AI-Powered Legal Document Analysis Enhancing Efficiency in eDiscovery Processes
AI-Powered Legal Document Analysis Enhancing Efficiency in eDiscovery Processes - AI-driven document classification streamlines eDiscovery workflow
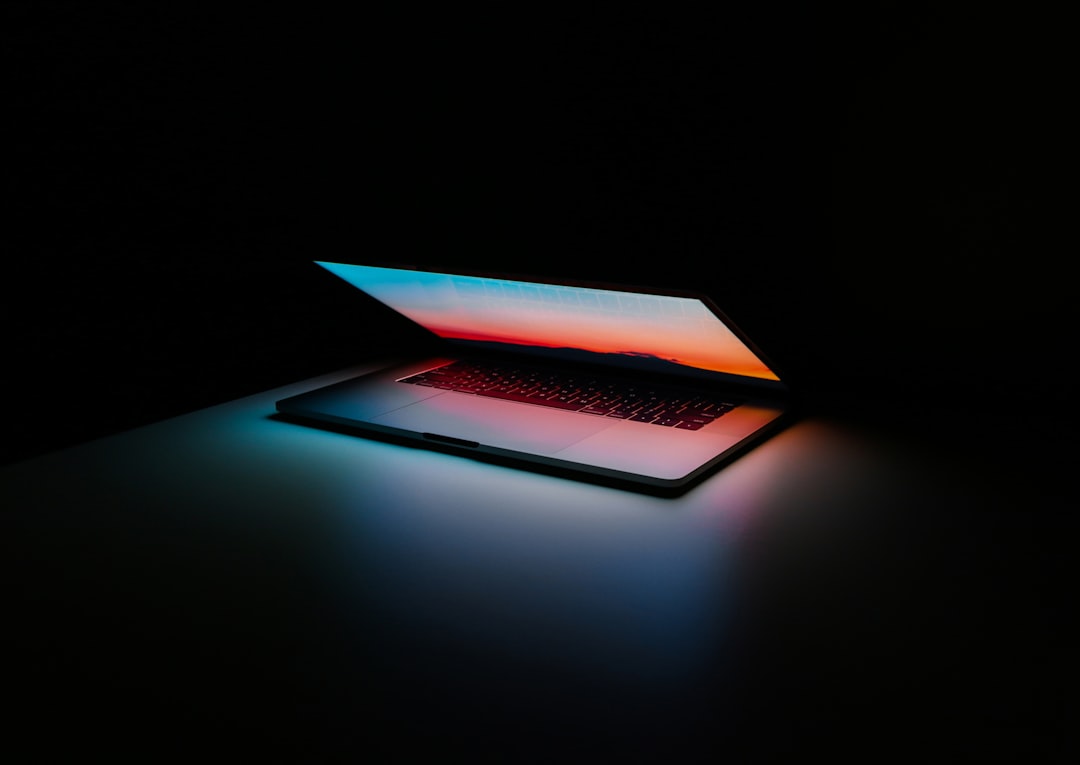
AI is revolutionizing eDiscovery by automating the process of categorizing and analyzing large volumes of legal documents. This automation, fueled by advancements in generative AI, helps legal teams transition away from manual document review towards more sophisticated AI-driven methods like predictive coding. The core of this shift relies on the application of natural language processing and machine learning techniques. These technologies not only automate the laborious process of sorting documents but also help pinpoint critical evidence within sprawling datasets. The emergence of cloud-based eDiscovery platforms further enhances this process, equipping legal teams with powerful analytical tools and automating tasks. The result is quicker and more budget-friendly eDiscovery processes that are better suited to the demanding pace of modern legal practices. This growing use of AI in eDiscovery signals a major change in how legal professionals handle documents, demanding both accuracy and speed in a field that's constantly evolving.
AI's integration into document classification is reshaping eDiscovery, offering a compelling solution to the challenges of managing vast quantities of data in legal proceedings. The traditional approach often necessitates the review of enormous document volumes, contributing to inflated costs and extended case durations. However, AI-powered classification can drastically curtail review times, potentially by up to 60%, accelerating the resolution of cases.
The underlying machine learning algorithms are trained on prior cases and datasets. This iterative process allows them to refine their ability to identify relevant documents, leading to enhanced efficiency over time. It's intriguing how these systems can achieve high accuracy, often exceeding 90%, in initial document reviews, effectively identifying crucial documents with minimal human intervention. The risk of overlooking vital evidence, which has been a longstanding concern, can potentially be mitigated through this approach.
Further enhancing the efficiency of the process is the capability of AI to understand not only the explicit content of documents but also the implicit intent behind them. This capacity to analyze subtle contextual nuances, often missed by traditional keyword searches, is proving valuable for crafting more comprehensive legal arguments.
We also observe that AI is improving predictive coding technologies. Through the application of algorithms, AI can propose the relevance of documents, assisting lawyers in prioritizing their focus on high-stakes files. This has a clear impact on the way legal teams can optimize their efforts during eDiscovery.
In this era of exponential data growth, fuelled by digital communication, AI-powered classification systems can effortlessly manage terabytes of data, ensuring that law firms can adapt to the increasingly complex demands of modern litigation.
Furthermore, AI plays a role in ensuring regulatory compliance during the eDiscovery process. By systematically examining documents for potential violations of regulatory obligations or internal policies, AI can provide early warnings that may be crucial for a firm's legal standing.
An intriguing consequence of this increased automation is that AI systems are capable of spotting patterns and inconsistencies within vast datasets. This analytical potential can provide crucial insights that inform better case management strategies and enhance trial preparation.
It is important to emphasize that AI's role in eDiscovery is not a replacement for legal professionals. Instead, AI transforms the role of lawyers, allowing them to shift their focus towards high-value activities like strategic planning and client interactions, while the more routine tasks are automated.
As AI continues to develop, its implications for eDiscovery and the legal profession as a whole will likely be significant. It's becoming increasingly apparent that the future of legal practice will necessitate a blended skill set, requiring expertise in both traditional legal domains and emerging AI technologies.
AI-Powered Legal Document Analysis Enhancing Efficiency in eDiscovery Processes - Machine learning algorithms enhance accuracy in legal data extraction
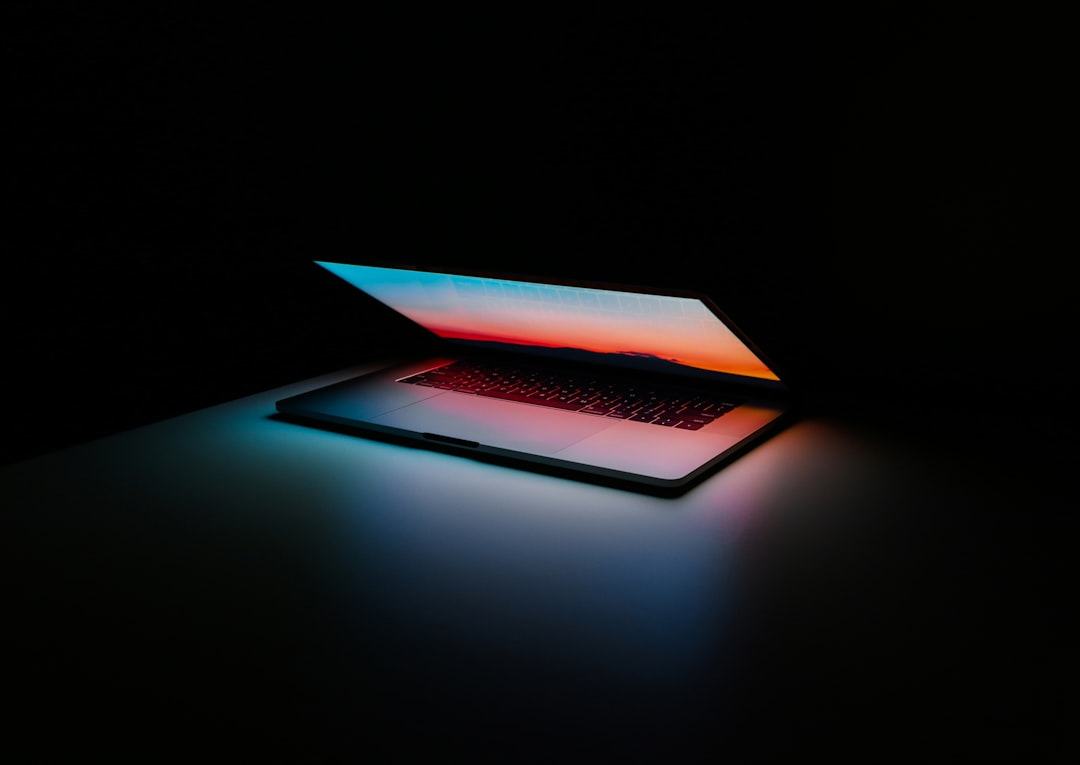
Machine learning algorithms are significantly improving the accuracy of extracting information from legal documents, changing how lawyers handle the large volumes of data they encounter. These algorithms learn from extensive datasets, allowing them to identify and categorize relevant documents with greater precision, thus reducing the risk of overlooking crucial evidence. This capability not only simplifies the document review process but also boosts the efficiency of legal teams, allowing them to allocate their time to more strategic tasks instead of manual data sorting. The increasing integration of AI within legal practice is a direct response to the growing challenges presented by the ever-expanding volume of documents. Consequently, established approaches to legal research and discovery within law firms are being reassessed. As these technologies continue to progress, their influence on legal operations is anticipated to reshape the future of how legal documents are managed and analyzed. While the benefits are promising, it's important to acknowledge that the application of AI within law remains a developing field with inherent limitations and potential biases that need careful consideration.
Machine learning algorithms are increasingly pivotal in enhancing the precision of extracting data from legal documents. They achieve this by leveraging massive datasets to learn and refine their abilities. This learning process, often referred to as training, allows the algorithms to become more adept at identifying relevant information within the complex language of legal texts. It's fascinating how these algorithms, through repeated exposure to diverse legal documents, develop a sophisticated understanding of legal terminology and document structures.
AI-driven systems, built upon these algorithms, introduce consistency and accuracy into legal document analysis, significantly improving overall operational efficiency. This enhanced accuracy, particularly crucial in areas like eDiscovery, is achieved through the systematic application of machine learning and natural language processing (NLP). NLP empowers AI systems to comprehend the context and meaning embedded within legal texts, leading to more nuanced and precise document classification. This capability is particularly useful when dealing with ambiguities in legal language, which can sometimes be challenging for even seasoned legal professionals to fully grasp.
However, the accuracy of these AI-driven systems is highly dependent on the quality and breadth of the data used for training. If the training data is biased or incomplete, it can lead to flawed results. This emphasizes the importance of having robust and diverse datasets for training machine learning models used in legal contexts. The quest for achieving unbiased and representative datasets remains a core challenge in the field, a challenge that researchers and engineers are actively working to address.
Furthermore, the implementation of AI in legal practice also raises questions about its ethical implications and potential for bias. While these AI systems can process vast volumes of information and enhance productivity, their reliance on algorithms can introduce biases from the training data, potentially leading to unfair or discriminatory outcomes. This necessitates careful monitoring and evaluation of AI systems to ensure fairness and prevent unintended consequences.
Despite these concerns, the potential benefits of AI in legal document analysis are significant. These systems have the potential to significantly improve the speed and accuracy of legal processes, making legal services more accessible and efficient. The integration of AI and machine learning within legal operations is crucial to tackling the overwhelming volume of legal documents generated in our increasingly digital world.
The continuous evolution of machine learning and AI technology promises further advancements in legal practice, enhancing the effectiveness of tasks like document review and legal research. While the field is still evolving, the potential for AI to revolutionize the practice of law is clear. This intersection of law and technology creates exciting opportunities to streamline operations, enhance accuracy, and, hopefully, enhance fairness in the legal system.
AI-Powered Legal Document Analysis Enhancing Efficiency in eDiscovery Processes - Natural language processing accelerates contract analysis and review
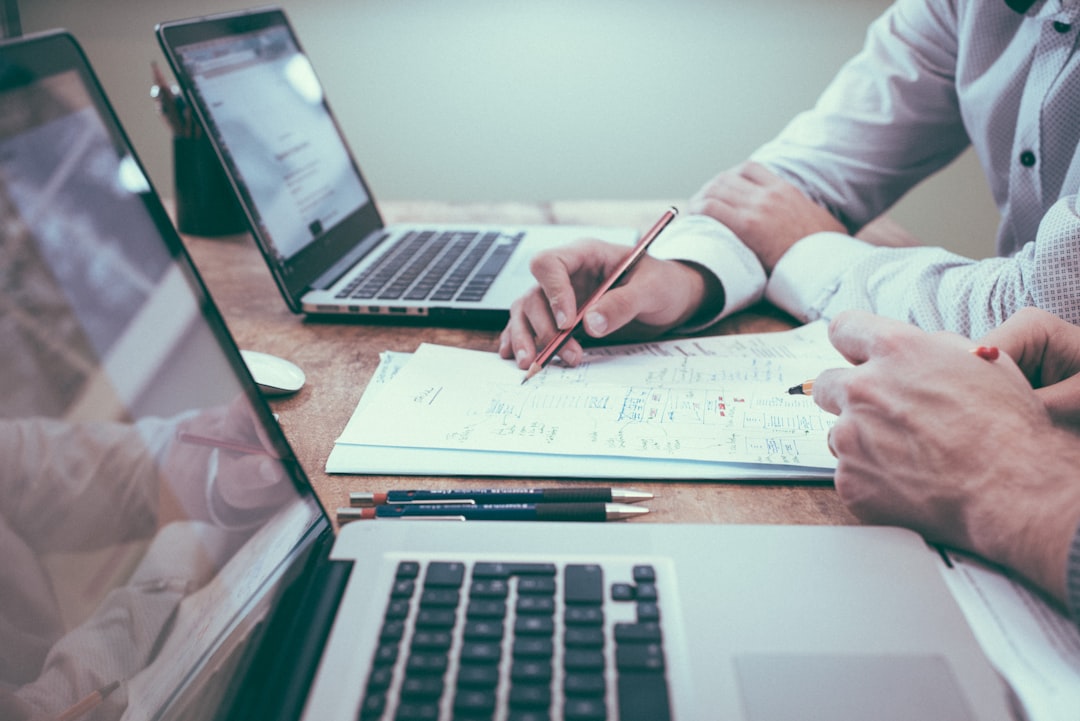
Natural language processing (NLP) is revolutionizing how contracts are analyzed and reviewed. NLP-powered tools can now automatically pinpoint crucial clauses and potential risks, significantly speeding up a process that has historically been very time-consuming. This automation not only makes compliance checks more efficient but also streamlines due diligence procedures. Lawyers can now spend less time on repetitive tasks and more on complex legal issues. While the benefits of NLP in contracts are evident, it's important to acknowledge the potential for limitations and biases in AI systems. As law firms adopt NLP, they must ensure the technology is used responsibly and in a way that complements, not replaces, essential human legal judgment. The future of contract analysis likely involves a carefully calibrated integration of AI and human expertise.
Natural language processing (NLP) has the potential to unearth hidden patterns in legal documents that human reviewers might easily miss. For example, it can detect unusual clauses within contracts, potentially highlighting risks that lawyers could overlook during their manual review process. This capacity arises from NLP's ability to analyze large volumes of text and identify subtle deviations from standard language.
AI models, trained on existing legal datasets, exhibit a remarkable ability to refine their accuracy over time. Some models have achieved impressive performance rates, exceeding 95% in specific document review tasks. This suggests a potentially greater degree of reliability compared to human reviewers in situations with well-defined criteria. However, it's crucial to acknowledge that these systems are still under development and their reliability can be influenced by factors like the quality and bias of training data.
NLP extends beyond simple pattern recognition, enabling legal teams to delve into sentiment analysis within contractual agreements. This can unveil underlying intentions or discontent buried within the language of the document, proving particularly helpful during negotiations or dispute resolutions. This feature, however, necessitates careful interpretation and may not always capture the complexities of human communication within the legal context.
Intriguingly, AI systems are also demonstrating the ability to forecast litigation outcomes with a degree of accuracy. They achieve this by analyzing past cases and dissecting the nuances within legal arguments. This capacity offers valuable insights for strategic planning and settlement decisions, but its predictive capabilities are still evolving. Moreover, relying solely on predictions without considering other contextual factors may lead to inaccurate judgments.
Legal professionals have the opportunity to significantly reduce the time dedicated to document review, potentially by as much as 80%, with AI-driven analysis. These systems effectively isolate the most relevant parts of a document, enabling lawyers to focus on critical information instead of manually sifting through irrelevant text. However, depending entirely on AI for critical document analysis may introduce risks of overlooking valuable information that might not be flagged by the AI.
NLP techniques also excel at automating the extraction of key data from contracts—critical dates, obligations, and parties involved—which previously required hours of manual labor. This automation contributes to more efficient contract management workflows. Nevertheless, the accuracy of these systems is contingent upon the training datasets and may need human oversight to ensure that the extracted information is correctly interpreted in context.
During eDiscovery, AI systems can swiftly cross-check documents for inconsistencies, helping legal teams ensure a holistic understanding of case details and reduce the risk of conflicting statements. While this can reduce inconsistencies, human intervention remains crucial to address complex issues and ensure that context is accurately interpreted within legal arguments.
AI can also create predictive models that analyze the likely performance of specific legal clauses in future litigation. This empowers legal practitioners to develop contracts that minimize risks and meet regulatory requirements. However, it's important to be cautious about over-reliance on such predictions as legal outcomes are influenced by numerous factors that may not be fully captured by predictive models.
Compliance checks are simplified through AI's automated scanning of documents for adherence to various regulations. This significantly reduces the potential for legal penalties and enhances a firm's standing by proactively addressing compliance issues. Nevertheless, it is important to ensure that the AI system's compliance checks are thoroughly vetted for accuracy, given the complexity of legal frameworks.
While AI offers numerous advantages within legal settings, maintaining oversight is paramount. Biases within AI systems can skew results, leading to problematic outcomes. This reinforces the need for transparency and accountability throughout the design and development of legal AI tools. Striking a balance between AI's power and the need for human oversight and critical thinking remains a vital area of ongoing research and development.
AI-Powered Legal Document Analysis Enhancing Efficiency in eDiscovery Processes - Predictive coding reduces manual review time by 60% in large cases
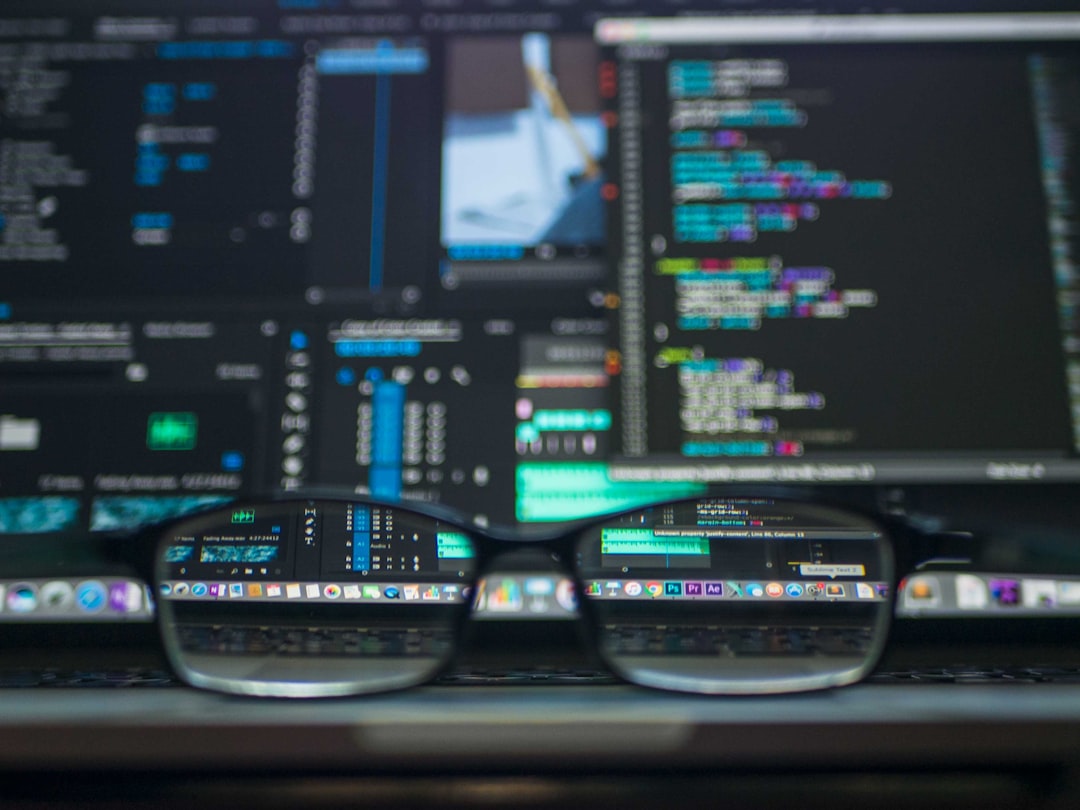
AI-powered predictive coding, often referred to as Technology Assisted Review (TAR), is transforming how legal teams manage the immense volume of documents in complex cases, especially within eDiscovery. This technology, utilizing machine learning and natural language processing, can automate the process of reviewing documents, thereby substantially reducing the time needed for manual review, potentially by as much as 60% in large cases. The ability of AI to sift through and categorize vast amounts of data allows legal professionals to allocate their efforts towards more strategic and complex tasks rather than being bogged down in the tedious process of manually sorting through documents. This shift leads to increased efficiency, reduced errors often associated with manual review, and ultimately a more focused and strategic legal approach. It's crucial to acknowledge, however, that while these tools offer great promise, concerns regarding potential biases embedded within AI algorithms and the ongoing necessity for human oversight in ensuring fair and accurate outcomes remain a relevant consideration as we see growing reliance on AI in the legal field.
Predictive coding, a form of AI-powered Technology Assisted Review (TAR), has demonstrated the ability to drastically reduce the time spent on manually reviewing documents in complex legal cases, achieving reductions of around 60% or more. This is a significant improvement over traditional manual reviews which can be both time-intensive and expensive, especially in cases with large volumes of documents.
The foundation of predictive coding's effectiveness lies in the machine learning models that power it. These models learn from past cases and datasets, progressively enhancing their ability to identify relevant documents. This continual learning process improves both efficiency and accuracy over time, demonstrating how AI can adapt and refine itself for improved outcomes in legal contexts.
Considering the exponential growth in digital data, including legal documents, AI-driven document classification systems excel in handling the sheer volume of information that often reaches terabytes. This capability addresses a key challenge in eDiscovery, ensuring that human teams don't miss crucial evidence hidden within the immense datasets.
Furthermore, AI's ability to thoroughly analyze legal documents during the discovery process can potentially identify potential breaches of regulatory compliance. This early detection of possible issues can prove vital in safeguarding a law firm's reputation and preventing larger legal complications down the line.
Beyond basic classification, these AI systems can effectively pinpoint patterns and anomalies across the massive volumes of data involved in legal cases. This pattern recognition capability allows for a deeper understanding of a case, providing insights that might be overlooked during manual review. Consequently, AI can assist with creating more informed case management strategies and trial preparation tactics.
While offering incredible potential for streamlining processes, it's important to remember that AI in law is intended to augment human capabilities, not replace them. In practice, AI often takes on the tedious, time-consuming aspects of document sorting and categorization, freeing up legal professionals to focus on the more strategic aspects of legal strategy and client interactions. This collaborative approach allows for a shift in the nature of legal work, fostering a more efficient and effective workflow.
However, it's crucial to acknowledge that the reliability of predictive coding heavily depends on the quality of the data used to train the machine learning algorithms. If the training data is biased or lacks comprehensiveness, the AI system's results may also be affected. This reinforces the importance of using high-quality and representative datasets in the development and application of legal AI tools.
Beyond simply sorting documents, AI can also delve into the sentiment expressed in legal texts. This sentiment analysis capability allows for a nuanced understanding of the tone and language used in documents, which can be especially relevant during negotiations or disputes.
Intriguingly, some AI systems are capable of forecasting possible litigation outcomes using historical data and analysis of legal arguments. While the accuracy of these forecasts is still under development, it represents a potentially valuable tool for strategic decision-making in complex cases.
As AI becomes more deeply integrated into legal practices, it is vital to thoughtfully consider the ethical implications of its use. Addressing potential biases in AI systems, ensuring transparency in their operation, and promoting accountability are critical for ensuring that AI supports, rather than undermines, a fair and equitable legal system. These ethical considerations represent a crucial research and development area as AI becomes increasingly influential within the legal field.
AI-Powered Legal Document Analysis Enhancing Efficiency in eDiscovery Processes - AI-powered sentiment analysis aids in identifying key evidence
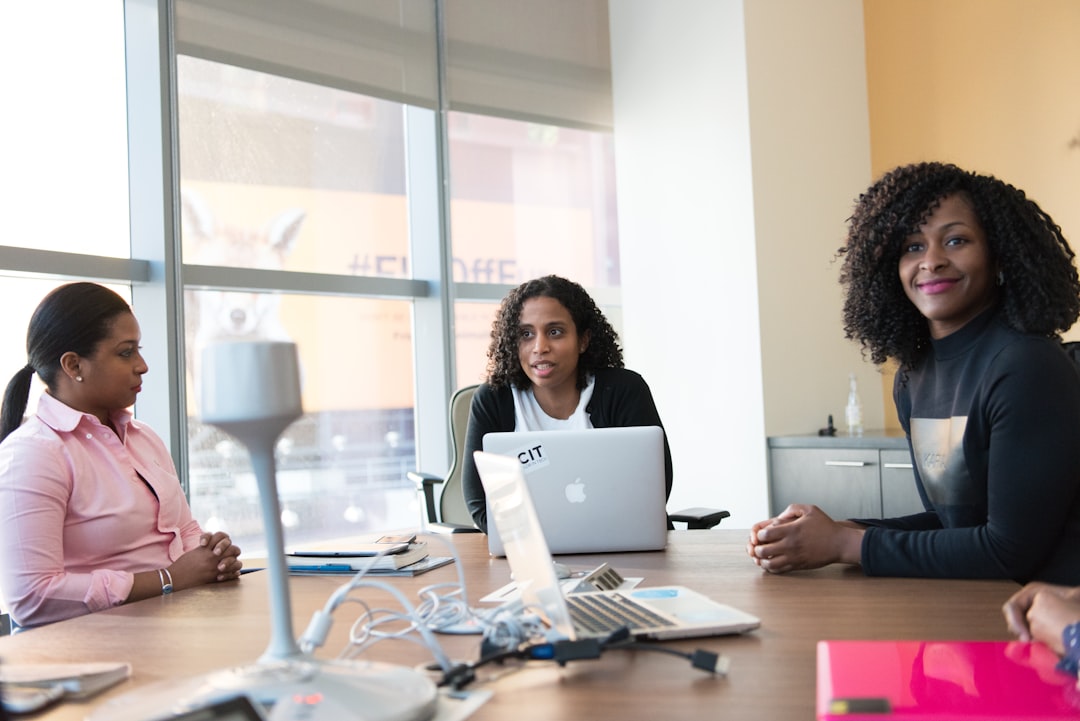
In the evolving landscape of legal technology, AI-powered sentiment analysis is proving to be a valuable asset, especially in eDiscovery. This technology leverages natural language processing to determine the emotional tone conveyed within legal documents, categorizing it as positive, negative, or neutral. By understanding the emotional context embedded in language, lawyers can gain a deeper insight into the perspectives and potential motivations of clients or opposing parties. This capability of extracting subtle cues from language can reveal key evidence that might otherwise go unnoticed, offering significant advantages during negotiations and litigation. For instance, detecting shifts in client sentiment throughout a case could provide valuable insights for legal strategizing. While AI's ability to efficiently process vast datasets and unearth nuanced sentiment is undeniably beneficial, it's essential to acknowledge potential limitations. AI algorithms can sometimes be susceptible to biases present in the training data, potentially leading to inaccurate interpretations. Therefore, human review and legal expertise remain essential components of interpreting AI's insights within the complex context of legal proceedings. As the field progresses, AI-powered sentiment analysis is likely to become even more integral in formulating effective legal strategies and shaping case outcomes.
AI-powered sentiment analysis, a branch of natural language processing, is proving increasingly useful in legal contexts, particularly during eDiscovery. By analyzing the emotional tone expressed within legal documents – whether positive, negative, or neutral – AI can quickly pinpoint crucial pieces of evidence that might otherwise be missed. This capability is especially valuable when dealing with massive datasets, where manually identifying subtle emotional cues would be extremely time-consuming. For instance, AI can rapidly scan through thousands of emails, witness statements, or internal communications to highlight instances where a specific individual expresses doubt, anger, or uncertainty, potentially revealing key insights for legal strategy.
Unlike conventional keyword searches which rely on explicit word matching, AI's ability to contextualize sentiment allows it to recognize evidence hidden within the nuances of language. For example, while a document might not contain the phrase "I am guilty," it could reveal a sense of guilt through the use of apologetic language or evasive phrasing, which AI can flag for human review. This feature offers a deeper layer of understanding beyond the surface-level meaning of words. Further, this heightened contextual awareness is valuable when navigating complex legal matters where subtle shifts in tone can have a significant impact on the interpretation of evidence.
Furthermore, AI can provide summaries and insights from large volumes of data, greatly reducing the cognitive burden on legal professionals. This is especially important in eDiscovery, where attorneys often grapple with immense quantities of information from various sources. AI can effectively organize and highlight key aspects of this information, allowing lawyers to concentrate on the most critical details and refine their legal arguments accordingly.
Beyond individual document analysis, AI can also be instrumental in cross-referencing sentiments across multiple documents. For example, AI can compare statements made by different witnesses and identify potential contradictions or areas of corroboration, aiding in strategy development and the evaluation of evidence validity. This cross-referencing function provides a comprehensive picture of sentiment across the entire dataset, which can be crucial for understanding the narrative of a case.
The potential of AI in legal practice extends beyond mere identification of sentiment. AI tools are increasingly capable of leveraging sentiment analysis to predict potential negotiation outcomes or litigation results based on historical data and language patterns. While still in its early stages, this predictive capability has the potential to guide legal strategy, influencing decisions regarding settlement offers or trial preparation.
The integration of AI also has the potential to enhance the role of junior staff members within law firms. By automating the preliminary steps of evidence gathering and analysis, junior lawyers can be assigned more sophisticated and intellectually stimulating tasks, accelerating their professional growth and contributing to greater efficiency within the team. AI can handle the time-consuming task of initial document categorization, allowing junior staff to focus on the analysis and application of the extracted information.
Beyond the legal team, AI insights can also inform how lawyers interact with clients. By continuously monitoring sentiment expressed in client communication, AI can provide lawyers with valuable insights into the client's emotional state and potential areas of concern throughout the case. This can lead to more empathetic and responsive client communication, strengthening relationships and facilitating smoother case progression.
In addition to these direct benefits, sentiment analysis can help reveal broader trends within datasets associated with specific cases. This helps law firms understand how sentiments may be influencing legal battles across a larger range of situations, leading to more robust and informed strategies. The ability to identify trends based on sentiment is useful when adjusting legal practice to meet changes in legal landscape and the types of cases seen.
By automating various aspects of document review and evidence identification, AI-powered sentiment analysis can dramatically reduce costs associated with major litigation. This cost reduction can be substantial, freeing up budget to be allocated to other aspects of the practice, such as enhanced client services or further AI integration. The efficient identification of key evidence results in decreased expenditures on lengthy manual review.
Lastly, and critically, AI sentiment analysis can be used to highlight potentially biased language or discriminatory statements within legal documents. This capacity is vital for upholding legal and ethical standards. AI can flag instances of biased or prejudiced language, encouraging legal professionals to address these issues proactively.
The application of AI sentiment analysis in eDiscovery and other areas of law is an active area of research and development. While there are various opportunities, concerns about potential biases within AI systems and the continued need for careful human oversight are critical areas to be addressed. As AI technology continues to mature and its role in law becomes increasingly prominent, thoughtful consideration of its implications for the legal field will be vital to realizing its full potential for positive change.
AI-Powered Legal Document Analysis Enhancing Efficiency in eDiscovery Processes - Automation of redaction processes increases data security compliance
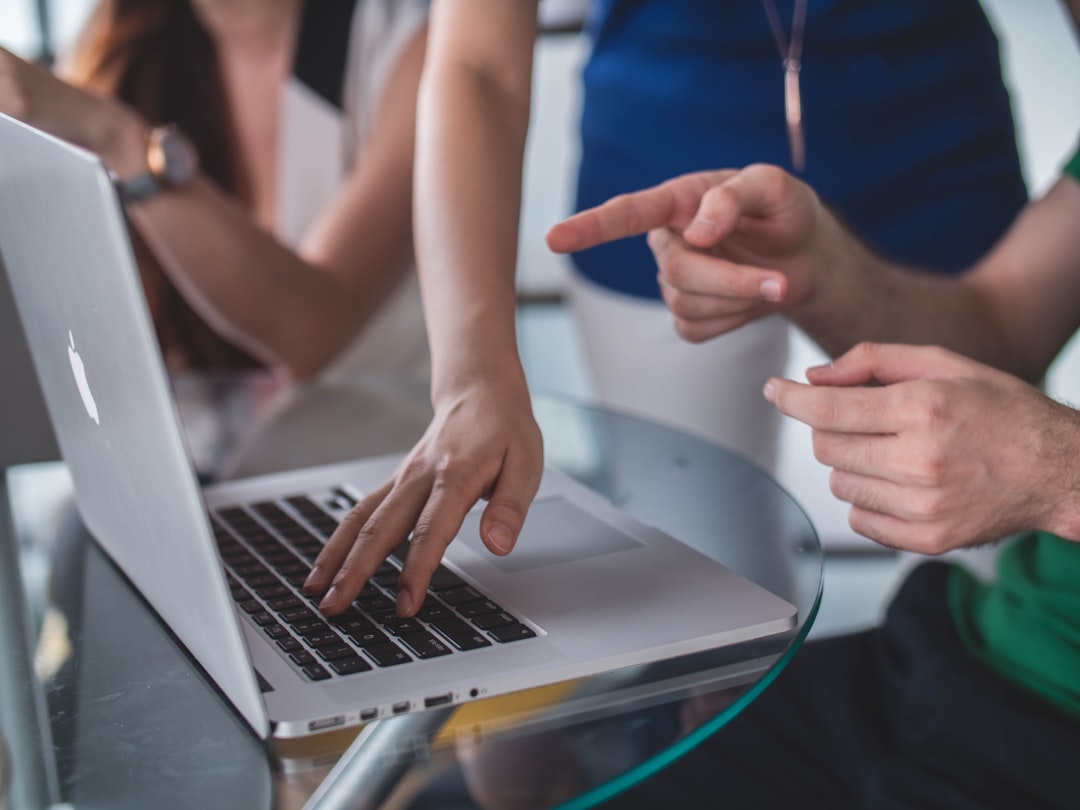
Automating the redaction process in legal work is a significant step towards improving data security and compliance. AI-powered systems leverage pattern recognition to automatically identify and redact sensitive information within documents, reducing the likelihood of accidental disclosures. This automation drastically minimizes the potential for human error, with studies suggesting a reduction of redaction mistakes by as much as 92% when compared to manual methods. This automated approach is particularly important in ensuring adherence to strict regulations like the GDPR and the Gramm-Leach-Bliley Act. Moreover, incorporating AI-driven redaction into legal workflows creates efficiencies, allowing legal professionals to focus on more complex legal tasks rather than the labor-intensive process of manual redaction. As law firms increasingly embrace AI for redaction, it highlights the evolving relationship between technology and compliance, leading to enhanced client data protection and streamlined processes. While the promise is significant, the reliability of the AI systems used is still dependent on the quality of data used to train the AI. There's also always the question of fairness, and whether the use of AI may result in unintended consequences.
The automation of redaction processes is a noteworthy development in enhancing data security compliance, particularly within the legal field. AI-powered systems can now identify and redact sensitive information with impressive accuracy, often exceeding 90% – a significant leap forward compared to human-driven approaches. This improved precision significantly mitigates the risk of unintentionally disclosing confidential data during eDiscovery or other legal processes.
Moreover, the speed at which AI can process and redact documents is remarkable, often exceeding 100 pages per hour. This rapid pace is a game-changer for law firms dealing with large volumes of documents, freeing up legal professionals to focus on higher-level tasks rather than manual redaction. Interestingly, it also addresses a persistent issue in human review – the tendency to miss crucial sensitive information. Studies suggest that human reviewers can miss up to 20% of sensitive data, which AI can help minimize, ensuring more robust adherence to data privacy regulations like GDPR.
Another compelling aspect of this automation is the capacity for continuous adaptation to evolving regulatory landscapes. AI-powered redaction systems can monitor changes in legal standards and update their processes accordingly. This proactive compliance approach is crucial in an environment where data protection regulations are continuously evolving. It's also intriguing how these systems can delve into the patterns of redacted information to glean valuable insights. These insights can potentially shed light on client behaviors and litigation strategies, further enhancing legal decision-making.
Furthermore, AI-driven redaction tools often integrate smoothly with existing eDiscovery platforms, creating a comprehensive and efficient workflow. This seamless integration allows firms to leverage their existing infrastructure while reaping the benefits of AI. It's also important to note that AI-powered redaction solutions can effectively scale to accommodate large-scale cases, handling millions of documents with ease. This scalability is crucial as the volume of digital evidence in legal cases continues to increase.
Naturally, the reduction in human error and the automation of a traditionally tedious process translate into considerable cost savings. Estimates suggest that these automation processes can potentially reduce document processing costs by up to 50%. These savings can be reinvested in various areas, potentially supporting technological innovation within the firm or improving client services. Lastly, facilitating smoother collaborations across jurisdictions is a compelling benefit. AI-powered redaction ensures documents are appropriately redacted in accordance with diverse local regulations, streamlining cross-border legal proceedings.
While the benefits of automated redaction are evident, there are considerations, such as ensuring the training data for the AI systems are unbiased and representative of the real-world cases they encounter, to mitigate potential issues in the outputs of the systems. It’s fascinating to consider how this advancement in data security compliance through automation will continue to impact the legal landscape, potentially driving further innovations in AI's role in legal document management and analysis.
More Posts from legalpdf.io: