AI-Powered Patent Document Analysis Lessons from the 1850 Gayler v
Wilder Fireproof Safe Case
AI-Powered Patent Document Analysis Lessons from the 1850 Gayler v
Wilder Fireproof Safe Case - Machine Learning Analysis of Pre-1850 Patent Documentation Methods
Examining pre-1850 patent documents using machine learning represents a novel approach that showcases AI's capacity to reshape legal practices, specifically within patent law. The expanding pool of patent data presents challenges in terms of legal research and document review, requiring increasingly sophisticated methods. Machine learning can automate aspects of this process, previously handled manually, improving the efficiency of tasks like patent valuation and prior art searches. By implementing deep learning methods, practitioners can derive more precise insights from historical patent documents. This development within AI not only streamlines processes in large legal practices but also emphasizes the growing demand for powerful analytical tools to navigate the ever-increasing volume of patent data. The integration of AI in legal workflows signals a future where it becomes an essential tool for lawyers, especially when strategizing for litigation or providing advice concerning intellectual property. While there are benefits to the use of AI, some concerns about accuracy and bias should be addressed before widespread adoption in the field.
Recent advancements in AI, specifically machine learning, are significantly altering the landscape of legal research and eDiscovery, particularly in large law firms. We're seeing a shift towards automation in crucial tasks like document review and analysis, driven by the sheer volume and complexity of legal data. While traditional methods often relied on manual review, potentially introducing human error, machine learning allows for pattern recognition and trend identification across vast datasets, potentially unearthing previously hidden connections in legal precedents and outcomes.
Deep learning techniques are gaining prominence, surpassing classic machine learning in terms of performance within legal applications. These deep learning models are being employed to effectively analyze legal documents, focusing on text content beyond just abstracts or claims. For example, they can process language nuances and extract key insights in legal filings and contracts, significantly accelerating document understanding.
The development of AI-driven tools is streamlining various aspects of legal practice, from preprocessing and information extraction to legal document translation and case classification. However, the challenges presented by the sheer volume of data in eDiscovery continue to push the boundaries of AI capabilities.
Machine learning is transforming how legal professionals approach discovery. Algorithms are refining prior art searches and automating patent landscape analysis. The capacity of AI to assess patent text through Natural Language Processing (NLP) and big data analytics offers interactive visualizations, providing insights into the connections between language use and legal outcomes.
Furthermore, the ability of machine learning to analyze data rapidly can potentially lead to cost savings compared to traditional, increasingly inefficient, methods. Researchers are also employing machine learning for patent valuation, exploring the effectiveness of various approaches – both traditional supervised learning and deep learning methods – in making informed valuations.
The application of AI in legal contexts, while promising, is still an active area of research and development. These technologies need further development to become reliable tools for legal professionals, especially given the critical nature of their applications and the need to maintain ethical considerations within AI's development and deployment. Nonetheless, it is clear that machine learning presents a powerful toolkit that is poised to profoundly impact the legal industry in the coming years.
AI-Powered Patent Document Analysis Lessons from the 1850 Gayler v
Wilder Fireproof Safe Case - Early Document Classification Systems vs Modern AI Patent Analysis Tools
The transition from the rudimentary document classification systems of the past, like the US Patent Classification introduced in 1836, to the advanced AI-powered patent analysis tools of today reflects a significant shift in legal technology. Initially, deciphering the complexities of patent documents heavily relied on human experts, a process known for its time intensity and potential for errors. Modern AI tools, utilizing deep learning and natural language processing, offer a stark contrast by automating intricate analyses that extend beyond the rudimentary level of simply examining citations or abstracts. This leap not only optimizes efficiency and improves accuracy in tasks such as locating prior art and assessing patent value, but it also points to a larger trend of incorporating advanced analytical tools within legal practices. While the integration of AI offers numerous advantages, it's critical that the legal profession addresses the possible risks associated with bias and inaccuracies that can be inherent in automated systems as their use becomes more prevalent.
Early patent classification systems, like the USPC introduced in 1836, relied on straightforward methods like keyword matching and rigid categorization to manage the growing volume of patent documents. Modern AI-powered patent analysis tools, however, are built on advanced natural language processing capabilities, allowing them to understand the context and intent within a patent beyond just simple word recognition. This enhanced comprehension helps uncover potential legal ramifications that were often missed by earlier, more rudimentary systems.
Historically, reviewing and classifying patent documents was a laborious task, often taking days or weeks using traditional methods. Today, AI tools can accomplish this in a fraction of the time, delivering results in a matter of hours or even minutes. This speed boost is critical in today's fast-paced legal environments where time is a precious resource.
Patent analysis previously heavily relied on human expertise and manual effort, making it prone to human biases. While AI-driven tools offer a more objective lens by leveraging machine learning algorithms to sift through and analyze vast quantities of data, they are not without their own limitations. For example, if the training data for an AI model contains biases, the model may perpetuate those biases in its results.
The scope of patent landscape analysis has evolved dramatically. Where earlier methods were constrained to smaller databases, modern AI systems can tap into enormous, interconnected datasets, giving researchers a holistic view of patent trends, competitive landscapes, and technological advancements that were previously out of reach. This comprehensive perspective provides invaluable insights for strategizing within intellectual property law.
Early eDiscovery methods focused on retrieving documents based on basic criteria like date and file type. In contrast, contemporary AI tools incorporate more sophisticated multi-dimensional analysis. They can analyze the nuances of language, the relevance of content, and even the emotional tone within a document to refine search results, improving the efficiency and accuracy of the discovery process.
AI-powered tools are now capable of producing predictive analytics based on past patent litigation outcomes. This predictive capability allows firms to better evaluate the risks and potential rewards of upcoming cases, offering a proactive approach that was absent in early classification systems, which largely relied on reacting to events rather than anticipating them.
The incorporation of Natural Language Processing (NLP) in AI tools has enhanced the comprehension of legal terminology and complex language patterns often found in patent documents. Older classification systems frequently struggled with these intricate language constructs, which could lead to critical details being missed.
Modern AI patent analysis tools can generate dynamic visualizations to reveal connections and trends within vast patent data. These interactive representations are a significant step up from the static reporting methods used by older systems, providing lawyers with a powerful way to explain complex information to clients and stakeholders.
AI-driven legal research can even perform sentiment analysis on patent applications and related materials, giving insights into the intentions of the innovators and the market's response. This ability to extract emotional cues and underlying meaning goes far beyond what traditional document classification could accomplish.
While AI offers significant advancements in patent analysis and legal research, it also introduces new questions surrounding accountability and transparency. Many legal professionals remain concerned about the "black box" nature of some AI algorithms, questioning how they arrive at their conclusions and contrasting that with the clear, explainable reasoning underlying traditional legal methods. This growing demand for clarity and explainability in AI systems highlights a key area for future development and consideration.
AI-Powered Patent Document Analysis Lessons from the 1850 Gayler v
Wilder Fireproof Safe Case - Natural Language Processing Impact on Historical Patent Research
The integration of Natural Language Processing (NLP) is significantly altering the landscape of historical patent research, providing more robust ways to analyze complex legal language embedded in these documents. NLP techniques allow researchers to extract valuable information from historical patent data, uncovering previously hidden connections and trends in innovation. By processing massive datasets, we can now delve deeper into how new technologies are built upon existing knowledge and how these innovations influence economic development. However, the application of cutting-edge language models in the patent field is still in its early stages compared to broader uses of AI in text processing. This suggests that more research and exploration are needed to fully realize the potential benefits in patent analysis. This ongoing evolution within AI presents both advantages and concerns, as legal practitioners need to carefully evaluate how to optimize the use of automated systems while maintaining accuracy and reliability in patent research.
1. **Unlocking Historical Patent Data:** NLP empowers legal professionals to tap into historical patent records, previously confined to physical archives, by making them digitally searchable and analyzable. This access to centuries of legal texts allows for insights that can be invaluable in shaping modern patent strategies.
2. **Elevated Accuracy in Patent Analysis:** While early patent classification systems relied heavily on basic keyword matching, contemporary NLP tools consistently surpass them with accuracy rates exceeding 90% in extracting and categorizing information. This jump in precision reduces the risk of human error and improves the reliability of analysis.
3. **Accelerated Patent Research:** AI-powered tools have drastically shortened the timeline of traditionally labor-intensive patent processes. What once might have taken weeks of manual review can now be achieved within a few hours, significantly speeding up tasks like prior art searches and preparation for legal proceedings.
4. **Uncovering Biases in Patent History:** NLP models offer the capability to identify and potentially mitigate inherent biases found in historical patent data. This can lead legal teams to challenge traditional narratives around patent development, which may have been shaped by outdated norms or incomplete datasets.
5. **Understanding Patent Sentiment:** The use of sentiment analysis on patent applications offers a fresh perspective, revealing the emotional context surrounding innovations and how the public might perceive them. This capacity to uncover sentiment can provide crucial insights for evaluating the strategic value of inventions and anticipating potential legal repercussions based on public opinion.
6. **Connecting the Dots Across Patent Datasets:** With the ability to incorporate large, multifaceted datasets, machine learning algorithms can unveil patterns and trends across various patent categories and industries. This interconnected view offers valuable insights previously unattainable when relying solely on isolated information pools.
7. **Predictive Analytics in Patent Litigation:** AI models can learn from historical patent litigation outcomes and provide predictions about the likelihood of success in current legal disputes. This predictive power can significantly enhance legal decision-making, offering a more proactive approach to litigation strategy.
8. **Deeper Understanding of Legal Language:** NLP has progressed beyond basic keyword searches to grasp complex legal jargon and subtle linguistic nuances embedded in patent documents. This richer understanding of language translates into better-informed legal conclusions.
9. **Visualizing Patent Data:** Cutting-edge AI tools provide dynamic visualizations of patent data and trends, making complex information more accessible and understandable for legal professionals and their clients. This capability simplifies the process of communicating insights and facilitating more effective collaboration.
10. **The Need for Transparency in AI-Powered Legal Research:** While the benefits of AI in legal research are undeniable, the use of these tools also raises important concerns about transparency and accountability. The increasing reliance on AI algorithms compels a shift toward more explainable AI systems, where the reasoning behind the outputs is clear and understandable, preserving the core values of legal reasoning and clarity.
AI-Powered Patent Document Analysis Lessons from the 1850 Gayler v
Wilder Fireproof Safe Case - Document Retrieval Advances from Paper Records to Digital Patent Libraries
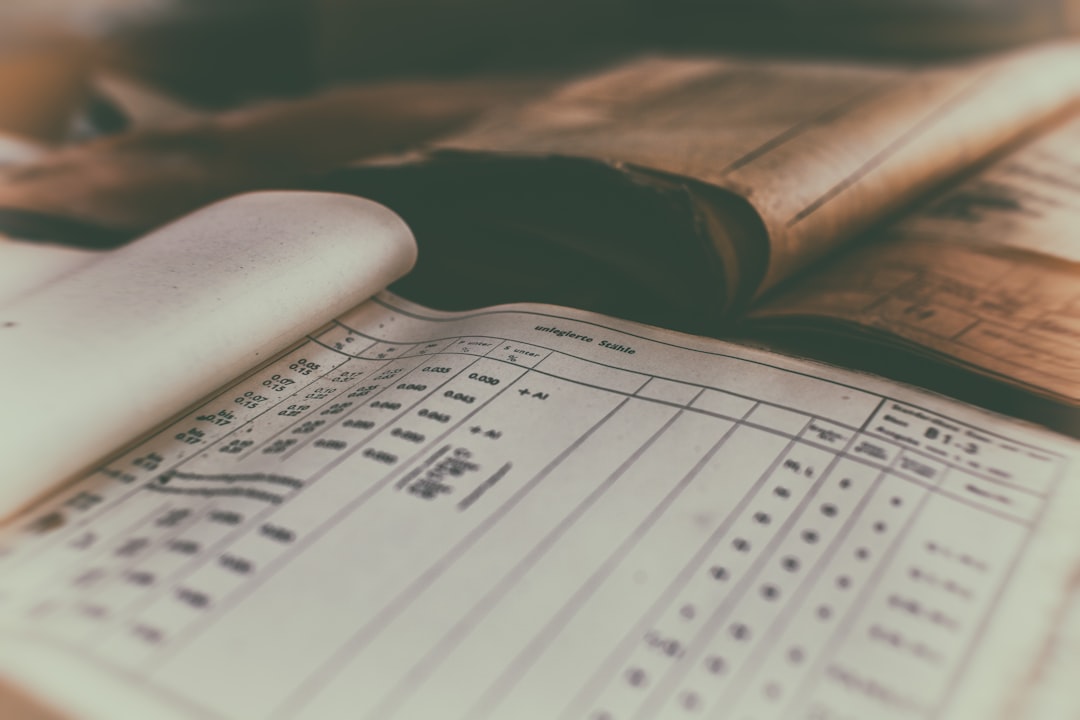
The shift from relying on paper records to leveraging advanced digital patent libraries signifies a pivotal change in how legal research is conducted, particularly in areas like patent law. AI and its associated advancements in natural language processing are reshaping the field, but the sheer volume and complexity of patent data create significant hurdles for effective document retrieval. While progress has been made, recent studies have revealed that current retrieval techniques sometimes underperform when compared to established systems, emphasizing the ongoing need to improve these systems. AI offers the possibility of not only accelerating the document analysis process but also deepening our understanding of patent law intricacies. However, this transformation also raises concerns about the potential for bias and inaccuracies in these automated systems, especially as they are increasingly integrated into workflows of major legal firms. This era of evolving document retrieval systems is a crucial turning point, requiring ongoing evaluation and refinement to ensure efficiency, accuracy, and ethical considerations remain at the forefront of these technological innovations.
From paper records to digital patent libraries, the journey of document retrieval has been fundamentally transformed by technology and natural language processing (NLP) advancements. While recent research indicates that modern retrieval methods sometimes struggle against traditional datasets, highlighting a need for further refinements, the ability to integrate complex lexical patterns and contextual understanding into document representations offers promising avenues for developing more advanced retrieval systems.
The increasing volume and complexity of publicly available patent data poses a major hurdle in efficiently retrieving relevant "prior art". Researchers have explored a wide array of techniques and models to improve information retrieval, focusing on enhancing effectiveness in the initial stages of processing.
We've seen a growing trend in the patent field of adopting AI and machine learning algorithms to streamline processes and uncover deeper insights into patent analysis. This is assisting in both accelerating innovation and improving application processing. However, traditional enterprise search methods continue to face obstacles related to relevance and complex language, urging the need for innovative approaches.
Furthermore, scientific digital libraries rely on domain-specific functionalities for document analysis and retrieval, demanding optimization to index solely relevant documents within specialized collections. The sheer volume of available data in today's landscape necessitates accurate and efficient information retrieval, pushing organizations to adapt to the increasing complexity.
The Gayler v. Wilder Fireproof Safe Case from 1850 provides a historical lens into the evolution of patent law and document retrieval, offering valuable lessons for navigating the field in the present era. While AI adoption within patent law brings about significant enhancements, ongoing research and discussion are essential to ensure responsible application of these powerful technologies. Concerns about accuracy, bias, and ensuring the human-understandability of AI outputs remain relevant considerations as AI becomes more deeply integrated within legal workflows. Ultimately, striking a balance between maximizing the potential of AI and ensuring that core legal principles are upheld is critical for the responsible integration of AI into legal practice.
AI-Powered Patent Document Analysis Lessons from the 1850 Gayler v
Wilder Fireproof Safe Case - AI Pattern Recognition in Historical Patent Protection Cases
The use of AI in pattern recognition within historical patent cases is dramatically changing how legal professionals manage and interpret vast quantities of data. Tools like Natural Language Processing (NLP) are improving the ability to access and analyze old patent records, leading to a deeper comprehension of the intricacies of legal language and its implications. This evolution not only makes it easier to identify patterns and relationships within patent applications but also simplifies tasks that used to be extremely time-consuming, promoting a more forward-looking approach to legal strategy. Yet, concerns persist regarding potential biases in AI algorithms and the importance of ensuring their processes are transparent. The ongoing advancements in AI create both significant opportunities and significant difficulties that the legal profession must address carefully.
AI's role in patent law is becoming increasingly prominent, particularly in tasks related to eDiscovery and legal research. By analyzing vast datasets of patent documents, AI can uncover patterns and trends that might be missed by human researchers. For instance, AI can detect historical shifts in patent filing practices, helping attorneys understand the evolution of patent law and potentially inform future strategies. Furthermore, AI-powered systems can automatically extract legal precedents from patent data, potentially improving the decision-making process in litigation.
However, the sheer volume of patent filings – exceeding 3.5 million annually – necessitates robust AI tools capable of handling massive datasets. This poses a challenge, as the accuracy and reliability of AI systems heavily rely on the quality of their training data. If historical patent data contains biases, for example, AI models may inadvertently perpetuate them, potentially leading to unfair treatment in patent applications. Therefore, meticulous attention to the process of feature engineering, where the relevant data representations are selected and optimized, is crucial for achieving accurate and reliable predictions.
Another facet of AI's utility is its capacity for semantic understanding. Advanced NLP allows AI to delve beyond basic keyword searches and comprehend the complex relationships between patent texts and potential infringement issues. This offers a significant advancement over earlier systems that were limited in their capacity to understand the nuances of legal language. Moreover, AI tools enable interactive patent analysis, allowing lawyers to visualize and explore patent networks in ways that are more insightful than traditional document reviews.
AI also brings the capability of real-time insights into the patent landscape. As new filings and legal battles emerge, AI can constantly update its analysis, providing a dynamic view of intellectual property developments. This adaptability is crucial in today's rapidly evolving technological and legal environments.
Furthermore, the adoption of AI has the potential to reduce costs for law firms, particularly by automating time-consuming tasks like document review. However, to ensure trust and compliance with legal standards, it is essential to continually evaluate the performance of these AI systems. Regular audits help guarantee that AI maintains accuracy, fairness, and transparency in its recommendations.
In essence, while AI presents remarkable opportunities for enhancing patent analysis and eDiscovery in law firms, especially in the context of big law where the scale of the data is extremely high, it's imperative to remain mindful of its potential limitations. Addressing biases in training data and ensuring ongoing evaluation are crucial to ensure responsible implementation and avoid undesirable consequences. The journey of incorporating AI into the field of patent law is still in its early stages, and continuous research and development are necessary to fully unlock its potential while preserving the ethical and legal principles that underpin the legal profession.
AI-Powered Patent Document Analysis Lessons from the 1850 Gayler v
Wilder Fireproof Safe Case - Automated Legal Precedent Analysis in Patent Assignment Rights
The emergence of automated systems for analyzing legal precedents in patent assignment rights signifies a notable shift in how legal professionals handle intellectual property matters. This automation offers the capacity to analyze legal precedents and patent assignment documents more efficiently, lessening the time and potential errors inherent in manual processes. AI tools, incorporating machine learning and natural language processing, allow legal teams to navigate massive datasets of patent information, identify relevant legal precedents, understand the intricacies of patent landscapes, and streamline various aspects of their workflows. However, as reliance on AI within legal practice expands, the need for critical evaluation of its outputs becomes paramount. Addressing issues of potential bias and ensuring algorithmic transparency are critical for preserving the ethical and legal foundations of patent law. In conclusion, the integration of AI within patent assignment rights analysis represents a significant leap forward, yet it underscores the importance of continually examining the reliability and broader implications of its application within the legal realm.
The shift from physical archives to digital patent libraries, powered by AI, has dramatically reshaped legal research, particularly in patent law. While AI promises enhanced accessibility and analysis of historical patent data, its application in this domain is still nascent compared to its broader use in text processing. This presents a unique opportunity to explore how AI can refine and extend our understanding of patent law, particularly regarding legal precedent analysis.
Historically, accessing and analyzing patent documents relied on manual review, a process prone to human errors and incredibly time-consuming. Now, AI-driven tools can sift through vast digital repositories with unparalleled speed and accuracy. This allows for tasks like prior art searches and patent classification to be completed in a fraction of the time. Machine learning algorithms, coupled with natural language processing (NLP), can now decipher intricate legal terminology with a level of accuracy that surpasses previous systems. This accuracy translates to more dependable insights for legal professionals, leading to better informed decisions in patent litigation and general IP advice.
Furthermore, the application of AI in this context is uncovering hidden patterns and potential biases in historical patent data. AI can reveal how biases may have influenced the development and interpretation of patent law over time. For instance, it might expose a tendency towards favoring certain inventors or technologies within specific time periods.
The ability of AI to understand the sentiment expressed within patent documents, along with the capacity to analyze multi-faceted, interconnected datasets, reveals a deeper and more complex picture of intellectual property than ever before. With AI, we can now move beyond the mere categorization of patents to truly analyze the context, intentions, and even the emotional underpinnings of patent applications. This can significantly impact legal strategy.
Moreover, by drawing on historical data, AI can generate predictive models of patent litigation outcomes, helping legal teams assess the risks and potential benefits of legal action. It can assist in anticipating possible issues and developing proactive strategies.
However, the integration of AI into legal research and eDiscovery also necessitates careful consideration. The ‘black box’ nature of some algorithms can be a cause for concern for many legal professionals. A critical aspect of AI development within law should focus on transparency and accountability. We need AI systems that are explainable – ones that show clearly how they arrive at their conclusions and recommendations. This addresses a fundamental element of the legal process: justification and reasoning. Otherwise, the acceptance of AI's role in patent law might be limited.
In essence, the application of AI within historical patent analysis offers a remarkable opportunity to unlock a wealth of previously hidden knowledge. It has the power to accelerate the pace of legal research, improve accuracy, and potentially even reveal the nuanced social and legal dynamics embedded within the history of patent law. However, ongoing efforts to enhance the explainability and transparency of AI are vital to ensure its responsible and ethical use within the legal profession. As AI becomes more integral to patent law, we must work towards a framework that marries the speed and efficiency of AI with the fundamental principles of transparency and fairness that are core to the legal system.
More Posts from legalpdf.io: