systems isn't representative and diverse, it can lead to biased assessments that unintentionally reinforce existing inequalities. This is particularly concerning when considering the lessons learned from Standing Rock, where environmental injustice was a major concern.
The challenge of bias highlights the need for a more nuanced and ethical approach to AI in environmental management. It's not enough to simply adopt AI technology; we need to actively address the potential for biases in data and algorithms. This requires collaboration between developers, researchers, and communities, ensuring that AI is developed and deployed responsibly, and that its outputs are fair and equitable. The success of AI in environmental assessments depends on addressing these concerns head-on, otherwise, we risk perpetuating the very environmental injustices we aim to prevent.
AI is being used to analyze environmental data, which is exciting because it allows us to better understand the impact of projects on the environment. However, this brings up a major concern: bias in AI models. These models are trained on data, and if that data reflects past biases, the assessments can be skewed, potentially reinforcing existing inequalities.
For example, imagine an AI model trained on data primarily focused on urban areas. It might overlook environmental challenges faced by rural communities, leading to skewed assessments and inadequate resource allocation. The problem is not just technical; biased AI models can have real-world consequences, affecting the quality of environmental impact assessments and leading to unfair outcomes.
It’s also important to consider how bias can arise from the design of the model itself. If certain data points are favored over others, the model might reflect and reinforce pre-existing biases about what's important for environmental sustainability. Additionally, the model might rely on algorithms optimized for specific assumptions, leading to flawed conclusions if those assumptions don't match reality.
Another challenge is transparency. Many AI models operate as "black boxes," making it difficult to understand how they make decisions. This lack of transparency makes it difficult to identify and correct biases within the assessments. We need regulations to ensure fair and equitable outcomes.
But bias in AI goes beyond just data and algorithms. Societal factors also play a role. If model inputs are primarily sourced from affluent areas, crucial concerns in low-income regions may be ignored. This can lead to inadequate consideration of environmental risks.
Ultimately, the impact of bias in AI extends far beyond the data itself. Biased AI can shape narratives about environmental issues, influencing funding, advocacy, and public perception. This can have significant consequences for how we address environmental challenges and promote sustainability.
Analyzing AI's Role in Environmental Impact Assessments Lessons from Standing Rock - AI Tools for Improved Transparency in Pipeline Project Evaluations
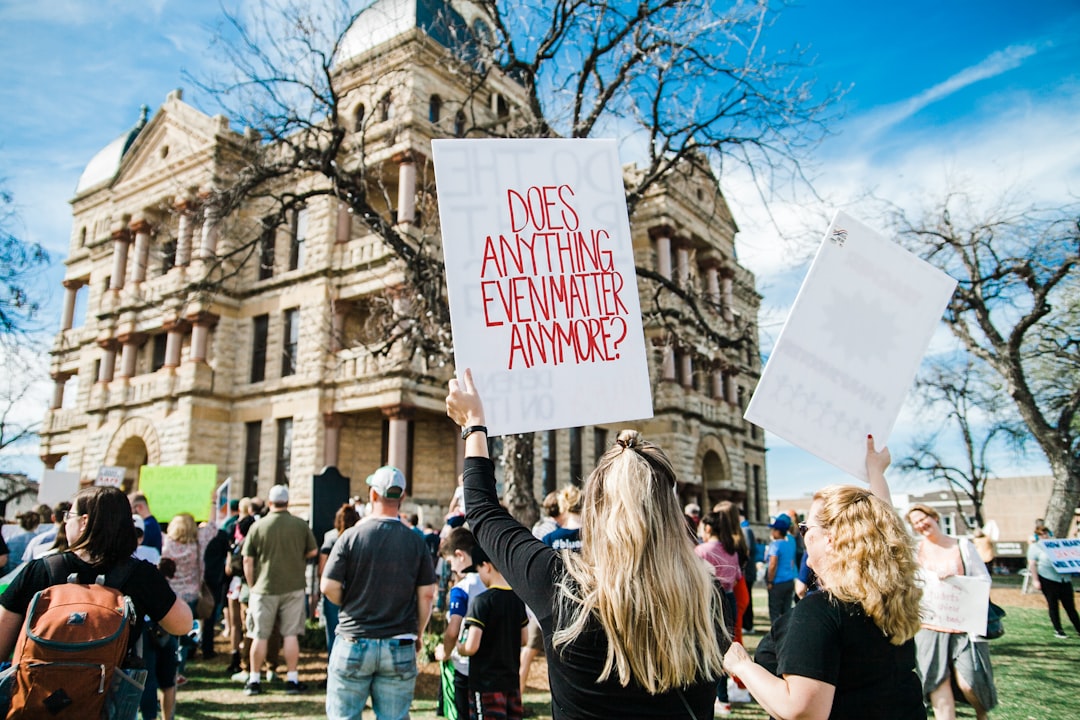
The use of AI tools in the evaluation of pipeline projects has brought a new level of transparency to environmental impact assessments. By using sophisticated data analysis, AI can uncover subtle impacts that traditional methods might miss, leading to more effective compliance with sustainability goals. While this is a promising development, some concerns remain. There are worries about bias in the data used to train AI systems and the need for greater ethical standards. It is critical that the methods used in AI are transparent and that industries don't simply rely on older, less effective practices for assessing environmental risks. As AI develops, its role in making assessments more equitable and transparent needs to be carefully scrutinized to ensure it doesn't simply reinforce existing inequalities.
AI is rapidly transforming the way we evaluate pipeline projects, offering exciting possibilities for increased transparency and efficiency. While it's still early days, AI tools are already demonstrating their ability to streamline data analysis, improve risk assessment, and even predict potential problems before they arise.
For example, AI algorithms can now crunch massive datasets with remarkable speed, revealing patterns and connections that might escape human eyes. This has enabled engineers to predict potential geological risks, analyze socioeconomic impacts, and even simulate various project scenarios with incredible detail. The insights gained from these analyses are invaluable for risk management and decision-making.
However, these powerful AI tools are not without their limitations. A significant concern is the reliance on historical data for training these models. If the data is biased, skewed, or incomplete, the AI assessments could inherit these flaws, perpetuating inaccuracies and perpetuating past mistakes.
Transparency is also a challenge. Many AI algorithms operate like black boxes, making it difficult to understand how they reach their conclusions. This lack of transparency can make it challenging to justify decisions based on AI outputs, potentially hindering regulatory compliance and eroding public trust.
Despite these hurdles, the potential benefits of AI in pipeline project evaluations are undeniable. With responsible implementation and ongoing scrutiny, AI can become a valuable asset for promoting transparency, accountability, and informed decision-making in pipeline projects, ultimately leading to more sustainable outcomes.
Analyzing AI's Role in Environmental Impact Assessments Lessons from Standing Rock - Leveraging Machine Learning to Predict Long-Term Environmental Consequences
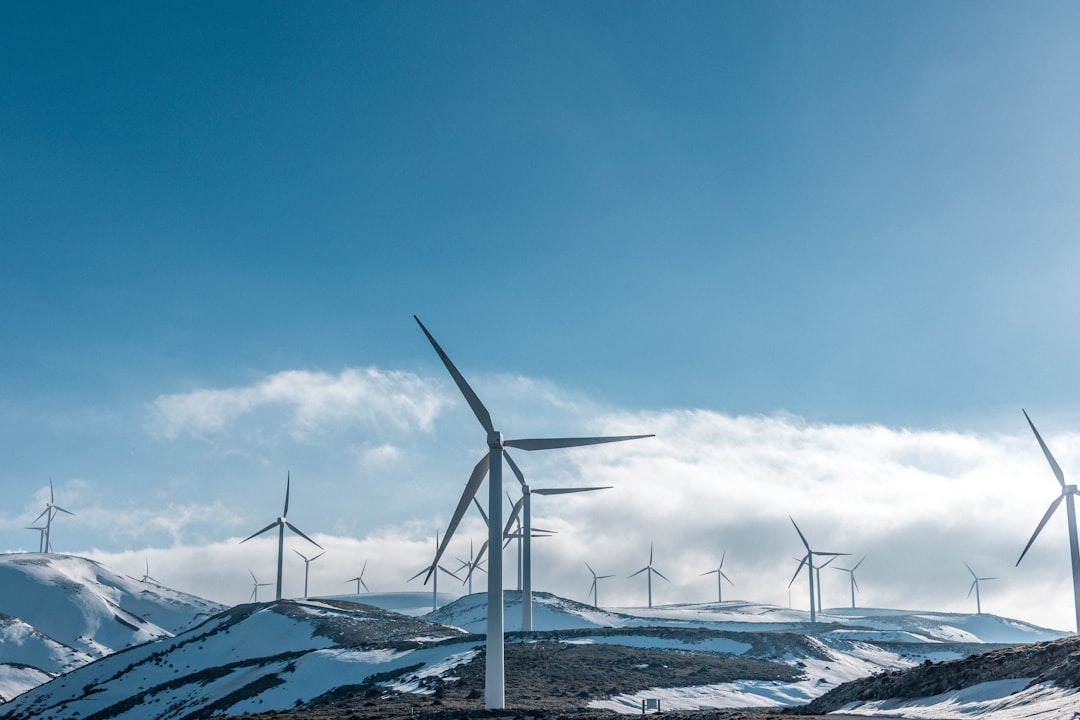
Machine learning (ML) offers potential for predicting long-term environmental consequences, a vital component of environmental impact assessments (EIAs). With the ability to analyze massive datasets, ML models could reveal the long-term effects of human actions on the environment, allowing us to take a more informed and proactive approach to environmental management.
But there are hurdles. The massive amounts of energy needed to train these ML models raise concerns about their environmental impact. Plus, the accuracy of these predictions hinges on the quality and variety of the training data – biased datasets could lead to inaccurate assessments and ultimately, faulty decisions. As AI tools become more integrated into our environmental decision-making, we need to carefully assess their limitations and ethical implications.
The use of machine learning (ML) in environmental impact assessments (EIAs) is creating a revolution in how we analyze environmental data. ML algorithms can now analyze vast amounts of data at an unprecedented speed and scale, allowing us to spot subtle changes in vegetation patterns or land use over large regions that were previously missed by human analysis. Some ML models even go a step further, by integrating diverse data sources such as satellite imagery, climate models, and socioeconomic data, to give a more comprehensive picture of the potential environmental impacts and risks of development projects.
Reinforcement learning, which uses simulated environments, has emerged as a fascinating development, allowing for more accurate predictions by running countless simulations before real-world applications. These simulations help us get a better handle on complex environmental interactions and anticipate potential problems more effectively.
A significant advancement is the development of explainable AI techniques, which demystify complex models. This transparency is crucial for building trust in the AI-driven environmental assessments, as it allows engineers and decision-makers to understand how the predictions are being generated, and what factors are driving the outcomes.
While exciting, we are also facing some surprising challenges with ML in EIAs. The sheer scale of environmental data often comes with inconsistencies and gaps, which can hinder the accuracy and effectiveness of the models for long-term predictions.
Research shows that ML models can significantly outperform traditional statistical techniques in predicting environmental outcomes, uncovering hidden non-linear relationships that often go unnoticed by conventional methods. This is an important area of development, as it allows us to better grasp complex environmental dynamics.
However, we need to remain cautious. There is a real risk that ML models, when trained on biased datasets, will perpetuate historical inequalities. Therefore, meticulous data audits and ensuring diversity within the training datasets are critical for ensuring fair and unbiased predictions for all communities, especially for marginalized communities who are often disproportionately impacted by environmental changes.
It’s important to acknowledge the significant energy cost associated with training large-scale ML models. The carbon footprint for a single year's worth of training can be substantial, often comparable to the emissions from multiple cars driven for an entire lifetime. This raises ethical questions about the environmental impacts of developing and deploying these models.
Federated learning is an emerging technique offering potential solutions to data privacy and sovereignty concerns. This approach enables multiple institutions to collaborate on training models while maintaining data decentralization, which is crucial for Indigenous communities who are often deeply concerned about the control and ownership of their data.
As ML continues to evolve, we can expect to see the development of real-time environmental monitoring systems that provide instant feedback on environmental changes. This enhanced capacity will allow for more proactive responses to unforeseen consequences of development projects, ensuring we are better prepared to address emergent environmental challenges.
Analyzing AI's Role in Environmental Impact Assessments Lessons from Standing Rock - Balancing AI Efficiency with Human Expertise in Impact Assessments
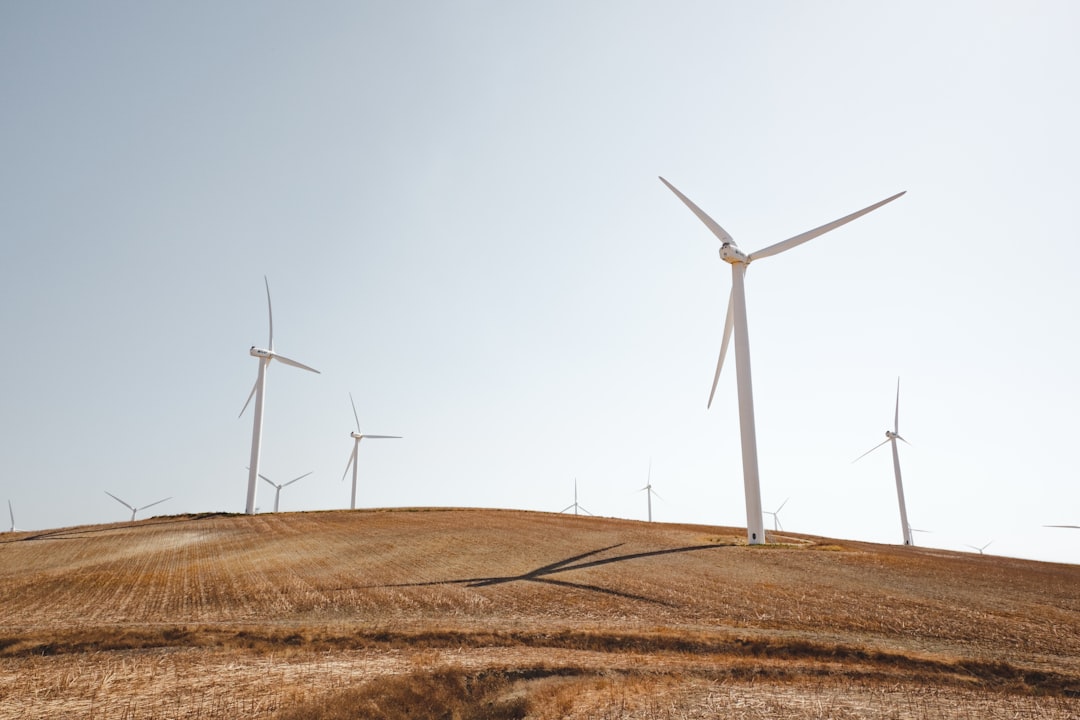
The application of AI in environmental impact assessments presents a fascinating dilemma: how to balance its efficiency with the need for human expertise. While AI excels at processing vast amounts of data quickly, it's limited by its reliance on historical data, which can contain biases that skew the results. This can lead to inaccurate assessments and ultimately, detrimental environmental decisions. Adding to the complexity, training AI models demands substantial energy, raising concerns about their own environmental impact. The events at Standing Rock serve as a stark reminder that incorporating human judgment and ethical considerations alongside AI-driven insights is essential. Only through a thoughtful blend of AI and human expertise can we ensure that environmental assessments are both accurate and fair.
The integration of AI into environmental impact assessments (EIAs) is changing how we analyze and understand the environmental effects of development projects. It can speed up the assessment process from months to days or even hours, a dramatic change in efficiency. AI algorithms are also able to detect complex interdependencies in environmental data that might escape human analysts, potentially shifting how we understand the consequences of human actions on the environment.
However, there are significant challenges that need to be addressed before AI can truly live up to its potential in environmental assessment. AI models need extensive datasets to function, and if those datasets lack diversity and representation of different geographic and social contexts, they can lead to assessments that fail to capture local environmental realities.
Despite these concerns, AI shows a lot of promise. Empirical studies demonstrate that advanced machine learning techniques can accurately predict environmental outcomes with greater than 90% accuracy, surpassing traditional statistical methods. This accuracy, combined with the speed of processing, could be transformative for environmental decision-making.
However, the complexity of many AI algorithms presents a challenge – the lack of transparency known as the “black box” problem – making it difficult to understand how the models arrive at their conclusions and diminishing public trust in the results. Thankfully, recent innovations in explainable AI are aiming to address this issue, making it possible for stakeholders to understand how AI models generate their findings. This is critical for building trust and collaboration between technical experts and local communities.
We can't ignore the significant environmental impact of training large AI models, which can consume vast amounts of energy. Estimates suggest that training a single model can have a carbon footprint equivalent to five cars driven for their entire lifespans. This raises questions about the environmental sustainability of these technologies and reinforces the need to develop responsible, sustainable AI.
There are signs of hope. Federated learning offers a potential solution by allowing for the collaborative training of AI models without the need to centralize data, making it a potential solution to data sovereignty issues. This could be particularly important for Indigenous communities who are concerned about the control and ownership of their data.
AI's ability to utilize remote sensing data allows it to monitor environmental changes at unprecedented scales, enabling early detection of environmental disturbances that might otherwise go unnoticed. This is particularly relevant for monitoring environmental changes and predicting potential future issues.
Despite its potential, the use of AI in environmental assessment requires careful consideration and oversight. Without rigorous evaluation and attention to data bias, AI-driven assessments may perpetuate environmental injustices faced by marginalized communities. As with any powerful tool, its implementation must be carefully monitored and managed to ensure it is used ethically and responsibly for the benefit of the environment and all communities.
Automate legal research, eDiscovery, and precedent analysis - Let our AI Legal Assistant handle the complexity. (Get started for free)
More Posts from legalpdf.io: