eDiscovery, legal research and legal memo creation - ready to be sent to your counterparty? Get it done in a heartbeat with AI. (Get started for free)
7 AI-Driven Process Optimization Techniques for Continuous Business Improvement in 2024
7 AI-Driven Process Optimization Techniques for Continuous Business Improvement in 2024 - Real-Time Process Mapping Through Computer Vision Systems
Real-time process mapping using computer vision is a game-changer for businesses looking to refine and streamline their operations. AI-powered visual analysis offers a very precise way to follow production steps, quickly highlighting where things are slowing down or going wrong. This approach not only simplifies the process mapping itself but also keeps the maps consistently updated, leading to faster and more informed decisions. What's more, incorporating computer vision systems into various industries, particularly manufacturing, pushes businesses toward more proactive problem-solving. The real-time data insights let them spot potential issues before they become major headaches. The push toward Industry 4.0 is making computer vision even more important for improving operations, further cementing its role in the ongoing drive for constant business growth and efficiency.
However, it's worth noting that this approach still relies on the quality and context of the visual data it captures and analyzes. If the system isn't trained on a diverse enough range of process variations, its ability to offer truly useful insights can be limited. Additionally, managing and interpreting the large volumes of data generated can present its own challenges. Despite these caveats, the potential for real-time process mapping through computer vision to significantly improve business processes is clear, particularly for businesses that are trying to achieve a more agile and responsive operating model.
Using computer vision to map processes in real-time relies on sophisticated deep learning methods to recognize and classify the steps within complex operations. This approach has shown the potential to improve operational efficiency, with some studies indicating gains of up to 30%.
One of the key benefits is the capacity to pinpoint deviations from normal process execution in real-time. This allows companies to address inefficiencies or mistakes immediately, which can prevent disruptions and minimize wasted resources. Furthermore, employing object detection methods, computer vision can also provide insights into the physical environment and employee interactions, revealing ergonomic issues that may be impacting productivity.
The data captured through vision systems can be seamlessly integrated with existing software like enterprise resource planning (ERP) systems. This eliminates the need for manual updates to process maps, ensuring that the data is always current. Early research suggests that these systems can boost the accuracy of process metrics, potentially decreasing error rates by as much as 25% when compared to traditional methods.
The flexibility of computer vision makes it applicable across a wide range of sectors, from manufacturing to healthcare, making it a potentially versatile tool for process improvement. However, we've noticed that the performance of vision systems can be affected by cluttered environments. Clearly organized and uncluttered workspaces are crucial for optimal data quality.
Beyond efficiency improvements, real-time process mapping through computer vision plays a vital role in ensuring compliance with regulations. It enables companies to monitor adherence to standards without constant manual oversight. By analyzing historical data combined with real-time information, these systems can predict future behavior and anticipate potential workflow issues before they escalate, enabling proactive changes.
It's exciting that augmented reality (AR) can be incorporated with these vision systems. By overlaying process performance directly onto workstations, AR can improve visualizations. This capability can lead to quicker and more informed decision-making by operators and engineers. While the promise is great, we need to continue to critically evaluate how these systems perform in different contexts, and how we can effectively manage the data and ensure the system is robust, accurate and bias-free.
7 AI-Driven Process Optimization Techniques for Continuous Business Improvement in 2024 - Machine Learning Models for Supply Chain Flow Predictions
Machine learning models are becoming a core part of improving how efficiently supply chains operate. These models use complex algorithms to analyze massive amounts of data, helping businesses better predict demand and spot potential disruptions in their supply chains. This ability to forecast more accurately leads to more precise capacity planning. Some newer ways of applying machine learning, like what's called "optimal machine learning," are helping to overcome the shortcomings of older forecasting methods. This means businesses can better handle the unpredictable nature of supply chains. While the use of AI in this way offers great promise for better prediction, it's still important to address things like data quality and the difficulties that come with setting up and running these models. So, while machine learning is a powerful tool for streamlining supply chains, it's important to have a balanced view of its practical applications and limitations to achieve lasting improvement.
The use of AI, particularly machine learning, for predicting supply chain flows is attracting a lot of interest, especially as it relates to sustainability considerations in logistics. Techniques like deep learning are improving the accuracy of things like capacity planning and predicting future demand within supply chains. Incorporating non-linear relationships between variables in machine learning models for demand forecasting seems to boost predictive accuracy. Overall, AI can automate parts of the supply chain, forecast future needs, and highlight areas where things could be better.
A more recent idea, called optimal machine learning, addresses how some older planning methods make it difficult for companies to adapt to disruptions. A recent study looked at 119 papers from 2015 to 2024 on machine learning and deep learning models used to predict demand in supply chains. Researchers are increasingly focused on making sure the AI methods they're using for optimizing logistics align with sustainable practices.
Companies are using machine learning algorithms and analyzing large datasets to get a better understanding of their supply chain processes and make better decisions. The use of AI in predictive analytics helps organize and structure ways to make supply chains perform better and achieve continuous improvement. Sophisticated computing methods are being used more and more to tackle the difficult problems that come up in supply chain management, leveraging both AI and machine learning.
While the potential is certainly there, it's worth pointing out that supply chain data is often unevenly distributed, with some product categories far more prominent than others. This can skew the results of prediction models if not managed properly, potentially impacting the accuracy of forecasts and leading to incorrect allocation of resources. A key challenge is that many advanced AI models can be difficult to interpret. The complexity of these models can sometimes make it hard to figure out exactly why they're making certain predictions, which can be a barrier to adopting them in industries where transparency is crucial.
We're also seeing that a lot of supply chain data contains outliers caused by unpredictable things like natural disasters or market fluctuations. This is important because it can make these forecasting models less accurate unless we use specific techniques to address these situations. It's interesting to note how the Internet of Things (IoT) is helping us to get better predictions. Because IoT devices are constantly sending data from different parts of the supply chain, we can get a much more detailed understanding and improve our predictions. The quality of the data fed into these AI models is extremely important. Poor data leads to inaccurate predictions.
Despite these challenges, companies that successfully use machine learning models for supply chain forecasting often gain a significant edge over their competitors. They can make better decisions, leading to reduced operational costs and happier customers. It seems clear that the intersection of AI, machine learning, and supply chain management is an exciting and rapidly developing field, and continued research in this space is needed to address the remaining challenges and further unlock its vast potential.
7 AI-Driven Process Optimization Techniques for Continuous Business Improvement in 2024 - Natural Language Processing for Customer Service Automation
Natural Language Processing (NLP) has become a vital tool for automating customer interactions in customer service, enabling AI-powered chatbots and virtual assistants to provide quick, accurate responses that mimic human conversation. The growing need for automated customer service, particularly in industries focused on immediate responses, has fueled the adoption of NLP. By leveraging NLP, conversational AI can now grasp customer requests without needing human intervention, resulting in automated and timely solutions. Implementing AI in customer service relies heavily on machine learning and NLP to efficiently process, respond to, and resolve customer inquiries, optimizing the entire support process. This automation extends across various channels like voice calls, website chats, and social media, enriching customer interactions.
These NLP-enabled chatbots provide faster responses and more personalized experiences, enhancing customer satisfaction and freeing up human agents to handle more complex issues. Beyond quick responses, NLP is effective in understanding customer sentiment and recognizing their needs, resulting in better-targeted responses that elevate overall service quality. NLP has seen a significant jump in capabilities with the inclusion of transformer-based models, which have dramatically improved the automation within customer service, transforming traditional support systems. It's worth noting that technologies like Robotic Process Automation (RPA) and AI Workflow Engines are often incorporated alongside NLP in these automated customer service systems, improving the efficiency of service delivery.
It's expected that continuous improvements in customer service will involve ongoing development of AI and NLP technologies, continually boosting efficiency and driving higher customer satisfaction rates. However, it's essential to acknowledge that NLP's success relies on the quality and diversity of the data it's trained on, and there can be limitations when handling complex or nuanced queries. While the advancements are promising, critically evaluating the performance and limitations of NLP within evolving customer service contexts is crucial for long-term effectiveness and continued improvement.
Natural language processing (NLP) has become crucial for automating customer interactions in service settings, enabling AI-driven chatbots and virtual assistants to provide near-instant responses with a level of accuracy that mimics human communication. The demand for automated customer support solutions has spiked, especially in industries where fast response times are paramount. NLP allows conversational AI systems to decipher customer inquiries without human intervention, triggering quick automated responses. Implementing AI in customer service often means using machine learning and NLP to improve the support process by understanding, answering, and resolving queries efficiently.
AI-powered customer service can utilize a range of channels like voice, website chat, and social media, enhancing interactions. Chatbots, enhanced by NLP technology, deliver faster responses and personalized experiences, leading to greater customer satisfaction while freeing human agents for more complicated issues. NLP is quite effective at gauging customer sentiment, recognizing their needs, and creating relevant responses, thus upgrading the quality of service.
The rise of transformer-based models within NLP has greatly boosted the capabilities of automation within customer service, revolutionizing the traditional support model. Tools for customer service automation often rely on technologies like Robotic Process Automation (RPA) and AI workflow engines to optimize and streamline service delivery. Continuous advancements in AI and NLP are likely to be central to refining customer service procedures, paving the way for greater efficiency and heightened satisfaction.
However, it is important to acknowledge the limitations of the technology. Training these systems often requires large amounts of high-quality data, which can be a challenge for some businesses. The accuracy of the AI response is highly dependent on the training dataset, so there's always a risk of bias or unintended consequences if the data isn't diverse and representative. Moreover, while AI can be quite good at handling standard queries, more complex and nuanced requests might still require human intervention. Despite these limitations, NLP-powered automation within customer service holds great potential to provide customers with faster and more effective support while also boosting overall business efficiency.
The integration of these systems can be challenging, and businesses need to carefully consider how they will integrate NLP into their existing workflows and systems. And like other advanced AI technologies, the potential for misuse or for unintended biases within these systems needs to be continuously monitored and addressed. Yet, despite these challenges, NLP technology remains a promising field with potential for significant improvements within customer service. There is an increasing need to research methods to ensure these systems are unbiased, and that their responses are consistent with established ethical guidelines. As these AI models evolve, they need to become more adaptable and resilient in order to offer the most benefit to both customers and companies.
7 AI-Driven Process Optimization Techniques for Continuous Business Improvement in 2024 - Automated Quality Control Systems with Deep Learning Networks
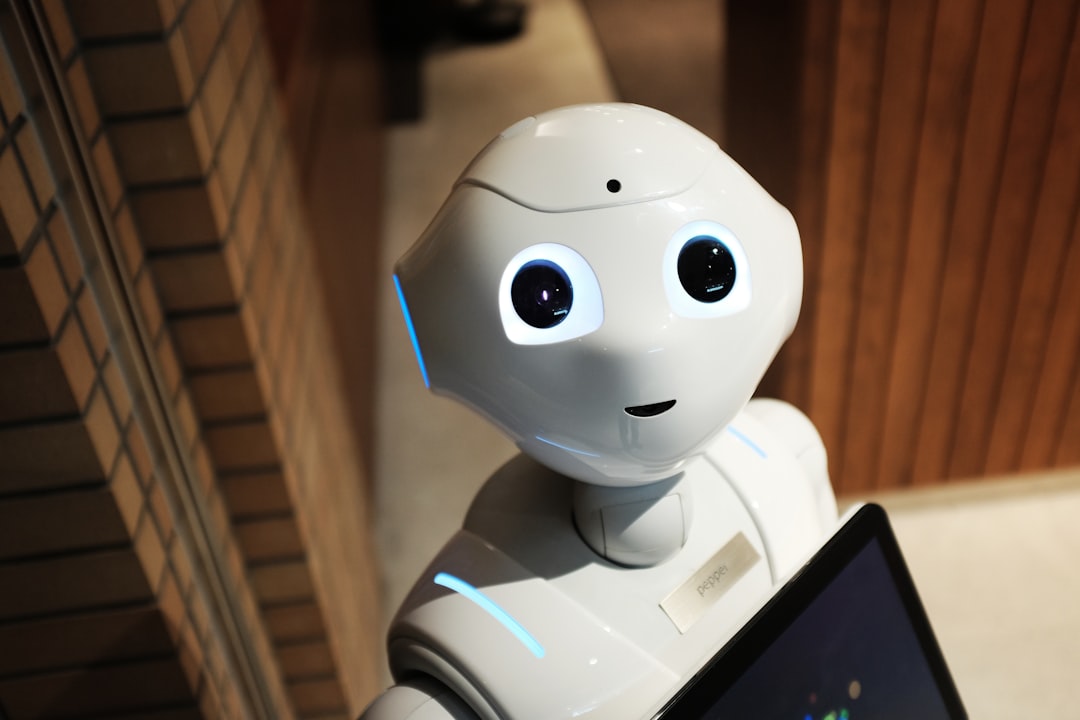
AI-powered quality control systems are increasingly using deep learning networks to automate the process of ensuring product quality and compliance. These systems are built on sophisticated AI algorithms that analyze data to evaluate product reliability and allow for immediate adjustments during production. As manufacturing embraces Quality 4.0, techniques like Learning Quality Control (LQC) are emerging as a way to improve existing quality management processes by making them more efficient and responsive to changes in the production environment. This shift involves incorporating human expertise into AI-driven quality control systems, often through a combination of approaches like inductive logic programming and convolutional neural networks. This results in a hybrid system that leverages both AI's speed and the context-specific knowledge of human inspectors. A key aspect of success for these systems is the need for high-quality data to enable accurate interpretations of various situations, ensuring that quality control is both efficient and reliable.
AI-powered quality control systems that utilize deep learning networks are becoming increasingly sophisticated, allowing for extremely sensitive defect detection. These systems can pinpoint defects as small as 0.1% of a product's expected value, ensuring even the slightest variations are caught. One of the interesting aspects of deep learning in this context is that it can achieve a high level of accuracy with less training data compared to traditional methods. Techniques like transfer learning enable models to learn from pre-trained networks, making it possible to implement quality control more rapidly. Moreover, these systems can automatically adjust production in real-time when they identify anomalies. This is a significant advantage as it enables immediate corrections and reduces waste, leading to more streamlined operations.
The use of AI in quality control can deliver substantial cost savings. For example, some companies have found their inspection costs can go down by up to 40%. This decrease is largely attributed to the fact that automated inspections are more consistent and less prone to errors than manual inspections, which can be subjective and dependent on human fatigue. Deep learning also helps when it comes to implementing quality control across several product lines. The algorithms can learn from one product category and apply it to others. This means businesses can adapt quality control procedures more quickly without having to develop a unique system for every new product they release.
Going beyond just visual data, these AI-based systems can process and combine information from different sources, such as images, sound, and sensor data. This integrated approach provides a more holistic view of the quality of a product, improving the decision-making process. An intriguing aspect of deep learning in quality control is that it can be leveraged for predictive maintenance. By analyzing past performance data, these systems can provide early warning signs of potential equipment failures. This means that maintenance can be planned more efficiently, minimizing disruptions to production.
Furthermore, these systems excel at detecting unusual patterns in production data, often identifying issues that more traditional systems would miss. The ability to learn from previously unidentified anomalies is valuable, offering a new level of insight into quality control. These AI systems constantly learn from the data they collect. This continuous feedback loop allows the systems to refine their algorithms over time, leading to ever-improving performance. In crisis situations, like a sudden drop in quality due to equipment malfunctions or supply chain issues, these deep learning models can help determine the root cause faster. This expedited root cause analysis is valuable in facilitating efficient corrective actions. While there are many benefits, it's always important to remember these AI systems are still under development and that we need to understand how to manage the data and minimize potential biases to make them effective and reliable.
7 AI-Driven Process Optimization Techniques for Continuous Business Improvement in 2024 - Predictive Maintenance Systems Using Sensor Data Analytics
Predictive maintenance systems, powered by sensor data analytics, are a significant step forward in extending the life of equipment and minimizing unplanned downtime. These systems employ data analytics and machine learning to anticipate potential equipment failures, moving away from reacting to problems towards a more preventative approach to maintenance. The use of the Internet of Things (IoT) allows for the real-time collection and analysis of equipment health data, which ultimately leads to more informed decisions about maintenance. But, relying on sensor data comes with challenges. Issues like noisy data and the interpretation of that data can lessen the reliability of the predictive models. Despite these hurdles, the use of AI within predictive maintenance is continuously being refined. This offers considerable potential for constant improvement within industrial operations as companies focus on both efficiency and dependable operations.
Predictive maintenance (PdM) uses data analysis and machine learning to anticipate equipment failures and fine-tune maintenance schedules. This proactive approach aims to minimize disruptions in industrial settings by preventing unplanned downtime. Compared to traditional maintenance strategies like preventive or corrective maintenance, AI-powered PdM systems are more accurate and efficient. They achieve this by leveraging sensor data, leading to a better understanding of equipment health. The emergence of the Internet of Things (IoT) has further enhanced PdM by allowing for continuous and real-time data capture and analysis, making it possible to monitor equipment condition constantly.
Interestingly, the foundation of many data-driven PdM systems lies in fuzzy logic systems. These systems translate raw sensor data into electronic signals that can be readily understood by computers, making maintenance decisions smoother and more accurate. However, a core question is how to best interpret this sensor data. There are generally three approaches to PdM in this era of Industry 4.0: data-based, knowledge-based, and physics-based. This variety in approaches reflects the challenges inherent in understanding complex machinery.
The successful implementation of PdM requires a robust process. This includes methods for gathering data, carefully choosing machine learning models that fit the specific context, and putting in place systems for continuous improvement. Beyond simple equipment monitoring, AI applications within PdM are increasingly diverse, covering predictive quality analysis, safety protocols, and even warranty management. The efficiency gains are evident. When you focus on maintaining equipment proactively rather than reactively, operational outcomes improve.
The combination of AI's advances and the rise of new manufacturing processes has broadened the adoption of PdM across various industries. It has pushed many businesses away from older, reactive maintenance methods. We can expect PdM systems to evolve further. These advances are intertwined with new developments in machine learning algorithms and improved data analytics tools. That said, it's important to note that while there is great potential, the accuracy of these predictions depends heavily on factors such as the sensors themselves, the calibration of the system, and the quality of the data collected. If these elements are not managed well, then the predictive power of the system may be compromised. It will be important in future research to identify ways to make these systems more robust and reliable.
7 AI-Driven Process Optimization Techniques for Continuous Business Improvement in 2024 - Digital Twin Integration with Machine Learning Algorithms
Combining digital twins with machine learning algorithms is a powerful new way to improve how businesses operate. Digital twins create virtual replicas of real-world assets, allowing us to use real-time data to make better choices and refine predictive models. For example, machine learning can make digital twins more precise, like helping predict how much energy a wind turbine will generate or improving production schedules by examining logistics data. As these systems become more sophisticated, collecting data throughout the whole product development cycle enhances the value of digital process twins. But there are still important challenges to overcome. Data quality can be an issue, and integrating machine learning into existing systems can be complex. These challenges are important to understand if we want to see successful results from these combined systems.
The merging of digital twins (DTs) with artificial intelligence (AI), specifically machine learning (ML) algorithms, is generating a new class of systems called AI-driven digital twins (AIDTs). These systems are proving quite useful for refining predictions and decisions, thanks to the rich data flowing from the digital twins. ML algorithms, by their nature, continually learn from data trends and refine their abilities to anticipate outcomes in new situations.
A compelling example is how digital twins are used to forecast wind turbine energy production. By applying ML techniques to wind turbine DTs, we can get a more accurate understanding of how much energy a given turbine will produce. This idea has inspired research on building digital twin frameworks that enhance production controls. These frameworks attempt to bring the physical factory and the virtual model together, frequently relying on the internet of things (IoT) and ML for seamless data flow.
For instance, there's research focused on combining production and logistics through integrated frameworks. These utilize ML-enabled DTs for the dynamic scheduling of manufacturing and for improving supply chain resilience. One hurdle is the need to guarantee that the ML models running within these AIDT frameworks are continuously refined and deployed effectively. A branch of AI called MLOps aims to address this very challenge, creating structures that decrease the chances of ML model failures in production environments.
Another research avenue explores using ML to manage the accumulation of data across the whole product development process. The goal is to establish improved digital process twins. Meanwhile, the role of DTs in preventative maintenance is being explored through the use of ML methods to enhance maintenance capabilities. The hope is to reduce unplanned shutdowns and increase system reliability.
Improving dynamic production scheduling is another area where DTs are being applied. By mimicking physical systems in virtual environments, businesses can see how changes in the production process ripple through the system. This adds real value for companies in manufacturing. To address the complexities of DT modelling, particularly where numerous decisions are made and resources are constrained, researchers are also employing techniques from data science, such as Constrained Mathematical Optimization. The ultimate aim is to improve the quality of solutions found using DT systems.
However, it's important to recognize that these systems are still evolving and there are remaining questions. While these early results are promising, we need to keep a close eye on how well these systems adapt to changing contexts. For example, we need to be especially aware of potential biases within ML algorithms. Furthermore, the sheer volume of data coming from these systems can be difficult to manage. It is still an exciting area of research, and we anticipate further refinement of these techniques as we develop a more complete understanding of their benefits and limitations.
7 AI-Driven Process Optimization Techniques for Continuous Business Improvement in 2024 - Process Mining with Advanced Pattern Recognition
Process mining, enhanced by advanced pattern recognition techniques, is gaining prominence as a method for optimizing business processes by unearthing hidden insights within operational data. The drive to refine process mining capabilities has led businesses to adopt tools incorporating generative AI and advanced analytics. These tools are vital for spotting inefficiencies and boosting overall operational performance. This combination not only speeds up the process of discovering patterns in how work gets done but also allows for making changes in real-time, supporting an ongoing commitment to improvement. Yet, while the potential of these technological integrations is substantial, businesses need to be mindful of the quality of the data they're using and potential biases embedded within algorithms, since these factors can limit the effectiveness of the implementation. As process mining tools continue to evolve, they will play a key role in defining the future of operational excellence for businesses navigating the complex world of business in 2024 and beyond.
Process mining, enhanced by advanced pattern recognition, is increasingly becoming a powerful tool for understanding and optimizing business operations. Researchers are finding that it goes far beyond just visualizing processes; it can unveil hidden insights that can drive significant improvements.
One interesting development is its ability to significantly boost anomaly detection. Studies have indicated that using advanced pattern recognition can improve the identification of unusual events in process logs by over 40% compared to older, rule-based methods. This allows businesses to quickly spot disruptions and take corrective actions, potentially minimizing downtime.
Furthermore, the capability to analyze process logs and uncover hidden variations has proven quite valuable. Process mining is revealing that up to 30% of delays can be attributed to unexpected variations in how processes are actually carried out. This kind of insight can inspire companies to re-think their standard operating procedures.
A notable trend is the ability to integrate data from various sources. By combining application logs, sensor data, and user interactions, process mining with advanced pattern recognition can provide a more holistic view of a process. Some studies suggest this multi-modal approach can improve process throughput by as much as 25%.
Another area where process mining is demonstrating its usefulness is in predictive maintenance. By analyzing usage patterns from process logs, it can now forecast maintenance needs with greater accuracy. This has led to reported cost reductions of up to 15% in some cases and also extends the lifespan of equipment.
In addition, advanced pattern recognition methods allow for the dynamic reallocation of resources. Businesses can now react to process insights in real-time, leading to more optimized workflows. This approach has shown potential to improve resource efficiency by up to 20%.
It's also interesting that process mining can help with compliance. It's becoming common to integrate compliance checks into existing workflows using process mining. This leads to a considerable decrease in compliance errors. Some researchers have even linked it to a decrease in audit findings by as much as 50%, which demonstrates its power for improving regulatory adherence.
Beyond that, advanced pattern recognition techniques can also streamline root cause analysis in more complicated processes. By automating some of the investigation tasks, it can cut down on the time it takes to find the root cause of a problem, enabling faster corrections and reducing the likelihood of the issue recurring. It's been shown to reduce investigation times by as much as 30%.
The ability to examine user interactions is another useful capability. Process mining can pinpoint abnormal user behavior related to inefficiencies within a process. This provides actionable insights for training programs and the refinement of system design.
Process mining systems are also getting better at correlating events in real-time. This capability allows them to catch process bottlenecks as they happen, leading to immediate changes in the process. Some researchers have found this can enhance overall productivity by 10-15%.
Lastly, researchers are experimenting with integrating advanced pattern recognition with simulation models. This allows us to forecast how changes to a process will affect outcomes. This approach can help businesses make better choices by reducing the need for experimentation, which can reduce costs by up to 20%.
While these results are exciting, it's important to acknowledge that process mining with advanced pattern recognition is still an active area of research. There are many challenges that still need to be addressed. These systems require good quality data, and they must be appropriately implemented and maintained to yield the desired results. But the promise is clear. As the capabilities of process mining continue to improve, it's likely to play an even greater role in how businesses operate and optimize their processes.
eDiscovery, legal research and legal memo creation - ready to be sent to your counterparty? Get it done in a heartbeat with AI. (Get started for free)
More Posts from legalpdf.io: