AI-Powered Legal Analysis Examining the Automated Detection of Section 3 Violations in Digital Evidence
AI-Powered Legal Analysis Examining the Automated Detection of Section 3 Violations in Digital Evidence - Machine Learning Models for Electronic Evidence Classification in Legal Cases 2024
The year 2024 sees a growing emphasis on employing machine learning models to classify electronic evidence within legal proceedings. These models are becoming more sophisticated, utilizing techniques like natural language processing and deep learning to sift through the massive quantities of unstructured data common in digital evidence. This has the potential to significantly enhance the speed and efficiency of evidence review. However, the reliability of these models is inherently tied to the quality and abundance of training data used to develop them. If the data is insufficient or biased, it can lead to inaccurate and unreliable results, impacting the trust and dependability of AI-driven outcomes. Further complexities arise when attempting to incorporate the intricate and often implicit knowledge legal experts rely on into machine learning frameworks. This intricate knowledge, crucial for understanding legal nuances, is not easily translated into a format AI systems can readily comprehend. Because of this, it's paramount that any AI system employed in legal contexts is subject to rigorous monitoring and evaluation to ensure its responsible application and avoid jeopardizing the fairness and accuracy of legal decisions.
1. **Data Variety's Impact:** The effectiveness of machine learning models in classifying electronic evidence hinges on the diversity of the training data. By incorporating a broad spectrum of data formats – emails, documents, multimedia, etc. – the models can develop a more robust understanding of patterns, leading to more accurate classification.
2. **NLP: Bridging the Language Gap:** Natural Language Processing (NLP) is becoming increasingly pivotal in eDiscovery. Legal language is complex, and NLP allows AI models to parse and comprehend this specialized language, thus extracting crucial insights from large datasets that might otherwise be missed by traditional methods.
3. **Predictive Coding: Streamlining Review:** Predictive coding algorithms are revolutionizing the document review process. By training on a subset of representative documents, these algorithms predict the relevance of the remaining data, significantly reducing the volume of documents needing manual review. Estimates suggest that this can lead to a reduction of 50-90% in manual work.
4. **Cost Reduction Potential:** There's growing evidence that the application of machine learning in legal document review significantly reduces costs. Lawyers spend less time on irrelevant data, resulting in potential savings that can range from thousands to millions in extensive cases.
5. **Minimizing Human Error:** Emerging research suggests that machine learning tools can help achieve lower error rates in document classification when compared to conventional review methods. This makes them a potentially valuable asset in intricate legal proceedings where accuracy is paramount.
6. **Contextual Understanding:** Advanced machine learning models are becoming capable of discerning the context within communications, such as interpreting intent within emails. This granular understanding can be crucial in identifying specific evidence supporting or refuting a case.
7. **Adaptability Through Continuous Learning:** EDiscovery systems that leverage machine learning can adapt to evolving legal landscapes and case specifics over time. These systems can learn from new data, enhancing their long-term effectiveness beyond their initial training.
8. **Human-AI Collaboration**: While AI can accelerate document classification, the intricacies of legal nuances and context sometimes remain beyond its grasp. The most effective approach appears to be a collaborative one, integrating machine learning tools with the expertise of legal professionals.
9. **Bias Risks:** It's crucial to acknowledge the potential for bias in machine learning models. If the training data is skewed or lacks representation, the models might inadvertently reflect and even exacerbate existing biases in the classification process. This potential bias carries serious implications in legal settings, highlighting the need for careful consideration when selecting and curating datasets.
10. **Real-Time Insights for Faster Decisions:** Some machine learning applications in legal settings offer real-time analytics and alerts during investigations. This capability enables lawyers to react quickly to emerging situations and to make more informed decisions in cases where timely action is crucial.
AI-Powered Legal Analysis Examining the Automated Detection of Section 3 Violations in Digital Evidence - Automated Document Analysis Detecting Section 3 Violations across Large Digital Archives
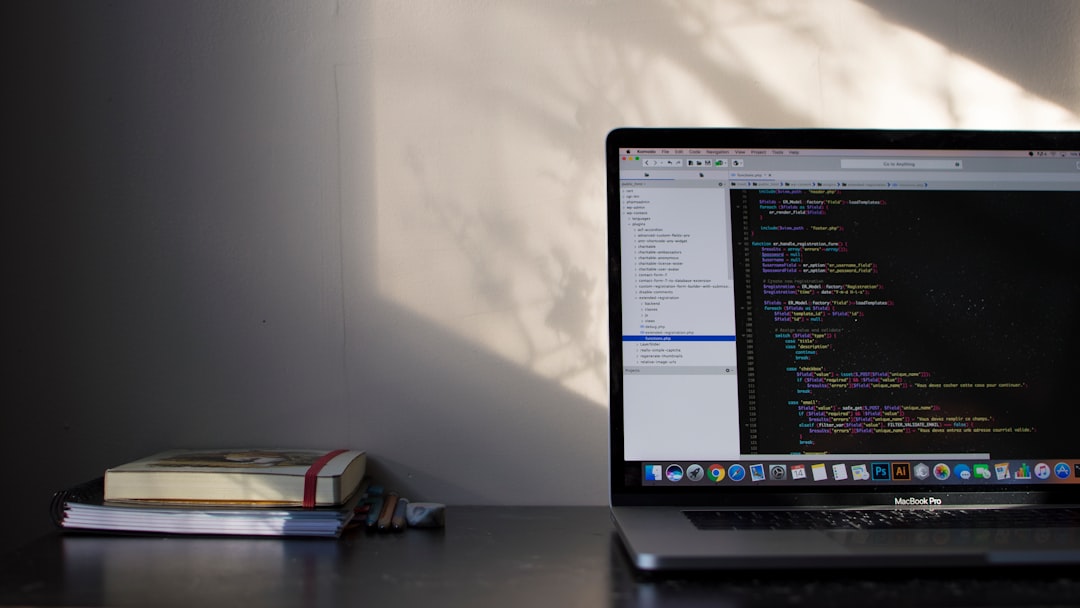
Automated document analysis is changing how legal professionals handle the task of uncovering Section 3 violations within extensive digital archives. These AI systems, powered by machine learning and natural language processing, can rapidly analyze massive amounts of legal documents. They pinpoint key clauses, potentially problematic areas, and highlight potential violations with a level of speed and accuracy that surpasses traditional manual review processes. The ability of AI to identify inconsistencies and automatically extract essential information lessens the likelihood of human errors, making it a potentially useful tool for legal research and eDiscovery procedures. However, the increasing reliance on these automated systems underscores the need for vigilance in mitigating biases present in the training data used to develop the AI. This is crucial to ensure that the outcomes generated by AI adhere to the principles of fairness and reliability that are vital in legal contexts. This technology has introduced a significant shift toward a more effective and intelligent method for analyzing legal documents, promising a new era in this domain. While these advancements are promising, caution is still warranted due to the complex and nuanced nature of legal matters.
AI's role in legal research and document review within large law firms is rapidly evolving, especially in the realm of eDiscovery and document creation. AI algorithms are increasingly capable of scrutinizing vast digital archives for legal relevance, moving beyond basic keyword searches towards a deeper understanding of legal language through semantic analysis. This ability to interpret the meaning of legal terminology and phrases is vital in pinpointing sections of documents relevant to specific legal requirements, potentially saving significant time and effort during the discovery process.
Interactive document review tools, powered by AI, have emerged, fostering a more dynamic experience compared to traditional methods. Lawyers can now pose questions directly to the AI system and receive prompt feedback, highlighting relevant sections within voluminous documents. This interactive approach is a valuable asset in streamlining the discovery process and reducing the time spent on manually sifting through irrelevant materials.
Furthermore, AI is being employed to predict potential legal failures or violations before final submissions. By flagging documents that potentially contain issues like Section 3 violations during early stages, legal teams can implement a proactive, rather than reactive, approach. This preemptive strategy can help prevent costly delays and complications later in legal proceedings.
Scaling legal operations is another benefit. AI-powered systems are well-suited to managing the extensive digital archives common in large law firms. This scalability ensures that consistent legal standards are applied across multiple cases, fostering uniformity in compliance and assessment.
However, despite these advancements, there's a growing acknowledgment that the sheer volume of data and complexity of legal knowledge sometimes remain a challenge for AI. The reduction of cognitive load for legal professionals, while a positive outcome, requires a nuanced approach. AI should augment human expertise, not replace it. There's a need to ensure the human element is always present, particularly in areas requiring expert judgment or complex legal interpretations.
Transparency in AI-driven decision-making is also crucial for maintaining trust. While some systems are built with transparent mechanisms, showcasing the reasoning behind their conclusions, others remain opaque. Developing trustworthy AI in the legal sphere demands that such systems are designed to provide detailed explanations for their output.
Moreover, the integration with cloud services and the creation of comprehensive audit trails are important developments. AI tools embedded within cloud platforms enable real-time access to case files, supporting seamless collaboration among geographically dispersed teams. Simultaneously, the comprehensive audit trails generated by these tools bolster accountability and regulatory compliance.
However, a persistent concern is the potential for bias in AI models. The training data used to develop these systems can inadvertently reflect and perpetuate biases, leading to skewed results in legal analyses. Careful consideration during data selection and curation is essential to minimize this risk and ensure fairness in legal outcomes.
In essence, while AI holds substantial promise in revolutionizing legal practices, it's crucial to approach its implementation critically and with a clear understanding of its limitations. A balance between human expertise and AI's capabilities is key to leveraging AI's benefits while mitigating potential risks. As AI's role in law evolves, continued research and development are needed to ensure that it serves as a tool for improving legal processes, rather than compromising their core principles of fairness and accuracy.
AI-Powered Legal Analysis Examining the Automated Detection of Section 3 Violations in Digital Evidence - Predictive Analytics Integration with Traditional Legal Discovery Methods
The fusion of predictive analytics with established legal discovery methods represents a significant change in how legal professionals approach the examination of evidence. AI empowers lawyers to process massive amounts of information far more efficiently than manual review, improving the accuracy of the process and lessening the burden on legal teams. This blending of AI and traditional methods not only streamlines legal operations but also allows for more strategic decision-making by using historical data to anticipate potential case outcomes. However, increased reliance on AI brings its own set of complexities, like concerns about bias in the data underpinning these systems. There's a crucial need for careful oversight to guarantee fairness in the legal process. The legal world faces a challenge in thoughtfully integrating this new technology while still valuing the critical role of human legal expertise in complex legal matters. Balancing AI's potential with the need for expert legal judgment will be key to reaping the benefits while mitigating the potential risks.
AI's integration with traditional legal discovery methods, particularly in eDiscovery, is reshaping how legal professionals approach document review and analysis. Predictive analytics can significantly streamline the review process, potentially reducing the time spent on manual review by a considerable margin. This shift allows legal teams to focus more on higher-level strategic tasks, which traditionally have been hampered by the sheer volume of documents involved.
However, this potential for efficiency is not without its caveats. The effectiveness of these AI-driven tools is profoundly linked to the quality of the training data they're built upon. Using poor quality or irrelevant data can lead to flawed results, potentially misclassifying critical documents. This highlights the need for meticulous curation of training datasets, an issue that continues to challenge the implementation of AI in law.
One of the intriguing possibilities is the potential for AI to automate the application of legal principles. Complex legal concepts can be incorporated into algorithms, allowing these systems to identify patterns and provide insights far beyond the capacity of traditional manual methods. This shift in the burden from tedious analysis to machine-driven insights is a potential game-changer for legal practice.
Moreover, these AI systems are often equipped with adaptive learning abilities. They can refine their performance as new data becomes available, allowing them to dynamically adjust to the nuances of a specific case. This capacity is crucial in maintaining accuracy throughout the discovery process as cases evolve.
The impact of these efficiency gains extends to law firm finances. The reduction in the time spent on document review can translate into substantial reductions in billable hours, potentially lowering client costs. This could reshape how law firms manage client relationships, potentially moving towards value-based billing models rather than traditional hourly billing.
However, caution must be exercised because AI systems are prone to biases present in their training data. This means AI-driven legal analyses can inadvertently perpetuate biases and produce unfair outcomes. Addressing this bias is a continuous challenge, demanding ongoing evaluation and calibration of these AI tools.
Interestingly, categorizing documents into specific types (like contracts, emails, or reports) can enhance predictive accuracy. Utilizing different statistical techniques for different document types allows algorithms to improve the accuracy of classification and relevance rankings.
Despite these advancements, human oversight is indispensable. A balance between AI automation and human review is vital for ensuring the reliability and compliance of the legal analyses produced. This collaboration is needed to validate AI-generated results against established legal standards and ensure their accuracy.
The evolving landscape of AI in law is also driving a transformation in the required skill set for legal professionals. The rising demand for data science expertise is causing law schools to integrate data analytics into their curricula. The next generation of lawyers needs proficiency in both law and data to function effectively.
Furthermore, the integration of AI with other technologies, like blockchain for contract management or enhanced security protocols for handling sensitive data, holds potential for even greater optimization in legal practice. This creates a more robust and secure ecosystem for managing legal documents within the context of eDiscovery and other legal domains.
In conclusion, while the integration of predictive analytics into traditional legal discovery methods has immense promise, it's essential to acknowledge its limitations. AI's role in law should be to augment human capabilities, not replace them. Achieving the best outcomes will require continuous critical evaluation, careful integration of AI tools, and ongoing research to ensure that AI’s application in law benefits legal practices without compromising core ethical and fairness standards.
AI-Powered Legal Analysis Examining the Automated Detection of Section 3 Violations in Digital Evidence - Digital Evidence Authentication through Natural Language Processing
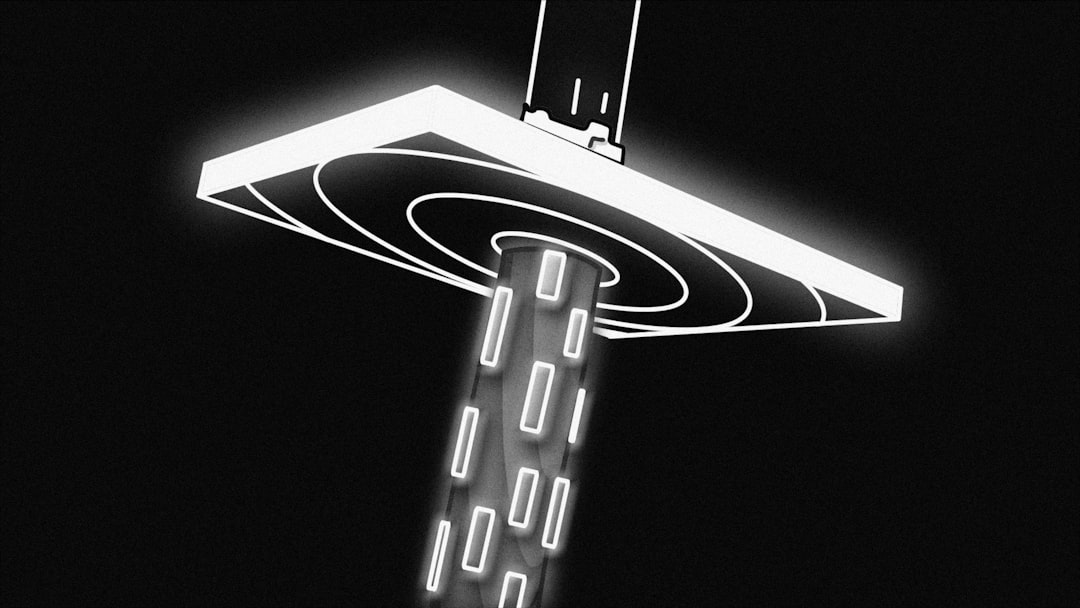
The increasing reliance on digital evidence in legal proceedings has brought to the forefront the critical need for robust authentication methods. Natural Language Processing (NLP) is emerging as a powerful tool in this area, offering the potential to enhance the authentication process for a wide range of digital evidence. NLP's capacity to analyze complex legal language and extract meaningful insights from massive datasets is particularly valuable in the context of increasing concerns surrounding issues like deepfakes and manipulated digital information. With the capability to identify patterns, inconsistencies, and other relevant details within digital data, NLP can assist in verifying the authenticity and integrity of electronic evidence.
However, the integration of NLP into the legal field for authentication purposes isn't without its challenges. Biases inherent in the AI models can lead to flawed outcomes, highlighting the need for careful oversight and a balanced approach that combines human legal expertise with AI capabilities. The legal system must carefully consider the potential ramifications of increased reliance on AI for evidence authentication, ensuring that these tools are used responsibly and do not undermine the fundamental principles of fairness and accuracy in the pursuit of justice. While NLP shows promise in improving the authentication process for digital evidence, it's imperative that human expertise remains central to the evaluation and interpretation of this crucial type of evidence.
Digital evidence authentication within the legal system is increasingly complex due to advancements in AI. While traditional authentication relied on circumstantial evidence like appearance and content, AI introduces new challenges like the potential for manipulated or synthetic data. Organizations like the National Center for State Courts are providing guidance on these issues, particularly with the emergence of deepfakes and the need for robust authentication procedures.
Natural Language Processing (NLP) plays a vital role here, improving the ability of AI to understand legal documents and extract crucial information. However, the capacity of NLP to comprehend legal jargon and nuanced language across different jurisdictions is still developing, especially with the variety of legal terms and specific legal frameworks. Researchers are working on algorithms that can refine their understanding by accounting for these variations, which will be crucial for ensuring accuracy in the face of regionally distinct legal language.
Furthermore, AI's use in risk assessment is evolving. While still in its nascent stages, AI-powered tools can identify documents that potentially raise compliance concerns, such as potential Section 3 violations. This proactive approach can help avoid issues before they lead to significant problems, though relying on AI for such assessments requires careful consideration and ongoing monitoring to avoid biased outcomes. It’s encouraging that in predicting case outcomes, AI coupled with historical data has been shown to improve accuracy, but there’s still a lot of work to be done in quantifying the exact improvements and mitigating the inherent biases that might influence predictions.
The effectiveness of AI in legal contexts depends significantly on the synergy between AI capabilities and human legal expertise. Law firms that have successfully implemented AI tools that support, rather than replace, human legal professionals have reported increased efficiency in handling cases. It seems clear that human insight into the subtleties of legal contexts and interpretation remains valuable when using AI's results.
Advanced machine learning models are becoming more adaptive, incorporating mechanisms to continually learn from new information. As legal precedents shift or as the specific circumstances of a case unfold, AI can update its understanding of the relevant legal landscape. This dynamic adaptation is vital for ensuring AI-driven insights remain relevant, however, we need to understand how the various methods of continuous learning impact accuracy and generalizability.
AI's ability to classify documents by type—contracts, emails, or reports—can help tailor analyses to specific legal needs of those documents. This refined approach to document classification has shown promise in boosting the accuracy of relevance assessments. It's interesting to think how this focus on specific document types can improve outcomes, but the optimal algorithms for different document types are still being researched and developed.
The potential impact of AI on the economics of law firms is also profound. The time savings from AI-powered document review are beginning to reshape traditional billing structures. It seems law firms are transitioning to outcome-based or value-based pricing models in some cases, a substantial change that reflects the efficiency AI can bring.
Moreover, AI-powered document review generates detailed audit trails, vital for both compliance and maintaining transparent client relationships. This aspect of AI tools has significant implications for accountability and is crucial when managing sensitive data and meeting regulatory demands.
Finally, as AI's role expands within law firms, the education of future legal professionals must change. Law schools are recognizing the need to integrate data science and computational thinking into legal education to prepare the next generation of lawyers to effectively leverage AI and deal with the large volume of data now relevant to legal practice. As AI continues to evolve, integrating it with technologies like blockchain for contract management will only continue to transform the landscape of legal practice.
Overall, while the integration of AI into the legal field offers remarkable potential for streamlining and enhancing legal practice, it’s crucial to remain mindful of its limitations. The careful balance between human expertise and AI capabilities will be critical in harnessing AI's power while upholding the fundamental principles of fairness and accuracy. A critical approach to AI's integration into legal practice is required to optimize its benefits while managing potential risks and mitigating biases in the ongoing quest to make the legal system more efficient and just.
AI-Powered Legal Analysis Examining the Automated Detection of Section 3 Violations in Digital Evidence - Real Time Pattern Recognition in Electronic Court Filing Systems
The integration of real-time pattern recognition into electronic court filing systems signifies a notable shift towards automating previously manual processes. Software bots, now integral to these systems, excel at sorting and structuring data within existing case management software, simplifying the often complex task of filing. This automation not only increases the efficiency of the filing process but also frees up legal professionals to dedicate more time to core legal analysis and strategy. However, the reliance on AI to interpret legal documents and data introduces a layer of complexity. While speed and efficiency are gained, ensuring the AI can accurately and consistently grasp the intricate details and nuances of legal language remains a key concern. Maintaining accuracy and upholding fairness in legal outcomes necessitate ongoing vigilance in the development and implementation of these AI-powered systems. As we move forward, robust governance and continuous monitoring are essential to ensure these advancements support, rather than compromise, the fundamental principles of the legal system.
AI's influence on legal processes, particularly in the realm of eDiscovery and document analysis, is rapidly evolving. Electronic court filing systems are incorporating real-time analytical capabilities, allowing lawyers to monitor case developments and document submissions as they occur. This instant feedback can be vital for quick responses and adaptive legal strategies, particularly in high-stakes situations.
Furthermore, AI is enabling the tracking of digital document origins, which is becoming increasingly crucial for authenticating evidence in a world where manipulated data is a growing concern. Some systems are even using adaptive feedback loops where AI learns from user interactions to improve classification and analysis accuracy over time. This constant refinement is especially useful in adapting to specific legal contexts and nuances.
Collaborative document review tools are also emerging, powered by AI and designed to facilitate real-time interactions between legal teams, regardless of their location. These tools promote streamlined communication and decision-making, which can be very beneficial for larger firms with dispersed teams.
Beyond collaboration, AI is being integrated to automate compliance checks during document reviews. This proactive measure helps identify potential issues early on, reducing the risks associated with non-compliant submissions. In eDiscovery, advanced pattern recognition algorithms are aiding in identifying recurring themes or anomalies in large datasets, revealing hidden evidence or inconsistencies potentially missed by humans.
The way AI is changing document review also impacts client relationships. Clients gain increased transparency into the process, and firms can tailor their services based on a client's specific needs, which potentially fosters a stronger bond. Moreover, some AI systems offer real-time risk assessment for cases, enabling quicker decision-making, especially in time-sensitive situations.
Interestingly, these tools aren't solely for large firms. Boutique firms are also finding them useful for managing substantial volumes of digital evidence effectively. This democratization allows smaller firms to compete more efficiently in complex cases.
The marriage of AI and blockchain technology is also promising, particularly for ensuring evidence integrity. Using blockchain for document verification allows for tracking any alterations to digital evidence, enhancing its trustworthiness in court.
While these advancements show promise, it's crucial to acknowledge the potential pitfalls of AI in legal contexts, such as the inherent risk of bias in the training data. Finding the balance between AI's potential and human expertise will be essential for reaping the rewards while mitigating risks. As with any technological advancement, constant vigilance and a cautious approach to implementation are vital for AI's positive impact on the legal system.
AI-Powered Legal Analysis Examining the Automated Detection of Section 3 Violations in Digital Evidence - Algorithmic Bias Detection Frameworks in Legal Evidence Analysis
The increasing use of AI in legal processes, such as eDiscovery and legal research, has raised significant concerns about the potential for algorithmic bias within these systems. This is particularly relevant when AI is used to process sensitive information or make decisions that impact individuals. While AI offers advantages in terms of efficiency and speed, the reliance on training data can lead to the perpetuation of existing biases, ultimately influencing the results. To ensure fairness and trust in AI-powered legal analysis, there's a critical need for robust algorithmic bias detection frameworks. These frameworks are designed to proactively identify and address biases that might be embedded within AI models.
The development of such frameworks is driven by the need to maintain public trust and uphold the integrity of legal outcomes. Transparency and fairness are paramount, and these frameworks are designed to build confidence in the way AI is utilized in legal contexts. Many studies have examined algorithmic bias from different angles, looking at both statistical methods and qualitative analysis. There is, however, a growing understanding that the legal field presents unique challenges when it comes to identifying and mitigating bias.
Furthermore, as discussions surrounding algorithmic decision-making in the legal system become more prominent, particularly in relation to discrimination cases, the development and implementation of fairness-aware AI techniques becomes increasingly critical. Strategies like data augmentation are being explored as a means of reducing bias in AI models. These areas of research are crucial as we continue to develop AI systems that are both powerful and ethical in their application.
Ultimately, a critical examination of the intersection between AI and law is crucial for the future of legal practice. While the benefits of AI in areas like document review are undeniable, we need to approach this technology with a healthy dose of skepticism, acknowledging the potential for bias and harm. The continued exploration of methods to identify and address bias in AI is essential for fostering greater trust in AI-powered legal systems and ensuring that technology serves the principles of justice and fairness.
1. **The Importance of Training Data:** The reliability of systems designed to detect algorithmic bias relies heavily on the quality and inclusiveness of the data used to train them. If the training data is limited or contains existing prejudices, the system itself might reinforce these biases, leading to unfair or inaccurate legal conclusions.
2. **The Challenge of Legal Language:** AI algorithms often struggle to fully grasp the intricacies and nuances of legal terminology and context. This can cause misunderstandings and misinterpretations, making it crucial for legal experts to remain actively involved in the process to ensure accurate understanding of the subtleties of legal language.
3. **Bias Amplification Risk:** Many algorithmic bias detection tools have the potential to unknowingly amplify the biases already present in the data they are trained on. By replicating existing biases, these systems could inadvertently create more pronounced inequalities in legal outcomes based on factors like socioeconomic background or race.
4. **Jurisdictional Variations in Frameworks:** The effectiveness of bias detection systems can vary across different jurisdictions because legal standards and terminology can differ substantially. This lack of uniformity raises questions about the general applicability and overall effectiveness of AI systems across diverse legal systems.
5. **Collaboration Between Humans and AI:** To effectively identify bias in legal settings, robust collaboration between AI and legal professionals is vital. Legal teams can contribute essential feedback that helps AI algorithms refine their models, ensuring that the outputs align with ethical principles and legal requirements.
6. **Real-Time Bias Detection Potential:** Advanced AI systems hold the possibility of analyzing data in real-time during legal proceedings. This allows for the immediate detection of emerging biases within evidence as it's presented, which could change the direction of a case quickly.
7. **Need for Oversight and Transparency:** Implementing monitoring systems is essential to observe and understand AI behavior. Regular audits of the system's performance can help ensure compliance with legal standards and mitigate potential risks associated with biased results.
8. **The Value of Diverse Perspectives:** Involving a diverse group of individuals—including legal professionals, ethicists, and data scientists—during the development of bias detection frameworks can significantly improve their effectiveness. This inclusive approach helps address a wider range of viewpoints and minimizes potential blind spots in the design and implementation of the system.
9. **Learning from Past Legal Decisions:** AI can use historical legal data to identify recurring patterns of bias or inequity in previous rulings. This retrospective analysis not only helps identify current biases but also strengthens the system's ability to anticipate potential biases in future legal cases.
10. **Ethical Considerations of Automation:** As algorithmic bias detection becomes more integrated into legal processes, the ethical considerations surrounding its use become increasingly important. Legal practitioners need to carefully examine the reliance on these automated systems, finding a balance between efficiency and the fundamental principles of justice and fairness.
More Posts from legalpdf.io: