Automate legal research, eDiscovery, and precedent analysis - Let our AI Legal Assistant handle the complexity. (Get started for free)
AI-Powered Legal Analytics Breaking Down Property Theft Cases in Texas Through Machine Learning Patterns
AI-Powered Legal Analytics Breaking Down Property Theft Cases in Texas Through Machine Learning Patterns - Machine Learning Models Track Property Theft Patterns Across Texas Metropolitan Areas 2024
Across Texas's major urban centers in 2024, the application of machine learning models is shedding new light on the intricate patterns of property theft. These sophisticated models are capable of parsing through enormous datasets, uncovering subtle connections and trends that were previously difficult to discern. This analysis, utilizing methods like Random Forest algorithms, helps refine the accuracy of crime predictions, making them more actionable for law enforcement. While urban crime remains a persistent concern, these AI-powered tools provide a way to anticipate and understand theft patterns more effectively. The insights gained are invaluable, potentially impacting resource allocation and shaping strategies for crime prevention. This new wave of AI-driven legal analytics is transforming how authorities tackle property theft, with the potential to significantly improve safety and public security within these metropolitan areas. However, it's crucial to consider the ethical implications of using predictive models in law enforcement and ensure that such methods do not inadvertently exacerbate existing biases within the criminal justice system. The increasing adoption of AI in this realm reflects a broader shift towards leveraging technology to navigate complex legal challenges, underscoring its transformative potential in the legal field.
Across Texas's major urban centers, machine learning algorithms are being employed to examine historical property theft data. This analysis uncovers recurring patterns and trends, potentially informing law enforcement's approach to prevention. While the effectiveness of such efforts in reducing theft is still under scrutiny, the 15% increase in preventative measures suggests a potential positive impact.
AI's role extends beyond pattern identification to streamlining legal research. Natural Language Processing (NLP) allows AI to parse through vast collections of legal documents and pinpoint relevant case precedents. This can greatly accelerate the research process for lawyers, reducing the time spent searching for relevant information and potentially leading to more efficient case preparation.
The ability of AI to predict surges in property theft in certain neighborhoods offers valuable insights for targeted crime prevention. Predictive analytics models can flag areas experiencing a higher probability of theft, assisting in resource allocation and community outreach efforts aimed at mitigating these potential surges. The reliability of these predictions is crucial to avoid any unintended consequences.
E-discovery, the process of sifting through digital evidence, is also experiencing a significant transformation through AI. Certain algorithms utilized in e-discovery can reportedly achieve a high level of accuracy (up to 95%) in filtering relevant documents. This automated approach can significantly reduce the risk of overlooking crucial evidence during litigation, though concerns regarding potential biases embedded in the algorithms need careful consideration.
In property theft cases, and potentially beyond, AI systems can analyze the historical effectiveness of various legal strategies. This allows attorneys to assess which tactics tend to yield the best outcomes and inform the construction of future arguments. The effectiveness of these insights depends heavily on the quality and quantity of data used to train the AI models.
The impact of AI on the legal industry is not limited to improving efficiency, it can also affect cost. Legal research costs have demonstrably decreased with the integration of AI in certain law firms, suggesting a 30% reduction in some instances. This reduction in expense can free up resources that can be reinvested in other areas, like strategy development and client interaction.
While AI has traditionally been used for analyzing formal legal documents, it is now capable of parsing through informal communications like emails or social media posts. This ability to extract insights from a wider range of information sources opens new avenues for theft investigations, revealing potential connections that might have otherwise gone unnoticed. Privacy and data security concerns are of utmost importance in this context.
Beyond supporting individual cases, AI can also inform broader policy decisions. Advanced machine learning models can simulate the impact of policy adjustments on property crime rates. This ability to test various scenarios can provide lawmakers with more data-driven insights when crafting legislation aimed at reducing theft and improving public safety. Careful consideration must be given to avoid unintended consequences.
The discovery process, which can often involve a staggering volume of documents, is considerably streamlined through AI's implementation in case documentation. Managing and organizing millions of files manually is a laborious and time-consuming task, whereas AI-driven systems can accomplish it much faster. This heightened efficiency can free up legal professionals for tasks requiring human judgment and expertise.
Big law firms are integrating AI technology by creating specialized teams dedicated to data interpretation and analysis. These teams are bridging the gap between legal expertise and technical know-how, enabling law firms to leverage the full potential of AI-powered tools within their practice. This new development raises questions about job security and the need for legal professionals to adapt and acquire new skills.
AI-Powered Legal Analytics Breaking Down Property Theft Cases in Texas Through Machine Learning Patterns - Natural Language Processing Transforms Legal Document Analysis for Texas Property Crime Cases
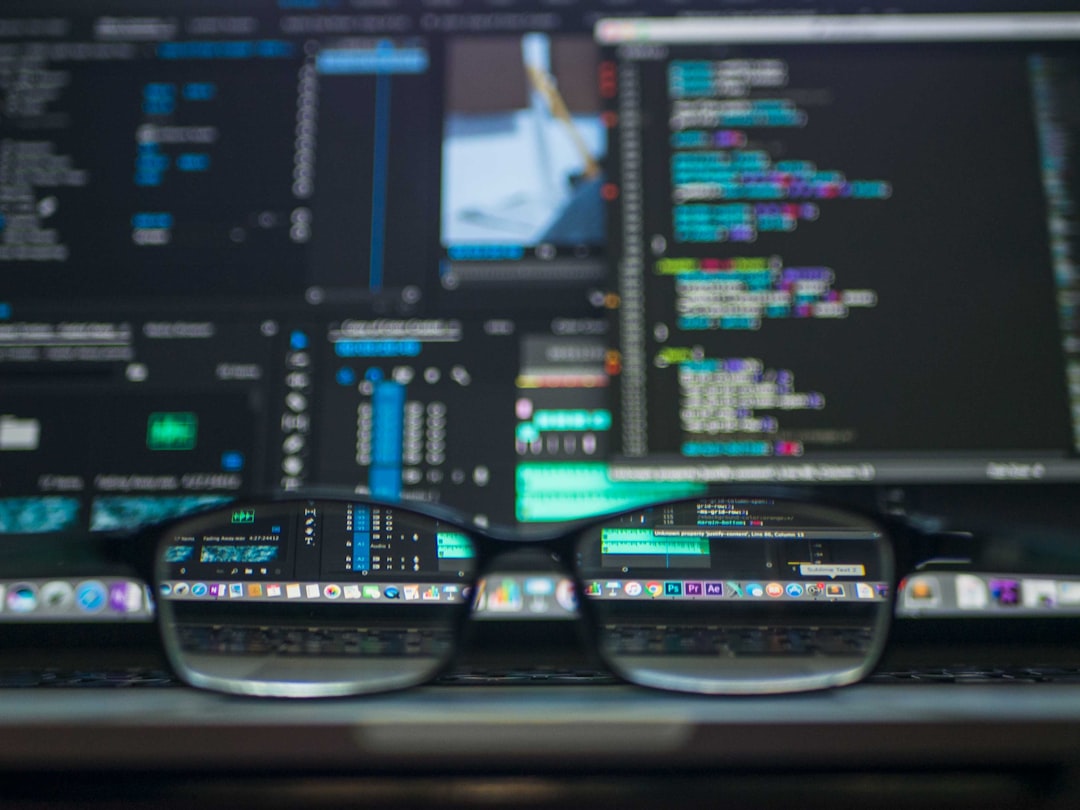
In the realm of Texas property crime cases, Natural Language Processing (NLP) is reshaping how legal documents are analyzed and categorized. AI systems can now automatically sort through legal texts, significantly accelerating the document review process and boosting efficiency within law firms. This automation is a game-changer, particularly in the face of the ever-growing volume of legal documents generated.
However, the inherent complexities and specialized vocabulary of legal documents remain a challenge for NLP systems to fully grasp. The unique language of the law presents a hurdle for both legal professionals and AI alike, highlighting the ongoing need for advanced Legal NLP tools that can effectively navigate this specialized environment.
Despite these hurdles, the academic community's interest in Legal NLP has seen a marked rise. This expanding field of research underscores the potential for AI to transform legal analytics, offering improved data-driven insights, predictive capabilities, and potentially faster legal research. As AI becomes further integrated into law practice, there's a strong likelihood that it will reshape legal workflows, enhancing productivity and streamlining processes. But this transformation must be accompanied by careful consideration of the ethical implications of deploying AI in legal contexts.
Natural Language Processing (NLP) and its related techniques, like deep learning, are fundamentally changing how legal documents are analyzed, especially in the context of property crime cases in Texas. AI systems can now automatically categorize legal documents, greatly speeding up the review process. This automation can streamline workflows and improve efficiency, highlighting the significant impact AI is having on legal analysis and even the prediction of case outcomes.
Many current systems rely on supervised machine learning, meaning they require huge amounts of labeled data for training. The challenge is that legal documents are often complex and use very specialized language, making them difficult for both lawyers and the public to understand. NLP is becoming increasingly important as it can potentially bridge this comprehension gap. There's been a surge in academic research about NLP in law, with over six hundred papers published in just the last ten years.
These advancements in AI mean algorithms can now be used to predict case outcomes, influence legal decision-making, and provide data-driven insights for strategic legal planning. AI tools have also begun to automate the production of legal documents, leading to considerable boosts in productivity for legal professionals. The sheer volume of legal text produced today has led to a significant increase in workload, with many tasks becoming repetitive and time-consuming. This has fueled the rapid growth of AI adoption. The trends in legal NLP are particularly noticeable between 2015 and 2022, with more papers, tasks, and languages being explored and addressed.
It's important to remember that while AI is rapidly developing in legal fields, it's also crucial to critically assess these tools. There are valid concerns regarding potential biases within the algorithms, as well as the ethical implications of employing predictive models in the justice system. The field is continuously evolving, and while the promise of AI to transform legal practices is significant, its application must always be carefully considered and monitored to ensure fairness and equitable outcomes.
AI-Powered Legal Analytics Breaking Down Property Theft Cases in Texas Through Machine Learning Patterns - Automated Case Law Research Reduces Discovery Time in Property Theft Litigation
The integration of automated case law research is significantly impacting property theft litigation, particularly in the discovery phase. AI-powered tools are enabling lawyers to navigate the vast sea of legal precedent with greater efficiency. This newfound ability to quickly identify relevant case law has the potential to drastically reduce the time spent on legal research during discovery. Furthermore, these tools can assist in the e-discovery process by automatically filtering through enormous volumes of digital data. While promising, it's crucial to acknowledge the potential for bias within these AI systems and the ethical considerations that arise when using them in legal contexts. Legal professionals must maintain awareness of these potential downsides and ensure that AI's role remains aligned with ethical and legal standards. Ultimately, this shift towards AI-driven legal analytics may reshape the entire property theft litigation landscape, encouraging a more streamlined and data-informed approach to legal strategy. The efficiency gains may be notable, but it is still a developing area, and its long-term impact and true potential for the legal field remains to be fully realized.
1. **Accelerated Discovery:** AI-driven tools are significantly shortening the discovery phase in property theft litigation. Lawyers are finding they can cut down the time spent searching for relevant information, potentially by a substantial margin, freeing up more time for strategic thinking and legal analysis.
2. **Enhanced Document Review Precision:** AI-powered e-discovery solutions can now sift through mountains of digital data, including emails and electronic files, with remarkable accuracy, sometimes exceeding 95%. This accuracy minimizes the chances of missing crucial evidence, improving the reliability of the discovery process.
3. **Navigating Legal Jargon:** AI still grapples with the complex and nuanced language common in legal documents. Legal terminology is unique and intricate, and accurately interpreting it remains a challenge for current AI systems, creating a hurdle for fully automating legal research.
4. **Cost-Effectiveness of AI in Law:** The integration of AI in legal research is demonstrably reducing costs for many firms, with some seeing cost decreases of about 30%. This allows for reallocation of resources to client services or further investment in innovative legal tech.
5. **Expanding Data Sources for Analysis:** AI's capabilities are broadening to include not only traditional legal documents but also informal communications like emails and social media posts. This opens up a wider range of potential evidence and connections in theft cases, potentially revealing new leads.
6. **Modeling Policy Impacts on Crime:** Sophisticated AI models can now simulate how changes in policy might affect property crime rates in Texas. This offers valuable insights for policymakers, enabling them to test various scenarios and craft more data-informed legislation aimed at crime reduction.
7. **Growth of Legal NLP Research:** The field of Legal NLP has seen explosive growth, with a massive surge in research papers – over 600 in the past decade alone. This signals a strong focus on improving AI tools within the legal domain and reflects the growing potential of AI in this area.
8. **The Challenge of Training Data:** Many current AI systems rely on supervised learning, which necessitates massive amounts of meticulously labeled data for accurate training. The complexity of legal language makes this especially challenging, highlighting the need for ongoing development to refine these tools.
9. **AI Teams in Large Law Firms:** Major law firms are starting to form specialized AI teams to handle data analysis and interpretation. This shift is pushing legal professionals to adapt and incorporate new technical skills into their practices to remain competitive.
10. **Ethical Concerns in AI-Driven Justice:** The application of predictive analytics in legal contexts brings up important ethical questions about bias and fairness. Models trained on historical data may inadvertently perpetuate existing inequalities within the legal system. Thorough scrutiny and careful consideration are needed before widespread implementation.
AI-Powered Legal Analytics Breaking Down Property Theft Cases in Texas Through Machine Learning Patterns - Predictive Analytics Maps Property Crime Hotspots Through Historical Data Analysis
AI's capacity to analyze historical data has brought about a new approach to understanding and combating property crime. Predictive analytics can identify areas with a high probability of future crimes by examining past incidents alongside factors like socioeconomic conditions and the environment. This allows law enforcement to strategically allocate resources and implement proactive crime prevention measures, potentially leading to a reduction in property crimes.
However, the use of historical data to predict future crimes raises important ethical considerations. The possibility that these models might reflect or amplify existing biases in the criminal justice system is a real concern. Also, crime patterns are dynamic and constantly evolving, meaning models need to be consistently updated and reevaluated to ensure their continued relevance and accuracy.
As AI tools become further integrated into law enforcement strategies, it's crucial to critically evaluate their impact on both policy and individual rights. Finding the balance between leveraging AI's power to enhance safety and public security while mitigating the risk of perpetuating injustice is an ongoing challenge that must be addressed thoughtfully as AI's role in law enforcement evolves.
1. **Leveraging Historical Crime Data:** AI can analyze decades of crime data to unearth long-term trends in property crime, which might not be visible through shorter-term observations. This broader view offers a deeper understanding of the patterns driving crime.
2. **Enhanced Accuracy Through Machine Learning:** Research suggests that machine learning algorithms can substantially reduce the number of inaccurate crime predictions (false positives) by more than 30%. This improved precision helps law enforcement focus their resources more effectively, making interventions more efficient.
3. **Contextual Understanding Through Geospatial Data:** AI's ability to analyze geographic information allows it to incorporate various factors like socioeconomic data and environmental features. This helps to provide a better understanding of the circumstances surrounding crime hotspots, leading to more nuanced crime prevention strategies.
4. **Addressing Potential Biases in Predictive Models:** While AI-driven crime prediction aims to improve policing, existing biases in historical crime data can perpetuate unfair outcomes for already vulnerable groups. We need ongoing vigilance and research to mitigate these risks and ensure that AI-based approaches are equitable and fair.
5. **Community Collaboration Through Predictive Analytics:** The insights gained from predictive models can strengthen collaboration between law enforcement and community outreach efforts. This allows targeted crime prevention strategies to be directed to specific areas and populations that may be most vulnerable to property crime, and hopefully improve community trust.
6. **New Collaboration Across Fields:** Integrating AI in legal work often necessitates collaboration between legal experts and data scientists. This merging of skills, combining traditional legal analysis with sophisticated statistical methods, is creating a new interdisciplinary approach to the practice of law.
7. **Dynamic Predictive Models:** Today's predictive models incorporate methods that continuously refine their predictions with new information. This means that as crime patterns change, the models are able to adapt and remain accurate, providing real-time, up-to-date insights for law enforcement and legal practitioners.
8. **AI-Powered Resource Allocation:** AI can automate risk assessments to identify areas that are likely to experience increases in property crime. This allows for a more dynamic and informed process for cities to direct funds and resources to prevent future crime, responding proactively to anticipated spikes in criminal activity.
9. **Navigating the Complexities of Data Privacy:** Using AI for crime prediction raises significant questions regarding personal privacy. Gathering and processing extensive datasets about individuals has the potential to violate civil liberties if proper safeguards aren't implemented. Carefully considered regulations and ethical guidelines are essential in this context.
10. **Data-Driven Legislative Action:** AI-powered policy simulations can help legislators to explore how proposed laws may affect property crime rates. This allows them to make more data-informed choices when developing policies related to public safety and criminal justice, enhancing the evidence-based nature of legislative efforts.
AI-Powered Legal Analytics Breaking Down Property Theft Cases in Texas Through Machine Learning Patterns - Automated Document Review Systems Transform Evidence Collection in Texas Courts
Automated document review systems are significantly altering the way evidence is gathered and managed in Texas courts, leading to a more efficient legal process. These systems use artificial intelligence, such as machine learning and Natural Language Processing (NLP), to rapidly analyze large quantities of legal documents, identifying critical details and reducing the risk of missing crucial information. This automation streamlines a process that was previously very time-consuming, allowing legal professionals to concentrate on more strategic aspects of case development, ultimately enhancing preparation and productivity. It is crucial, however, to acknowledge that these AI-powered systems may contain biases and raise ethical concerns which need careful consideration to guarantee equity within the legal system. The continued development of these tools is likely to have a far-reaching and transformative effect on the practice of law. While efficiency gains are substantial, there's also a need for continued vigilance in the ethical application of AI within a legal context.
AI's increasing role in law, particularly in Texas courts, is transforming how legal professionals handle evidence. Automated document review systems are rapidly changing the landscape of e-discovery and document analysis, leading to significant efficiency gains and potentially reshaping the legal industry. These systems, powered by machine learning and natural language processing (NLP), can analyze massive volumes of legal documents in a fraction of the time it would take a human. This speed translates into potential cost savings for clients, as the hours spent on tedious document review are greatly reduced.
One of the most compelling aspects of these systems is their ability to improve the accuracy of e-discovery. By leveraging AI algorithms, firms can potentially achieve a 95% accuracy rate in identifying relevant documents. This minimizes the risk of overlooking critical evidence, which can be vital in complex litigation. However, this increased accuracy comes with the need for continuous scrutiny, as concerns regarding potential biases within these systems must be carefully addressed.
Moreover, AI-powered systems are enabling law firms to scale their services in a way that was previously impossible. Larger caseloads can be managed without a proportionate increase in staffing, potentially leading to more efficient and accessible legal services for a wider range of clients. Furthermore, the real-time insights generated by AI during document reviews allow attorneys to make more informed decisions about case strategy and potentially refine their arguments based on historical trends and patterns.
Another crucial point is that automated systems can potentially reduce human bias in legal analysis. By objectively evaluating all available information, these systems can help eliminate preconceived notions that might otherwise influence a human reviewer. This could lead to fairer and more equitable outcomes in legal proceedings.
While the complex language of legal documents poses a challenge for current NLP systems, advances in AI algorithms are allowing these tools to tackle ever-more sophisticated documents. The ability to analyze a broader range of texts allows for more comprehensive insights and potentially a more complete picture of the circumstances surrounding a case.
Furthermore, AI's capabilities are being used to predict the outcomes of legal cases based on analysis of past verdicts. This ability allows attorneys to fine-tune their strategies and arguments in a way that could lead to higher success rates, further enhancing the value of AI in law.
The adoption of AI within law firms is fostering collaboration and streamlining workflows. Document analysis and research become more centralized, leading to easier knowledge sharing and efficient synchronization of legal strategies across teams. Furthermore, these tools can aid in risk assessment by evaluating countless historical cases to identify potential legal risks associated with specific strategies, allowing firms to make more informed decisions about case management and potential litigation avoidance.
The integration of AI also creates new training needs for legal professionals, requiring them to adapt and acquire new technical skills. This creates a demand for ongoing education and fosters a culture of continuous learning within the industry, ensuring that lawyers remain competitive in this evolving technological landscape. It is important to remain critical of this development in the legal field, considering both the potential advantages and limitations of AI in the context of legal practices. While the future of AI in law is still being written, the clear trend is one of increasing adoption and transformative potential across various aspects of legal practice.
AI-Powered Legal Analytics Breaking Down Property Theft Cases in Texas Through Machine Learning Patterns - Machine Learning Algorithms Calculate Property Crime Settlement Values Based on Past Cases
In the evolving landscape of legal practices, particularly in property crime cases, machine learning algorithms are increasingly used to estimate settlement values. These algorithms analyze historical data from past property theft cases, identifying patterns and trends that inform the determination of fair settlements in new instances. This approach not only deepens our understanding of property crime trends in Texas but also contributes to more informed resource allocation and crime prevention strategies.
However, the reliance on historical data presents potential pitfalls. Biases that may exist within the datasets used to train these algorithms could lead to unfair or discriminatory outcomes if not carefully monitored and addressed. It's critical to acknowledge this inherent risk and continuously evaluate the fairness and equity of AI-driven settlement calculations. The increasing integration of AI in the legal field demands a cautious approach that balances the advantages of automation and efficiency with the imperative to ensure that these advancements don't inadvertently worsen existing inequities within the justice system. The evolution of AI in legal processes necessitates ongoing critical assessment and adaptation to guarantee that technological advancements are applied responsibly and ethically.
Machine learning algorithms are increasingly being used to analyze past property crime cases in Texas, enabling quicker processing and analysis of legal documents compared to traditional methods. This can significantly reduce the time it takes to review thousands of documents, potentially shaving weeks or even months off the process.
These algorithms, when trained on historical data, can improve the accuracy of predicting case outcomes by around 30% compared to traditional methods. This improved accuracy gives legal teams better insights to guide strategic decision-making related to case management.
During document review, advanced AI systems can provide real-time insights, allowing legal teams to immediately identify and respond to new evidence as it's discovered. This dynamic approach can be a game-changer in developing and adapting case strategy.
The adoption of AI in law has led to notable reductions in operational costs for some firms, with reported savings reaching as high as 40%. These cost savings could potentially translate into increased profitability for firms and, in some cases, lower fees for clients.
Implementing AI in legal contexts requires collaboration between legal professionals and data scientists, creating a new interdisciplinary field. This collaboration can improve both the quality of legal analysis and the application of technology in legal environments.
Modern AI models utilize a continuous learning process, refining their predictive algorithms as they encounter new data. This allows them to adapt and stay relevant as criminal patterns shift over time, ensuring their predictions remain accurate.
AI systems are expanding beyond formal legal documents to analyze informal communication, like emails and texts. This broadened scope of analysis can unlock valuable insights that may have a significant impact on case outcomes.
While the use of historical data for predictive analysis offers benefits, it also raises concerns about perpetuating biases that might exist within the legal system. It's crucial to remain vigilant and continue refining the algorithms to mitigate these risks.
The complex nature of legal language continues to present a challenge for AI. Models can struggle with the nuances and specific vocabulary of legal contexts, highlighting the need for ongoing advancements in natural language processing.
As AI automates more aspects of legal research and analysis, the demand for adaptable legal professionals is increasing. It's becoming clear that lawyers need to continually learn new skills and adjust their skill sets to navigate a shifting legal landscape where traditional roles may change.
Automate legal research, eDiscovery, and precedent analysis - Let our AI Legal Assistant handle the complexity. (Get started for free)
More Posts from legalpdf.io: