7 Critical Terms Often Overlooked in AI-Related NDAs A Contract Review Perspective
7 Critical Terms Often Overlooked in AI-Related NDAs A Contract Review Perspective - Training Data Usage Rights and Ownership Boundaries in AI Development
The use of data to train AI systems is increasingly complex, demanding a clear understanding of data usage rights and ownership boundaries. While AI development pushes forward, laws like the proposed AI Act are starting to emphasize data governance as a key component, from data acquisition to model deployment. This emphasis is particularly important for high-risk AI systems, and extends beyond existing regulations like GDPR. However, much remains unsettled, especially regarding copyright and its application to AI training data. The legal concept of "fair use" is being hotly debated in relation to the massive datasets used to train AI models. Moreover, the drive towards more transparency, potentially requiring developers to disclose training materials, seeks to give content creators more control, but also presents a challenge. Developers must tread carefully, balancing the desire for innovation with the ethical and legal responsibilities inherent in the use of data, especially where copyrighted or sensitive data is concerned. Failure to address these aspects could lead to costly legal entanglements, and possibly damage the reputation of developers and their AI creations.
AI model development relies on vast amounts of data for training, and the legal landscape surrounding this data is complex. While it might seem like publicly available data is free for the taking, the original data creators often retain specific rights and restrictions on how it can be used, even for AI purposes. This can be a surprise for developers assuming unrestricted access.
Furthermore, the legal landscape isn't uniform. What's acceptable in one country concerning data usage might be legally problematic in another. This makes it crucial for developers aiming for global AI deployments to be extremely mindful of the varying data regulations in different jurisdictions.
Data licensing agreements can be particularly tricky. They often have clauses restricting how AI models can be trained on the data, such as prohibiting commercial uses or mandating attribution, which may be difficult to implement in certain AI contexts. Open-source data, while appearing freely available, can also be misleading. Often, contributors maintain particular rights, making a careful review of the license essential before incorporating the data into an AI project.
The process of enhancing or modifying datasets, known as data augmentation, can bring up new ownership issues. If the alterations are extensive enough to be considered a new dataset, it could spark disputes over who owns that derived data. Similarly, contracts often touch on what constitutes a “derivative work” based on the original data, including outputs generated by AI, leading to uncertainty on rightful ownership of these results.
Another common oversight is the unclear fate of data once a training contract ends. Many agreements are silent on whether the data can be kept for future development after the contract's termination, creating ambiguity. Furthermore, copyright laws relating to training data might differ depending on whether it contains personal information, introducing consent complexities.
The evolving nature of AI also poses new legal questions. Courts are starting to recognize AI-generated outputs as copyrightable material, a development that could significantly alter our understanding of data ownership in AI development. It's also a significant problem because training data often includes copyrighted works not specifically exempt, raising the risk of infringement if developers fail to secure the appropriate rights before incorporating that data into AI training. The need for responsible data practices and legal awareness becomes paramount for AI development to avoid future issues.
7 Critical Terms Often Overlooked in AI-Related NDAs A Contract Review Perspective - Model Output Licensing Terms and Derivative Works Definition
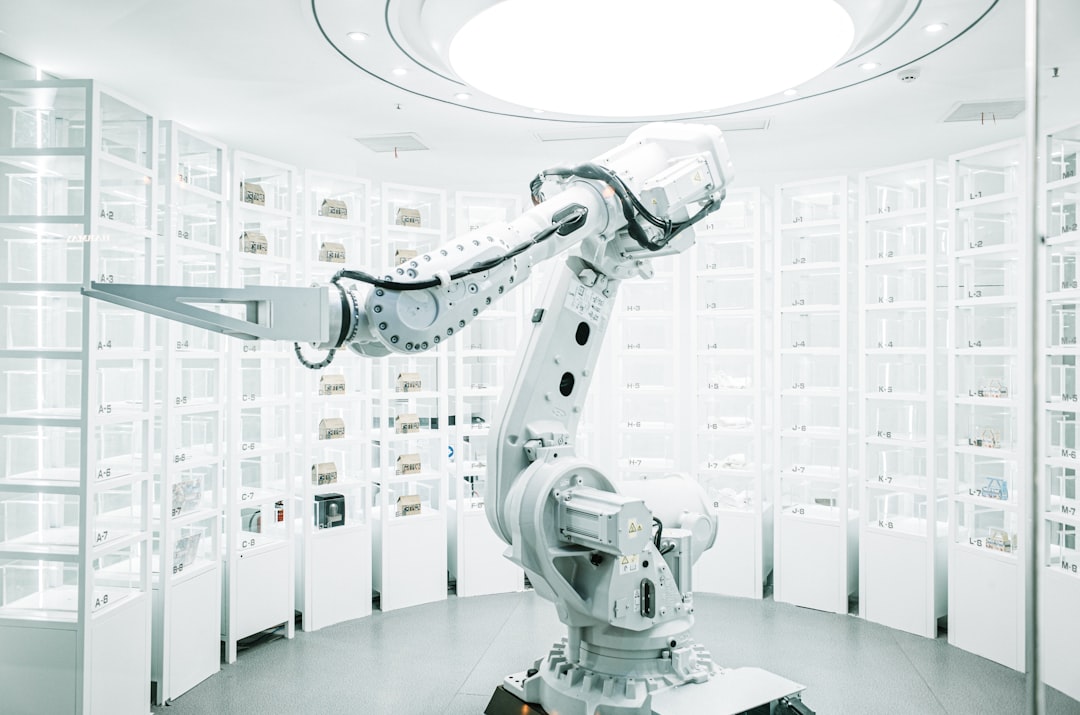
When dealing with AI agreements, the terms related to model output licensing and the definition of derivative works are often overlooked, yet are fundamentally important. These terms become particularly complex as AI evolves, especially when considering how models are fine-tuned or retrained. The definition of a "derivative work" in this context can shift, leading to ambiguity around who owns the resulting intellectual property.
The way AI-generated outputs are classified greatly impacts the control creators have over their work. Generative AI, in particular, challenges traditional notions of originality when it comes to copyright law. This blurring of lines makes it increasingly crucial for everyone involved – developers, users, and creators – to understand how these terms are defined within the agreements. Establishing clear boundaries is essential for protecting the rights of all parties and creating effective policies around AI-generated content. Failing to pay attention to these seemingly small details could cause significant future conflicts.
When we delve into the nitty-gritty of AI contracts, especially those related to using models, a lot of focus is put on things like data usage rights. But often overlooked are the licensing terms around the model's output itself and how it's defined as a "derivative work." It's fascinating how these licenses often reimagine what a derivative work is, especially in the context of fine-tuning or transfer learning for AI models. The way many licenses treat retrained models—whether they're seen as new creations or still bound by the original terms—can be quite perplexing.
Furthermore, you'll find that the end-user license agreements (EULAs) associated with AI models often come with specific rights and responsibilities for users. It's really crucial to grasp these terms to safeguard your data and the model output. Since many companies utilize AI models they've licensed rather than creating from scratch, it becomes imperative to acknowledge the potential risks and rewards inherent in these licensing agreements.
It seems there's a growing interest in establishing a clear framework for open-source AI, including areas like managing training data and code. Organizations like the Open Source Initiative are playing a key role in this endeavor, aiming to develop comprehensive licensing guidelines. Copyright law generally defines derivative works as any adaptation or transformation that features original creative input. This definition is quite broad, and in the context of AI, it becomes particularly significant when it involves artistic works used for AI training. Licensing terms can play a huge role in determining the degree of control an artist or creator retains over their work if that work becomes part of an AI model's training dataset.
Defining "derivative works" when it comes to AI-generated outputs is becoming increasingly crucial. This is particularly relevant for establishing copyright policies around large language model (LLM) outputs. It's intriguing how generative AI often gets dubbed as having a "poison pill" effect, primarily because of the broad interpretation of "derivative works" in relation to copyright implications.
Comprehending these quirks of AI licensing can help avoid potential pitfalls. It's crucial to ensure that licensees are fully aware of their commitments and the legal implications, so that companies don't oversell the capabilities of models that are bound by restrictive licenses. The vagueness that sometimes crops up in licensing terms regarding AI-generated content can present real issues, especially if there are geographic discrepancies in legal interpretation. For instance, what's legally permissible in one country might be entirely off-limits in another. This poses a challenge for those seeking global AI deployment. Additionally, contract clauses concerning what happens to the data at the end of the license agreement can be ambiguous. Developers may believe they have perpetual rights to utilize the data for future projects, whereas the licensing agreement may have a termination clause that dictates otherwise.
There are also concerns when dealing with a model's output: many licensing agreements require developers to take certain actions if they inadvertently violate terms. This can lead to delays or legal complexities that could significantly hinder ongoing projects. A common issue is understanding Creative Commons licenses and how they relate to derivative works and AI. Many who contribute data under Creative Commons may not fully grasp the implications of their licensing choices and how they impact AI's outputs. There's also the general misunderstanding about the implications of public domain data. Many developers seem to think that it's fair game to incorporate such data without constraints or limitations. In reality, there might still be stipulations like attributions that must be adhered to. Finally, some AI license agreements require attribution of the origin of outputs. It’s important to maintain transparency while staying compliant with contractual obligations.
7 Critical Terms Often Overlooked in AI-Related NDAs A Contract Review Perspective - Data Privacy Standards and Cross Border Transfer Rules
Data privacy is increasingly important in AI development, particularly when it involves transferring data across borders. Efforts like the Global Cross-Border Privacy Rules (CBPR) aim to create a framework that promotes trust and safeguards privacy while allowing for the international movement of data. However, this is a complex issue as countries implement their own data protection laws with differing levels of stringency. China, for instance, has its own set of laws around data security and the protection of personal information, like the Personal Information Protection Law (PIPL), which can complicate matters for businesses operating internationally.
The challenge is to navigate a path where the free flow of data, essential for economic growth and AI innovation, is balanced with robust data protection measures. This has led to global conversations about how to harmonize privacy standards and create a consistent approach to cross-border data transfers.
At the same time, there's a growing use of privacy-enhancing technologies to address these challenges and help manage the risks involved in transferring data. It's vital that developers and businesses engaged in international AI projects fully understand and comply with various data privacy standards. Failure to do so can lead to legal problems and damage the reputation of those involved. The future of AI will likely depend on finding a healthy middle ground between facilitating global data exchange and protecting individual privacy.
Data privacy standards and cross-border transfer rules are becoming increasingly complex, especially as AI development accelerates. Different regions have wildly varying regulations, with the EU's GDPR being a prime example of stringent controls over personal data. This contrasts sharply with the more piecemeal approach seen in the US, which can make navigating international data transfers quite tricky for businesses.
One issue that often gets overlooked is the potential for hefty fines related to data breaches when crossing borders. The GDPR, for instance, can slap companies with fines up to 4% of global turnover, really highlighting the importance of being careful. The EU-US Privacy Shield, meant to make things easier, ended up being declared invalid a few years ago, illustrating how quickly these rules can change and the need to always be looking for updates.
The idea of keeping data within a country's borders, called "data localization," is gaining momentum. This has major implications for both logistics and costs for companies running operations in multiple countries. It's interesting to note that a company can still be held responsible if a third-party vendor they use to handle data has a breach, even if the company itself has followed the rules.
Consent to data transfers is another murky area. When it comes to huge datasets or anonymized info, it's unclear what exactly counts as informed consent, especially in a global context. The increasing reliance on AI for data processing makes robust data privacy safeguards even more important, given how much data AI systems require.
It's also tricky to decide if data is truly anonymized as new re-identification methods seem to pop up all the time. This means that anonymization might not always offer the protection it is intended to. The GDPR's “one-stop-shop” mechanism is meant to make compliance easier by centralizing it in a single EU country for multinational companies, but this can also lead to uncertainty about jurisdiction and potential fragmentation of regulation.
Finally, we must remember that data privacy standards and regulations are constantly evolving with technology. Practices considered acceptable now might be scrutinized later on, forcing organizations to revisit their methods. This constant need for adjustment means that AI companies, especially, must stay vigilant about compliance in this ever-changing environment.
7 Critical Terms Often Overlooked in AI-Related NDAs A Contract Review Perspective - Algorithm Adaptation and Modification Permissions
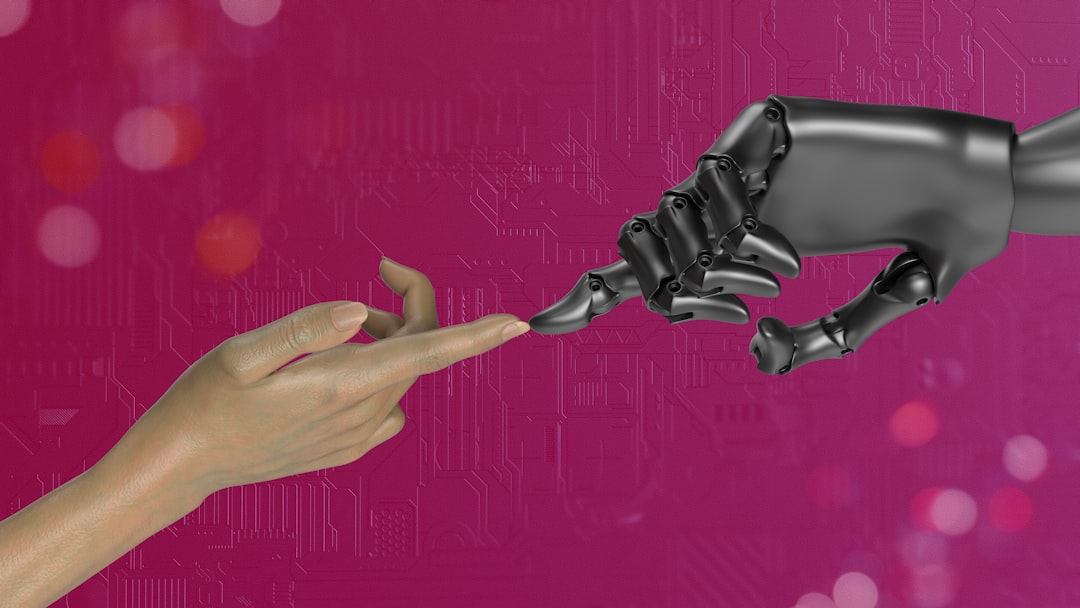
**Algorithm Adaptation and Modification Permissions**
Within the intricate world of AI agreements, the permissions related to adjusting and changing algorithms are crucial for establishing clear ownership and rights over any new intellectual property that arises. Because the field of AI is constantly changing, it's vital to have clear contract language about what counts as an acceptable modification. This can help prevent legal issues and make sure everyone's interests are protected.
Determining who owns changes to an algorithm can be tricky, especially when algorithms are refined or retrained. This can blur the lines of who is the original creator, leading to uncertainty. Furthermore, as conversations about algorithmic fairness and transparency become more important, the permissions laid out in contracts must consider ethical implications. It's essential to ensure that modifying algorithms doesn't accidentally reinforce biases or create discriminatory outcomes.
In conclusion, having a clearly defined structure for algorithm adaptation and modification permissions is essential for promoting responsible AI development while also safeguarding the rights of those who created the original algorithm. It’s a crucial element for a strong AI agreement.
When examining AI-related agreements, the details around adapting or changing algorithms are often overlooked, yet they're surprisingly crucial. It's not always clear exactly what "algorithm modification" means across different countries and legal systems. This lack of a universal definition can be confusing for developers, leading to uncertainty about what changes they're permitted to make.
The question of who owns a changed algorithm can be particularly messy. A seemingly small tweak can result in the altered algorithm being categorized as a "derivative work," triggering potential copyright infringement if the original creator hasn't given specific permission. This issue becomes even more complex when considering the type of AI being used. For instance, the rules governing changes to algorithms in proprietary versus open-source AI can be very different.
If an algorithm is modified without clear permission, it can open up the developer to substantial liability, especially if something goes wrong due to those changes. This is particularly true in fields like healthcare, where a faulty algorithm could have serious negative consequences. This highlights the importance of clear agreement before making any adaptations.
When multiple parties work together on an AI project, having fuzzy language regarding algorithm changes can quickly lead to conflicts that hinder development. Defining modification rights upfront is key to ensuring everyone is on the same page.
Algorithms often contain valuable trade secrets. If the terms concerning changes aren't precisely defined, discussing potential modifications within collaborative projects can become problematic. There's a real chance sensitive information could be inadvertently exposed.
There's a growing recognition that algorithm licensing needs its own specific structure, resulting in platforms designed for this very purpose. This shift suggests a move toward clearer and more structured agreements in the broader AI space.
What's considered acceptable in terms of algorithm modification can vary drastically across countries, leading to headaches for global AI teams. If you're working across borders, understanding these international differences is paramount.
Many developers might not realize that simply adjusting a publicly available algorithm can potentially violate copyright law. This is particularly true if the modified version still leans heavily on the original work without a proper license.
Open source AI often comes with its own set of regulations surrounding changes to algorithms. This typically involves stipulations about attribution and sharing adapted versions under the same licensing terms as the original. These rules need to be closely examined to prevent future legal problems.
In the realm of AI contracts, the often-overlooked details of algorithm adaptation and modification can have significant implications. Navigating these complexities requires a thoughtful approach to minimize potential risks and foster a more responsible and ethical AI landscape.
7 Critical Terms Often Overlooked in AI-Related NDAs A Contract Review Perspective - Performance Metrics and Liability Thresholds
When negotiating AI contracts, it's crucial to include clear performance metrics and liability thresholds to ensure both parties understand their responsibilities and potential risks. This is especially important when working with third-party AI developers or providers. Properly designed contracts should include specific performance metrics, such as cost, speed, and accuracy of the AI's outputs, to measure the success of the project. Having well-defined performance metrics helps both parties stay aligned and makes it easier to spot potential issues early on.
Equally crucial are liability thresholds – these outline the level of responsibility each party assumes if the AI fails to meet the agreed-upon performance metrics. Without them, disputes about who is to blame for a problem or how much it will cost to fix it can drag on, potentially hindering future projects.
Often, these elements – performance metrics and liability thresholds – are missing or vaguely defined in AI agreements, leaving room for ambiguity and potential conflict. Contractual clarity on these points helps prevent misunderstandings and clarifies the expectations for both parties, making the collaborative effort more productive and protecting both sides from unnecessary financial or reputational damage. This level of detail is often overlooked, yet it's vital for fostering successful collaborations and managing risks effectively, especially in the still-evolving AI landscape.
Performance metrics in AI contracts aren't simply about how accurate a model is. We're seeing a growing emphasis on things like fairness, how well the system can be explained, and whether it's accountable for its actions. This wider view of performance recognizes that AI's influence extends beyond producing correct outputs and into broader societal and ethical considerations.
The level of responsibility (liability) outlined in these contracts can fluctuate wildly based on how complex the algorithms are. More intricate AI systems usually call for more detailed liability agreements to effectively divide the risk. This can add to the costs of development, making it a significant factor for companies to consider.
It's surprising how many organizations don't fully appreciate the value of consistently tracking performance metrics. There's a tendency to think that an initial assessment is sufficient. However, continuous monitoring is critical because AI models can lose their effectiveness over time as the nature of the data used to train them changes – a phenomenon called model drift.
When deciding which performance metrics to use, we must be cautious of inadvertently introducing biases. For example, using only accuracy as a measure can obscure fairness problems for underrepresented communities. A diverse range of metrics is crucial to comprehensively assess the effects of an AI system.
Legal ramifications concerning liability thresholds can quickly become intense. Decisions regarding who's liable could lead to damages in the millions, especially in sensitive areas like healthcare, where AI models directly affect patient outcomes.
Defining performance metrics before launching an AI model can help reduce liability risks. A clear understanding of what counts as acceptable performance serves as a baseline to assess liability if the model falls short of expectations.
Intriguingly, performance metrics can have a dual nature when interacting with regulations. They're fundamental for accountability, but overly restrictive metrics could stifle innovation by focusing on compliance instead of fostering creativity.
In many countries, defining performance metrics and liability thresholds are viewed as part of 'due diligence'. This suggests that failing to adequately define these terms in a contract could be interpreted as negligence.
The process of incorporating liability thresholds into contracts is still developing. Many AI developers aren't fully aware that they could be unknowingly restricting their ability to address potential issues by phrasing their liability clauses too narrowly.
The debate surrounding performance metrics isn't purely technical; it's interwoven with broader discussions about transparency and ethics in AI. If the metrics used aren't made clear, it can lead to suspicion and mistrust from various stakeholders, hindering broader AI adoption and regulatory acceptance.
7 Critical Terms Often Overlooked in AI-Related NDAs A Contract Review Perspective - Competitive Development Restrictions and Timeframes
Within AI-related agreements, "Competitive Development Restrictions and Timeframes" are often overlooked but are incredibly important. These contractual provisions define the legal boundaries surrounding how parties can engage in competitive development activities after a collaboration or agreement ends. They essentially establish a period of time where one or both parties may be limited in developing or deploying similar AI technologies.
These limitations can help protect a company's investments in innovative technologies by preventing immediate competition from partners or collaborators. However, they can also act as obstacles to innovation, slowing down development within the broader field. The challenge lies in crafting restrictions that are both protective and reasonable, so they don't unduly stifle future advances.
Defining these terms requires great care. If the language used is unclear, it's more likely there will be disagreement over what constitutes a violation. This ambiguity can lead to prolonged disputes and, possibly, legal action, creating a chilling effect on future collaborative projects. As the AI landscape rapidly changes, companies need to carefully consider how they incorporate these competitive development restrictions to balance protection of their interests with the need for a competitive ecosystem. Failure to do so can lead to problems for both the companies involved and potentially the AI field as a whole.
Competitive development restrictions and their associated timeframes can significantly impact how companies and individuals operate within the AI space. While many contracts focus on a timeframe of one to two years, some stretch to five or more. This can be particularly problematic in fields like AI, where breakthroughs happen rapidly. It can effectively stunt a person's or company's ability to capitalize on what they've learned or invent new things.
It's also noteworthy that the geographical scope of these restrictions isn't always aligned with the original agreement's geographical focus. Some clauses extend worldwide, which can cause complications for developers working on international projects or across various legal jurisdictions. It’s surprising how such constraints can impact what people can work on or even where they can work.
Additionally, developers sometimes don't realize that these restrictions can impact future work related to a project. Imagine a researcher who has honed a skill through working on a project only to find that their contract restricts them from applying similar knowledge in later ventures. It creates a strange paradox where learning itself can be stifled due to these agreements.
The legal enforceability of these clauses is also a factor, as courts often evaluate whether the restrictions are reasonable. Interestingly, there's no guarantee that the wording of a restriction is uniformly applied across regions. This inconsistency in enforcement can be confusing for everyone involved.
Another area of concern is the interpretation of "competitive" itself. The definition can fluctuate greatly. Some contracts explicitly define it in terms of specific industries or market segments, while others are much more broad, encompassing nearly any future AI development. This lack of clarity creates challenges in understanding the restrictions’ reach.
It gets even more complicated when you have situations with overlapping restrictions. If a developer has worked for two companies that both have restrictive clauses, it can create a "double jeopardy" situation, limiting the person's career path and options. I can imagine this would lead to some frustration for individuals trying to advance in their field.
Similar situations can arise if a developer is involved in multiple projects for different companies. If the projects are related, it's easy to imagine a developer unknowingly violating a clause, potentially leading to legal disputes. The danger here is that the sharing of knowledge, which is generally considered beneficial, can get tripped up.
A related issue is how employment terms intersect with competitive development restrictions. While a programmer might have left a job, they can still be bound by restrictive clauses that continue well beyond their employment period. This has a knock-on effect on the person’s job prospects and future career.
Beyond individual researchers, these restrictions can impede collaborative efforts between organizations. This is particularly relevant to the advancement of AI, where research benefits from the open sharing of ideas and results. Yet, developers may be hesitant to participate in collaborations out of fear of running afoul of a contract clause. This could ultimately slow the progress of AI as a whole.
Finally, startups can be particularly vulnerable to these limitations. Since they frequently rely on attracting talent from larger companies, it's not surprising they can find themselves at a disadvantage compared to established organizations when competing for talent. This is potentially detrimental, as startups are often at the forefront of innovation and often depend on agile talent. This creates a situation where competitive clauses can stifle the innovation capacity that defines a successful startup.
7 Critical Terms Often Overlooked in AI-Related NDAs A Contract Review Perspective - Model Retraining and Update Protocols During Partnership
When partners collaborate on AI projects, defining how models will be retrained and updated is essential for ensuring their ongoing effectiveness. This means establishing processes for incorporating new data to combat issues like model drift, where a model's performance degrades because the environment it operates in changes, and concept drift, where the relationship between input and output data changes. It also requires open communication with everyone involved about the resources needed for ongoing model upkeep.
Ideally, you'd have a well-defined plan for retraining, including when to automatically retrain (trigger-based) or at set intervals (scheduled). This approach ensures that models adapt to changing data landscapes and environments. Moreover, during retraining, it's important to think carefully about how the dataset will be managed, especially if it's being used in situations that are different than it was originally intended. Failing to give enough attention to these areas can lead to models that perform poorly, legal troubles, and damage to everyone's reputations.
When examining AI partnerships through the lens of NDAs, a few aspects of "Model Retraining and Update Protocols" often get overlooked, yet they're crucial for avoiding potential headaches. Here's a look at some unexpected complications engineers might encounter:
First, the specific retraining methods and update schedules can vary significantly across different AI models. This can be a major challenge when partners are integrating models that were built separately, potentially causing compatibility issues that weren't foreseen in the agreement.
Secondly, a surprising number of NDAs don't clearly define who actually owns the changes made during model retraining. In fact, many agreements completely skip this topic. This lack of clarity can quickly lead to conflicts over intellectual property if the parties involved have differing interpretations of how model updates impact ownership.
Furthermore, AI algorithms can become outmoded surprisingly fast due to the rapid pace of technological advancement. This raises a critical question: can the update protocols agreed upon in a partnership keep up with these changes? Or do they unintentionally put the brakes on innovation?
Another point often overlooked is the inconsistency in performance metrics across different retrained models. There's no universal standard for measuring a model's effectiveness after an update. As different organizations use varied benchmarks, this lack of standardization can complicate performance evaluations during partnerships and lead to disagreements on a model's overall effectiveness post-update.
Moreover, partnership-driven model updates could inadvertently create compliance issues with existing regulations, particularly in sectors with fast-evolving rules, like healthcare or finance. These compliance requirements often aren't accounted for in the model retraining details within agreements.
Another pitfall is that retraining with biased data can strengthen existing biases within a model, even unintentionally. This can have severe repercussions for an organization's reputation if the updated model's outputs reflect those biases, a risk many partnership protocols don't consider.
Furthermore, the retraining process can necessitate access to new external datasets that weren't covered in the initial agreement. This could leave companies scrambling to obtain the necessary rights to use those new datasets for future model updates.
Additionally, without well-structured feedback loops in a collaborative environment, the model retraining process might not capture the most recent insights, leading to suboptimal AI system performance. This delayed response to new knowledge can inadvertently limit the AI system's ability to perform.
When multiple stakeholders are involved in a partnership, a lack of standardized procedures for updating models can create conflicts. Each party might have different ideas about how and when updates should be made, resulting in operational friction that might not have been anticipated.
Finally, after a partnership contract ends, the legal standing of retrained models can become ambiguous. Many agreements fail to specify if the technology or updates remain subject to the initial contract, which might lead to unforeseen ownership disputes or unwanted liabilities.
These observations demonstrate the intricate nature of legal and practical aspects surrounding model retraining and partnership agreements, highlighting the need for meticulous attention to detail when crafting these contractual obligations.
More Posts from legalpdf.io: