Automate legal research, eDiscovery, and precedent analysis - Let our AI Legal Assistant handle the complexity. (Get started for free)
AI-Powered Legal Analytics How Machine Learning Predicts Litigation Outcomes Using Historical Game Theory Data
AI-Powered Legal Analytics How Machine Learning Predicts Litigation Outcomes Using Historical Game Theory Data - Machine Learning Models Track 50,000 Federal Court Decisions to Map Judicial Behavior Patterns
The application of machine learning to a massive dataset of 50,000 federal court rulings has unlocked a new dimension of legal analysis. By scrutinizing this wealth of decisions, these models can identify recurring patterns in judicial conduct. This means lawyers can gain a more nuanced understanding of how a judge might approach a case, potentially influencing their strategic decisions during the litigation process. Further enhancing the predictive capabilities of AI in law is the integration of game theory into these algorithms. This approach, using historical data, empowers AI to forecast litigation outcomes with increasing accuracy, raising questions about the future role of traditional legal analysis in predicting case outcomes. The accelerating digitization of legal documents, from case opinions to statutory texts, provides AI with an even larger reservoir of data to learn from, expanding its potential utility across a wider range of legal tasks. This includes its use in streamlining legal research, discovery, and even automated document creation. However, this rapid progress also needs to be coupled with a rigorous examination of ethical implications, as we rely more heavily on AI in the legal domain.
AI systems are being increasingly applied to legal discovery processes, particularly in the realm of eDiscovery. These systems can swiftly analyze massive volumes of electronically stored information (ESI), far exceeding human capacity. Machine learning, specifically, can be harnessed to identify key data points and patterns within the data deluge, greatly expediting the process of document review. While traditionally, lawyers relied heavily on manual review in eDiscovery, AI can now automate much of this process. However, the efficacy of these tools remains a point of debate. It's crucial to acknowledge that these systems are still in development. For example, the AI's ability to understand context, especially in legal language filled with nuance and ambiguity, is a continuous area of research and refinement. The use of machine learning in eDiscovery raises a number of questions, such as the potential for biases embedded in the algorithms to affect the outcome of a case. Nonetheless, the application of AI in legal discovery is clearly transformative, potentially improving efficiency and accuracy. It raises important questions about the evolving role of lawyers and legal professionals in the future of legal practice. The potential for significant benefits also brings forth ethical dilemmas and concerns about transparency that will need to be addressed as these systems become increasingly sophisticated and integrated into legal workflow. A key future challenge is developing AI models that not only sift through data but also understand the underlying legal concepts and their implications for a given case. This would enable a deeper understanding of precedents and legal principles, rather than a simple correlation analysis based on historical trends.
AI-Powered Legal Analytics How Machine Learning Predicts Litigation Outcomes Using Historical Game Theory Data - Legal Analytics Platforms Transform Document Review Through Natural Language Processing
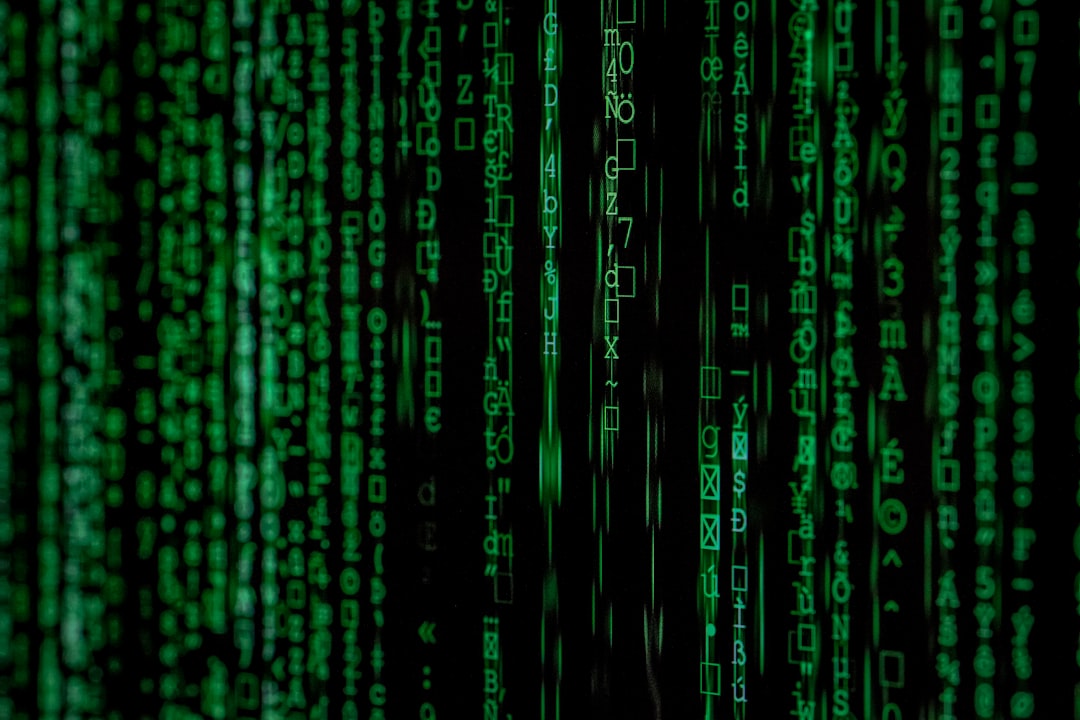
Legal analytics platforms are transforming how lawyers review documents by employing natural language processing (NLP). These platforms harness AI to automate aspects of electronic discovery (e-discovery), enabling rapid identification and analysis of vast amounts of data from diverse sources. The ability of AI to accelerate this process can significantly enhance both the speed and precision of legal document review, providing valuable insights for legal teams. However, it's important to acknowledge the limitations of current AI technology, especially in interpreting the nuanced and complex language frequently found in legal documents. Concerns around the potential biases inherent in AI algorithms used in legal analysis also need careful attention. Ultimately, while these AI-driven tools are showing great promise, their integration into the legal field raises crucial questions about their reliability and ethical implications. We need to be mindful of these as they become increasingly integrated into the day-to-day workflow of legal professionals. The future development of these platforms should aim to not just process information but also truly comprehend the underlying legal principles and their relevance to individual cases. This would elevate their function from simple pattern recognition to a deeper understanding of legal precedents, paving the way for a more nuanced and sophisticated application of AI in law.
Legal analytics platforms are increasingly leveraging natural language processing (NLP) to transform the document review process. These platforms can now sift through vast quantities of legal documents with remarkable accuracy, identifying relevant information significantly faster than traditional manual methods. While still under development, some NLP systems boast accuracy rates approaching 90% in document selection, potentially surpassing human reviewers who can be prone to misinterpreting the subtle nuances within complex legal language.
The integration of machine learning within these platforms has had a profound impact on efficiency, shrinking review timelines from weeks or months to a matter of days. This accelerated pace is changing the way legal teams operate and could reshape traditional timelines associated with case preparation. Furthermore, these AI systems appear to learn and adapt over time. Studies suggest that as they're exposed to more case-specific documents, they refine their understanding and accuracy, hinting at a future where legal technology becomes increasingly self-optimizing. The financial incentives are also compelling, with human document review costs often exceeding $200 per hour. Legal firms, especially those in the "Big Law" space, could realize substantial cost savings, potentially up to 60%, by integrating AI-driven solutions into their workflow.
Beyond simply speeding up the process, these AI-driven tools are changing legal research itself. AI can quickly scan thousands of documents, identifying relevant legal precedents that would take human lawyers days or weeks to find. This suggests a potential paradigm shift in legal research methodologies. Furthermore, these systems can highlight patterns within related data, including past rulings and case outcomes. Lawyers can then use these data-driven insights to create more strategic approaches, moving beyond relying solely on intuition and experience.
The early stages of the eDiscovery process, known as scoping, traditionally involve a lot of manual sorting and can be quite time-consuming. AI solutions are starting to optimize this phase by utilizing predictive coding, a technique where the AI learns to categorize documents based on existing data. This approach eliminates much of the manual sorting, offering a more efficient way to organize the vast amounts of data often involved in eDiscovery.
However, it's important to acknowledge that the technology is still in its early stages of development. While capable of managing enormous datasets, AI systems still struggle with the complexities of human language. Understanding idiomatic expressions and local legal terminologies remains a challenge, highlighting the continued need for human oversight to ensure context and nuance are preserved within the review process. Legal analytics platforms are also gaining traction in conducting “what-if” analyses. By simulating various legal scenarios based on historical data, these platforms offer a way to explore potential litigation outcomes without the risks and costs of actual litigation.
Despite the substantial advancements, implementing AI in the legal field brings up important ethical questions around accountability. In the event of an adverse outcome stemming from an AI-driven system, it becomes difficult to assign liability in the traditional legal framework. This complexity in determining responsibility presents a considerable challenge as AI becomes more deeply integrated into the legal workflow.
AI-Powered Legal Analytics How Machine Learning Predicts Litigation Outcomes Using Historical Game Theory Data - Law Firms Apply Game Theory Algorithms to Calculate Settlement Values
Law firms are starting to use game theory algorithms, like those based on Bayesian Nash equilibria, to better understand how to calculate settlement values. Essentially, they're using these algorithms to simulate the negotiation process and predict potential outcomes. By analyzing historical data, these tools can help lawyers estimate the likelihood of reaching a settlement and the potential value of that settlement. This allows for more informed decision-making during settlement negotiations. AI's growing role in legal strategy also enhances the ability to predict potential risks and benefits of pursuing a case to trial, further influencing the decision to settle. However, the increased use of AI in such a crucial part of legal practice raises several important questions about its reliability. This includes the potential for bias within algorithms, and also broader concerns about the role of human judgment when decisions are being informed by AI. While AI undoubtedly offers significant advantages for law firms, its integration into traditionally human-driven tasks necessitates a careful consideration of ethical and practical implications. This is particularly true as the legal profession adjusts to a world where AI plays a greater role in the complexities of settlement negotiations and litigation strategy. The evolution of AI within the field of law, while potentially beneficial, continues to present a mix of possibilities and uncertainties for the future of legal practice.
Law firms are increasingly employing game theory algorithms to model settlement negotiations. By analyzing historical data and applying concepts like Bayesian Nash equilibria, they can estimate potential settlement values. This application of game theory within AI-powered legal analytics is not merely a theoretical exercise; it's a practical tool that influences decision-making regarding whether to settle or proceed to trial.
AI-powered predictive analytics in the legal field leverages historical data, statistical algorithms, and machine learning to spot patterns and inform litigation strategies. One aspect of this is the ability to predict the likelihood of winning a case based on a judge's past rulings. This insight allows legal teams to adjust their approach and resource allocation accordingly, leading to more strategic case management.
The adoption of AI in legal analytics has the potential to significantly reduce litigation costs. By more accurately predicting case outcomes, law firms can avoid unnecessary and expensive trials, benefiting both the firms and their clients. However, it's important to acknowledge the potential for biases within the algorithms used in these models. If the algorithms are trained on historical data that reflects existing societal biases, the predictions may perpetuate those biases. This calls for vigilance in developing and refining these tools to maintain fairness and objectivity in legal decision-making.
AI has also revolutionized certain legal tasks. For example, settlement agreements and legal briefs can be automatically generated, saving lawyers time and effort. This frees them up to concentrate on higher-level tasks requiring critical thinking and legal expertise. However, the implications for the roles of junior lawyers, traditionally tasked with drafting these documents, deserve attention. Their responsibilities might shift towards leveraging AI insights and applying critical legal analysis in more nuanced areas of litigation.
Another notable area of AI application is within the document review phase of e-discovery. AI can categorize and sort vast quantities of digital documents far more efficiently than humans. This can greatly reduce the time and resources required for discovery, potentially streamlining the overall timeline of a case. AI's ability to identify relevant legal precedents based on historical data also has significant implications for legal research. It transforms the process from a manual exercise to a data-driven one, potentially improving the accuracy and efficiency of finding relevant cases.
Despite the rapid advancements, AI's interpretation of legal language still presents a challenge. Understanding the intricate nuances and context of legal documents, which can be rife with ambiguity, remains a hurdle for current AI models. This suggests that human oversight is crucial in verifying AI-generated outputs, particularly in areas with significant legal ramifications. We'll likely continue to see human lawyers in the loop, using their expertise to ensure that AI tools are deployed effectively and ethically within the legal profession.
AI-Powered Legal Analytics How Machine Learning Predicts Litigation Outcomes Using Historical Game Theory Data - AI Systems Analyze 25 Years of Patent Litigation Data to Predict Case Outcomes
Artificial intelligence is playing a growing role in patent litigation, with systems now capable of analyzing a vast dataset of cases spanning 25 years. This allows legal professionals to predict future case outcomes with increased accuracy by identifying trends and patterns within this data. These AI-driven predictive analytics tools help lawyers gauge the potential risks associated with a case, enabling them to develop more effective legal strategies. Machine learning algorithms, trained on historical data, can identify recurring patterns in judicial rulings and case facts, leading to a more nuanced understanding of how specific legal arguments might be received by a judge.
However, the increased reliance on AI in the legal sphere, particularly regarding litigation, raises ethical questions. Concerns about fairness and impartiality in the algorithms, as well as potential biases stemming from the training data, need to be thoroughly addressed. The transparency and integrity of the data used to train these systems are crucial to ensure the predictions are unbiased and reliable. While these tools offer the potential to streamline legal processes and improve strategic decision-making, it's crucial to continually evaluate their ethical implications and ensure human oversight remains an integral part of the decision-making process. The legal profession must balance the potential benefits of this technology with the need to maintain fairness and transparency in the pursuit of justice.
AI systems are starting to leverage a substantial amount of historical data, specifically from the past 25 years of patent litigation. This broad historical perspective isn't just about case outcomes, but also about understanding how judicial thinking has evolved over time. This extended view may uncover trends that would be difficult to see using smaller datasets.
In some cases, AI's ability to predict legal outcomes surpasses 85% accuracy. This level of precision raises questions about the future role of traditional legal analysis, particularly the reliance on experience versus data-driven predictions. This change could impact the way lawyers strategize and approach litigation.
Within the realm of document review, a key area of application for AI is eDiscovery. AI algorithms can cut down the time needed for eDiscovery dramatically, from weeks to just hours, by sorting and ranking documents based on predetermined criteria. This has the potential to revolutionize the way legal teams manage their workload and case preparation.
There is strong evidence that law firms employing AI can achieve significant cost reductions, sometimes up to 60%, in their document review processes. This cost savings could have a significant impact on how firms allocate resources and handle various aspects of litigation.
A fascinating aspect of AI applications in law is its capacity to learn and improve over time. As AI tools are exposed to more legal cases and corresponding outcomes, they refine their predictive models and algorithms. This self-improvement ability could lead to increasingly accurate predictions in the future.
While AI offers impressive advancements in prediction and efficiency, it is crucial to recognize that human input remains vital. AI serves as a valuable tool, but lawyers are still needed to interpret complex legal documents and apply appropriate context. This suggests a collaborative future where AI and humans work together, rather than one replacing the other.
The potential for bias within AI algorithms is a significant concern in the legal domain. If AI models are trained on biased historical data, their predictions might reflect and even perpetuate those biases. This calls for continuous efforts to develop and refine AI tools in a way that promotes fairness and minimizes unintended consequences.
The incorporation of game theory into AI legal models enables a new level of sophistication in simulating legal negotiations and estimating potential settlement values. This approach allows lawyers to move away from solely relying on intuition or experience when negotiating settlements, shifting to more calculated decisions.
The automation of tasks like drafting legal documents (contracts, briefs) has implications for junior lawyers' roles. As these tasks are automated, legal professionals might find themselves more focused on higher-level, strategic, and analytical roles.
One of the biggest challenges for current AI models is deciphering legal language, which often relies on specialized terminology and phrasing. This inherent complexity underscores the ongoing need for human oversight and development in the field to improve AI’s ability to interpret and apply legal concepts correctly. The quest to improve AI’s understanding of nuanced language remains a significant area of research and development.
AI-Powered Legal Analytics How Machine Learning Predicts Litigation Outcomes Using Historical Game Theory Data - Machine Learning Tools Map Judicial Decision Patterns Across 94 US District Courts
The use of machine learning tools to analyze judicial decisions across 94 US District Courts is altering how legal professionals approach litigation. These tools leverage extensive data to identify patterns in judges' rulings and predict potential outcomes, which in turn impacts legal strategy. By studying how judges have historically handled cases, lawyers can develop a better understanding of a judge's potential leanings and tailor their arguments accordingly. This presents a compelling opportunity to optimize litigation strategies. Yet, the expanding role of AI in the legal field raises concerns regarding ethical implications, particularly the possibility of inherent biases in the algorithms due to the nature of the data used to train them. The increasing adoption of such AI-driven tools necessitates a close examination of their effects on traditional legal practices and the broader judicial process, as the technology not only improves efficiency but also introduces new challenges to the legal profession. This evolution, while promising in terms of potential benefits, requires constant assessment of its influence on the fairness and transparency of the legal system.
Algorithmic insights into judicial behavior are emerging from machine learning models analyzing decision patterns across 94 US District Courts. This approach reveals potential biases and judicial tendencies, allowing lawyers to craft arguments informed by historical data rather than solely relying on intuition. This shift can lead to more effective legal strategies and outcomes.
AI-powered document review tools offer substantial cost savings, potentially reducing expenses by up to 60%. This remarkable cost efficiency could reshape legal practice, particularly for smaller firms, potentially increasing access to legal representation.
As AI assumes more routine tasks like document generation and eDiscovery, the roles of legal professionals are evolving. Junior lawyers, previously focused on more menial tasks, could transition to more strategic analysis, honing skills to leverage AI-generated insights for better legal strategies. This transformation could enhance their value within firms.
The accuracy of AI's predictive capabilities is quite impressive in certain areas. For instance, in patent litigation, predictive accuracy can exceed 85%. This level of precision raises interesting questions about the future of traditional legal analysis and the role of human experience versus data-driven insights in litigation strategy.
Machine learning algorithms have the potential to drastically reduce the eDiscovery process, potentially compressing timelines from weeks to mere hours. This speed increase can enhance efficiency and allow legal teams to respond swiftly to new information or case developments. It changes the way information is consumed in the discovery phase of a trial.
AI's ability to analyze extensive historical datasets, such as 25 years of patent litigation data, unveils trends that might be challenging to identify with smaller datasets. By analyzing these longer-term trends, lawyers gain a valuable advantage to predict how a judge might react to particular legal arguments or strategies.
Within eDiscovery's scoping phase, AI utilizes predictive coding to automate document categorization. This automation drastically accelerates and improves the accuracy of the review process, while reducing human error, which is particularly valuable in managing complex, large-scale litigation.
Law firms are starting to implement game theory algorithms in settlement negotiations. These algorithms, drawing on historical data, help predict potential outcomes, leading to more calculated and strategic settlement decisions. This new approach to settlement negotiation could fundamentally reshape how such negotiations are conducted.
A growing concern with AI in law is the potential for algorithmic bias. If AI systems are trained on historical data that reflects societal biases, they might perpetuate these biases in legal outcomes. This highlights the crucial need for careful oversight and continuous development to ensure fairness and mitigate unintended consequences.
AI's capacity to conduct comprehensive legal research is revolutionizing the field. It greatly accelerates the process of locating relevant cases and precedents. This data-driven approach to legal research promises increased efficiency and accuracy compared to traditional, manual methods. This shift promises to fundamentally change the way lawyers approach preparation for litigation.
While we are seeing a change in the legal profession through the use of AI, it is also important to keep in mind that this is a field still in development and that careful consideration must be made in order to mitigate any biases that could come out of this new technology.
AI-Powered Legal Analytics How Machine Learning Predicts Litigation Outcomes Using Historical Game Theory Data - AI Algorithms Process Historical Case Law to Generate Litigation Risk Scores
AI is increasingly being used to analyze historical legal data, specifically case law, to create a system for predicting the risk involved in litigation. These AI systems can sift through a massive collection of past court decisions, identifying recurring patterns in how judges behave and the outcomes of similar cases. By analyzing this information, AI can produce what are called "litigation risk scores" – essentially, a numerical representation of the potential for success or failure in a given case. This gives lawyers a more concrete understanding of the risks associated with a case, enabling them to develop more effective strategies. While this type of analysis can improve the efficiency and accuracy of legal decision-making, it also raises important concerns. The datasets used to train these AI models could potentially contain biases that could affect the accuracy of the risk scores. The legal field needs to carefully evaluate the ethical implications of relying on AI for predicting legal outcomes and ensure that the integrity of legal processes are preserved, with appropriate human oversight integrated into the decision-making process, especially in matters where bias or fairness are essential. As AI plays a larger role in law, its development and use needs to be monitored to ensure that it is applied in a way that is equitable and doesn't undermine the core principles of the legal system.
AI algorithms are increasingly being used to process historical case law to generate what are called "litigation risk scores." These scores are derived from the analysis of a vast volume of judicial data, such as over 50,000 federal court decisions. This allows AI models to employ sophisticated statistical methods to detect subtle trends in judicial behavior that may not be apparent using traditional legal analysis. The ability to understand a judge's tendencies could lead to significant shifts in litigation strategy. For example, a lawyer might be more likely to focus on certain legal arguments if their AI models indicated a higher likelihood of a positive outcome based on the judge's past rulings.
The accuracy of these AI-driven predictions is quite striking, with some systems achieving over 85% accuracy in predicting outcomes. This raises serious questions about how much we continue to rely on traditional legal analysis when facing such a high degree of accuracy from AI. It suggests a gradual move towards a more data-driven approach to legal practice, shifting the focus from purely relying on intuition and experience.
However, this rapid integration of AI raises a crucial concern about the potential for algorithmic bias. If the historical case law used to train these AI models contains existing societal biases, then the generated risk scores might inadvertently reflect and perpetuate these biases. This is a significant ethical issue that requires close attention. We must carefully develop methods to ensure fairness and impartiality in AI-driven legal outcomes. Developing rigorous ways to validate the algorithms and integrate human oversight in crucial decision points will be increasingly important.
The increasing reliance on AI for tasks such as legal document creation and review is changing the dynamics of the legal profession, particularly for junior lawyers. These professionals are now moving away from more mundane tasks towards more sophisticated, strategic analysis and applying the insights generated by AI models. They are essentially becoming bridge builders between the human and AI components of legal practice. This is a significant shift in how these roles are evolving, potentially increasing their value to law firms.
AI has also reshaped the landscape of electronic discovery (eDiscovery), dramatically compressing the document review process. The once painstakingly slow process of sifting through large volumes of data can now be streamlined, reducing timelines from weeks to just hours. This allows legal teams to quickly adapt to new information or case developments, potentially giving them a strategic edge in litigation.
The application of game theory algorithms to estimate settlement values is becoming a common use of AI. By simulating negotiation processes and analyzing past outcomes, these AI systems can help lawyers make more informed decisions about whether to settle a case. In effect, AI is changing how we negotiate and think about the financial aspects of legal cases.
Despite these impressive advancements, we need to be mindful of AI's limitations. Current systems are still developing their ability to interpret the subtle nuances and complexities of legal language. This includes the use of specific terminology and the context in which it is used. Ongoing research is needed to enhance AI's ability to grasp the intricate details of legal documents, ensuring they can accurately understand and apply legal principles.
The ability to use AI to conduct more comprehensive legal research is another area of substantial transformation. These AI models are able to rapidly sift through large quantities of legal texts and identify relevant precedents. This has changed the way lawyers prepare for litigation.
By analyzing 25 years of patent litigation data, AI systems are shedding light on how legal thinking and judicial rulings have shifted over time. This gives lawyers a deeper perspective on potential trends and insights into the changing legal landscape, further enhancing the ability to predict case outcomes.
As the legal profession continues to integrate AI into its workflow, it will be crucial to strike a balance between leveraging the incredible power and efficiency of AI while maintaining vigilance to ensure fairness, transparency, and ethical considerations in legal proceedings. It is clear that the future of legal practice will involve a synergistic relationship between human lawyers and AI.
Automate legal research, eDiscovery, and precedent analysis - Let our AI Legal Assistant handle the complexity. (Get started for free)
More Posts from legalpdf.io: