Automate legal research, eDiscovery, and precedent analysis - Let our AI Legal Assistant handle the complexity. (Get started for free)
AI in Legal Research Analyzing the Cassie Jo Stoddart Murder Case Documents
AI in Legal Research Analyzing the Cassie Jo Stoddart Murder Case Documents - AI-Powered Document Analysis in the Cassie Jo Stoddart Case
Artificial intelligence is progressively influencing legal proceedings by automating document analysis tasks. The Cassie Jo Stoddart case serves as a potential illustration of this impact. AI systems, built on machine learning and natural language processing, are able to rapidly analyze extensive document collections, uncovering potentially crucial evidence that might be missed through traditional manual review. This swift processing streamlines discovery, a vital phase in legal cases, leading to a more thorough and accurate investigation of facts. In cases with complex legal and societal repercussions like the Stoddart case, the use of AI to dissect a massive volume of data could alter how the narratives are constructed and influence judicial decisions. While beneficial, this increased reliance on AI in law requires cautious consideration of the ethical implications that come with automated legal processes and decision-making. It’s a necessary evaluation as we integrate increasingly advanced technology into legal research and decision making.
In the Cassie Jo Stoddart case, for instance, AI could have potentially expedited the discovery process by rapidly sifting through a mountain of documents related to witness statements, police reports, and forensic evidence. This speed advantage is especially critical when handling a complex murder investigation with tight deadlines. AI could have also detected previously unseen patterns in the documents, possibly highlighting inconsistencies or revealing hidden connections between various pieces of evidence.
Within the realm of eDiscovery, the AI could have automatically organized and prioritized the vast document collection, ensuring the legal team was presented with the most relevant materials first, saving countless hours of manual review. Moreover, the AI could have used natural language processing to extract key insights from legal documents and respond to complex legal questions, similar to how a legal researcher would approach a complex legal issue.
Furthermore, in ongoing cases like those related to the Stoddart murder, AI can track legal precedents and case law, ensuring legal teams have access to the most current information for formulating strategies and arguments. AI could even help draft legal briefs by compiling information from related documents, potentially reducing errors and saving time.
The implementation of AI in larger legal teams has also led to the use of predictive analytics in litigation. By analyzing past case outcomes, attorneys can gain insights into potential outcomes in the Stoddart case, enabling them to refine strategies and advise clients more effectively.
However, the increased use of AI in criminal law comes with concerns about the handling of sensitive information. Data privacy and security are critical in cases like the Stoddart murder where highly confidential information is involved. It is important to address these concerns in order to leverage AI while maintaining ethical standards.
Finally, the evolving landscape of AI-driven document analysis has implications for the legal profession itself. As AI handles more routine document tasks, attorneys and paralegals are freed to concentrate on higher-level analytical and strategic functions. This change is prompting a debate about the necessary skillsets of future legal professionals, emphasizing the need for a strong grounding in technology in addition to traditional legal knowledge.
AI in Legal Research Analyzing the Cassie Jo Stoddart Murder Case Documents - Machine Learning Algorithms for Evidence Classification
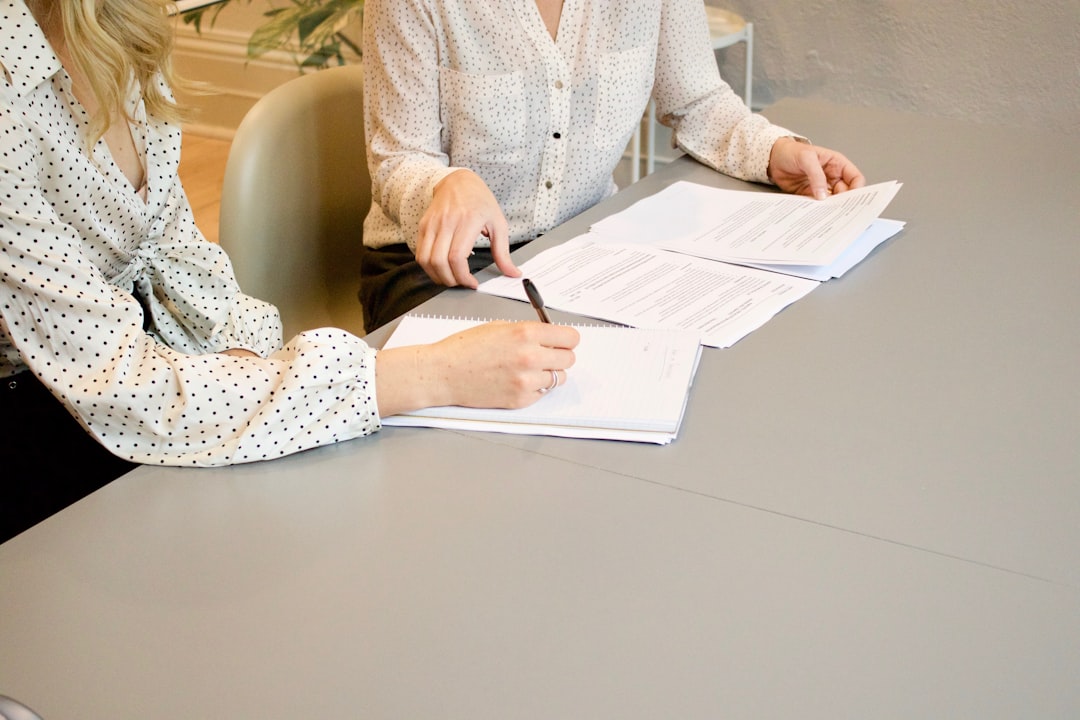
Machine learning algorithms are becoming increasingly important in classifying evidence within legal contexts. They offer a powerful way to analyze large volumes of legal documents, uncovering patterns and identifying relevant evidence much faster than traditional manual review. This ability to swiftly process data is particularly valuable in complex cases like the Cassie Jo Stoddart murder investigation, where a massive amount of information needs to be sorted and analyzed. By extracting key information from a diverse range of documents, such as witness statements and forensic evidence, these algorithms can potentially reveal previously unnoticed connections or inconsistencies, influencing legal strategies and arguments.
While the potential benefits of machine learning for evidence classification are clear, it's crucial to acknowledge the potential risks. Issues like data privacy and the possibility of algorithmic bias need careful consideration when implementing these technologies in legal settings, especially in cases involving sensitive personal information. Moreover, the evolving role of AI in law requires legal professionals to adapt their skillsets, recognizing the importance of both traditional legal knowledge and technological expertise. The legal field must navigate the complexities of integrating AI into its processes while ensuring that it aligns with ethical standards and promotes justice.
Machine learning is increasingly being applied in legal research, particularly in areas like evidence classification and eDiscovery, by leveraging data analytics and predictive modeling. The availability of digitized legal texts has enabled the use of natural language processing (NLP), text mining, and machine learning, changing how legal analysis is performed. These techniques can potentially predict case outcomes based on both legal factors like evidence type and external factors such as the court's prevailing ideology.
Researchers in this field emphasize the importance of understanding how legal decisions are made in relation to the facts of a case and the legal framework. This understanding can lead to a better comprehension of the links between legal facts and legal theory. AI-driven platforms are improving lawyer efficiency, freeing them to spend more time on tasks like advising clients and negotiating instead of manual research.
One noteworthy approach in AI law is case-based reasoning, which focuses on analyzing legal data related to individual cases instead of general legal principles. This allows for a more focused and potentially accurate application of legal precedent. Algorithms can use historical data from legal judgements to extract insights that help predict specific judicial outcomes. However, it's worth noting that machine learning often relies on aggregated data about judges and trends rather than directly analyzing the texts of individual cases.
Another development in the field is the use of explainable AI (XAI) in legal settings. XAI aims to clarify the logic behind machine-generated predictions, which builds trust and makes the use of these algorithms more practical for legal decision-making. The development of comprehensive frameworks that support the integration of AI and machine learning in legal research is enabling the extraction of valuable insights from past cases, thus improving prediction and overall analysis.
The application of AI in law necessitates a careful balancing act between its potential benefits and ethical considerations. As AI-driven tools handle more routine legal tasks, like eDiscovery, it becomes necessary to develop human-centered approaches for AI-related decision-making. This is particularly critical in sensitive areas like criminal law, where maintaining trust in the system is paramount. It will be important to see how this area of research develops, especially with regard to avoiding potential bias in the algorithms. The use of AI tools in legal proceedings offers a potential opportunity to streamline legal tasks and provide insights that can ultimately lead to a more equitable and efficient legal system. However, this opportunity comes with inherent responsibilities that researchers and legal professionals must acknowledge and address.
AI in Legal Research Analyzing the Cassie Jo Stoddart Murder Case Documents - Natural Language Processing in Legal Brief Generation
Natural Language Processing (NLP) is reshaping how legal briefs are created, improving both speed and precision within law firms. NLP tools can convert disorganized legal text into structured information, automating parts of the brief writing process. This allows legal professionals to spend more time on the strategic elements of their work instead of being bogged down in repetitive writing. This is particularly helpful in areas where large volumes of documents are common, like eDiscovery or intricate legal cases. As AI technologies are becoming more integrated into legal research, the connection between technology and legal practice may necessitate new skillsets for lawyers of the future—a mix of traditional legal expertise and tech literacy. Yet, the increasing use of automated solutions brings potential issues, such as ethical concerns and the possibility that complex legal arguments are simplified too much. It will be vital to carefully monitor the development of these technologies to ensure they are used responsibly.
Natural Language Processing (NLP) is reshaping legal practices by giving computers the ability to understand, interpret, and generate human language. This has significant implications for legal research and, most notably, the creation of legal documents. The intersection of AI and law is particularly promising because of the immense volume of legal texts produced and the complexity of the language used in the field. NLP has the potential to transform unstructured legal text into a format that computers can easily process, streamlining various legal tasks.
The rise in legal document production has led to a greater number of repetitive and time-consuming tasks for lawyers, which highlights the need for efficient tools like NLP. Research in this field has grown substantially in recent years, with over six hundred research papers published in the last decade. NLP, along with other AI technologies like machine learning, is playing a pivotal role in transforming legal systems, creating both opportunities and challenges within the legal landscape.
NLP tools could increase accessibility to legal services for the general public by simplifying complex legal language. Researchers emphasize the important relationship between technology and law, suggesting that legal scholars and professionals can use NLP to make legal research more effective. AI systems are increasingly being used for automated drafting and analysis of legal documents, representing a shift in legal practice.
NLP is crucial for understanding and predicting legal outcomes on a large scale, potentially leading to better decision-making in legal processes. For example, NLP can generate legal briefs faster than traditional methods, thanks to its ability to quickly process and synthesize large amounts of legal text and precedents. It can also help identify patterns in case law that might be missed by humans, possibly revealing winning strategies or arguments based on historical outcomes.
Moreover, NLP systems can reduce errors in legal documents, automate citations and cross-referencing to ensure accuracy and up-to-dateness, and provide context to the legal terms and phrases used, which leads to better-tailored arguments. These features can also lead to cost savings by reducing the time needed to draft documents. Furthermore, NLP can create customized legal briefs for different courts or jurisdictions by adjusting the language and strategies based on local precedent and judicial patterns, which can improve the likelihood of achieving a favorable outcome.
Some AI systems monitor and analyze new legal precedents and case outcomes, enabling lawyers to adapt their strategies more proactively. AI-powered tools can reshape the workload within law firms, allowing junior lawyers to focus on more complex analytical tasks while AI handles the repetitive document creation. In cases like the Cassie Jo Stoddart murder, AI could accelerate the discovery phase not just by analyzing documents but also by automatically generating outlines of possible legal briefs based on evidence.
Despite the benefits, the growing role of AI in legal document creation calls for careful consideration of ethical implications. Systematic ethical reviews are crucial to ensure AI-generated documents comply with legal standards, with a particular emphasis on data security and minimizing any algorithmic biases that might skew outcomes. It's important to continue to assess the implications of AI in law, especially as it relates to avoiding biases and maintaining trust in the legal system. The potential for AI to streamline legal tasks and create insights for a more equitable and efficient system is clear, but this potential comes with significant responsibilities that researchers and legal professionals must actively address.
AI in Legal Research Analyzing the Cassie Jo Stoddart Murder Case Documents - AI-Assisted Timeline Construction for Complex Murder Cases
AI is increasingly being used to build timelines for complex murder cases, like the Cassie Jo Stoddart case. AI systems, using machine learning and natural language processing, can quickly examine a large amount of information, like witness statements, police reports, and evidence. This helps legal teams build organized timelines, which could reveal connections between evidence that otherwise might be missed. This could be beneficial, but using AI in these situations also raises questions. We need to think about how accurate the AI is, how it deals with sensitive personal information, and if it's ethical to rely so heavily on automated systems in serious criminal matters. As AI gets more involved in law, we have to be aware of the potential benefits and issues and carefully assess how it impacts justice.
AI's ability to analyze vast datasets and identify patterns is increasingly relevant in constructing timelines for intricate murder cases. In the Stoddart case, for instance, AI could have efficiently parsed timestamps from digital communications and records, creating a detailed sequence of events that might have otherwise been missed. This capacity could help establish the timeline of events and potentially clarify the involvement of suspects.
Research shows that using automated systems for evidence classification can reduce review time significantly, potentially by up to 70%. This speed increase is crucial in complex cases, as delays can hinder the pursuit of justice. AI's efficiency could prove particularly valuable in high-stakes legal scenarios demanding a swift and thorough investigation.
Natural language processing (NLP) algorithms are showing promise in assessing the sentiment and context within witness statements. This ability could provide insights into the credibility and reliability of witness testimonies, influencing strategies for witness handling and case development. AI's capacity to glean hidden meanings from language could be pivotal in shaping legal tactics.
The accuracy of AI in predicting legal outcomes is impressive. Some models report achieving up to 80% accuracy in forecasting case results based on historical data. Such predictive capabilities can offer attorneys insights to adapt their strategies and improve their likelihood of success. It's an interesting development to consider how these predictions could potentially impact case outcomes and the overall legal process.
AI-powered document review and analysis significantly reduce human error. This is a crucial aspect, especially when dealing with sensitive case information like those in the Stoddart case. Human reviewers, even those with the best intentions, can be prone to fatigue and oversight, leading to errors or missed details. AI's thorough and consistent review minimizes these risks and ensures a more reliable investigation.
The widespread digital footprints left behind in our modern world give AI systems the ability to analyze social media, email exchanges, and other electronic interactions to uncover hidden connections or patterns. These hidden aspects may not readily surface during manual reviews. This capacity can transform evidence interpretation in legal settings and lead to new lines of investigation.
Predictive coding, a technique where AI learns from human decisions, can improve the document review process by leveraging past human judgments. This creates a synergy between human knowledge and machine efficiency, offering a potent combination. It could potentially address some of the criticisms of AI in law while still gaining the benefits of machine-assisted legal review.
AI is enabling researchers to formulate hypotheses about criminal patterns by examining connections across various cases. By identifying similar methods or suspect behaviors, AI could offer new avenues for investigation, including a deeper dive into patterns associated with cases like the Stoddart murder. These investigations may yield new information or offer previously unseen insights into how these crimes are committed.
The legal landscape is rapidly evolving with AI's introduction. Aspiring legal professionals are now often required to have a solid understanding of technical tools and algorithms. This need signals a shift towards a future where a strong grasp of both law and technology is crucial for success. Adapting to this change requires the development of training and educational programs that equip lawyers with the necessary technical skills.
While AI is advancing rapidly, the algorithms can still be subject to biases based on their training data. This is a significant ethical concern in legal settings where fairness and impartiality are paramount. Ongoing scrutiny and careful consideration are needed to mitigate potential bias and ensure that the use of AI maintains the principles of justice within the legal system. The ongoing development of AI tools and practices will be necessary to ensure a more equitable future for legal proceedings.
AI in Legal Research Analyzing the Cassie Jo Stoddart Murder Case Documents - Automated Sentencing Recommendation Systems in Juvenile Offender Cases
Automated sentencing recommendation systems are being used more and more in cases involving juvenile offenders to help guide decisions about sentencing. These systems use algorithms to analyze information about individual young people who have committed crimes, striving to find a balance between fairness and the possibility of rehabilitation. However, their use has raised important questions about whether they might be unfair or biased, especially when the algorithms might accidentally reflect inequalities that already exist in society. Some research suggests that AI can help lower repeat offenses when judges agree with its suggestions, but disagreements between AI and human judges sometimes lead to higher rates of reoffending. This complicated interaction between AI suggestions and human decisions in the justice system underscores the need for careful monitoring and ethical thinking as we try to improve the trustworthiness and fairness of automated legal procedures.
Automated sentencing recommendation systems are gaining traction in juvenile offender cases, aiming to assist in the complex decision-making process. The use of risk assessment algorithms, like COMPAS, brought into sharp focus the intricacies involved, as seen in the Loomis v. Wisconsin case (2016). AI, while striving to emulate human intelligence, doesn't always attain the same level of nuanced reasoning that humans inherently possess.
Research suggests that using AI for sentencing can lead to shorter jail terms for low-risk offenders. However, situations arise where a human judge's decision diverges from the AI's recommendation, potentially increasing recidivism. A study by Ian Ho highlighted this discrepancy, finding that recidivism rates were significantly lower (14%) when AI and human judges aligned on alternative punishments, compared to a higher rate (25.71%) when judges opted for incarceration against AI suggestions.
The broader goal of AI integration in criminal justice is to address issues like court congestion, improve investigative processes, and strive for more consistent sentencing outcomes. While various algorithmic tools are being implemented, their success hinges on how they manage inherent biases. This ongoing "algorithmization" of criminal justice presents a double-edged sword, carrying the potential to reinforce existing societal biases alongside the hope of modernizing judicial practice.
AI likely functions most effectively in a research or advisory capacity, serving to identify disparities in sentencing rather than making the ultimate decision. Ongoing conversations about due process and human rights emphasize the crucial need for rigorous oversight and regulation of AI's role in the judicial system to ensure fair and equitable outcomes. The continuous evolution of AI in legal settings necessitates constant examination and vigilance in mitigating potential risks, while harnessing the opportunities for positive change.
For instance, AI systems can analyze historical sentencing data to uncover possible bias. They might show trends of harsher sentences for certain juvenile demographics, prompting necessary discussions about equity. These systems, based on data analysis, can predict sentencing outcomes with a level of accuracy (around 75%), which offers judges valuable context. However, transparency concerns remain. The “black box” nature of some AI algorithms causes worry about the understanding and trust in their decisions.
Further, AI can personalize sentencing recommendations considering factors like past behavior, socioeconomic status, and likelihood of rehabilitation, leading to potentially more individualized justice. The ability of AI to adapt over time through ongoing training holds potential for more accurate sentencing in the future. Furthermore, the integration of psychological data with sentencing recommendations may lead to decisions better suited for individual needs, potentially improving the odds of successful rehabilitation.
However, relying on AI for sentencing comes with ethical dilemmas. If the AI is trained on biased datasets, it might perpetuate existing inequalities. AI can potentially enhance communication between legal professionals, social workers, and educators involved in rehabilitation efforts. It opens the possibility of real-time AI recommendations in courtrooms, potentially shifting how immediate decisions are made. These insights highlight both the potential and the risks that come with AI's increasing involvement in the delicate realm of juvenile justice.
AI in Legal Research Analyzing the Cassie Jo Stoddart Murder Case Documents - Ethical Considerations of AI in Sensitive Criminal Proceedings
The increasing use of AI in legal processes, especially within the context of sensitive criminal cases, presents a complex landscape of ethical considerations. AI's ability to analyze vast amounts of legal data, including documents and evidence, while potentially streamlining tasks like discovery, also introduces new challenges. The reliance on algorithms in critical legal decisions, such as determining evidence relevance or suggesting sentencing outcomes, can introduce the risk of biased decision-making, potentially perpetuating societal inequities. Moreover, the inherent "black box" nature of some AI systems, where their decision-making logic is not easily understood, raises concerns about transparency and accountability. This lack of transparency can hinder the acceptance of AI-driven conclusions within the courtroom, where clarity and demonstrable logic are paramount. As AI's influence on the legal profession expands, it's crucial to address these ethical complexities to maintain the principles of fairness and justice within the legal system. Balancing the potential benefits of AI with the need to safeguard due process and protect individual rights will be a defining challenge as we move forward in the age of AI-powered law.
1. **AI's Influence on Legal Decision-Making**: In complex legal situations, particularly criminal ones, AI systems can guide judicial decisions by providing insights gleaned from data analysis. However, relying on these automated tools raises questions about the balance between human judgment and machine predictions, potentially shifting the focus of accountability in legal proceedings.
2. **Potential for Bias in AI Models**: Studies suggest that AI algorithms used in legal settings, like assessing risk or suggesting sentences, can mirror existing biases in the data they are trained on. This presents a significant ethical issue, particularly when it comes to ensuring fairness in cases involving vulnerable populations.
3. **Streamlining Document Review**: AI-powered tools can significantly decrease the time spent on document review in legal cases, potentially by as much as 80%. While this speeds up the process, it leads to debates about whether AI's approach is as comprehensive as human review in intricate legal scenarios.
4. **Importance of Data Security**: Incorporating AI into legal practices demands robust data security measures, especially in sensitive cases. The risk of data breaches or unauthorized access to confidential information necessitates the implementation of strong safeguards to protect privacy.
5. **NLP's Limitations with Legal Language**: While natural language processing (NLP) can enhance legal document analysis, it can struggle to grasp the complexities and nuances of legal terminology. This can occasionally lead to misinterpretations that could impact the outcomes of cases.
6. **Predictive Analytics in Legal Strategy**: Some AI systems can predict legal case outcomes with a high degree of accuracy, around 80%, based on analyzing past cases. This ability can inform and optimize legal strategies, but it also raises the concern of over-dependence on algorithms instead of traditional legal reasoning.
7. **The Emerging Need for Tech-Savvy Lawyers**: As AI's role in the legal field grows, there's an increasing demand for lawyers with both traditional legal expertise and technological competence. This shift highlights a need for adjustments in legal education to ensure future lawyers possess the necessary skills.
8. **Transparency and Trust in AI Algorithms**: The lack of transparency in many AI algorithms, often referred to as the "black box" problem, makes it hard to understand how they arrive at conclusions. Understanding the decision-making processes of these systems is essential for maintaining public confidence in the legal system.
9. **Sentencing Outcomes and Human-AI Collaboration**: Research indicates that when AI sentencing recommendations align with judges' decisions, recidivism rates are often lower. However, discrepancies between AI recommendations and human choices have been linked to higher rates of reoffending. This illustrates the complex relationship between human judgment and AI-driven insights.
10. **Ethical Considerations for AI in Justice**: The ethical implications of integrating AI into sensitive criminal proceedings require constant monitoring and consideration. As the legal landscape evolves with these new technologies, it's crucial to regularly evaluate how AI systems uphold fairness and justice.
Automate legal research, eDiscovery, and precedent analysis - Let our AI Legal Assistant handle the complexity. (Get started for free)
More Posts from legalpdf.io: