AI-Powered Analysis of Disguised Partnership Interest Sales New Machine Learning Tools Streamline Complex IRS Classifications
AI-Powered Analysis of Disguised Partnership Interest Sales New Machine Learning Tools Streamline Complex IRS Classifications - Machine Learning Models Map Complex IRS Section 707 Classifications For Law Firms
Artificial intelligence, specifically machine learning models, are fundamentally changing how law firms tackle the complex landscape of IRS Section 707, especially when dealing with disguised partnership interest sales. These sophisticated models offer the capability to pinpoint risks and uncover the most advantageous tax planning strategies, potentially illuminating hidden areas where clients' situations might be overlooked. The application of machine learning allows for the development of predictive tools which leverage IRS data to improve the precision of industry coding and anticipate outcomes with greater accuracy compared to traditional statistical methods. While the use of AI in legal practice is growing, the complexities of tax regulations may become more amenable to management, empowering lawyers to concentrate on more strategic aspects of their client's cases. Yet, despite the increasing implementation, the full potential of the vast quantities of data generated by these systems remains largely unexplored and underutilized.
1. AI algorithms are now adept at sifting through countless IRS Section 707 classifications, substantially cutting down the time lawyers dedicate to complex tax allocation dilemmas. These issues, traditionally requiring weeks or even months to settle, can now be addressed more efficiently.
2. Utilizing AI, law firms can analyze past litigation records to predict the possibility of IRS challenges to particular classifications. This predictive power is invaluable in formulating proactive risk-management approaches.
3. These sophisticated systems employ natural language processing to analyze a vast body of regulations. They can dissect subtle and intricate aspects of the law that might escape human eyes, enhancing legal understanding and preparedness.
4. AI streamlines the process of ensuring legal compliance with these nuanced tax laws. This frees up legal practitioners to focus on more strategic legal aspects, shifting from information retrieval to high-level strategy development.
5. Automating the classification procedure significantly reduces human errors. This is especially crucial in the context of partnership interest sales, where human mistakes have been a recurring factor leading to tax-related disputes.
6. Advanced machine learning applications, including unsupervised learning, allow models to constantly refine their classification accuracy. This means the algorithms continuously learn and adapt to new rulings and changes in tax law, thus becoming more accurate in their predictions.
7. Integrating AI into document creation enables law firms to craft highly detailed agreements that specifically address various Section 707 classifications. This ensures that potential tax liabilities are thoughtfully addressed from the outset of the transaction.
8. Applying AI in e-discovery processes allows law firms to dramatically filter through vast quantities of information and identify documents relevant to particular IRS classifications. This process significantly reduces time and cost during litigation by filtering out irrelevant information.
9. These AI systems, particularly those leveraging deep learning, provide insightful information about the classification process. This allows law firms to foresee potential IRS inquiries or audits based on past case resolutions and classification trends.
10. AI accelerates workflows within law firms, but it also enhances cooperation between tax specialists and legal teams. It achieves this by presenting a centralized platform for analyzing and interpreting intricate datasets, fostering collaboration and clarity.
AI-Powered Analysis of Disguised Partnership Interest Sales New Machine Learning Tools Streamline Complex IRS Classifications - AI Document Analysis Tools Transform Partnership Interest Sale Detection
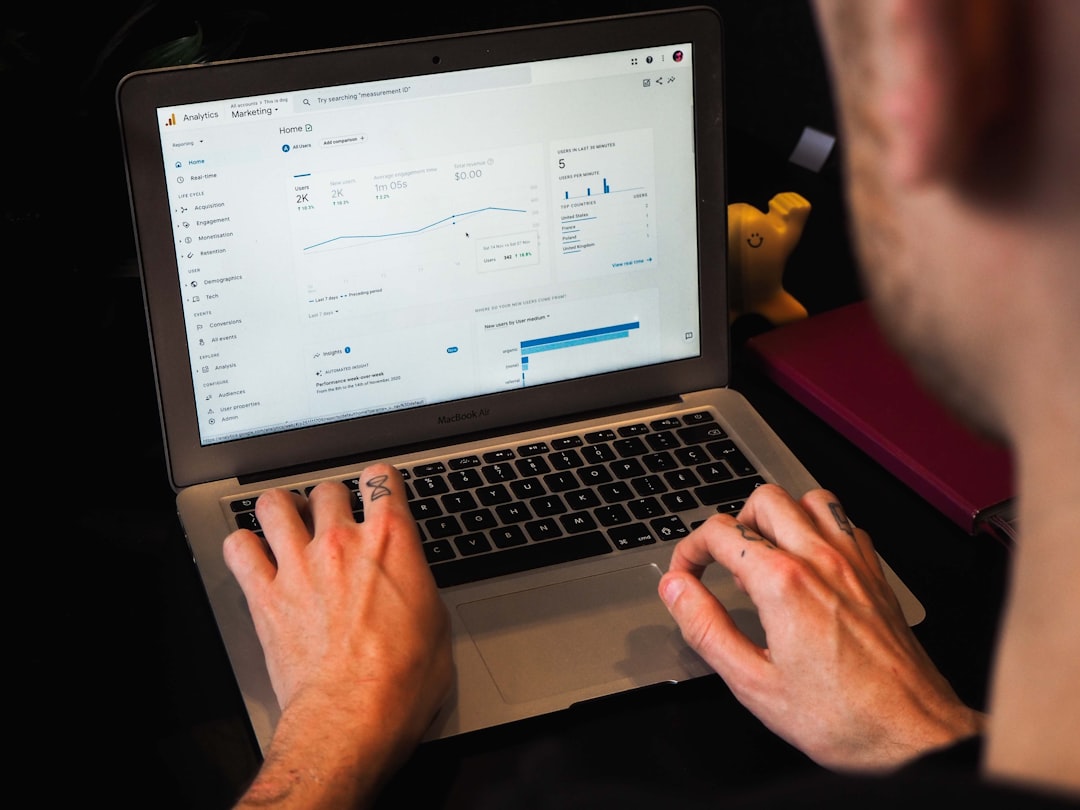
AI-driven document analysis tools are transforming how legal professionals identify partnership interest sales, especially within the intricate framework of IRS classifications. A significant portion of legal data remains in unstructured formats, highlighting the importance of AI tools in extracting insights from these documents. These tools, utilizing techniques like natural language processing, can dissect complex legal structures and regulations, allowing lawyers to streamline compliance and risk assessment processes. The result is a reduction in the time spent on routine tasks, enabling lawyers to dedicate more effort to strategic decision-making for their clients. While the increased use of AI in law is promising, it's crucial to ensure that these technological advancements are implemented responsibly, maintaining a balance between AI assistance and the essential role of human judgment and legal expertise in complex cases. There's a danger of over-reliance, potentially overshadowing the critical thinking needed in legal analysis and interpretation.
AI-driven document analysis tools are reshaping how legal professionals approach partnership interest sales, specifically regarding IRS classifications. The ability to process vast amounts of unstructured data – a significant portion of the information available in this field – is crucial for effective analysis. Platforms like Azure's AI Document Intelligence are at the forefront, employing advanced machine learning to extract key information from various document formats, encompassing text, tables, and intricate structures. Integrating this with models like Azure OpenAI GPT-4 Turbo further allows for sophisticated insights and potentially, more nuanced interpretations of complex legal language.
While these advancements are exciting, it's important to acknowledge that the full potential of AI's role in legal discovery and analysis isn't fully realized. These technologies still require further development and refinement for optimal effectiveness. Other platforms like Petal, focused on context-aware generative AI, or Jedaa, which translates complex documents into easily understood visualizations, offer alternative approaches to document exploration and collaboration.
These evolving tools aren't just applicable to tax law, they are also proving valuable across legal practice domains. Thomson Reuters’ Document Intelligence, for example, is focused on building AI-powered models to identify crucial facts in legal documents. Similarly, DealRoom uses AI-powered tools to boost the M&A process, specifically streamlining due diligence. The broader trend towards incorporating generative AI functionalities into these platforms is notable, enabling features like summarization, translation, and even automatic drafting of legal documents.
However, there are also potential issues to consider. Despite improved accuracy, these AI models can still be susceptible to errors, particularly when presented with ambiguous or poorly structured legal documents. The risk of bias in the training data could also inadvertently lead to skewed outputs. These limitations underline the necessity for human oversight and ongoing evaluation of AI-generated insights.
Furthermore, the rapid evolution of tax law requires constant updates to the AI models. Continuous training and refinement will be vital to maintaining accuracy and ensuring the models adapt to legislative changes and legal precedent. The current state-of-the-art might still require human experts to validate interpretations, especially in cases with significant financial implications. Despite these nuances, the integration of AI into legal practice is undeniable. It is accelerating research, enhancing legal strategies, and transforming workflows in areas like e-discovery. These applications are leading to new avenues for collaboration within law firms and a greater capacity to efficiently navigate the complex realm of tax regulations.
AI-Powered Analysis of Disguised Partnership Interest Sales New Machine Learning Tools Streamline Complex IRS Classifications - Large Language Models Process Multi Year Financial Data To Track Disguised Sales
Large language models are increasingly being used to analyze complex financial data, including multi-year records, to identify instances of disguised sales. These models, particularly advanced ones like LLMs, can sift through extensive datasets and identify potential irregularities, making it easier for legal and financial professionals to detect disguised sales related to partnership interests. The ability of these models to understand context and process unstructured data offers a substantial advantage over traditional methods, providing a more accurate and faster analysis of financial patterns.
While the potential of this technology is evident, it's crucial to acknowledge that these models, even those using advanced techniques like deep learning, are not without limitations. There's a risk of over-dependence on AI-powered insights, potentially neglecting the importance of legal expertise and critical thinking needed for complex financial cases. Also, there's a need for consistent updates to these models, as financial regulations and the intricacies of tax law are constantly evolving.
Despite these caveats, the ability of LLMs to enhance the efficiency of analyzing financial information and uncovering hidden transactions has substantial benefits. This includes speeding up discovery processes, allowing lawyers to dedicate more time to high-level strategic considerations, and improving the accuracy of compliance and risk assessments in the specific context of partnership interest sales. However, the balance between leveraging the power of AI and maintaining human oversight will be essential to ensure reliable outcomes and responsible implementation within the legal landscape. The future of financial investigations and legal strategy development may be increasingly shaped by this technology, but maintaining human oversight and critical thinking will remain essential.
Large language models, similar to how a human financial expert might, can analyze extensive financial data spanning multiple years to detect subtle patterns suggesting disguised sales. This capability enhances the detection of potential tax compliance issues that might otherwise be missed by traditional methods.
AI can integrate various data sources, such as financial records and past tax filings, enabling the cross-referencing of information and identification of inconsistencies indicative of disguised sales. This proactive approach helps law firms preemptively manage potential tax risks.
The precision of AI in classifying transactions based on IRS guidelines is demonstrably refined through feedback loops. The models learn from past legal decisions and incorporate that knowledge to enhance future predictive accuracy, making them more robust over time.
AI tools can recognize intricate connections between various financial entries that might not be apparent to humans, potentially pinpointing areas where clients might be subject to IRS scrutiny. This type of foresight is crucial for informed risk management strategies within law firms.
AI-powered document analysis tools can expedite the review of a large volume of contracts and uncover potentially hidden clauses or partnership interests that might indicate disguised sales. This process empowers legal teams to develop more precise compliance strategies.
The successful deployment of machine learning in financial law is contributing to significant reductions in litigation expenses. AI’s capacity to swiftly pinpoint critical evidence allows legal counsel to provide more informed and timely advice, streamlining the overall legal process.
Sentiment analysis, using AI on communications related to partnership sales, can unveil underlying intentions and perceptions, giving a richer understanding of the client's motives. This nuanced understanding can greatly influence legal strategies and positioning.
Law firms using AI for electronic discovery have reported substantial time reductions – up to 70% – allowing legal teams to shift their focus to high-impact strategic considerations rather than the tedious task of sifting through data to identify disguised sales.
Machine learning algorithms are constantly being improved. As more data related to IRS audits and classifications becomes available, these algorithms adapt and become increasingly sophisticated in their predictive abilities.
The use of AI in legal analysis is raising ethical questions. Reliant on software to assess financial compliance introduces concerns about responsibility, especially in situations where models misinterpret legal language or context. These are complex areas that need further exploration and careful consideration as AI applications in legal practice mature.
AI-Powered Analysis of Disguised Partnership Interest Sales New Machine Learning Tools Streamline Complex IRS Classifications - Neural Networks Calculate Real Time Tax Implications Across Transaction Types
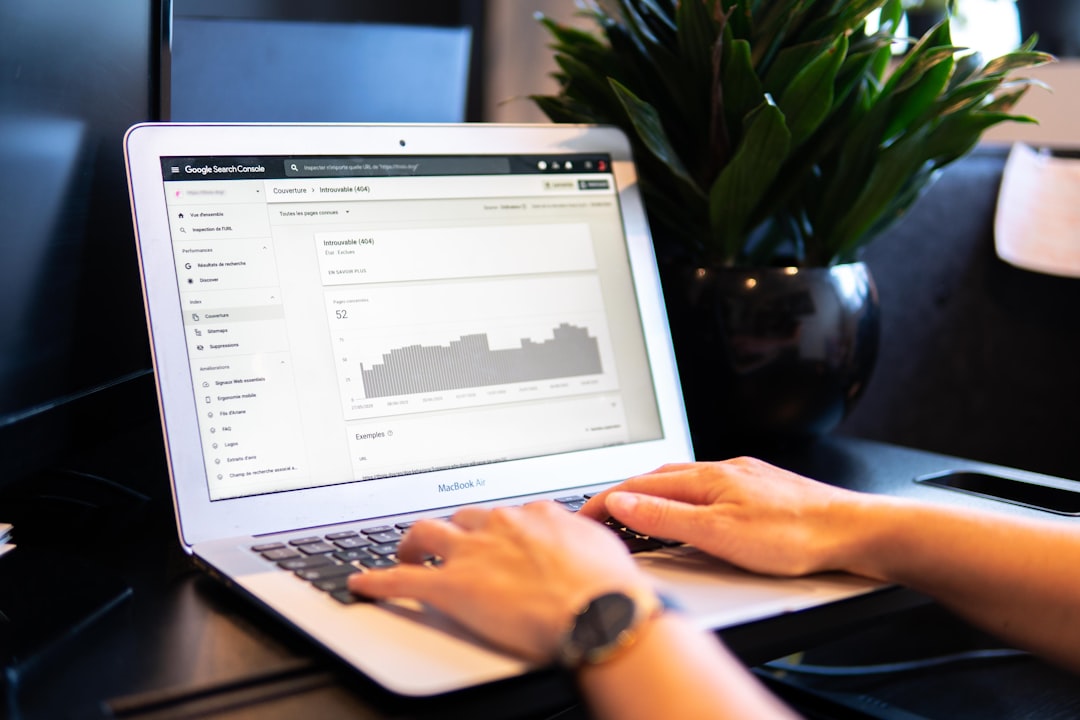
Neural networks are increasingly capable of calculating tax implications in real-time across a range of transactions. This development has the potential to significantly improve the speed and accuracy of tax analysis within the legal field. These AI systems are able to analyze intricate financial data, making it easier to identify potentially problematic situations like disguised partnership interest sales. Furthermore, they are playing a larger role in the streamlining of IRS classifications, allowing lawyers and tax professionals to focus on higher-level strategic considerations, rather than tedious manual tasks. The evolution of these tools is undeniably reshaping legal practice. However, it's crucial that the implementation of AI in this context is thoughtfully managed. There is a risk of over-reliance on AI-generated insights, which could potentially overshadow the necessity of human legal expertise and critical analysis in complex tax scenarios. As AI becomes more integrated into the legal process, ethical considerations regarding AI's role in decision-making will need ongoing evaluation. Overall, the advancement of AI tools in areas like tax law represents a significant shift within the field, but it's imperative to ensure that the technology's application is balanced with a continued focus on legal expertise and ethical practice.
Neural networks are now being used to analyze vast amounts of financial data in real time, which speeds up the identification of disguised sales that previously required weeks or months of manual review. This allows for faster compliance checks and potentially reduces the time to identify issues.
AI systems can now link together many different data sources, such as financial statements, tax filings, and market data. This ability to cross-reference data enables detection of inconsistencies that may signal disguised partnership violations, acting like a digital auditor uncovering hidden aspects of transactions.
Sophisticated algorithms can learn from the results of IRS audits and refine their classification abilities as new information comes in. This real-time adaptability means that these models can not only assess current tax situations, but they can also adapt to predict future risks based on the patterns seen in historical audit results.
Some AI tools are capable of spotting language patterns in documents related to partnership transactions. This reveals subtle intentions that might otherwise go unnoticed by human analysts, and these intentions could lead to later tax problems.
By examining the sentiment expressed in communications related to partnership interest sales, law firms can get a better sense of the motivations behind transactions. This gives them the opportunity to develop more specific legal strategies that can reduce risks associated with potential issues.
Machine learning models that analyze tax regulations can detect subtle changes in language or numbers that could have a significant impact on legal standing. This ability to monitor the regulatory landscape helps firms stay ahead of compliance deadlines and potential changes in the tax laws.
The ability of AI to gather years of financial data into a unified view allows for faster and more accurate assessments of possible disguised sales. This process leverages historical trends and helps to minimize errors that can happen when humans are trying to interpret complex financial data.
The growing role of AI in legal research allows lawyers to discover relevant precedents and regulations in a fraction of the time it used to take. Searching through large collections of documents and databases is something that AI can do much quicker than a human.
As AI tools continue to improve, they will be able to make predictions about a client's financial activities. This predictive ability helps firms proactively give advice on how to structure deals in a way that avoids possible IRS scrutiny or problems related to disguised sales.
While AI offers many benefits to the legal profession, there are concerns about bias in the data used to train the AI models. Inaccuracies in the training datasets could lead to mistakes in how financial transactions are classified and assessed for compliance. This is a complex issue that is still being studied, and researchers and practitioners need to be careful and thoughtful about the potential issues related to bias.
AI-Powered Analysis of Disguised Partnership Interest Sales New Machine Learning Tools Streamline Complex IRS Classifications - Automated Systems Flag High Risk Partnership Restructuring Patterns
Automated systems are becoming adept at identifying potentially risky patterns in partnership restructurings, especially when dealing with intricate IRS tax classifications. These systems, driven by AI and machine learning, can sift through large amounts of data and detect hidden financial connections that could pose problems. This early warning capability aids compliance efforts and streamlines operations, potentially helping companies avoid tax-related penalties. Yet, the growing use of AI in this area raises questions about how well current legal and regulatory structures can handle the complexity AI introduces. There is a need for thoughtful oversight and integration of these tools to ensure proper use within legal practices. The success of these AI-driven solutions rests on a careful balance between automated analysis and the expertise of human legal professionals. It is crucial that legal expertise continues to guide important decisions within the legal arena. Striking this balance is critical to the future development and use of AI in legal matters related to partnership restructuring.
AI systems are becoming increasingly sophisticated in their ability to dissect intricate partnership structures and flag potentially problematic restructuring patterns related to tax compliance. These systems can analyze the interconnectedness of different tax classifications, providing a more holistic view of how changes in one area can ripple through others within the context of a partnership interest sale. For instance, AI can visualize how altering a specific tax classification might lead to unforeseen consequences elsewhere in the transaction, potentially influencing the overall tax implications. This level of understanding surpasses traditional methods that often focus on individual elements in isolation.
Furthermore, AI's capacity to monitor legal developments in real-time is transforming how law firms navigate the complex landscape of tax regulations. As new laws and IRS rulings are released, AI algorithms can immediately analyze their impact on existing strategies and compliance requirements. This dynamic responsiveness, which has been absent from traditional legal practices, offers an edge in proactively adjusting strategies as needed. It's important to recognize, however, that relying solely on these systems without a human component could be a blind spot. Tax law is nuanced and interpreting specific language and its intended meaning is crucial to understanding potential consequences.
This increased efficiency can be observed in the marked reduction of time spent on routine tasks. Law firms are reporting a reduction in the time devoted to basic compliance checks by as much as 70%. This allows lawyers to focus on tasks that require the complexities of legal expertise, such as strategic planning and high-level risk assessments. These benefits can be particularly pronounced in the context of partnership interest sales, where a misclassification can trigger lengthy and costly legal battles. However, the reliance on automated systems does raise a concern about the potential erosion of skills that were traditionally central to legal practice. It's something that needs to be monitored and addressed.
One of the most promising applications of AI in this area is the development of predictive models to identify high-risk transactions before they occur. AI tools can analyze the specifics of a proposed transaction against existing tax laws, patterns from past legal disputes, and IRS classifications, pinpointing potential points of contention. This early intervention can prevent costly complications down the line. However, as in the development of other automated systems, there's always the danger of blind spots in the model's awareness of particular subtleties that the human legal mind might notice. This is not simply an AI issue, but a problem that extends to all areas of automated legal practice.
Leveraging the power of neural networks, AI is capable of creating simulations of different financial scenarios. These simulations can anticipate the potential tax implications associated with a proposed transaction, allowing firms to understand and model the possible outcomes. The ability to test various structures in a virtual space empowers attorneys to craft contracts and navigate complex financial dealings in a more informed manner. This is where AI has shown the most promising impact on legal practice. However, there is the concern about bias, whether explicit or inherent to these models. This necessitates ongoing human intervention to verify or adjust the outputs to ensure a fair and equitable outcome, not just one that looks to prioritize a particular desired outcome.
In addition, risk assessment algorithms have evolved to incorporate behavioral data. By analyzing a client's past compliance history, AI models can better assess future risks and tailor compliance strategies accordingly. This customized approach can aid in mitigating the likelihood of future IRS scrutiny and potential disputes. However, this type of analysis can easily become problematic if the original data is skewed, biased, or misrepresented. This can lead to outcomes that might not be fair and might be biased in favor of particular outcomes.
The intricate nature of language in legal documents can often lead to misunderstandings or disputes. AI tools with their capacity to delve into the subtleties of legal text are mitigating this risk by providing more precise interpretations of contracts and regulations. This reduces the potential for disagreements stemming from unclear clauses and promotes a more precise alignment between contractual obligations and legal interpretations. The increasing dependence on these tools will require ongoing vigilance in auditing and refining the models to ensure that the legal and contextual meanings are understood and interpreted in a consistent and reliable way.
AI is significantly impacting the speed and efficiency of due diligence processes in M&A transactions involving partnerships. By automatically processing vast amounts of contractual and financial data, AI platforms can rapidly pinpoint potential red flags, often much earlier than would be possible with traditional methods. This empowers law firms to delve into areas of risk more quickly and enables them to provide more informed advice to their clients. The application of these techniques extends beyond mere detection of risk factors; in cases where fraud is suspected or is uncovered, this is often the very first step in the building a case.
AI-driven insights are also transforming courtroom strategies. Attorneys are increasingly leveraging the ability of AI tools to analyze data from historical cases, which allows them to craft compelling arguments supported by data-driven trends. This data-centric approach strengthens the presentation of legal arguments and positions lawyers to be better prepared in the courtroom. However, it's crucial to note that presenting only what is mathematically probable may not be enough to determine or sway the outcome of a trial. The human legal mind and reasoning will still be needed to contextualize facts and to craft narratives that are persuasive to the trier of fact.
Despite the numerous benefits offered by AI, it's crucial to recognize the limitations of these systems. Even the most sophisticated AI models can fall prey to biases present in the data used for their training. This issue is of critical importance in a legal context where accuracy and fairness are paramount. As such, ongoing human oversight is essential to ensure that AI-driven analysis remains objective and does not perpetuate biases that could lead to unfair or erroneous outcomes. As the role of AI continues to expand within the legal field, researchers and practitioners must remain vigilant to recognize these limitations and strive to create systems that produce just and equitable outcomes.
AI-Powered Analysis of Disguised Partnership Interest Sales New Machine Learning Tools Streamline Complex IRS Classifications - AI Enhanced Regulatory Compliance Tools Guide Partnership Classification
AI is significantly impacting how partnership structures and related transactions are classified under IRS regulations, particularly in the realm of compliance. These AI-powered tools, driven by machine learning, offer an unprecedented ability to quickly spot changes in regulations, improving compliance procedures and reducing errors that often stem from manually reviewing complex documents. Through the use of these tools, automated systems can effectively sift through substantial amounts of data to identify potentially risky restructuring patterns and hidden financial relationships that could indicate disguised sales. This automation allows legal professionals to shift their focus from mundane compliance tasks to more complex strategic decision-making for their clients.
Despite the clear advantages of utilizing AI for compliance and classification in this area, the increased reliance on such technology brings with it certain concerns. One significant risk is the potential for AI-powered systems to overlook the nuanced legal interpretations that are critical for handling complex partnership structures. Additionally, it's imperative that these systems are implemented in a way that ensures human legal professionals remain at the forefront of the decision-making process. This is needed to guarantee the accuracy and fairness of any legal decisions based on the analyses provided by these AI systems.
As these AI-driven tools continue to develop, it's crucial that their integration into the legal profession is approached with care. The legal field must carefully consider how best to leverage these technologies within existing legal frameworks to maintain the integrity and effectiveness of the legal system. Achieving a successful outcome will require balancing the improvements in efficiency offered by AI with the ongoing importance of legal expertise and human judgment.
AI-driven tools are increasingly being integrated into the legal landscape, particularly in areas like e-discovery and document analysis, with significant implications for legal strategy and compliance. Current AI models can now efficiently analyze and cross-reference data from multiple sources in real-time, offering a dynamic view of financial data that can reveal anomalies suggestive of disguised partnership sales, for instance. This real-time capability is changing how law firms respond to evolving tax regulations, requiring more adaptive approaches than traditional methods allowed.
Furthermore, the continuous learning capabilities of machine learning models are a key advantage. They can adjust their ability to classify and predict potential tax issues based on historical IRS audit data. This is superior to the older, static learning approaches and helps firms remain up-to-date on compliance requirements. AI significantly enhances the efficiency of e-discovery processes, potentially reducing timelines by as much as 70%. This translates to cost savings and allows lawyers to prioritize strategic work instead of tedious document review.
These AI systems can also analyze the complex interrelationships within partnership restructurings, providing insights into potential tax compliance risks that might otherwise be missed. Human analysts might not see these connections, so the ability of AI to discern such complex patterns is advantageous in avoiding costly missteps. Another intriguing use of AI is in risk assessment, where models can incorporate an entity's history of compliance, leading to more tailored, proactive risk management strategies. This personalized approach is far more effective than reacting to potential problems after they emerge.
These tools also help to form predictive models for high-risk transactions, offering an opportunity to address potential tax complications proactively. In addition, sentiment analysis applied to legal documents and communications can shed light on the intent behind transactions, which is valuable when negotiating or disputing these deals. AI algorithms are also increasingly precise in their interpretation of complex legal texts, reducing ambiguity and the likelihood of disputes based on poorly worded contractual clauses.
However, it's crucial to acknowledge the limitations of AI, particularly the possibility of bias in its training data. This is a significant consideration, particularly in the legal context where accuracy and fairness are essential. Careful ongoing assessment and corrective action will be necessary to ensure that AI's application remains equitable and promotes fair outcomes.
Finally, the courtroom is also seeing the influence of AI-powered tools. Lawyers can now build more robust legal arguments using data-driven insights gleaned from analyzing historical cases. This trend underscores the rising prominence of AI in legal strategy, but it’s crucial to acknowledge that data analysis, while important, still needs the interpretive and narrative-building capacities of the human mind to persuade a jury or judge. It is imperative that human judgment and legal expertise continue to guide decision-making as AI tools become increasingly sophisticated. The future of legal practice will likely involve a complex interplay of advanced technologies and human expertise, requiring careful thoughtfulness and responsible development to ensure that AI applications maintain the highest ethical standards.
More Posts from legalpdf.io: