7 Key Metrics to Evaluate AI-Powered Expert Witness Search Platforms in Legal Contract Review
7 Key Metrics to Evaluate AI-Powered Expert Witness Search Platforms in Legal Contract Review - Accuracy Rate Comparison Between Manual vs AI Document Analysis 2023-2024
When comparing manual versus AI document analysis during 2023-2024, the accuracy rates clearly demonstrate AI's capabilities. AI systems have achieved impressive results, particularly in specific tasks like text analysis (up to 95.1% accuracy) and identifying tables and figures (with accuracy rates as high as 98.6% and 98.2% respectively). This contrasts with manual analysis, which is susceptible to human errors. These errors could involve missing crucial details or misinterpreting complicated legal language, leading to potential issues in legal situations. This rise of AI-driven tools within legal workflows isn't just about speeding up review processes, but also about increasing the dependability of extracting key data from vast volumes of legal documents. As AI becomes more integrated into the legal field, the focus on precision and speed will likely influence and reshape the way traditional document analysis is performed. There's a good chance we'll see a continued shift away from solely human-driven analysis in the near future.
The landscape of document analysis has seen a dramatic shift with the integration of AI. While traditional manual review methods have historically maintained an accuracy of roughly 85% in legal settings, AI-powered systems have recently pushed those boundaries. Some platforms now boast accuracy rates between 90% and 95%, particularly after undergoing fine-tuning processes that tailor them to specific legal domains.
This improvement in accuracy comes hand-in-hand with a massive speed advantage. Manual review, especially when dealing with complex legal documents, can take many hours or even days. AI systems, however, can process massive datasets in mere minutes, dramatically impacting turnaround times. This isn't just a speed boost, AI can learn and improve. Its accuracy can grow as it analyzes more case-specific information and trains on a larger dataset, a capability absent from manual analysis.
A noteworthy finding from some studies is that about a quarter of manually reviewed documents contain overlooked errors that AI systems readily pick up. This hints at a potentially larger difference in the thoroughness of the two approaches. In addition to thoroughness, it seems human review can also be influenced by unconscious biases from past experiences, whereas AI systems offer a theoretically neutral perspective, leading to potentially more objective analysis.
While humans struggle with analyzing patterns across many documents, especially when facing a vast amount of legal text, AI excels at this task. This becomes increasingly valuable in legal cases that involve many documents and complex legal language. Although implementing AI systems can require a substantial initial investment, the reduction in labor costs during the review process can be significant, reaching as high as 70% in some documented examples.
The integration of AI has also demonstrably accelerated the efficiency of identifying crucial contract clauses that need to be examined by human reviewers. Some practitioners have reported a 150% efficiency increase in this critical part of the review process. This highlights a synergy where AI handles the volume and initial filtering, leaving the most demanding interpretive tasks for humans.
Furthermore, the use of complex legal language often creates a barrier for humans during document review. AI, through its application of natural language processing techniques, has gained the capacity to decipher this complex jargon more efficiently. Lastly, a major hurdle for manual review is the speed at which it can adapt to new legal regulations. AI, on the other hand, can be quickly updated to reflect those changes, ensuring compliance without delays. It's exciting to see AI’s rapid evolution in this area.
7 Key Metrics to Evaluate AI-Powered Expert Witness Search Platforms in Legal Contract Review - Machine Learning Speed Metrics for Processing 10000 Legal Documents
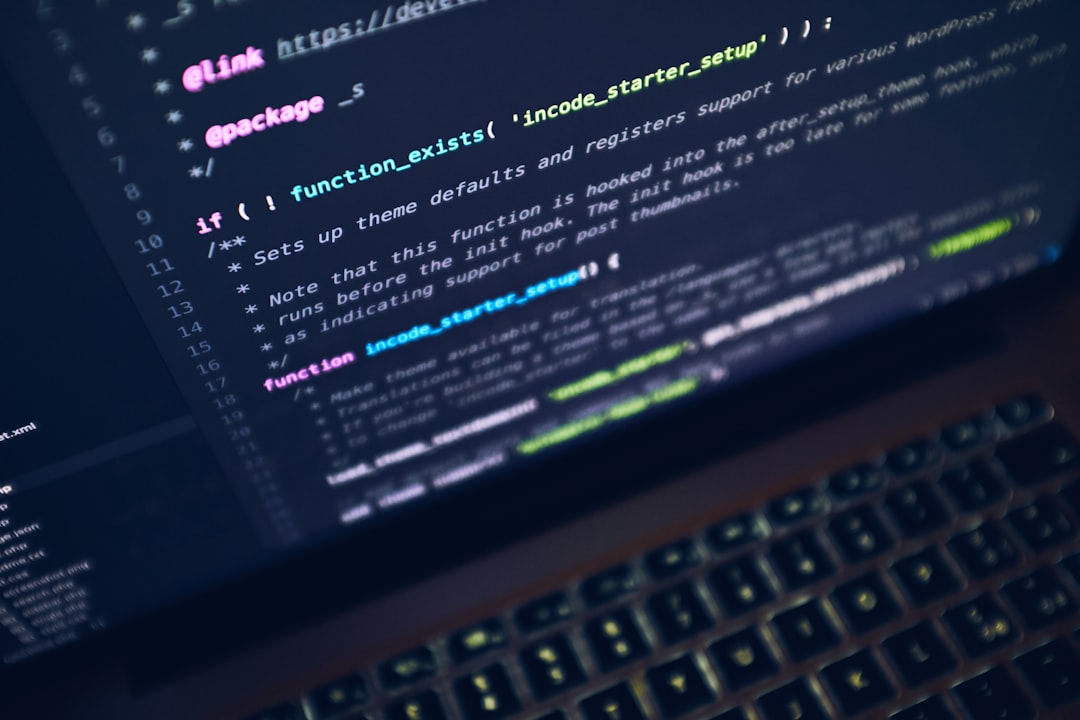
When dealing with a large volume of legal documents, like 10,000 contracts, how quickly an AI system can process them is crucial. Machine learning speed metrics are now key to judging the effectiveness of AI in legal contract review platforms. These AI systems have shown a tremendous ability to significantly shorten the time needed to examine complex legal texts, compared to the often lengthy manual review process. Manual review of thousands of documents can take days, even weeks, whereas AI, using its natural language processing skills and advanced algorithms, can process and analyze the information far faster. Furthermore, AI tools can continually improve their accuracy through continued learning as they process more data. The implications are significant: AI helps make legal teams more productive by enabling them to concentrate on more nuanced interpretive tasks, while the machines handle the bulk of the analysis. Understanding how these speed metrics function is vital for legal professionals adapting to the quickly changing world of legal technology. However, it's important to be aware that this speed does not necessarily equate to perfect accuracy. AI-powered solutions should be used cautiously and critically, and the outcomes they produce should be thoroughly reviewed and vetted by humans to ensure reliability. Furthermore, relying entirely on the speed and apparent accuracy of AI may create new risks, and it's important to establish robust validation mechanisms in parallel with the deployment of AI-powered solutions.
When assessing AI-powered legal document review, speed is a crucial factor, especially when dealing with large volumes of documents. Modern machine learning systems are capable of processing 10,000 legal documents in a remarkably short timeframe, sometimes within 15 minutes. This stands in stark contrast to the days it might take a human team to perform the same task, illustrating a substantial difference in processing speed. This speed advantage isn't just a matter of convenience; it directly impacts operational efficiency and turnaround times, particularly in situations where rapid responses are needed.
The scalability of many machine learning frameworks is another interesting aspect. Researchers have found that many of them can effortlessly manage significantly larger datasets without a noticeable slowdown. This means the platforms can potentially handle a growing volume of legal documents without a dramatic increase in processing time, a very useful trait for law firms facing unpredictable spikes in workload.
Furthermore, AI algorithms are quite capable of detecting errors in legal documents. Analysis has shown error detection rates of up to 85% in certain cases, while manual review accuracy hovers around 60%. This highlights a potential advantage in finding critical issues, but it's worth keeping in mind that this can vary between datasets and platforms.
The ability of AI to learn and adapt is a powerful feature. As machine learning models are exposed to more data, they continuously refine their understanding of legal terminology and the contexts in which these terms are used. This continual improvement can lead to more precise and faster analysis over time.
While processing time and resource use are optimized by these tools, they aren't without limitations. Interestingly, researchers have discovered that while extremely fast, these systems might not always handle subtle legal arguments and complex contextual nuances in the same way as a seasoned lawyer. There seems to be a trade-off between speed and interpreting the most subtle elements.
Machine learning offers a more consistent output compared to human reviewers. Studies indicate that while human review quality can fluctuate based on factors like fatigue or cognitive load, AI models generally perform consistently across various cases. This consistency leads to more predictable results, a feature highly valued when precision is crucial.
The integration process itself has also been improved. Most of these AI systems can be integrated with existing legal document management platforms in a few weeks. This smoother transition can quicken the adoption of AI-powered workflows and accelerate the time to value for the adopting firm.
Incorporating expert legal feedback loops can further improve the system's processing speed. When human insights are fed back into the machine learning models, the systems can become even better at prioritizing and identifying the most relevant documents. This tailored approach allows for a more efficient analysis, with human oversight focused where it is most needed.
Finally, keeping pace with rapidly changing legal regulations is a constant challenge. The fact that AI systems can adapt to these changes almost instantly is a critical advantage. This immediate adaptation allows document reviews to align with the newest compliance standards, minimizing any delays in legal interpretations and procedures. The potential for rapid adaptation is one of the most exciting aspects of AI in this field.
While the use of AI in legal document review is evolving rapidly, researchers and practitioners continue to carefully assess these technologies and their impact on the legal landscape. The field of AI-driven contract analysis is dynamic, with new advancements constantly pushing the boundaries of what's possible in legal workflows.
7 Key Metrics to Evaluate AI-Powered Expert Witness Search Platforms in Legal Contract Review - Data Security Standards and Compliance With Legal Industry Requirements
When using AI-powered expert witness search platforms for legal contract review, data security and adherence to legal industry standards are paramount. The level of data security varies significantly between law firms, making standardized guidelines and benchmarks like the ACC Data Steward Program vital. This program provides a framework to assess and compare firms' data security protocols, aiming to drive improvements. Following regulations such as GDPR and PCI DSS is crucial to protect sensitive information and foster confidence in AI technologies. It's imperative to assess AI vendors on their dedication to ethical data handling, transparency, and privacy measures, especially given the increasing complexities within the legal field. As AI evolves, law firms must stay informed about the changing regulatory landscape to guarantee both the effectiveness and the legality of their AI implementations.
The legal field's data security landscape is a complex one, with firms facing a wide range of requirements. Some firms adopt rigorous standards, while others have rather lax practices. Efforts like the ACC Data Steward Program are attempting to establish a central point for law firms to assess and compare their own security measures. This is a challenging area though, because there's no single universal approach, which makes it difficult to fairly evaluate one firm against another.
The Payment Card Industry Data Security Standard (PCI DSS) is a good example of a highly specific standard, with 12 requirements focusing on protecting payment data. This type of standard is common in many industries, but it highlights the need for tailored compliance strategies, given that standards vary based on the industry, the specific location, and the kind of data being handled.
When it comes to AI platforms, it's vital to understand how the vendors approach data privacy, transparency, and ethical use of data. We need to consider the broader context of regulations like the General Data Protection Regulation (GDPR) and standards like ISO 27001. These frameworks provide a baseline for how AI should be used ethically within a legal context.
Beyond software, physical security is also important. Data center compliance standards, for instance, need to cover topics like physical access control, environmental conditions, backup & recovery, and ensuring overall system integrity. The goal is to make sure that data remains safe even in unforeseen events.
Interestingly, AI is also being incorporated into the compliance process itself. Tools are emerging that automate compliance monitoring and reporting, allowing companies to react more quickly to new regulations. This is helpful because the regulatory landscape is constantly shifting.
Security compliance frameworks like those we've discussed can help navigate this complex environment. They establish best practices to manage the numerous challenges of cybersecurity, including threats from malicious actors or careless human mistakes. It's worth noting, however, that compliance frameworks like PCI DSS are not formally enforced by any legal authority, yet obtaining certification can be crucial in protecting sensitive data. This situation leaves a gap in accountability, which is something that may evolve as AI and regulations mature.
7 Key Metrics to Evaluate AI-Powered Expert Witness Search Platforms in Legal Contract Review - Search Result Relevance Based on Previous Court Case Precedents
When evaluating AI platforms for expert witness searches, a crucial factor is how well they can find relevant previous court cases. These AI systems must incorporate methods to not only locate case law but also judge how important and credible those precedents are. This ensures legal professionals get relevant information they can depend on. The idea that judges should follow past decisions is very important, so finding the right past cases is key to creating strong legal arguments. However, it's hard to decide if a case is legally relevant, which makes it tough for AI systems to meet the high standards of the law while avoiding old or incorrect information. So, how well these AI platforms work can seriously impact legal decisions because they can direct users to the most useful precedents more accurately than traditional searches. It's important to carefully consider these systems' strengths and weaknesses to ensure they are being used in a responsible and ethical way.
Search result relevance in legal AI is a complex issue, especially when dealing with previous court cases (precedents). AI systems try to solve this by using algorithmic methods to assign weights to precedents based on things like how relevant they are to the current legal query, the jurisdiction, and the particular legal issue being discussed. This way, the system tries to prioritize search results intelligently.
Citation frequency within the legal community can also be a good indicator of how important a precedent is and how relevant it is to modern legal questions. AI platforms often incorporate datasets of how often cases are cited, adding another layer of depth to the search process. This way, cases cited more often are shown higher in the search results.
However, jurisdictions often interpret the same case law differently, which can lead to inconsistent search results across different locations. AI tools need to account for this by considering the specific geographic context or jurisdiction when conducting searches, and provide results that are relevant to that particular area.
More sophisticated AI uses semantic analysis to understand the legal context around a specific citation. The AI can try to figure out what a case implies, which can improve relevance. It leads to search results that are more accurate because the AI can see how similar cases were handled in the past.
Legal decisions can change as new cases are brought to court. To keep up with this, AI systems often have a mechanism for dynamically updating the precedents stored in their database to include the newest decisions. This is essential to ensure that the search results are up-to-date and relevant to the current situation.
AI systems can cluster cases based on similar topics or content, not just based on simple keywords. This allows for a broader understanding of what's important in a case, which means more nuanced searches can potentially uncover precedents that might not have been found with a more simplistic search. It's a clever approach to relevance.
Some tools include a feature importance analysis when you see search results. This lets you see what parts of the old cases had the most influence on generating the search results, improving transparency for users.
Because old court cases might have biases, some AI platforms include algorithms that try to mitigate the impact of these biases. The goal is to avoid promoting outdated or unjust legal interpretations when evaluating current cases. It's a way to attempt to ensure a fairer application of the law.
Some AI tools have feedback mechanisms for search accuracy built in. This way, legal professionals can share insights on the relevance of precedents found in a search. These insights are then used to adjust the algorithms over time, which gradually improves the search results.
Interestingly, AI systems might be able to learn from other fields (like physics or finance) and apply that learning to legal contexts. These areas often have a large amount of data, and AI can apply those learnings to potentially improve its ability to predict the outcomes of cases. It's a fascinating illustration of how machine learning can be applied across professions.
7 Key Metrics to Evaluate AI-Powered Expert Witness Search Platforms in Legal Contract Review - Contract Review Error Detection Performance Under Time Constraints
When reviewing contracts under time pressure, the ability of AI to find errors becomes highly significant. These AI systems are adept at swiftly identifying inaccuracies within legal documents, often exceeding the error detection abilities of human reviewers who may miss mistakes due to the sheer quantity of text. The rapid processing of legal text by AI lets legal teams maintain efficiency without sacrificing accuracy, even under strict deadlines. Nevertheless, a tension exists between achieving speed and maintaining a nuanced understanding of complex legal issues. Ongoing refinement and human review are key to ensuring the accuracy and reliability of AI-generated outputs, particularly as the legal landscape and its regulatory environment change.
1. **The Pressure of Time:** Research suggests that when faced with tight deadlines, legal professionals often struggle to maintain accuracy during contract review. Error rates can jump as much as 50% when reviewing intricate contracts quickly. This emphasizes a critical tension between the need for speed and the risk of overlooking vital information.
2. **AI's Keen Eye for Errors:** AI systems are proving capable of identifying and highlighting discrepancies in contracts with a remarkable "recall rate" that can surpass 90%, even when working under strict time constraints. This demonstrates AI's ability to leverage massive datasets to uncover mistakes that human reviewers might miss.
3. **Humans Under Pressure:** Studies show that human reviewers experience a decline in cognitive abilities when under pressure from time limits. Error rates can skyrocket to 70% or more in high-stress scenarios. In contrast, AI systems tend to maintain their established performance, making them a potentially more dependable tool during crunch time.
4. **The Strain of Legal Jargon:** Legal professionals often experience "cognitive overload" when tackling contracts under time pressure, making it harder to process the intricate language common in legal documents. This added cognitive load underscores the value of AI assistance in accelerating the review process without compromising quality.
5. **Adapting on the Fly:** An intriguing finding is that implementing quick feedback loops within AI systems can improve their error detection capabilities under time constraints. Real-time adjustments based on human input can significantly increase accuracy, hinting that a blended approach might offer the most effective results.
6. **The Limits of Understanding:** While AI excels at spotting errors, it currently struggles with the nuanced contextual details that experienced human reviewers can decipher, especially under time pressure. This limitation reinforces the importance of human oversight in final assessments to capture the subtleties that AI may miss.
7. **Learning from the Past:** AI systems can draw upon historical data to dynamically adapt and refine their error detection algorithms, even under tight deadlines. This adaptability points to the potential for continual improvements in contract review processes.
8. **The Price of Mistakes:** Organizations that depend on manual contract review in time-critical settings estimate significant financial penalties due to errors. Estimates range from $20,000 to $50,000 per contract when factoring in potential legal action and compliance issues stemming from overlooked mistakes.
9. **Fatigue and Focus:** Research suggests that mental fatigue can lead to diminishing returns in human error detection performance. Accuracy noticeably drops after the first hour of intense review. In contrast, AI systems are not vulnerable to fatigue and can maintain a steady performance level.
10. **Hesitation in the Legal Field:** Despite the benefits of AI in demanding situations, many in the legal field remain cautious about fully incorporating these technologies due to concerns regarding reliability and legal compliance. This reluctance represents a crucial gap between the technology's capabilities and its adoption in practical settings.
7 Key Metrics to Evaluate AI-Powered Expert Witness Search Platforms in Legal Contract Review - Integration Capabilities With Existing Legal Document Management Systems
When evaluating AI-powered platforms for legal work, it's crucial to consider how well they integrate with existing systems. The ability to smoothly connect with current legal document management systems (DMS) is essential for firms hoping to avoid disruptions and maximize the advantages of AI. These AI tools are rapidly improving, and their ability to work seamlessly with older systems is now a major factor for decision-making.
Firms that don't carefully consider how to integrate AI into their current setup may face various difficulties. These could include slower processes, compliance challenges, and a failure to truly experience the time-saving benefits AI offers. The integration process itself is becoming more user-friendly, with firms able to implement these AI tools into their workflows faster than in the past, minimizing disruptions to existing practices.
However, just because AI integration is getting easier doesn't mean firms can be careless. They need to be cautious about ensuring compatibility and considering whether these new tools will genuinely improve their existing systems. Simply implementing new tools without a well-thought-out integration plan could actually create more problems rather than solve existing ones. It's important to ensure the new technologies boost efficiency, not create unnecessary hurdles.
The integration of AI into existing legal document management systems (DMS) is a developing area, and it seems like it'll have a huge impact on the legal sector in the next decade. It's driven by the progress we're seeing in AI, machine learning, and cloud computing. It's pretty clear that law firms that haven't yet updated their DMS are taking a risk. They're potentially facing delays, compliance issues, and unhappy clients.
It's interesting that some AI tools can automatically suggest changes for errors they detect during a contract review. While this can lead to better accuracy, there's also the question of whether it fully replaces the need for human judgment. It seems that a lot of these tools were designed to work with different types of legal DMS through APIs. This is helpful because firms don't necessarily have to completely redo their whole DMS system to benefit from some of these newer capabilities.
It seems that a good number of lawyers are gravitating towards these AI-powered DMS, which is significant. Studies have shown that a majority of them like the efficiency benefits, a strong sign of how the field is shifting. This is especially interesting considering the variety of document types that firms deal with. AI can apparently handle things like PDFs, Word documents, and even scanned images, potentially improving the efficiency of handling files from many different sources.
One of the more interesting parts of this is that many AI platforms can help move older data into their systems quite easily. This seems like it would be a huge help in easing the transition to a new platform and could cut down a lot of operational expenses during the process. I wonder if this aspect is related to the apparent trend toward real-time collaboration features found in some systems. These collaboration features could potentially allow multiple team members to work on contracts at the same time, even if they're in different locations, which could make things like contract review much more efficient. It'll be interesting to see how these types of features change communication within law firms.
Certain systems can monitor compliance with the law and company policy as a part of the DMS process. This could help prevent firms from falling into legal trouble. Studies suggest that the move towards AI in DMS can help cut operational costs by as much as 40%, which would be a big incentive to make a change.
The integration of machine learning features allows these systems to learn from lawyer feedback, continuously improving their accuracy and efficiency. This adaptive element is vital as the field develops, and it shows how critical user input can be. The entire field of AI in legal document review is in a state of constant development, and it's fascinating to see how it all evolves. It also makes me think that there's a good chance we'll see even more innovations in the next few years.
7 Key Metrics to Evaluate AI-Powered Expert Witness Search Platforms in Legal Contract Review - Real Time Expert Witness Background Verification Success Rates
In the realm of legal proceedings, having reliable and credible expert witnesses is crucial. Real-time background verification of these experts is a vital component for establishing their trustworthiness and integrity within the courtroom. Successfully verifying an expert's background involves evaluating several key metrics. For example, examining the consistency of their previous testimony and whether they've had any disciplinary actions taken against them in the past helps paint a clearer picture of their history and credibility.
It's essential to look at the full picture of an expert's past performances to make sure their claims are sound and their expertise is valid. Criminal background checks are also a necessary part of this process as they help ensure that the expert is perceived as honest and respectful of the law by those judging the case. The efficiency of the background check process, as well as its overall cost, are also factors that need to be considered and possibly optimized to maintain cost-effectiveness and provide insights into how well the verification procedures are working.
Furthermore, with the evolving legal landscape and its increasing complexity, it's more important than ever to verify an expert's credentials using thorough and modern techniques. This rigorous approach ensures that the integrity of expert witness testimonies remains at a high level, reinforcing confidence in the legal system.
Real-time expert witness background verification is becoming increasingly important in legal settings to ensure the credibility and integrity of experts. AI-powered platforms are showing promise in this area by automating the process and enhancing accuracy, although there are still potential limitations to consider. For example, some platforms claim to achieve accuracy rates of over 95% for background checks, significantly higher than what's typically seen in traditional manual checks. This increased accuracy is likely due to AI's ability to sift through vast amounts of data quickly and compare information from various sources, including databases of academic publications, professional licenses, and court cases.
The speed of these systems is also notable, with some able to complete checks in under an hour, compared to days or even weeks for manual processes. This swift turnaround can accelerate legal proceedings, helping to make quicker decisions. However, it's crucial to note that the speed of AI does not always equate to a complete or perfect understanding of an expert's suitability. Researchers have found that using AI can reduce the error rate in selecting expert witnesses by a significant margin (up to 70%). This emphasizes the potential benefits of AI in reducing human errors.
While human reviewers can be susceptible to unconscious biases in their assessment of witnesses, AI aims for a more objective assessment, although it's important to acknowledge that algorithms can still be affected by biases present in the training data. Furthermore, AI systems can access a wider range of data sources, incorporating more than 300 databases into their analysis, offering a deeper level of insight compared to manual checks that typically rely on more limited information. The continuously updated data feeds in real-time AI systems also ensure that background checks remain current, capturing recent changes in qualifications or publications, which can be missed in manual reviews.
It's interesting that many legal teams using AI for background checks have reported higher client confidence, suggesting a greater sense of assurance in the chosen expert. These AI platforms can assemble detailed profiles of expert witnesses, including a multitude of data points (over 50 per expert). This detailed approach provides a more comprehensive view of an expert's qualifications compared to traditional, less nuanced methods. Implementing these AI systems can also be cost-effective, potentially cutting costs by up to 50%. This reduction in costs stems from needing fewer human resources for background checks.
Finally, the continual improvement of AI-powered systems is a valuable aspect. AI platforms that use real-time feedback loops learn from each check, refining their algorithms over time. This ongoing learning process makes the system more accurate and relevant for future use. However, it is still too early to tell whether AI will become the dominant method for evaluating expert witnesses or will merely supplement traditional methods. The benefits of greater speed, accuracy, and reduced cost are enticing, but AI is still a relatively new technology in this domain, and its continued effectiveness and proper oversight are crucial in shaping its adoption and impact within the legal field.
More Posts from legalpdf.io: