eDiscovery, legal research and legal memo creation - ready to be sent to your counterparty? Get it done in a heartbeat with AI. (Get started for free)
AI-Powered Document Analysis Revolutionizing Discovery in Insurance Litigation by 2024
AI-Powered Document Analysis Revolutionizing Discovery in Insurance Litigation by 2024 - AI-driven eDiscovery reduces document review time by 60% in insurance litigation
The application of AI within eDiscovery processes is transforming insurance litigation, with the potential to slash document review time by as much as 60%. This advancement facilitates the rapid analysis of extensive document sets, freeing up legal professionals to concentrate on more complex and valuable work, ultimately boosting productivity within law firms. AI-powered eDiscovery not only expedites document analysis but also delivers a more uniform assessment compared to human reviewers, enabling even less experienced lawyers to contribute meaningful work sooner. This consistency and efficiency can lead to demonstrable improvements in both legal workflows and outcomes. The continued evolution of AI tools is poised to significantly influence how law firms handle tasks involving document management and review, influencing everything from due diligence to legal research. While concerns remain about the reliability and biases inherent in AI systems, the potential for streamlined processes and improved consistency is undeniable and promises to reshape the legal field.
In the realm of insurance litigation, the application of AI in eDiscovery has demonstrably shown the potential to expedite the document review process. Research suggests that AI can decrease the time spent on this crucial step by as much as 60%, a compelling finding. This is achieved through the sophisticated algorithms used in AI that efficiently categorize and analyze extensive datasets of legal documentation, effectively pinpointing key information much more swiftly than traditional manual review methods.
While human reviewers often grapple with the sheer volume and complexity of legal documents, AI tools can sift through hundreds of pages with significantly improved speed. This isn't just about speed, though. Studies have shown that the error rate associated with AI-driven document analysis can, in some instances, be lower than human error rates, raising questions about the accuracy and reliability of traditional approaches.
The ability of AI to learn and adapt through machine learning techniques is another significant advantage. These systems continually refine their performance through feedback from human reviewers, incrementally improving their accuracy and efficiency in the process of identifying pertinent documents. This iterative process suggests a future where AI plays an even more central role in eDiscovery.
The financial implications of these advancements are also worth noting. Reduced review times directly lead to a decrease in the amount of lawyer and paralegal time required, translating to substantial cost savings for law firms. This economic benefit is another driver of AI adoption in legal practices.
Moreover, AI doesn't simply accelerate the review process; it can also prioritize documents intelligently based on context and relevance. This functionality ensures that legal teams focus their energy and resources on the most critical documents, further streamlining the entire discovery process.
Interestingly, AI systems leverage natural language processing (NLP) to glean key information and extract insights from documents that would normally require hours of manual review. This highlights the potential of AI to move beyond simple document categorization and into a realm of providing valuable, condensed insights for attorneys.
The implications for fairness and bias are also intriguing. By automating the document analysis process, AI potentially diminishes the impact of human bias, thereby suggesting a path towards more equitable legal outcomes. However, the inherent biases present in training data used to develop AI models remain a concern that needs to be continuously addressed.
This heightened focus on AI in eDiscovery has driven rapid growth in the number of legal technology companies specializing in these solutions. This indicates a broader trend in the legal profession, where integrating AI is increasingly viewed as essential for maintaining efficiency and competitiveness.
One interesting consequence of AI integration is its influence on the training and development of new lawyers. Young attorneys are now expected to be adept in using AI-driven tools for legal research and document management, requiring a shift in law school curricula and practical training. However, this raises the question of whether current legal education adequately prepares students for the demands of a technology-driven legal landscape.
Finally, we need to acknowledge the inevitable security concerns that arise with any system that handles sensitive client information. As law firms integrate AI into their processes, robust safeguards and measures are needed to ensure compliance with privacy regulations and protect the confidentiality of the data involved. Addressing these security concerns will be a vital aspect of AI's continued adoption in the legal domain.
AI-Powered Document Analysis Revolutionizing Discovery in Insurance Litigation by 2024 - Natural Language Processing streamlines analysis of complex insurance policies
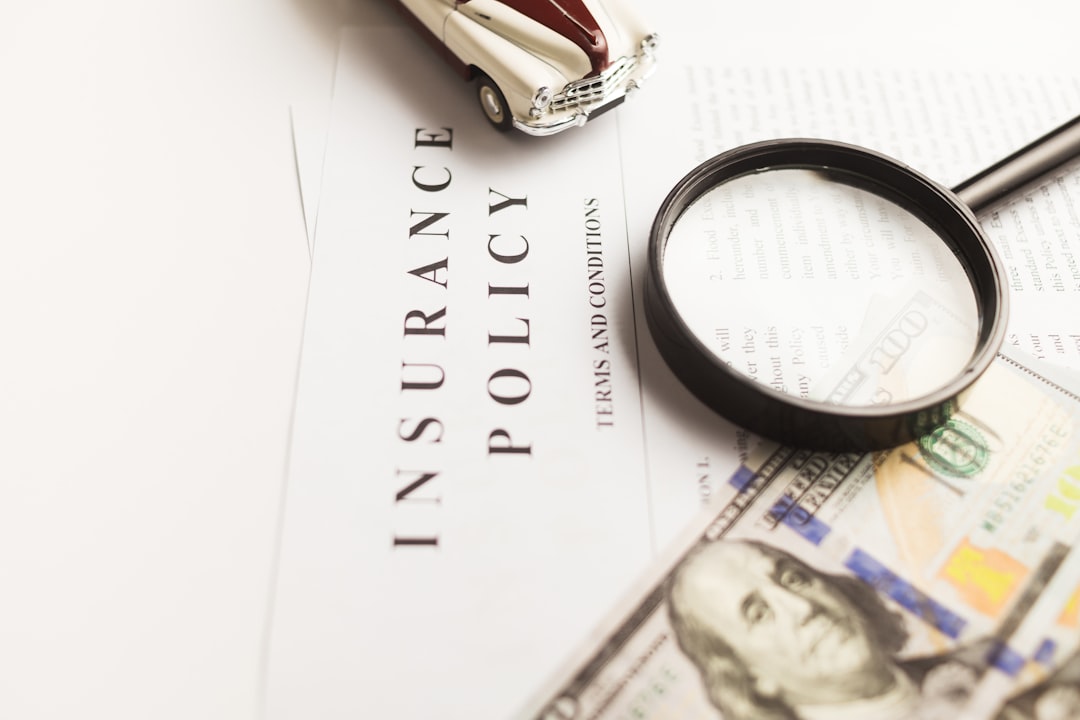
Natural Language Processing (NLP) is transforming the way insurance companies navigate the complexities of their policies. The intricate language and sheer volume of insurance documents have traditionally presented significant challenges. NLP tackles these issues by automatically processing policy details and streamlining claims processing, thereby increasing operational efficiency. Furthermore, by recognizing patterns in large datasets, NLP enables the identification of potential fraudulent claims, enhancing risk management within the insurance sector.
While human error remains a constant risk in manual analysis, NLP offers a more consistent and objective approach. This leads to faster, more reliable decision-making, ultimately contributing to improved client experiences and satisfaction. The integration of NLP into insurance operations reflects a broader shift towards automation and data-driven practices. As NLP technology continues to evolve, its influence on insurance practice, particularly in the context of litigation and dispute resolution, will become increasingly significant. The future of insurance likely involves a more prominent role for AI-driven insights and solutions.
Natural Language Processing (NLP) is proving to be a valuable tool in deciphering the often convoluted language found in insurance policies. It can swiftly pinpoint specific clauses and terms that are crucial for understanding the policy's intricacies, ultimately speeding up the process of gaining insights needed for negotiations during litigation. This accelerated understanding can potentially shave off precious time in the often-lengthy legal battles surrounding insurance disputes.
Some recent research suggests that NLP systems have the ability to detect subtle patterns embedded within legal language that could potentially indicate hidden problems or ambiguities within insurance policies. By spotting these patterns, law firms might be able to proactively identify and address potential litigation risks before they escalate into major issues. This preemptive approach to risk management could have substantial benefits in minimizing future legal conflicts.
One fascinating application of NLP is the creation of concise summaries of often lengthy and complex insurance policies. Instead of poring over hundreds of pages of dense legal text, lawyers can use AI to distill these policies into digestible summaries that encapsulate the key points. This capability has the potential to streamline the preparation phase for cases, allowing attorneys to focus on the most crucial aspects of the policy without getting bogged down in the details.
Surprisingly, AI systems that utilize NLP can even map out the relationships between different policy documents. This allows lawyers to quickly identify inconsistencies or overlaps that might not be apparent during a traditional review. Such an ability to cross-reference and analyze multiple documents simultaneously can be instrumental in developing stronger case strategies, ensuring no stone is left unturned in the pursuit of a favorable outcome.
NLP is also making its mark in legal research by significantly reducing the time it takes to locate relevant case law and precedents related to insurance litigation. This speedier access to related cases frees up lawyers to concentrate on crafting their legal strategies and communicating more effectively with their clients. A more focused approach to client interactions can, in turn, contribute to building stronger and more productive attorney-client relationships.
Analyzing historical claims data through NLP has unlocked a new avenue for gaining insights into how specific policy terms have been interpreted in previous court cases. By learning from past rulings, law firms can develop more predictive models for the likely outcomes of similar cases in the future. This ability to predict outcomes, based on historical data patterns, could significantly influence decision-making in insurance litigation.
Interestingly, in several studies, the accuracy of AI-assisted document review has been surprisingly high. In one study, AI outperformed even seasoned human reviewers in analyzing intricate insurance policies. This raises the question of whether traditional methods of document review may be less efficient or accurate than previously believed. The prospect of AI exceeding human performance in document analysis suggests a paradigm shift in how legal professionals approach certain tasks.
Law firms can leverage AI's ability to learn from past litigation outcomes by fine-tuning their document review procedures. This means AI can be trained to proactively identify specific terms or clauses that have historically led to unfavorable outcomes. This kind of targeted approach can enhance overall case management, optimizing efforts and resources for greater impact.
As AI systems become increasingly sophisticated, their potential applications extend beyond simple document review. They may eventually play a role in the actual drafting of insurance policies themselves. This presents an exciting opportunity for creating more easily understandable and straightforward policy language, benefitting both insurance companies and policyholders alike.
The shift towards AI-driven analysis has created a new wave of consumable legal advice formats. AI systems are now capable of generating summaries and insights that help clients understand the nuances of their insurance policies, empowering them with knowledge and fostering greater transparency. This democratization of legal knowledge through accessible and understandable AI-generated formats can potentially level the playing field between individuals and large insurance corporations.
AI-Powered Document Analysis Revolutionizing Discovery in Insurance Litigation by 2024 - Machine learning algorithms identify patterns in claim histories for fraud detection
Machine learning algorithms are increasingly vital in insurance for spotting patterns within claim histories that hint at fraud. These algorithms, using techniques like Decision Trees, Bayesian Classifiers, and Neural Networks, sift through complex data to uncover irregularities, such as forged documents or false billing, which contribute significantly to financial losses. While the primary focus has been on supervised learning to detect fraud, the potential of unsupervised methods to identify more intricate fraud schemes is largely unexplored. With AI-powered systems evolving, the accuracy and efficiency of fraud detection are poised to enhance, enabling insurance companies to proactively combat fraud and protect their assets from increasingly clever scams. This advancement is critical, as the high volume of fraudulent claims and associated rejection rates seen in places like Saudi Arabia reveal a need for stronger, more sophisticated fraud detection solutions.
1. **Identifying Hidden Patterns**: Machine learning algorithms are adept at uncovering subtle patterns within claim histories, which can reveal fraudulent activity that might escape human notice. This ability to recognize complex relationships within data is particularly useful for exposing intricate fraud schemes.
2. **Dynamic Risk Assessment**: By processing large volumes of claim data, machine learning can improve risk assessment models. This allows insurance companies to continuously refine their underwriting practices, adapting to shifting fraud trends and minimizing potential losses.
3. **Significant Financial Impact**: The insurance industry faces a substantial financial burden from fraud, estimated to be in the tens of billions annually. Machine learning offers a means to mitigate these losses, benefiting both insurers and policyholders.
4. **Geographic Fraud Trends**: AI can identify geographic patterns in fraudulent claims, pinpointing areas with a higher incidence of suspicious activity. This information assists insurers in focusing investigative resources where they are most needed, potentially preventing future fraud in specific regions.
5. **Shift to Proactive Detection**: Instead of simply reacting to fraudulent claims after they are submitted, some advanced machine learning systems can now analyze claims in real-time. This proactive approach allows for immediate flagging of suspicious claims, accelerating response times and enhancing fraud prevention efforts.
6. **Understanding Claimant Behavior**: Beyond simply analyzing claim transactions, machine learning can evaluate the behavioral patterns of claimants. Algorithms can learn from past behaviors, offering insurers a more nuanced understanding of potential risk factors and fraudulent intent.
7. **Textual Data Analysis**: The integration of natural language processing (NLP) allows for analysis of the language used in claim submissions. This enables the identification of inconsistencies or unusual wording that might indicate fraudulent activity, providing another layer of scrutiny.
8. **Potential for Reduced Bias**: While the issue of bias in algorithms is a valid concern, careful design of machine learning systems can potentially minimize the biases present in traditional human-based risk assessments. This could lead to fairer and more equitable outcomes in claim processing.
9. **Leveraging Past Case Law**: Machine learning can analyze the outcomes of past insurance litigation and similar claims to inform future legal strategies. By learning from historical data, legal teams can better predict how courts might interpret specific claims, which can significantly influence litigation approaches.
10. **Continuous Learning and Adaptation**: Machine learning models can be continuously retrained with updated claims data, ensuring that the algorithms adapt to evolving fraud tactics. This ability to learn and improve over time is critical for maintaining the effectiveness of these systems in a constantly changing environment.
AI-Powered Document Analysis Revolutionizing Discovery in Insurance Litigation by 2024 - Cloud-based AI platforms enhance collaboration among distributed legal teams
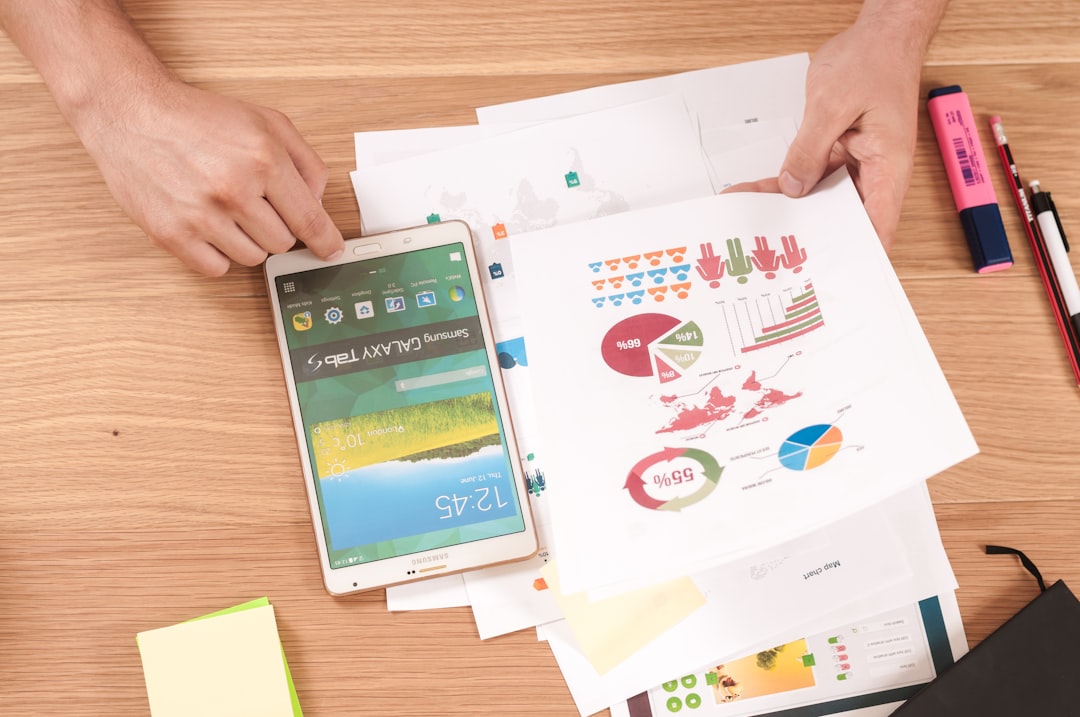
Cloud-based AI platforms are transforming how geographically dispersed legal teams work together, especially in areas like document review for insurance cases. These platforms allow for simultaneous access and sharing of research materials, which improves cooperation among team members. Even if lawyers are working remotely, they can contribute effectively. AI features within these platforms streamline the work process, allowing lawyers to focus on the more demanding aspects of their job while AI handles the intricate aspects of document analysis. This not only makes things more efficient but also leads to a more consistent evaluation of large document sets, decreasing the need for external help. As AI continues to shape legal practices, its role in promoting collaboration and improving communication amongst legal professionals will be crucial for navigating the complexities of modern litigation. There are however, still concerns about the accuracy and fairness of AI, particularly with the inherent bias that can arise from the data AI platforms are trained on. These are aspects that need constant monitoring and reassessment in order for the field to develop ethically.
Cloud-based AI platforms are fostering a new era of collaboration, particularly within geographically dispersed legal teams. The ability to access and share research data in real-time across different locations breaks down traditional barriers to knowledge sharing. While some might raise concerns about the potential security risks of storing sensitive legal information in the cloud, the benefits of streamlined communication and simultaneous document review appear compelling.
AI's influence extends to how legal teams manage eDiscovery. These cloud-based platforms now enable in-house document review, a departure from the traditional reliance on third-party vendors. This internalization of the review process can potentially lead to cost savings and improved control over sensitive data. However, successfully transitioning to in-house solutions requires not only technological infrastructure but also the development of internal expertise and robust security protocols.
Natural Language Processing (NLP) is becoming an increasingly valuable tool in the arsenal of legal professionals. It significantly enhances the ability to extract meaningful insights from the voluminous documents involved in discovery. It empowers legal teams to quickly identify relevant information within large datasets, offering a significant efficiency boost. While the accuracy and unbiased nature of NLP still requires scrutiny, its speed and efficiency for preliminary analysis are undeniable.
The shift towards cloud-based eDiscovery solutions reflects a larger trend in legal practice. It underscores the evolving technological landscape within the industry and how firms are adopting innovative tools to improve efficiency. This, however, raises questions about the adaptability of current legal training. Do law schools adequately prepare future legal professionals for an environment where AI plays a central role in eDiscovery and document management? The demand for a technically-savvy workforce suggests that adapting curricula may be necessary to meet the evolving needs of the legal field.
The incorporation of AI within legal workflows extends beyond document analysis and eDiscovery. It's also transforming legal writing and research. AI-powered tools, for instance, can now perform initial fact-checking, freeing up lawyers to focus on higher-level strategic tasks. This type of automation reduces the administrative burden on junior lawyers and allows them to dedicate more time to developing legal arguments and building complex cases. While AI shows great promise in automating specific tasks, ethical and legal considerations regarding its role in creating legal arguments will likely require careful attention and ongoing scrutiny.
Furthermore, AI is increasingly being leveraged in predictive analytics tools. By analyzing data patterns from past cases, these tools can potentially provide insights into the probable outcomes of current legal disputes. This can aid legal research and shape strategic decision-making. Although this remains an emerging field, the prospect of gaining an advantage by predicting court outcomes has huge potential for influencing the course of litigation.
Generative AI is also starting to make its mark in legal practice. Its capacity for improving legal drafting and generating legal documents is improving the efficiency of tasks that previously were time-intensive. However, the question of reliability and ensuring legal accuracy when AI generates legal documents remains a key area of focus. It will be interesting to observe how the legal profession addresses these concerns and develops standards for validating AI-generated legal texts.
Beyond these specific applications, AI's impact on collaboration within legal teams is profound. AI-powered communication and coordination tools enhance productivity among lawyers working remotely or in hybrid work environments. The flexibility and accessibility of these tools support a more adaptable work environment, which is becoming increasingly important.
The evolution of eDiscovery towards cloud-based and AI-powered solutions is transforming the legal landscape. Historically, eDiscovery was time-consuming and costly, requiring considerable manual intervention. The shift highlights the continuous drive within legal practices to optimize processes, increase efficiency, and ultimately, reduce costs. But with the evolving role of AI in legal practice comes a new set of concerns and challenges related to data security, ethical considerations, and adapting legal education. Navigating these new realities will be a defining feature of the legal profession in the years to come.
AI-Powered Document Analysis Revolutionizing Discovery in Insurance Litigation by 2024 - Predictive coding improves accuracy of privilege reviews in high-stakes cases
Predictive coding is emerging as a valuable tool for improving the accuracy of privilege reviews, especially in complex legal cases with high stakes. Leveraging machine learning, it helps minimize human error and bias, enabling swifter identification of privileged information within large document sets. This can lead to a substantial reduction—as much as 70%—in the number of documents that need manual review by lawyers, resulting in significant cost savings during eDiscovery. While these efficiency gains are appealing, legal professionals still hold reservations. There is a lingering uncertainty about how courts will view documents identified through this automated process. As AI-driven technologies mature, the potential for transforming discovery through predictive coding and related methods is significant, potentially becoming a cornerstone of modern legal practices.
1. **The Rise of Predictive Coding:** Predictive coding, powered by machine learning algorithms, is transforming how legal teams approach document review, specifically for privilege assessment. It automates the classification of documents based on relevance and privilege, effectively lightening the cognitive load on human reviewers. This allows lawyers to focus on fewer, more critical documents, leading to a streamlined process and improved overall case outcomes.
2. **Challenging Traditional Assumptions:** Interestingly, research suggests that AI-driven document review using predictive coding can have lower error rates compared to experienced human reviewers. This observation prompts us to reconsider the inherent assumption that human insight is always superior in document analysis, especially in the face of large and complex datasets.
3. **Continuous Learning and Refinement:** Predictive coding models continuously improve with feedback from human reviewers. This ongoing learning process leads to a gradual increase in the accuracy and efficiency of privilege reviews. This iterative approach suggests that the quality of legal analysis can be significantly enhanced through repeated application and feedback loops.
4. **Time Savings in Complex Matters:** The integration of predictive coding into the discovery process, particularly for privilege review, can drastically reduce the time spent on these tasks—potentially by up to 75% in high-stakes cases with significant volumes of documents. Such efficiency gains are particularly valuable in situations with stringent deadlines or a large body of evidence to analyze.
5. **Proactive Risk Management:** Predictive coding not only identifies relevant documents but also highlights those with a high probability of containing sensitive or privileged information. This automated risk awareness is crucial in complex litigation, where the risk of unintentional disclosure during discovery is a constant concern. This proactive approach can help minimize legal complications.
6. **Empowering Junior Lawyers:** By automating a significant portion of the initial document review process, predictive coding can provide junior lawyers with opportunities to engage with more substantive aspects of legal work earlier in their careers. This potential shift towards earlier engagement with complex legal work could contribute to a more equitable environment for professional development within law firms.
7. **Learning from Past Cases:** Predictive coding can be leveraged to analyze outcomes in historical cases. By identifying patterns between specific document types, arguments used, and successful litigation strategies, legal teams can draw insights that inform decision-making in current cases. This historical perspective can potentially enhance strategic planning in complex litigation scenarios.
8. **Mitigating Human Bias?** One fascinating potential of predictive coding lies in its capacity to minimize human biases that might influence document review. While it’s crucial to ensure that the training data for these algorithms is diverse and representative to prevent the embedding of existing biases, the concept of AI-driven impartiality in a traditionally subjective process is worth exploring.
9. **Beyond Privilege Review:** The capabilities of predictive coding extend beyond privilege reviews. Its applications can be broadened to encompass tasks like contract analysis and compliance checks, showcasing the versatility of AI-driven tools for enhancing operational efficiency across different legal domains.
10. **Ethical Considerations in a Technological Age:** The adoption of predictive coding and similar AI technologies in legal contexts brings forth essential ethical considerations. Transparency, accountability, and the implications of relying on machine learning for significant legal decisions require careful thought. As law firms integrate these technologies, maintaining a focus on ethical alignment and proper oversight becomes crucial.
AI-Powered Document Analysis Revolutionizing Discovery in Insurance Litigation by 2024 - AI-powered redaction tools ensure compliance with data privacy regulations
AI-powered redaction tools are increasingly vital for legal professionals, especially when it comes to ensuring compliance with data protection regulations like GDPR and HIPAA. Traditional manual redaction methods, prone to human errors and time-consuming, are being replaced by AI-driven automation. This automation provides a quicker and more precise way to redact sensitive information, making it easier to handle the ever-increasing number of digital documents and minimizing legal risks associated with data breaches. By automating the removal of personally identifiable information (PII) in documents, AI allows legal professionals to concentrate on more critical legal matters while maintaining the highest standards of data privacy for their clients. However, as the reliance on AI grows, it's critical that the legal field carefully evaluates the potential for bias and other ethical considerations that are often embedded within AI algorithms.
AI-powered redaction tools are increasingly important in ensuring compliance with data privacy regulations like GDPR and HIPAA. They automate the process of identifying and removing sensitive information, effectively reducing the chance of human error that often comes with manual review. This automated approach not only expedites the compliance process but also contributes to more consistent application of redaction standards, which is a challenge for manual processes.
These tools leverage machine learning, which allows them to learn and improve over time. As they process more documents and receive feedback from human reviewers, their ability to accurately identify protected data becomes increasingly refined. This dynamic learning capability enables law firms to continuously enhance their compliance protocols.
Many eDiscovery platforms now integrate AI-powered redaction seamlessly, making the entire process more fluid. Redaction can now be applied as documents are reviewed within the platform, leading to a more cohesive workflow. This tight integration is important for maintaining data integrity throughout the review and analysis process.
The potential for AI to mitigate human bias is also intriguing. Cognitive overload and subconscious biases can lead to human reviewers missing or misinterpreting sensitive information. AI-driven redaction can, at least in part, address this problem, fostering a fairer and more objective discovery process.
There's also a clear benefit in terms of time and cost. Studies have shown that these tools can decrease the time spent on manual review by a significant margin, often by 70%. This translates into substantial savings in labor costs and allows law firms to allocate resources to more complex aspects of legal work.
Some sophisticated tools use predictive analytics to anticipate potential compliance problems before they become issues. By studying historical data patterns, they can flag documents that are likely to contain sensitive information. This forward-thinking approach minimizes the chances of inadvertently breaching privacy regulations.
The capacity of these tools to adapt to varying jurisdictions is another advantage. They can incorporate the data privacy rules of different locations into their algorithms, making them suitable for multinational law firms managing cases across regions with distinct compliance requirements.
These AI tools not only redact sensitive information but also automatically create and track documentation of the redaction actions. This thorough approach assists in maintaining compliance records, which is often vital for audits and legal proceedings.
Furthermore, the improved NLP capabilities in AI redaction tools allow them to understand the intricacies of legal language and context. This helps them go beyond simple keyword searches, identifying sensitive content that might otherwise be missed.
However, the growing use of AI in legal practices, including redaction, raises complex ethical questions. It's essential that law firms use these tools responsibly, prioritizing transparency and accountability to avoid inadvertently compromising client confidentiality or legal due process. While AI offers great efficiency, it’s imperative that its application is guided by a strong sense of ethical responsibility.
eDiscovery, legal research and legal memo creation - ready to be sent to your counterparty? Get it done in a heartbeat with AI. (Get started for free)
More Posts from legalpdf.io: